Abstract
This study aimed to identify hub genes and drugs for ovarian cancer (OC) using bioinformatics analysis. Gene expression data and clinical information were downloaded from public databases. Differentially expressed genes (DEGs) were identified, and ovarian disease-related genes were extracted from DISEASES database. Disease-associated DEGs were further screened. Functional enrichment, protein–protein interaction (PPI) analysis, drug prediction, and survival analysis were performed. Key gene-drug interactions were also predicted. Finally, hub genes expression in an OC cell line (SKOV3) was verified using real-time quantitative reverse transcription polymerase chain reaction (RT-qPCR). Totally 354 overlapping DEGs were identified. PPI network revealed genes such as CCL5 and CXCR4 with higher degrees, which were mainly involved in chemokine-related functions. CCND1, CCNE1, CXCL10, ERBB4, LPAR3, and SST were correlated with prognosis of OC patients. Among them, CXCL10 was considered as an independent prognostic factor. Moreover, 63 possible drugs were predicted and the sirolimus-DCN pair was screened via a literature search. RT-qPCR confirmed that the levels of PDGFRB, DCN, PDGFRA, LYN, and CENPE were consistent with the bioinformatics analysis results. Our findings provide new insights into the underlying mechanisms of OC pathogenesis. The identified hub genes and drugs may improve individualized OC diagnosis and therapy.
Introduction
Ovarian cancer (OC) has the highest mortality rate among female genital tract cancers (Torre et al. Citation2018). Although the therapeutic effects of surgery and chemotherapy have improved owing to advancements in medical technology, clinical therapeutic results are still not ideal (Brackmann et al. Citation2018). Early diagnosis of OC is anticipated to substantially improve survival rates compared to cases of late diagnosis (Chatterjee et al. Citation2017). Therefore, it is necessary to identify the molecular mechanisms of OC development and metastasis.
Molecular genetic studies have shown that mutations in tumor suppressor genes, such as BRCA1, are responsible for the majority of hereditary OCs (Holschneider and Berek Citation2000). A recent study showed that certain differentially expressed genes (DEGs), such as TRAF4, contribute to metastasis and poor prognosis (Javdan et al. Citation2018). In a bioinformatics study, DEGs, including CCND1 and CCNB2, were found to be involved in several pathways related to OC development (Fu and Wang Citation2013), such as the cell cycle, drug metabolism, and drug resistance (Richardson and Kaye Citation2005). A previous gene expression network analysis revealed that securitine inhibits the expression of its target gene, STAT3, which contributes to OC progression (Chen et al. Citation2017). Despite such studies, the complex mechanisms associated with OC progression are not yet fully understood.
The Cancer Genome Atlas (TCGA) contains data describing the molecular characteristics of over 20,000 primary cancers and is widely used in cancer research (Wang et al. Citation2016). Most previous studies have analyzed data from a single database, which does not reflect the complex regulatory mechanisms of OC. Therefore, multiple databases must be integrated to identify potential biomarkers and therapeutic targets for OC. In this study, gene expression data of OC tumors and normal samples were downloaded from the University of California Santa Cruz Xena (UCSC-Xena) platform. Furthermore, ovarian disease-related genes were screened using the DISEASES database. Next, these genes were intersected with DEGs, and overlapping DEGs were selected for further analysis. Subsequently, a series of bioinformatics analyses, including functional enrichment analysis, protein–protein interaction (PPI) analysis, survival analysis, and drug prediction, were performed to identify key genes and drugs. The expression levels of hub genes in human OC cell lines were determined through quantitative reverse transcription real-time polymerase chain reaction (qRT-PCR). The objective of this study was to investigate the detailed molecular mechanisms underlying OC progression and explore potential drugs for OC therapy.
