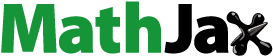
Abstract
Objectives: Previous studies have suggested a potential link between glycan levels and pancreatic cancer. To better characterize this relationship, we performed a study to evaluate the association between genetically predicted N-glycan levels in plasma and pancreatic cancer risk.
Methods: Using genetic variants identified to be associated with plasma N-glycan levels in large genome-wide association studies (GWAS) as instruments, we evaluated the associations between plasma N-glycans and pancreatic cancer risk by analyzing data from 8275 pancreatic cancer cases and 6723 controls.
Results: We observed that lower predicted PGP110 levels were significantly associated with an increased risk of pancreatic cancer after adjusting for multiple comparisons.
Conclusions: Our study did not support strong associations between human plasma N-glycans and the risk of pancreatic cancer, although the observed association with PGP110 warrants further investigation.
Simple summary
Objectives: Pancreatic cancer is a fatal malignancy. Therefore, there is an urgent need to better understand its risk factors and identify markers for better predicting individual risk of developing this cancer. Previous research has suggested that glycans may play a role in the development of pancreatic cancer. To better understand their relationship, we conducted a study to assess the associations between N-glycan levels predicted by genetic factors and pancreatic cancer risk. Methods: We used genetic factors related to blood N-glycan levels as instruments. We further evaluated the associations between plasma N-glycans and pancreatic cancer risk by analyzing data from 8275 pancreatic cancer patients and 6723 controls. Results: We only observed one association between predicted levels of PGP110 and pancreatic cancer risk. Conclusions: Our study did not support strong associations between N-glycan levels and pancreatic cancer risk, although the observed association with PGP110 can be further studied.
Introduction
Pancreatic cancer is the fourth leading cause of cancer-related deaths in the United States, with an estimated 5-year survival rate of only 8% (Siegel et al. Citation2021). Tobacco smoking, heavy alcohol consumption, obesity, chronic pancreatitis, type 2 diabetes, and a family history of pancreatic cancer are the few established risk factors for pancreatic cancer (Moore and Donahue Citation2019). Currently, no effective screening test is available for pancreatic cancer. There is an urgent need to better characterize the etiology and identify biomarkers for effective risk assessment.
There is growing evidence supporting the potentially important roles of glycans in carcinogenesis and cancer progression (Turovskaya et al. Citation2008; Ruhaak et al. Citation2016; Samraj et al. Citation2018; Munkley Citation2019; Liu et al. Citation2021). Glycans are known to regulate cancer proliferation, invasion, angiogenesis, and metastasis (Pinho and Reis Citation2015; Munkley Citation2019). Aberrant glycosylation is a hallmark of cancer (Munkley and Elliott Citation2016). Carboxylated glycans have been reported to play an essential role in inflammation-associated colon carcinogenesis (Doherty et al. Citation2018). A wide range of glycan alterations have been identified in pancreatic cancer, such as increased sialyl Lewis X antigen, truncated O-glycans (Tn and sTn), branched and fucosylated N-glycans, specific proteoglycans and galectins, and O-GlcNAcylation (Munkley Citation2019). Focusing on N-glycans, highly branched sialyted N-glycans were upregulated in Panc-1 and MIA PaCa-2 pancreatic cancer cells compared with normal HPDE pancreatic cells (Park et al. Citation2015). Furthermore, the level of high-mannose type glycans was higher among Capan-1 cells (pancreatic cancer cells that have metastasized to the liver) than among Panc-1 and MIA PaCa-2 cells (Park et al. Citation2015). Increased fucosylation was also reported in the serum of patients with pancreatic cancer (n = 23) compared to control subjects (n = 23) (Yue et al. Citation2009). Another recent study found that serum N-glycan monitoring might help in the early detection of pancreatic cancer in a pancreatic surveillance program (Levink et al. Citation2022). Due to the nature of the in vitro study and the limited sample sizes of studies focusing on human samples, it is unclear whether N-glycan levels are causally associated with pancreatic cancer risk. The direct profiling of N-glycans in prospective studies is challenging because of their complexity and high cost, resulting in limited power. Moreover, several biases exist in conventional epidemiological studies, such as selection bias, potential confounding, and reverse causation (Burgess et al. Citation2013; Wu et al. Citation2019; Ghoneim et al. Citation2020). An alternative design to characterize the relationship between exposures and outcomes would be to use genetic factors as instruments, similar to the Mendelian Randomization (MR) design (Shu et al. Citation2019; Ghoneim et al. Citation2020). To better characterize the associations between N-glycans and pancreatic cancer risk, we used a genetic instrument design, which can reduce several of these limitations and is highly cost efficient (Wu et al. Citation2019).
Materials and methods
Genetic instruments
Detailed procedures for determining the genetic instruments used are described elsewhere (Liu et al. Citation2021). To determine genetic variants associated with plasma levels of N-glycans, we conducted a literature search up to April 2020. Briefly, we first checked a collection of published GWAS with Glycomics included on the website http://www.metabolomix.com/a-table-of-all-published-gwas-with-glycomics/, which is curated by Dr. Karsten Suhre. Briefly, relevant GWAS with glycomics traits (glycGWAS) are included in this website. We also conducted literature search of the PubMed database using terms of (‘variants’ OR ‘single nucleotide polymorphisms’) AND ‘N-glycan’. We further checked the references of related review papers and consulted additional experts in the field. We focused on N-glycans released from all plasma proteins or a single protein that has been extensively studied: immunoglobulin G (IgG). Finally, we included the two largest and most comprehensive studies: one for total plasma N-glycome and the other for IgG N-glycome (Sharapov et al. Citation2019; Klarić et al. Citation2020). From each study, we determined the variant(s) associated with each glycan based on the stringent thresholds used in the studies. We only retained SNPs that were independent (R2 < 0.1, based on 1000 Genomes Project Phase 3 version 5 data for European descendants) of each other for each glycan as the genetic instrument. The SNPs used for N-glycan level prediction are generally independent of the disease of interest.