Material and methods
Microarray data
RNA sequencing data were downloaded from the ‘UCSC Toil RNAseq Recompute’ Data Hub (https://toil.xenahubs.net) through the UCSC-Xena platform (https://xena.ucsc.edu/) (Goldman et al. Citation2020). RNA-seq expression data of 19,131 patients were downloaded from https://xenabrowser.net/datapages/?data set=TcgaTargetGtex_rsem_gene_tpm&host=https%3A%2F%2Ftoil.xxenahub.net&removeHub=https%3A%2F%2Fxena.treehouse.gi.ucsc.edu%3A443. Their corresponding clinical information was collected from https://xenabrowser.net/datapages/?dataset=TcgaTar-getGTEX_phenotype.txt&host=https%3A%2F%2Ft-oil.xexenahu.net&removeHub=https%3A%2F%2Fxe-na.treehouse.gi.ucsc.edu%3A443. Subsequently, the TCGA-OV samples with detailed_category set to ‘Ovarian Serous Cystadenocarcinoma’ were extracted and 427 cases with tumors were obtained. The GTEx samples with detailed_category set to ‘Ovary’ were extracted and 88 normal samples were obtained. The clinicopathological characteristics of these patients are presented in Supplementary Table 1.
Based on the gene annotation information (hg38) from the GENCODE database (https://www.gencode-genes.org/) (Harrow et al. Citation2012), 60,498 Ensembl IDs were converted to gene symbols. Genes with low expression levels were filtered out, and genes with expression levels > 0 in over 1/3 of samples were retained. Finally, a total of 16,746 genes were identified.
Identification of DEGs between OC and normal samples
Linear regression and empirical Bayesian methods (Quadrianto and Buntine Citation2016; Koop Citation2017) in the R limma package (version 3.10.3, http://www.biocon-ductor.org/packages/2.9/bioc/html/limma.html) (Smyth Citation2015) were used to analyze the DEGs between the tumor and normal groups. P-values were corrected using the Benjamini-Hochberg method. An adjusted P-value < 0.05 and |log2 fold change (FC)| > 2 were selected as the thresholds for DEG screening. Volcano plots were constructed using ggplot2 (version 3.2.1).
Screening of disease-related genes
Genes related to ovarian diseases including Brenner tumors, OC, and ovarian carcinosarcoma were screened from the DISEASES database (https://diseases.jensenlab.org/Search) (Pletscher-Frankild et al. Citation2015) using ‘ovarian’ as the keyword. Disease-related genes were integrated with the DEGs, and overlapping DEGs were selected for further analysis.
Functional enrichment analysis
Gene Ontology-Biological Process (GO-BP) (Ashburner et al. Citation2000) and Kyoto Encyclopedia of Genes and Genomes (KEGG) pathway (Kanehisa and Goto Citation2000) enrichment analyses of the overlapping DEGs were performed using the Metascape online tool (http://metascape.org) (Zhou et al. Citation2019). Only terms with P-value < 0.01, a minimum count of 3, and an enrichment factor > 1.5 were considered significant. Clustering analysis was conducted according to the similarity of genes enriched in each term (similarity > 0.3). The most statistically significant terms (those with minimum P-values) were selected and used to construct the cluster. The top 20 clusters based on P-values were visualized using a histogram.
To further explore the relationships among terms, an interaction network of the top 20 clusters was constructed using Cytoscape (version 3.4.0, http://chianti.ucsd.edu/cytoscape-3.4.0/) (Shannon et al. Citation2003). Terms were included in the network only if they had the minimum P-value in their cluster and similarity > 0.3.
PPI network and module construction
PPI pairs of identified DEGs were identified using three databases in Metascape software (Zhou et al. Citation2019), namely BioGrid (Chatr-Aryamontri et al. Citation2017), InWeb_IM (Li et al. Citation2017), and OmniPath (Türei et al. Citation2016), with default parameters (Min Network Size: 3; Max Network Size: 500). After obtaining pairs, PPI networks were constructed using Cytoscape software (version 3.4.0) (Shannon et al. Citation2003).
Significantly enriched modules from the PPI network were identified using Molecular Complex Detection (MCODE; version 1.5.1) (Bader and Hogue Citation2003). GO-BP and KEGG analyses of genes in the modules were performed using Metascape (Zhou et al. Citation2019).
Drug-gene interaction and drug-related pathway analysis
Drug-gene pairs were predicted using the Drug-Gene Interaction database (DGIdb version 2.0; http://www.dgidb.org/search_interactions) (Cotto et al. Citation2018), and the drug-gene network was established using Cytoscape software (Shannon et al. Citation2003).
Additionally, the online database DrugPath (http://www.cuilab.cn/drugpath) (Shah et al. Citation2014) was used to search for KEGG pathways affected by the drugs. The drug-pathway network was constructed using Cytoscape software (Shannon et al. Citation2003). The three-dimensional (3D) structures and PubChem IDs of the predicted drugs were downloaded from PubChem (https://pubchem.ncbi.nlm.nih.gov/).
Survival analysis of potential genes
Nodes in both the PPI modules and drug-gene network were selected as potential genes. The expression levels of the genes were analyzed using Gene Expression Profiling Interactive Analysis 2 (GEPIA2) (http://gepia2.cancer-pku.cn/#index) (Tang et al. Citation2019). The prognostic value of each gene was assessed using the Survival Analysis package with the quartile grouping method, and the samples were divided into different groups based on gene expression. Briefly, the top and bottom 25% were defined as high– and low–expression groups, respectively. Differences in overall survival (OS) and disease-free survival (DFS) between the two groups were compared using Kaplan – Meier survival curve analysis (Bland and Altman Citation1998), and statistical significance was set at P-value < 0.05.
Univariate and multivariate Cox regression analyses were performed to assess whether the OS-related genes were independent prognostic factors. The following clinicopathological variables were considered: age, clinical stage, neoplasm histological grade, postoperative_rx_tx, and response to primary therapy. Statistical significance was set at P-value < 0.05.
Literature research and protein expression verification
Among the candidate genes, key genes were further screened from the National Center for Biotechnology Information (NCBI) PubMed database (https://pub-med.ncbi.nlm.nih.gov/) using Biopython (Cock et al. Citation2009) with the keyword ‘gene symbol + ovarian cancer’. Genes reported in more than three and less than 10 articles were considered hub genes. The Human Protein Atlas (HPA; https://www.proteinatlas.org/) (Uhlén et al. Citation2015) was used to verify the protein expression levels of key genes.
Investigation of key drug-gene interactions
The key genes and their corresponding target drugs were extracted. Briefly, the drugs were identified from the NCBI PubMed database using Biopython (Cock et al. Citation2009) with ‘drug + ovarian cancer’ as keywords. To narrow down the results, drugs reported in more than three and less than 10 studies were regarded as key drugs. Finally, the obtained drugs and their target genes were considered key drug-gene interactions.
Cell culture
The human normal ovarian cell line IOSE80 and human OC cell line SKOV3 were purchased from the Cell Bank of the Chinese Academy of Sciences (Shanghai, China). The cells were cultured in 1640 medium (Cuisi Bio, Shanghai, China) containing 10% fetal bovine serum (Gibco, Shanghai, China) in a 37°C incubator with 5% CO2.
qRT-PCR
Total RNA was extracted from SKOV3 and IOSE80 cells using TRIzol reagent (TaKaRa Bio, Beijing, China) and RNA purification and integrity were assessed using an Agilent Bioanalyzer (Santa Clara, CA, USA). RNAs were then reverse-transcribed into complementary DNA (cDNA) using a Reverse Transcription kit (TOYOBO, Shanghai, China), and qRT-PCR was performed using SYBR Green PCR mix (Thermo Fisher Scientific, Waltham, USA) on an ABI 7900HT FAST Real-Time PCR System (Applied Biosystems, Foster City, CA, USA). (Glyceraldehyde-3-phosphate dehydrogenase) GADPH was used as an internal control and the relative mRNA expression levels were calculated using the 2−ΔΔCT method. The following primers were used: platelet-derived growth factor receptor, beta polypeptide (PDGFRB, forward (F): 5’-AGCACCTTCGTTCTGACCTG-3’; reverse (R): 5’-TATTCTCCCGTGTCTAGCCCA-3’), decorin (DCN, F: 5’-ATGAAGGCCACTATCATCC-TCC-3’; R: 5’-GTCGCGGTCATCAGGAACTT-3’), platelet-derived growth factor receptor, alpha polypeptide (PDGFRA, F: 5’-TGGCAGTACCCCATGTCT-GAA-3’; R: 5’-CCAAGACCGTCACAAAAAGGC-3’), aurora kinase B (AURKB, F: 5’-CAGTGGGACACCC-GACATC-3’; R: 5’-GTACACGTTTCCAAACTT-GCC-3’), v-yes-1 Yamaguchi sarcoma viral related oncogene homolog (LYN, F: 5’-GCTTTTGGCACCA-GGAAATAGC-3’; R: 5’-GCTTTTGGCACCAGGAA-ATAGC-3’), centromere protein E (CENPE, F: 5’-GATTCTGCCATACAAGGCTACAA-3’; R: 5’-GAT-TCTGCCATACAAGGCTACAA-3’), and GAPDH (F: 5’-GGAGCGAGATCCCTCCAAAAT-3’; R: 5’-GGC-TGTTGTCATACTTCTCATGG-3’).