In a study by Klaric et al., data from four cohorts including CROATIA-Korcula, CROATIA-Vis, ORCADES (Orkney Complex Disease Study), and TwinsUK including 8090 samples from individuals of European ancestry were used to determine IgG N-glycan-associated variants (Klarić et al. Citation2020). To control for experimental variation in glycan measurements across involved studies, raw data of glycan levels were firstly total area normalized and batch corrected, by using the ‘ComBat’ function of ‘sva’ R package centrally (Leek Citation2014). Each glycan trait of interest was further rank transformed to fit a normal distribution. For the association analyses with 77 ultra-performance liquid chromatography (UPLC) IgG N-glycan traits, covariates including age, sex, study-specific covariates, and cryptic relatedness were corrected. The HapMap2 (release 22) imputed genetic data were analyzed, and P-value (≤ 2.40×10−9) (Bonferroni corrected for 21 independent glycan traits) was used to determine genome-wide significant associations (Lauc et al. Citation2013). The EGCUT, FINRISK, COGS, and SDRNT1BIO data were further used for replication analysis, and 19 of the 27 significant loci identified were replicated.
Sharapov et al. (Sharapov et al. Citation2019) conducted a genome-wide association study of human plasma N-glycome composition in up to 3811 subjects. Genotyping was conducted using TwinsUK samples with a combination of Illumina SNP arrays (HumanHap300, HumanHap610Q, 1 M-Duo, and 1.2MDuo 1 M) (Moayyeri et al. Citation2013). After standard quality control (QC), genotyped variants were imputed using 1000G stage phase 1 version 3 as reference data. UPLC was used to quantify the plasma N-glycome content of the samples. Harmonized glycan data were further normalized and batch-corrected (Akmačić et al. Citation2015). Briefly, total area normalization was used. Normalized glycan levels were log10-transformed. After removing samples with outlying measurements, the ComBat method was used to perform batch correction (Sharapov et al. Citation2019). After association tests between genetic variants and glycan levels, an association was determined to be significant if it reached a Bonferroni correction threshold of P-value < 1.66 × 10−9 (the full formula is alpha × 10−6/(29 + 1), here alpha = 0.05). Here, 29 is the estimated effective number of traits. Three other cohorts (QMDiab (Mook-Kanamori et al. Citation2014; Suhre et al. Citation2017) SOCCS (Theodoratou et al. Citation2016; Vučković et al. Citation2016), and PainOR (Allegri et al. Citation2016) were further used to replicate the identified significant associations. Genetic instruments for 138 N-glycan traits were determined and used for downstream analyses, as described in our previous publication (Liu et al. Citation2021).
To calculate the variation percentages, Klarić L et al. (Klarić et al. Citation2020) performed a conditional analysis on summary statistics from a discovery meta-analysis. For each independent SNPi, they calculated the proportion of explained phenotypic variance using the formula σi = 2*pi*qi*βi2. In addition, for every glycan, they estimated the total joint variance by summing the contribution of each independently contributing SNP, defined as σJi = 2*pi*qi*βiU*βiJ. Here, βi represents the effect estimate of SNPi in the univariate meta-analysis, and pi and qi represent the minor and major allele frequencies of SNPi calculated in the Generation Scotland cohort (Smith et al. Citation2013), respectively. βiU is the effect estimate of SNPi in the univariate analysis and βiJ is the joint effect estimate of the same SNP in the joint analysis. In Sharapov et al (Sharapov et al. Citation2019), the proportion of glycome trait variance explained by genetic instrumental variables (e.g. coefficient of determination) was estimated using genome-wide association studies (GWAS) summary statistics and expressed in percentages. The proportion of glycome trait variance explained was estimated using the following equation: R2 = T2/N, where R2 is the coefficient of determination, T2 = (beta/se)2 and N is the sample size, whereas beta is the affected size of the genetic instrumental variable on the glycan trait and se is its standard error.
Genetic association datasets of pancreatic cancer risk
For association analyses with pancreatic cancer risk, four large pancreatic cancer GWAS conducted in PanScan I, PanScan II, PanScan III, and PanC4, which were downloaded from dbGaP (Study Accession: phs000206.v5.p3 and phs000648.v1.p1), were used. Detailed information on these datasets, including the characteristics of the cases and controls, has been described elsewhere (Klein et al. Citation2018; Liu et al. Citation2020). Briefly, genotyping was performed on Illumina HumanHap550, 610-Quad, OmniExpress, and OmiExpressExome arrays. Standard QC was performed according to the guidelines recommended by the consortia (Liu et al. Citation2020). Only subjects of European ancestry, based on genetic estimation, were retained (Liu et al. Citation2021). The QCed data were pooled for the imputation. Genotype imputation was conducted with a reference panel of the Haplotype Reference Consortium (r1.1 2016) using Minimac3 after prephasing with SHAPEIT. SNPs with an imputation quality of at least 0.3 were retained. To control for population heterogeneity of the retained subjects, we carefully adjusted for top genetic principal components, in addition to age and sex, when assessing the associations between individual SNPs and pancreatic cancer risk (Liu et al. Citation2020; Zhu et al. Citation2020). Overall, 8275 pancreatic cancer patients and 6723 controls were included in the analyses.
Association analysis between genetically predicted N-glycan levels and pancreatic cancer risk
We used the inverse variance weighted (IVW) approach to estimate the association between genetically predicted N-glycan levels and pancreatic cancer risk (Wu et al. Citation2019). Briefly, the beta coefficient of the association between genetically predicted N-glycan levels and pancreatic cancer risk and the corresponding standard error were estimated using and
, respectively (Burgess et al. Citation2013; Zhu et al. Citation2021). Here, βi,GX represents the beta coefficient of the association between i ith variant and the glycan, βi,GY represents the beta coefficient of the association between i ith variant and pancreatic cancer risk, and σi,GY represents the standard error of the association between variant-pancreatic cancer risk. The association odds ratio (OR), confidence interval (CI), and P value were further estimated. Specifically, we estimated them using the formulas OR = exp (beta); CI ranges from exp (beta-1.96*standard error) to exp (beta+1.96*standard error), and Z = beta/standard error. P value was further estimated based on Z statistics. A Bonferroni-corrected threshold was used to determine significant associations. Based on the power calculation, the minimal detectable ORs per standard deviation of genetically predicted N-glycan levels ranged from 0.56 to 0.90, given 80% power at an alpha of 0.05 (Brion et al. Citation2013).