Statistical analysis
The qRT-PCR data were analyzed using GraphPad Prism v.8.0.2 (GraphPad Software, San Diego, CA) and reported as mean ± standard deviation. A two-tailed t-test was performed to compare the values between the normal and tumor groups. Statistical significance was set at P-value < 0.05.
Results
Identification of DEGs
A total of 3987 DEGs (2031 upregulated and 1956 downregulated) were identified between the tumor and normal groups (Figure (A)). Furthermore, 1442 ovarian disease-related genes were obtained from the DISEASES database. Subsequently, 354 overlapping DEGs were selected for further analyses (Figure (B)).
Functional enrichment analysis of DEGs
DEGs were significantly enriched in 319 GO-BP terms and 20 KEGG pathways. The top 20 enriched categories are shown in Figure (A). Briefly, genes were mainly enriched in GO-BP terms such as negative regulation of cell cycle, regulation of protein kinase activity, and negative regulation of cell proliferation. DEGs were mainly enriched in pathways such as cell cycle and pathways in cancer. Furthermore, a network based on the top 20 enriched GO terms and KEGG pathways was constructed to observe the interactions among terms (Figure (B)). The terms were divided into different clusters, indicating potential close connections between different biological functions during OC pathogenesis.
Figure 2. Functional enrichment analysis of DEGs. (A) Top 20 enriched Gene Ontology (GO) terms and Kyoto Encyclopedia of Genes and Genomes (KEGG) pathways. (B) Network of enriched GO terms and KEGG pathways. Different node colors indicate different clusters, and the lines indicate similarities among genes annotated with each term.
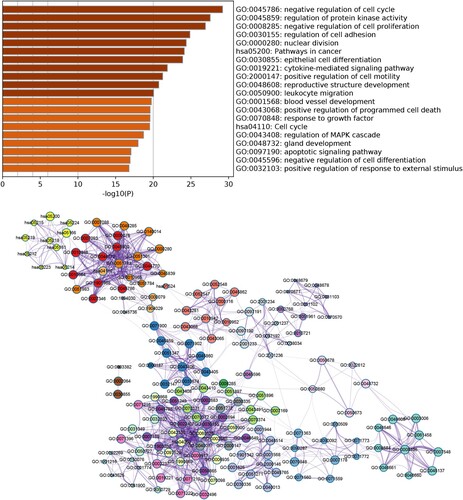
PPI network and module analysis
A PPI network composed of 1134 edges and 292 nodes was constructed (Figure ). Ten modules were selected from the PPI network based on MCODE analysis (Figure S1), and functional enrichment analysis was performed for the genes in these modules. Several genes, including chemokine (C-X-C motif) ligand 10 (CXCL10), chemokine (C–C motif) ligand 5 (CCL5) and chemokine (C-X-C motif) receptor 4 (CXCR4), were mainly enriched in GO-BP terms such as chemokine-mediated signaling, cellular response to chemokines, and response to chemokines. The genes in the modules were mainly enriched in KEGG pathways, such as cell cycle, pathways in cancer, and proteoglycans in cancer. The top three enriched functions and pathways are listed in Table .
Figure 3. Protein-protein interaction (PPI) network. Different colors indicate the different modules identified using Molecular Complex Detection (MCODE). Triangles and inverted triangles indicate the upregulated and downregulated genes, respectively. Node size indicates the degree of connectivity, and gray lines represent interactions between proteins.
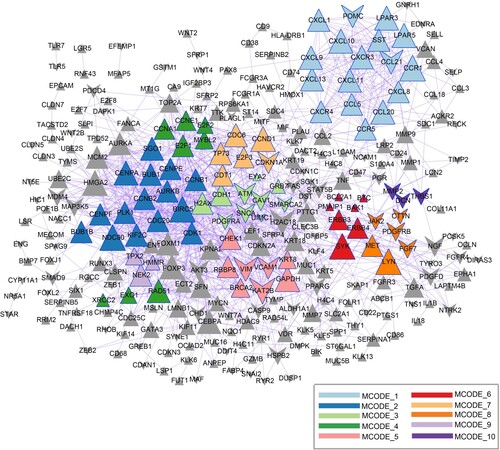
Table 1. The GO-BP function and KEGG pathway of DEGs in 10 modules extracted from PPI network.
Drug target prediction and drug pathway analysis
Based on the 354 DEGs, the online DGIdb database was used to predict the potential drugs. A total of 202 drug-gene interactions were identified, involving 70 DEGs and 63 drugs. The detailed results are shown in Figure (A). To further understand the pathways affected by the identified drugs, the online database, DrugPath, was used to search for KEGG pathways. As shown in Figure (B), the drug pathway network included 69 KEGG pathways (including the chemokine signaling pathway) and 16 drugs (including sirolimus). The 3D structures and corresponding PubChem IDs of the 16 drugs are shown in Figure S2.
Figure 4. Gene-drug interaction network (A) and drug-pathway network (B). (A) Red triangles indicate upregulated genes; green inverted triangles indicate downregulated genes; gray rectangles indicate predicted drugs. (B) Yellow squares indicate KEGG pathways and white squares indicate predicted drugs.
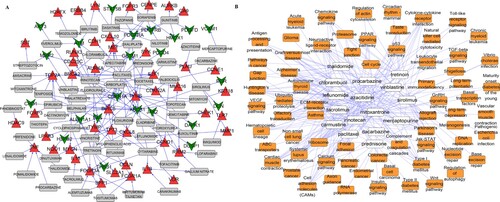
Survival analysis of potential genes
In total, 27 potential genes were identified after overlaying the genes in the PPI modules and the drug-target network. GEPIA2 was used to analyze the expression levels of 27 genes, which were consistent with the RNA-seq results (Figure S3). Furthermore, survival analysis showed that six genes (cyclin D1 (CCND1), cyclin E1 (CCNE1), CXCL10, v-erb-b2 avian erythroblastic leukemia viral oncogene homolog 4 (ERBB4), lysophosphatidic acid receptor 3 (LPAR3), and somatostatin (SST)) were significantly correlated with OS, and two genes (ERBB4 and LPAR3) were significantly associated with DFS (Figure ).
Figure 5. Survival analysis of key genes in OC using Kaplan – Meier analysis. (A) CCND1, (B) CCNE1, (C) CXCL10, (D) ERBB4, (E) LPAR3, (F) SST, (G) ERBB4, and (H) LPAR3. Patients were split into high and low expression groups based on the median expression of genes.
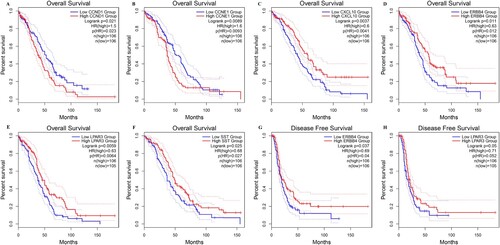
Moreover, OS-related genes and clinical variables, including age, clinical stage, neoplasm histologic grade, postoperative_rx_tx, and primary therapy response, were analyzed using univariate and multivariate Cox regression analyses. In the univariate analysis, age, clinical stage, postoperative_rx_tx, primary therapy response, and four genes (SST, CXCL10, ERBB4, and LPAR3) were associated with clinical outcomes (P < 0.05; Figure S4). In the multivariate analysis, only age, primary therapy response, and CXCL10 levels were considered independent prognostic factors (P < 0.05).
Literature review and protein level verification
Following a literature review, 11 key genes meeting the screening criteria were identified. The protein levels of the genes were verified using the HPA database. The results revealed that the protein expression levels of PDGFRB, DCN, and PDGFRA were downregulated in OC tissues, whereas those of AURKB, LYN, and CENPE were upregulated (Figure ).