Results
The overall flow of the study is shown in Figure . We evaluated the associations between the genetically predicted levels of 138 N-glycan traits and pancreatic cancer risk. Among these traits, 68 reflected total plasma N-glycome features, and 70 reflected IgG N-glycome features. Detailed information on the instrument SNPs for N-glycan traits is shown in Supplementary Table 1 of our previously published study (Liu et al. Citation2021). The proportion of variation in plasma N-glycan levels explained by associated variants ranged from 0.57% to 20.32%. The smallest proportion of variation explained was for IGP27 (0.57%) and the largest proportion of variation explained was for IGP29 (20.32%).
The associations between the genetically predicted N-glycan levels and pancreatic cancer risk are shown in Table , Figures and . Among the tested N-glycans, we found that lower predicted levels of PGP110 were significantly associated with an increased risk of pancreatic cancer (OR = 0.57; 95% CI, 0.45-0.74; P = 1.92×10−5) after Bonferroni correction (Table and Figure ). The structure of PGP110 is shown in Figure .
Figure 2. Associations between genetically predicted levels of IGP glycans and pancreatic cancer risk.

Table 1. Associations between genetically predicted plasma N-glycan levels and pancreatic cancer risk.
Discussion
In the current study, using genetic instruments, we did observe a single strong association between the percentage of trisialylated and tetrasialylated tetragalactosylated glycan structures N – levels in plasma and pancreatic cancer risk. In general, this observation is in concordance with evidence from cell lines, animal experiments, and human studies. Changes in glycosylation, especially on the surface of cancer cells, have been demonstrated to affect the properties of already existing cancers (Demetriou et al. Citation1995; Yoshimura et al. Citation1995; Seberger and Chaney Citation1999; Granovsky et al. Citation2000; Zhao et al. Citation2008; Takahashi et al. Citation2009), and were correlated with the stage of cancer (Liu et al. Citation2021). Glycans in tumor sites are known to play important roles in cell signaling, cancer cell invasion, cell-matrix interactions, angiogenesis, metastasis, as well as immune modulation (Munkley and Elliott Citation2016). There is strong evidence supporting relevance of N-glycan patterns with cell-to-cell adhesion for tumor cells and stroma (Bellis et al. Citation2022; Stanley et al. Citation2022). Research also reported that disrupting N-glycan expression on the surface of cancer cells could boost chimeric antigen receptor T cell efficacy against pancreatic adenocarcinoma, lung, ovary, and bladder cancer (Greco et al. Citation2022). On the other hand, limited research has supported levels of glycosylation changes in blood to modify the risk of developing future cancer. Note, that genetically predicted glycan levels were used as instrumental variables to establish an association and direction of causality, but not as a proposed genetic test for pancreatic cancer. Such study requires another design, methodology, and data collection and it was out of the scope of the present manuscript.
Protein glycosylation is the most abundant post-translational modification, and is involved in cellular and organ homeostasis (Moremen et al. Citation2012). It can be affected by the development of specific diseases (Demetriou et al. Citation1995; Yoshimura et al. Citation1995; Seberger and Chaney Citation1999; Granovsky et al. Citation2000; Hakomori Citation2002; Zhao et al. Citation2008; Takahashi et al. Citation2009; Moremen et al. Citation2012; Brion et al. Citation2013; Taniguchi and Kizuka Citation2015; Liu et al. Citation2021; Zhu et al. Citation2021; Bellis et al. Citation2022; Greco et al. Citation2022; Stanley et al. Citation2022). Several studies have suggested that human serum glycome can potentially serve as cancer biomarkers (de Leoz et al. Citation2011; Hua et al. Citation2011a; Hua et al. Citation2011b; Hua et al. Citation2013). N-glycans have been suggested to play a major role in protein glycosylation (Stanley et al. Citation2009; Kizuka and Taniguchi Citation2016). In one retrospective case–control study, Zhao et al. reported that the diagnostic efficacy of pancreatic cancer can be improved by combining N-glycan markers and CA19-9 (Zhao et al. Citation2017). N-glycans have also been reported to be potentially related to prostate cancer (Ishikawa et al. Citation2017; Matsumoto et al. Citation2019; Wu et al. Citation2019), breast cancer (Guo et al. Citation2017; Sindhura et al. Citation2017; Terkelsen et al. Citation2018; Herrera et al. Citation2019; Peng et al. Citation2019), ovarian cancer (Zhang et al. Citation2014; Everest-Dass et al. Citation2016; Chen et al. Citation2017; Briggs et al. Citation2019), colorectal cancer (Sethi et al. Citation2016; Shinozaki et al. Citation2018), bladder cancer (Yang et al. Citation2015), and gastric cancer (Kodar et al. Citation2012; Liu et al. Citation2013). PGP110 (the percentage of trisialylated and tetrasialylated tetragalactosylated structures) is composed of four N-glycan structures, which are heavily galactosylated, sialylated with inclusion of fucosylation. In blood plasma, three of these structures are linked only to alpha-1 acid glycoprotein. This protein is secreted to the bloodstream by liver cells – hepatocytes (Timoshchuk et al. Citation2023). Two genetic loci are identified to be associated with PGP110 levels at genome-wide significance threshold. The first locus is marked by rs7310409 (chr12:121424861 (GRCh37)) and contains HNF1A gene encoding hepatic nuclear factor 1 alpha. The post-GWAS studies showed, that HNF1A gene regulates expression of fucosyltransferase – enzymes, such as FUT6 and FUT8, involved in the fucosylation of the proteins (Sharapov et al. Citation2019). The second locus is marked by rs7255720 (19:5828064 (GRCh37)). This locus contains FUT6 gene, encoding FucT-VI – fucosyltransferase enzyme, that transfers fucose residual to acceptors, including N-glycan part of N-glycoproteins. Because of its highly branched structure, PGP110 is thought to be related to inflammation; thus, it is anticipated that a positive association with pancreatic cancer should be observed. To explore whether chances may play a role in our identified association, we conducted a similar analysis to evaluate associations between predicted N-glycan levels and breast cancer risk (Michailidou et al. Citation2017; Timoshchuk et al. Citation2023). In this analysis, involving a much larger sample size (122,977 cases and 105,974 controls), none of the tested N-glycans showed a significant association (Supplementary Table 1). Future work on independent validation and/or functional characterization is warranted to better understand our association with pancreatic cancer risk.
Further work is needed to better characterize the exact relationship between N-glycans and pancreatic cancer. Specifically, glycan genetic instruments established in larger cohorts and larger pancreatic cancer GWAS datasets will provide improved power to understand these associations. In addition, a more careful design considering the different clinical and pathological features of pancreatic cancer would be meaningful to provide a comprehensive picture of the research question of interest. It would also be useful to incorporate family history information into analyses to differentiate glycans that play different roles in familial and sporadic pancreatic cancer. Furthermore, with an improved method of direct glycan profiling, conventional epidemiological studies assessing the overall glycan levels can provide additional insights into this relationship. It would also be meaningful to conduct analyses focusing on the N-glycan pathways. However, this is challenging for the current study, as there is no available research investigating the genetic associations with N-glycan pathways to date. Future work in this area will provide new opportunities for such analyses.
As the proportion of variance in N-glycan levels that can be explained by the summed association magnitudes of GWAS-identified loci is relatively high for at least a proportion of the assessed N-glycans, the instrumental variables used in this study are not weak. On the other hand, it is worth noting that the possibility of horizontal pleiotropy bias could not be excluded in this analysis. The current study should be viewed as preliminary, as no rigorous validation or secondary analyses were conducted owing to a lack of relevant resources/information. Further studies are required to better characterize the relationship between changes in N-glycosylation and pancreatic cancer risk.
Conclusions
Our agnostic study did not support strong associations between N-glycans and pancreatic cancer risk, although the detected association with PGP110 warrants further investigation. Further work is needed to better characterize the relationship between N-glycans and pancreatic cancer.
Contribution to the field
Pancreatic cancer is a fatal malignancy. There is an urgent need to better characterize the etiology and identify biomarkers for effective risk assessment. Previous studies have suggested a potential link between glycans and pancreatic cancer. To better characterize the relationship between N-glycans and pancreatic cancer risk, we performed a study to comprehensively characterize the associations between genetically predicted N-glycan levels and pancreatic cancer risk.
In the present study, we evaluated the associations between plasma N-glycans and pancreatic cancer risk by analyzing data from 8275 pancreatic cancer cases and 6723 controls, using genetic variants reported to be associated with plasma N-glycan levels in large genome-wide association studies as instruments. After careful analysis, we only observed a significant association between genetically predicted levels of PGP110 in the plasma and pancreatic cancer risk, after adjusting for multiple comparisons.
Author contributions
Study concept and design: LW conceived the project; JZ contributed to the study design; LW, DL, TZ, and SS contributed to the literature search and evaluation; DL, TZ, SS, and ET contributed to data extraction; TZ, DL, and LW contributed to data analysis; all authors contributed to result interpretation; DL, LW, TZ, JZ, and SS contributed to manuscript writing; ET contributed to revision of the manuscript; all authors have read and agreed to the final version of the manuscript.
Supplemental Material
Download MS Word (80.5 KB)Acknowledgements
We would like to thank Yurii Aulchenko, Azra Frkatović, and Gordan Lauc for their guidance and information for eligible relevant studies for consideration of this work. The pancreatic cancer GWAS datasets used for the analyses described in this manuscript were obtained from dbGaP at http://www.ncbi.nlm.nih.gov/sites/entrez?db=gap through dbGaP accession phs000206.v5.p3 and phs000648.v1.p1. The authors would like to thank all the individuals for their participation in the parent studies and all the researchers, clinicians, technicians, and administrative staff for their contribution to the studies. This study was supported by the University of Hawaii Cancer Centre. DL was supported by the Harbin Medical University Cancer Hospital Outstanding Youth Fund (JCQN-2021-04). The work of ET was supported by a grant from the Russian Science Foundation (RSF), No. 19-15-00115. The work of SS is an output of a research project implemented as part of the Research Program at the MSU Institute for Artificial Intelligence. The PanScan study was funded in whole or in part with federal funds from the National Cancer Institute (NCI), US National Institutes of Health (NIH), under contract number HHSN261200800001E. Additional support was received from NIH/NCI K07 CA140790, the American Society of Clinical Oncology Conquer Cancer Foundation, Howard Hughes Medical Institute, Lustgarten Foundation, Robert T., and the Judith B. Hale Fund for Pancreatic Cancer Research and Promises for Purple. A full list of acknowledgments for each participating study is provided in the Supplementary Note of the manuscript (PubMed ID:25086665). For the PanC4 GWAS study, patients and controls were derived from the following PANC4 studies: Johns Hopkins National Familial Pancreas Tumor Registry, Mayo Clinic Biospecimen Resource for Pancreas Research, Ontario Pancreas Cancer Study (OPCS), Yale University, MD Anderson Case Control Study, Queensland Pancreatic Cancer Study, University of California San Francisco Molecular Epidemiology of Pancreatic Cancer Study, International Agency of Cancer Research, and Memorial Sloan Kettering Cancer Center. This work was supported by NCI R01CA154823 Genotyping services were provided by the Center for Inherited Disease Research (CIDR). CIDR is fully funded through a federal contract from the National Institutes of Health to Johns Hopkins University (contract number HHSN2682011000111). The content is the sole responsibility of the authors and does not necessarily represent the official views of the National Institutes of Health.