Identification of key drug-gene interactions
Among the six gene-targeted drugs, only sirolimus met the literature search criteria. By combining the results of the identified key genes and drugs, sirolimus-DCN was identified as a key drug-target pair.
Experimental verification of six key mRNAs
To verify the results of the bioinformatics analysis, qRT-PCR was performed on the six key nodes. Excluding AURKB, the expression of the remaining five genes differed significantly between normal and cancer cells (P < 0.05; Figure ). Briefly, PDGFRB, DCN, and PDGFRA mRNA were downregulated, whereas LYN and CENPE were upregulated in the OC cell line SKOV3, consistent with our bioinformatics analysis results.
Discussion
Although the expression of certain genes may contribute to OC progression, the details of the underlying molecular mechanisms remain unclear. A total of 354 DEGs were identified between tumor and normal samples. PPI analysis showed that genes such as CCL5 and CXCR4 with higher degrees, which were mainly enriched in chemokine-associated functions. Moreover, 63 drugs were predicted using DGIdb. Survival analysis showed that CCND1, CCNE1, CXCL10, ERBB4, LPAR3, and SST were correlated with OS, and ERBB4 and LPAR3 were associated with DFS. Among them, CXCL10 expression was considered an independent prognostic factor. Moreover, the protein and mRNA levels of PDGFRB, DCN, LYN, PDGFRA, and CENPE were consistent with the results of the bioinformatics analysis. Finally, sirolimus-DCN was selected as the key drug-gene pair.
CCL5 is a chemokine-encoding gene that promotes cancer cell migration under several pathological conditions (Liou et al. Citation2012), and has been widely used as a clinical prognostic factor to predict cancer progression (Yaalhahoshen et al. Citation2006). Notably, CCL5 expression is closely associated with OC invasion and migration (Long et al. Citation2012), and OC development has been reported to be influenced by the CCL5-triggered epithelial–mesenchymal transition (Long et al. Citation2015). CXCR4 is a prognostic marker in various types of cancers, including OC (Jiang et al. Citation2006), and the overexpression of CXCR4, which plays an important role in cancer-associated signaling, promotes metastasis (Obermajer et al. Citation2011). In an animal model, CXCR4 inhibition prolonged survival in mice with OC (Righi et al. Citation2011). Although CCL5 and CXCR4 have been detected in a large number of solid tumors (Weitzenfeld and Benbaruch Citation2014), the detailed molecular mechanisms whereby these genes influence OC progression remain unclear.
The biological functions of DEGs in OC are realized via certain mechanisms and signaling pathways (Zhou et al. Citation2016). A previous study showed that chemokine (C–C motif) ligand 2 (CCL2)/chemokine (C–C motif) receptor 2 (CCR2) influences the survival and motility of breast cancer cells via the chemokine signaling pathway (Fang et al. Citation2012). Studies on the chemokine signaling system in the OC microenvironment have contributed to the development of anti-angiogenic therapies (Agarwal et al. Citation2010). In the present study, we found that CCL5 and CXCR4 were enriched in chemokine-associated biological functions. Therefore, we speculated that CCL5 and CXCR4 may participate in OC progression via chemokine-associated functions.
Drug resistance and effectiveness affect the success of chemotherapy (Norouzi-Barough et al. Citation2018). Although various drugs, such as cisplatin, are widely used in the clinical treatment of OC, drug resistance prevents their widespread clinical use (Samuel et al. Citation2018). Thus, we screened for potential drugs for use in OC treatment. Sirolimus (also called rapamycin) is a class I mammalian target of rapamycin (mTOR) inhibitor that exhibits antitumor activity and has been approved for breast cancer treatment (Pintoleite et al. Citation2009; Acevedogadea et al. Citation2015). The efficacy and safety of sirolimus for the treatment of OC have been demonstrated in previous studies (Jiang and Feng Citation2004; Stippel et al. Citation2005). Additionally, rapamycin can block growth differentiation factor 15 (GDF-15)-mediated invasion of human ovarian tumor tissues by inhibiting mTOR-matrix metalloproteinases (MMP) signaling (Griner et al. Citation2013). In this study, we found that DCN was a target for sirolimus. DCN encodes a member of the leucine-rich proteoglycan family and is involved in the regulation of various important cellular functions, such as matrix assembly, cell proliferation, and migration (Halari et al. Citation2021). Binding of this protein to several cell surface receptors can mediate its tumor-suppressive effects, including the inhibition of angiogenesis and tumorigenesis. Recent studies have shown that DCN is downregulated in OC tissues and can inhibit growth of OC cells (Nash et al. Citation2002). DCN is also associated with the upregulation of TNFα and downregulation of VEGF, suggesting that it may play a key role in OC angiogenesis and tumor progression (Grisaru et al. Citation2007). These studies have revealed that DCN may be a tumor suppressor and a potential biomarker of OC. However, the relationship between DCN and sirolimus in OC has not been reported. Therefore, whether sirolimus exerts a therapeutic effect on OC by regulating the expression of DCN remains to be confirmed.