Disclosure statement
No potential conflict of interest was reported by the author(s).
Data availability statement
The pancreatic cancer genetic data analyzed in the current study are available in the database of Genotypes and Phenotypes (dbGaP) at http://www.ncbi.nlm.nih.gov/sites/entrez?db=gap through dbGaP accession phs000206.v5.p3 and phs000648.v1.p1. The association results for all analyzed N-glycans are deposited to Zenodo and are publicly available (https://doi.org/10.5281/zenodo.6872744).
Additional information
Funding
References
- Akmačić IT, Ugrina I, Štambuk J, Gudelj I, Vučković F, Lauc G, Pučić-Baković M. 2015. High-throughput glycomics: optimization of sample preparation. Biochemistry (Mosc). 80(7):934–942. doi:10.1134/S0006297915070123.
- Allegri M, De Gregori M, Minella CE, Klersy C, Wang W, Sim M, Gieger C, Manz J, Pemberton IK, MacDougall J, et al. 2016. ‘Omics’ biomarkers associated with chronic low back pain: protocol of a retrospective longitudinal study. BMJ Open. 6(10):e012070. doi:10.1136/bmjopen-2016-012070.
- Bellis SL, Reis CA, Varki A, Kannagi R, Stanley P, Varki A, Cummings RD, Esko JD, Stanley P, Hart GW, et al. 2022. Glycosylation changes in cancer. In: Essentials of glycobiology [internet]. 4th edition. Cold Spring Harbor, NY: Cold Spring Harbor Laboratory Press; Chapter 47.
- Briggs MT, Condina MR, Klingler-Hoffmann M, Arentz G, Everest-Dass AV, Kaur G, Oehler MK, Packer NH, Hoffmann P. 2019. Translating N-glycan analytical applications into clinical strategies for ovarian cancer. Proteomics Clin Appl. 13(3):1800099. doi:10.1002/prca.201800099.
- Brion MJ, Shakhbazov K, Visscher PM. 2013. Calculating statistical power in Mendelian randomization studies. Int J Epidemiol. 42:1497–1501. doi:10.1093/ije/dyt179.
- Burgess S, Butterworth A, Thompson SG. 2013. Mendelian randomization analysis with multiple genetic variants using summarized data. Genet Epidemiol. 37(7):658–665. doi:10.1002/gepi.21758.
- Chen H, Deng Z, Huang C, Wu H, Zhao X, Li Y. 2017. Mass spectrometric profiling reveals association of N-glycan patterns with epithelial ovarian cancer progression. Tumor Biol. 39(7):1010428317716249.
- de Leoz ML, Young LJ, An HJ, Kronewitter SR, Kim J, Miyamoto S, Borowsky AD, Chew HK, Lebrilla CB. 2011. High-mannose glycans are elevated during breast cancer progression. Mol Cell Proteomics. 10(1):M110.002717. doi:10.1074/mcp.M110.002717.
- Demetriou M, Nabi IR, Coppolino M, Dedhar S, Dennis JW. 1995. Reduced contact-inhibition and substratum adhesion in epithelial cells expressing GlcNAc-transferase V. J. Cell Biol. 130(2):383–392. doi:10.1083/jcb.130.2.383.
- Doherty M, Theodoratou E, Walsh I, Adamczyk B, Stöckmann H, Agakov F, Timofeeva M, Trbojević-Akmačić I, Vučković F, Duffy F, et al. 2018. Plasma N-glycans in colorectal cancer risk. Sci Rep. 8(1):8655. doi:10.1038/s41598-018-26805-7.
- Everest-Dass AV, Briggs MT, Kaur G, Oehler MK, Hoffmann P, Packer NH. 2016. N-glycan MALDI imaging mass spectrometry on formalin-fixed paraffin-embedded tissue enables the delineation of ovarian cancer tissues. Mol Cell Proteomics. 15(9):3003–3016. doi:10.1074/mcp.M116.059816.
- Ghoneim DH, Zhu J, Zheng W, Long J, Murff HJ, Ye F, Setiawan VW, Wilkens LR, Khankari NK, Haycock P, et al. 2020. Mendelian randomization analysis of n-6 polyunsaturated fatty acid levels and pancreatic cancer risk. Cancer Epidemiol Biomarkers Prev. 29(12):2735–2739. doi:10.1158/1055-9965.EPI-20-0651.
- Granovsky M, Fata J, Pawling J, Muller WJ, Khokha R, Dennis JW. 2000. Suppression of tumor growth and metastasis in Mgat5-deficient mice. Nat. Med. 6(3):306–312. doi:10.1038/73163.
- Greco B, Malacarne V, De Girardi F, Scotti GM, Manfredi F, Angelino E, Sirini C, Camisa B, Falcone L, Moresco MA, et al. 2022. Disrupting N-glycan expression on tumor cells boosts chimeric antigen receptor T cell efficacy against solid malignancies. Sci Transl Med. 14(628):eabg3072. doi:10.1126/scitranslmed.abg3072.
- Guo J, Liu C, Zhou X, Xu X, Deng L, Li X, Guan F. 2017. Conditioned medium from malignant breast cancer cells induces an emt-like phenotype and an altered N-glycan profile in normal epithelial MCF10A cells. Int J Mol Sci. 18(8):1528. doi:10.3390/ijms18081528.
- Hakomori S. 2002. Glycosylation defining cancer malignancy: new wine in an old bottle. Proc Natl Acad Sci USA. 99(16):10231–10233. doi:10.1073/pnas.172380699.
- Herrera H, Dilday T, Uber A, Scott D, Zambrano JN, Wang M, Angel PM, Mehta AS, Drake RR, Hill EG, et al. 2019. Core-fucosylated tetra-antennary N-glycan containing A single N-acetyllactosamine branch Is associated with poor survival outcome in breast cancer. Int J Mol Sci. 20(10):2528. doi:10.3390/ijms20102528.
- Hua S, An HJ, Ozcan S, Ro GS, Soares S, DeVere-White R, Lebrilla CB. 2011a. Comprehensive native glycan profiling with isomer separation and quantitation for the discovery of cancer biomarkers. Analyst. 136(18):3663–3671. doi:10.1039/c1an15093f.
- Hua S, Lebrilla C, An HJ. 2011b. Application of nano-LC-based glycomics towards biomarker discovery. Bioanalysis. 3(22):2573–2585. doi:10.4155/bio.11.263.
- Hua S, Williams CC, Dimapasoc LM, Ro GS, Ozcan S, Miyamoto S, Lebrilla CB. 2013. Isomer-specific chromatographic profiling yields highly sensitive and specific potential N-glycan biomarkers for epithelial ovarian cancer. J Chromatogr A. 1279:58–67. doi:10.1016/j.chroma.2012.12.079.
- Ishikawa T, Yoneyama T, Tobisawa Y, Hatakeyama S, Kurosawa T, Nakamura K, Narita S, Mitsuzuka K, Duivenvoorden W, Pinthus JH, et al. 2017. An automated micro-total immunoassay system for measuring cancer-associated α2, 3-linked sialyl N-glycan-carrying prostate-specific antigen may improve the accuracy of prostate cancer diagnosis. Int J Mol Sci. 18(2):470. doi:10.3390/ijms18020470.
- Kizuka Y, Taniguchi N. 2016. Enzymes for N-glycan branching and their genetic and nongenetic regulation in cancer. Biomolecules. 6(2):25. doi:10.3390/biom6020025.
- Klarić L, Tsepilov YA, Stanton CM, Mangino M, Sikka TT, Esko T, Pakhomov E, Salo P, Deelen J, McGurnaghan SJ, et al. 2020. Glycosylation of immunoglobulin G is regulated by a large network of genes pleiotropic with inflammatory diseases. Sci Adv. 6(8):eaax0301. doi:10.1126/sciadv.aax0301.
- Klein AP, Wolpin BM, Risch HA, Stolzenberg-Solomon RZ, Mocci E, Zhang M, et al. 2018. Genome-wide meta-analysis identifies five new susceptibility loci for pancreatic cancer. Nat Commun. 9(1):1–11. doi:10.1038/s41467-017-02088-w.
- Kodar K, Stadlmann J, Klaamas K, Sergeyev B, Kurtenkov O. 2012. Immunoglobulin G Fc N-glycan profiling in patients with gastric cancer by LC-ESI-MS: relation to tumor progression and survival. Glycoconj J. 29(1):57–66. doi:10.1007/s10719-011-9364-z.
- Lauc G, Huffman JE, Pučić M, Zgaga L, Adamczyk B, Mužinić A, Novokmet M, Polašek O, Gornik O, Krištić J, et al. 2013. Loci associated with N-glycosylation of human immunoglobulin G show pleiotropy with autoimmune diseases and haematological cancers. PLoS Genet. 9(1):e1003225. doi:10.1371/journal.pgen.1003225.
- Leek JT. 2014. svaseq: removing batch effects and other unwanted noise from sequencing data. Nucleic Acids Res. 42(21):e161. doi:10.1093/nar/gku864.
- Levink IJM, Klatte DCF, Hanna-Sawires RG, Vreeker GCM, Ibrahim IS, van der Burgt YEM, Overbeek KA, Koopmann BDM, Cahen DL, Fuhler GM, et al. 2022. Longitudinal changes of serum protein N-glycan levels for earlier detection of pancreatic cancer in high-risk individuals. Pancreatology. 22(4):497–506. doi:10.1016/j.pan.2022.03.021.
- Liu D, Zhou D, Sun Y, Zhu J, Ghoneim D, Wu C, Yao Q, Gamazon ER, Cox NJ, Wu L. 2020. A transcriptome-wide association study identifies candidate susceptibility genes for pancreatic cancer risk. Cancer Res. 80(20):4346–4354. doi:10.1158/0008-5472.CAN-20-1353.
- Liu D, Zhu J, Zhao T, Sharapov S, Tiys E, Wu L. 2021. Associations between genetically predicted plasma N-glycans and prostate cancer risk: analysis of over 140,000 European descendants. Pharmgenomics Pers Med. 14:1211–1220.
- Liu L, Yan B, Huang J, Gu Q, Wang L, Fang M, Jiao J, Yue X. 2013. The identification and characterization of novel N-glycan-based biomarkers in gastric cancer. PloS one. 8(10):e77821. doi:10.1371/journal.pone.0077821.
- Matsumoto T, Hatakeyama S, Yoneyama T, Tobisawa Y, Ishibashi Y, Yamamoto H, Yoneyama T, Hashimoto Y, Ito H, Nishimura SI, et al. 2019. Serum N-glycan profiling is a potential biomarker for castration-resistant prostate cancer. Sci Rep. 9(1):1–8. doi:10.1038/s41598-018-37186-2.
- Michailidou K, Lindström S, Dennis J, Beesley J, Hui S, Kar S, Lemaçon A, Soucy P, Glubb D, Rostamianfar A, et al. 2017. Association analysis identifies 65 new breast cancer risk loci. Nature. 551(7678):92–94. doi:10.1038/nature24284.
- Moayyeri A, Hammond CJ, Hart DJ, Spector TD. 2013. The UK Adult Twin Registry (TwinsUK Resource). Twin Res Hum Genet. 16(1):144–149. doi:10.1017/thg.2012.89.