CXCL10 has been widely used as a prognostic indicator of the progression of various diseases (Jiang et al. Citation2010; Bronger et al. Citation2016). A previous study showed that CXCL10 participates in the development of ovarian tumor cells (Rainczuk et al. Citation2014). In an animal model, CXCL10 altered the OC tumor immune microenvironment (Au et al. Citation2017) and could be used to predict survival in patients with advanced serous OC (Bronger et al. Citation2016), which is consistent with our findings. Thus, we speculate that CXCL10 may be a novel prognostic marker for OC.
The drug-gene interactions explored in the present study may provide insights into novel targets and therapeutic drugs associated with the development and metastasis of OC; however, this study has several limitations. Although there are different types of OC, the TCGA database does not provide a detailed OC classification; therefore, OC type was not considered in this analysis. We plan to include patients with different types of OC in future studies. Moreover, drug-gene interactions, such as sirolimus-DCN, were obtained via bioinformatics analyses, and the results need to be verified via additional experiments.
Conclusion
In summary, key genes and potential drugs associated with OC were identified using bioinformatics analyses. These key genes, such as DCN, may serve as reliable molecular biomarkers for OC diagnosis, and predicted drugs, such as sirolimus, may serve as potential drugs for OC treatment. Further research is required to explore the biological functions of these genes and the mechanisms underlying OC pathogenesis.
Author contributions
Conceptualization and design of the research: JS and XZ; acquisition of data: ZS, JC, and DW; analysis and interpretation of data: ZS, JC, and DW; statistical analysis: ZS, JC, and DW; funding acquisition: JS; drafting of the manuscript: XZ; revision of the manuscript for important intellectual content: JS. All authors have read and approved the final version of the manuscript.
Supplemental Table
Download MS Word (21.9 KB)Supplemental Figures
Download Zip (6.5 MB)Disclosure statement
No potential conflict of interest was reported by the author(s).
Data availability statement
The data were derived from the following resources available in the public domain: the UCSC Xena database (https://xena.ucsc.edu/), GENCODE database (https://www.gencodegenes.org/), and DISEASES (https://diseases.jensenlab.org/Search). The qRT-PCR data in this study are available in 4TU. ResearchData under DOI: https://doi.org/10.4121/22267030 at https://doi.org/10.4121/22267030.
Additional information
Funding
References
- Acevedogadea C, Hatzis C, Chung G, Fishbach N, Lezongeyda K, Zelterman D, Digiovanna MP, Harris L, Abukhalaf MM. 2015. Trastuzumab, sirolimus, HER2. Breast Cancer. 150:157–167.
- Agarwal A, Tressel SL, Kaimal R, Balla M, Lam FH, Covic L, Kuliopulos A. 2010. Identification of a metalloprotease-chemokine signaling system in the ovarian cancer microenvironment: implications for antiangiogenic therapy. Cancer Res. 70(14):5880–5890. doi: 10.1158/0008-5472.CAN-09-4341.
- Ashburner M, Ball CA, Blake JA, Botstein D, Butler H, Cherry JM, Davis AP, Dolinski K, Dwight SS, Eppig JT, et al. 2000. Gene ontology: tool for the unification of biology. Nat Genet. 25(1):25–29. doi: 10.1038/75556.