- Mook-Kanamori DO, Selim MM, Takiddin AH, Al-Homsi H, Al-Mahmoud KA, Al-Obaidli A, Zirie MA, Rowe J, Yousri NA, Karoly ED, et al. 2014. 1,5-Anhydroglucitol in saliva is a noninvasive marker of short-term glycemic control. J Clin Endocrinol Metab. 99(3):E479–E483. doi:10.1210/jc.2013-3596.
- Moore A, Donahue T. 2019. Pancreatic cancer. Jama. 322(14):1426. doi:10.1001/jama.2019.14699.
- Moremen KW, Tiemeyer M, Nairn AV. 2012. Vertebrate protein glycosylation: diversity, synthesis and function. Nat Rev Mol Cell Biol. 13(7):448–462. doi:10.1038/nrm3383.
- Munkley J. 2019. The glycosylation landscape of pancreatic cancer. Oncol Lett. 17(3):2569–2575.
- Munkley J, Elliott DJ. 2016. Hallmarks of glycosylation in cancer. Oncotarget. 7:35478–35489. doi:10.18632/oncotarget.8155.
- Park HM, Hwang MP, Kim YW, Kim KJ, Jin JM, Kim YH, Yang YH, Lee KH, Kim YG. 2015. Mass spectrometry-based N-linked glycomic profiling as a means for tracking pancreatic cancer metastasis. Carbohydr Res. 413:5–11. doi:10.1016/j.carres.2015.04.019.
- Peng W, Goli M, Mirzaei P, Mechref Y. 2019. Revealing the biological attributes of N-glycan isomers in breast cancer brain metastasis using porous graphitic carbon (PGC) liquid chromatography-tandem mass spectrometry (LC-MS/MS). J Proteome Res. 18(10):3731–3740. doi:10.1021/acs.jproteome.9b00429.
- Pinho SS, Reis CA. 2015. Glycosylation in cancer: mechanisms and clinical implications. Nat Rev Cancer. 15(9):540–555. doi:10.1038/nrc3982.
- Ruhaak LR, Stroble C, Dai J, Barnett M, Taguchi A, Goodman GE, Miyamoto S, Gandara D, Feng Z, Lebrilla CB, et al. 2016. Serum glycans as risk markers for non-small cell lung cancer. Cancer Prev Res (Phila). 9(4):317–323. doi:10.1158/1940-6207.CAPR-15-0033.
- Samraj AN, Bertrand KA, Luben R, Khedri Z, Yu H, Nguyen D, Gregg CJ, Diaz SL, Sawyer S, Chen X, et al. 2018. Polyclonal human antibodies against glycans bearing red meat-derived non-human sialic acid N-glycolylneuraminic acid are stable, reproducible, complex and vary between individuals: total antibody levels are associated with colorectal cancer risk. PLoS One. 13(6):e0197464. doi:10.1371/journal.pone.0197464.
- Seberger PJ, Chaney WG. 1999. Control of metastasis by Asn-linked, β1-6 branched oligosaccharides in mouse mammary cancer cells. Glycobiology. 9(3):235–241. doi:10.1093/glycob/9.3.235.
- Sethi MK, Hancock WS, Fanayan S. 2016. Identifying N-glycan biomarkers in colorectal cancer by mass spectrometry. Acc Chem Res. 49(10):2099–2106. doi:10.1021/acs.accounts.6b00193.
- Sharapov SZ, Tsepilov YA, Klaric L, Mangino M, Thareja G, Shadrina AS, Simurina M, Dagostino C, Dmitrieva J, Vilaj M, et al. 2019. Defining the genetic control of human blood plasma N-glycome using genome-wide association study. Hum Mol Genet. 28(12):2062–2077.
- Shinozaki E, Tanabe K, Akiyoshi T, Tsuchida T, Miyazaki Y, Kojima N, Igarashi M, Ueno M, Suenaga M, Mizunuma N, et al. 2018. Serum leucine-rich alpha-2-glycoprotein-1 with fucosylated triantennary N-glycan: a novel colorectal cancer marker. BMC Cancer. 18(1):406. doi:10.1186/s12885-018-4252-6.
- Shu X, Wu L, Khankari NK, Shu XO, Wang TJ, Michailidou K, Bolla MK, Wang Q, Dennis J, Milne RL, et al. 2019. Associations of obesity and circulating insulin and glucose with breast cancer risk: a Mendelian randomization analysis. Int J Epidemiol. 48(3):795–806. doi:10.1093/ije/dyy201.
- Siegel RL, Miller KD, Fuchs HE, Jemal A. 2021. Cancer statistics, 2021. CA Cancer J Clin. 71(1):7–33. doi:10.3322/caac.21654.
- Sindhura B, Hegde P, Chachadi VB, Inamdar SR, Swamy BM. 2017. High mannose N-glycan binding lectin from Remusatia vivipara (RVL) limits cell growth, motility and invasiveness of human breast cancer cells. Biomed Pharmacother. 93:654–665. doi:10.1016/j.biopha.2017.06.081.
- Smith BH, Campbell A, Linksted P, Fitzpatrick B, Jackson C, Kerr SM, Deary IJ, Macintyre DJ, Campbell H, McGilchrist M, et al. 2013. Cohort profile: generation Scotland: Scottish Family Health Study (GS:SFHS). The study, its participants and their potential for genetic research on health and illness. Int J Epidemiol. 42(3):689–700. doi:10.1093/ije/dys084.
- Stanley P, Moremen KW, Lewis NE, Taniguchi N, Aebi M, Varki A, Cummings RD, Esko JD, Stanley P, Hart GW, et al. 2022. N-Glycans. In: Essentials of glycobiology [internet]. 4th edition. Cold Spring Harbor, NY: Cold Spring Harbor Laboratory Press; Chapter 9.
- Stanley P, Schachter H, Taniguchi N. 2009. N-glycans. In: Varki A, Cummings RD, Esko JD, Freeze HH, Stanley P, Bertozzi CR, Hart GW, Etzler ME, editor. Essentials of glycobiology. 2nd edition. Cold Spring Harbor, NY: Cold Spring Harbor Laboratory Press.
- Suhre K, Arnold M, Bhagwat AM, Cotton RJ, Engelke R, Raffler J, Sarwath H, Thareja G, Wahl A, DeLisle RK, et al. 2017. Connecting genetic risk to disease end points through the human blood plasma proteome. Nat Commun. 8:14357.
- Takahashi M, Kuroki Y, Ohtsubo K, Taniguchi N. 2009. Core fucose and bisecting GlcNAc, the direct modifiers of the N-glycan core: their functions and target proteins. Carbohydr Res. 344(12):1387–1390. doi:10.1016/j.carres.2009.04.031.
- Taniguchi N, Kizuka Y. 2015. Glycans and cancer: role of N-glycans in cancer biomarker, progression and metastasis, and therapeutics. Adv Cancer Res. 126:11–51. doi:10.1016/bs.acr.2014.11.001.
- Terkelsen T, Haakensen VD, Saldova R, Gromov P, Hansen MK, Stöckmann H, Lingjaerde OC, Børresen-Dale AL, Papaleo E, Helland Å, et al. 2018. N-glycan signatures identified n tumor interstitial fluid and serum of breast cancer patients: association with tumor biology and clinical outcome. Mol Oncol. 12(6):972–990. doi:10.1002/1878-0261.12312.
- Theodoratou E, Thaçi K, Agakov F, Timofeeva MN, Štambuk J, Pučić-Baković M, Vučković F, Orchard P, Agakova A, Din FV, et al. 2016. Glycosylation of plasma IgG in colorectal cancer prognosis. Sci Rep. 6:28098. doi:10.1038/srep28098.
- Timoshchuk A, Sharapov S, Aulchenko YS. 2023. Twelve years of genome-wide association studies of human protein N-glycosylation. Engineering. S2095-8099(23)00201-1. doi:10.1016/j.eng.2023.03.013.
- Turovskaya O, Foell D, Sinha P, Vogl T, Newlin R, Nayak J, Nguyen M, Olsson A, Nawroth PP, Bierhaus A, et al. 2008. RAGE, carboxylated glycans and S100A8/A9 play essential roles in colitis-associated carcinogenesis. Carcinogenesis. 29(10):2035–2043. doi:10.1093/carcin/bgn188.
- Vučković F, Theodoratou E, Thaçi K, Timofeeva M, Vojta A, Štambuk J, Pučić-Bakovic M, Rudd PM, ,z--Derek L, Servis D, et al. 2016. Igg glycome in colorectal cancer. Clin Cancer Res. 22(12):3078–3086. doi:10.1158/1078-0432.CCR-15-1867.
- Wu L, Shi W, Long J, Guo X, Michailidou K, Beesley J, Bolla MK, Shu XO, Lu Y, Cai Q, et al. 2018. A transcriptome-wide association study of 229,000 women identifies new candidate susceptibility genes for breast cancer. Nat Genet. 50(7):968–978. doi:10.1038/s41588-018-0132-x.
- Wu L, Shu X, Bao J, Guo X, Kote-Jarai Z, Haiman CA, Eeles RA, Zheng W, PRACTICAL, CRUK, BPC3, CAPS, PEGASUS Consortia et al. 2019. Analysis of over 140,000 European descendants identifies genetically predicted blood protein biomarkers associated with prostate cancer risk. Cancer Res. 79(18):4592–4598. doi:10.1158/0008-5472.CAN-18-3997.
- Yang G, Tan Z, Lu W, Guo J, Yu H, Yu J, Sun C, Qi X, Li Z, Guan F. et al. 2015. Quantitative glycome analysis of N-glycan patterns in bladder cancer vs normal bladder cells using an integrated strategy. J Proteome Res. 14(2):639–653. doi:10.1021/pr5006026.
- Yoshimura M, Nishikawa A, Ihara Y, Taniguchi S, Taniguchi N. 1995. Suppression of lung metastasis of B16 mouse melanoma by N-acetylglucosaminyltransferase III gene transfection. Proc. Natl Acad. Sci. 92(19):8754–8758. doi:10.1073/pnas.92.19.8754.
- Yue T, Goldstein IJ, Hollingsworth MA, Kaul K, Brand RE, Haab BB. 2009. The prevalence and nature of glycan alterations on specific proteins in pancreatic cancer patients revealed using antibody-lectin sandwich arrays. Mol Cell Proteomics. 8(7):1697–1707. doi:10.1074/mcp.M900135-MCP200.
- Zhang X, Wang Y, Qian Y, Wu X, Zhang Z, Liu X, Zhao R, Zhou L, Ruan Y, Xu J, et al. 2014. Discovery of specific metastasis-related N-glycan alterations in epithelial ovarian cancer based on quantitative glycomics. PloS one. 9(2):e87978. doi:10.1371/journal.pone.0087978.
- Zhao Y, Sato Y, Isaji T, Fukuda T, Matsumoto A, Miyoshi E, Gu J, Taniguchi N. 2008. Branched N-glycans regulate the biological functions of integrins and cadherins. FEBS J. 275(9):1939–1948. doi:10.1111/j.1742-4658.2008.06346.x.
- Zhao YP, Zhou PT, Ji WP, Wang H, Fang M, Wang MM, et al. 2017. Validation of N-glycan markers that improve the performance of CA19-9 in pancreatic cancer. Clin Exp Med. 17(1):9–18. doi:10.1007/s10238-015-0401-2.
- Zhu J, Shu X, Guo X, Liu D, Bao J, Milne RL, Giles GG, Wu C, Du M, White E, et al. 2020. Associations between genetically predicted blood protein biomarkers and pancreatic cancer risk. Cancer Epidemiol Biomarkers Prev. 29(7):1501–1508. doi:10.1158/1055-9965.EPI-20-0091.
- Zhu J, Wu C, Wu L. 2021. Associations between genetically predicted protein levels and COVID-19 severity. J Infect Dis. 223(1):19–22. doi:10.1093/infdis/jiaa660