- Au KK, Peterson N, Truesdell P, Reid-Schachter G, Khalaj K, Ren R, Francis J-A, Graham CH, Craig AW, Koti M. 2017. CXCL10 alters the tumour immune microenvironment and disease progression in a syngeneic murine model of high-grade serous ovarian cancer. Gynecol Oncol. 145(1):436–445. doi: 10.1016/j.ygyno.2017.03.007.
- Bader GD, Hogue CW. 2003. An automated method for finding molecular complexes in large protein interaction networks. BMC Bioinform. 4(1):2. doi: 10.1186/1471-2105-4-2.
- Bland JM, Altman DG. 1998. Statistics notes: survival probabilities (the Kaplan-Meier method). Br Med J. 317(7172):1572–1580. doi: 10.1136/bmj.317.7172.1572.
- Brackmann M, Stasenko M, Uppal S, Erba J, Reynolds RK, McLean K. 2018. Comparison of first-line chemotherapy regimens for ovarian2 carcinosarcoma: a single institution case series and review of the literature. BMC Cancer. 18(1):172. doi: 10.1186/s12885-018-4082-6.
- Bronger H, Singer J, Windmüller C, Reuning U, Zech D, Delbridge C, Dorn J, Kiechle M, Schmalfeldt B, Schmitt M. 2016. CXCL9 and CXCL10 predict survival and are regulated by cyclooxygenase inhibition in advanced serous ovarian cancer. Br J Cancer. 115(5):553–563. doi: 10.1038/bjc.2016.172.
- Chatr-Aryamontri A, Oughtred R, Boucher L, Rust J, Chang C, Kolas NK, O’Donnell L, Oster S, Theesfeld C, Sellam A. 2017. The BioGRID interaction database: 2017 update. Nucleic Acids Res. 45(D1):D369–D379. doi: 10.1093/nar/gkw1102.
- Chatterjee M, Hurley LC, Tainsky MA. 2017. Paraneoplastic antigens as biomarkers for early diagnosis of ovarian cancer. Gynecol Oncol Rep. 21:37–44. doi: 10.1016/j.gore.2017.06.006.
- Chen M-W, Yang S-T, Chien M-H, Hua K-T, Wu C-J, Hsiao SM, Lin H, Hsiao M, Su J-L, Wei L-H. 2017. The STAT3-miRNA-92-Wnt signaling pathway regulates spheroid formation and malignant progression in ovarian cancer. Cancer Res. 77(8):1955–1967. doi: 10.1158/0008-5472.CAN-16-1115.
- Cock PJA, Antao T, Chang JT, Chapman BA, Cox CJ, Dalke A, Friedberg I, Hamelryck T, Kauff F, Wilczynski B. 2009. Biopython: freely available python tools for computational molecular biology and bioinformatics. Bioinformatics. 25(11):1422–1423. doi: 10.1093/bioinformatics/btp163.
- Cotto KC, Wagner AH, Feng YY, Kiwala S, Coffman AC, Spies G, Wollam A, Spies NC, Griffith OL, Griffith M. 2018. DGIdb 3.0: a redesign and expansion of the drug-gene interaction database. Nucleic Acids Res. 46(D1):D1068–D1073. doi: 10.1093/nar/gkx1143.
- Fang WB, Jokar I, Zou A, Lambert D, Dendukuri P, Cheng N. 2012. CCL2/CCR2 chemokine signaling coordinates survival and motility of breast cancer cells through Smad3 protein- and p42/44 mitogen-activated protein kinase (MAPK)-dependent mechanisms. J Biol Chem. 287(43):36593–36608. doi: 10.1074/jbc.M112.365999.
- Fu LJ, Wang B. 2013. Investigation of the hub genes and related mechanism in ovarian cancer via bioinformatics analysis. J Ovarian Res. 6(1):92. doi: 10.1186/1757-2215-6-92.
- Goldman MJ, Craft B, Hastie M, Repečka K, McDade F, Kamath A, Banerjee A, Luo Y, Rogers D, Brooks AN, et al. 2020. Visualizing and interpreting cancer genomics data via the xena platform. Nat Biotechnol. 38(6):675–678. doi: 10.1038/s41587-020-0546-8.
- Griner SE, Joshi JP, Nahta R. 2013. Growth differentiation factor 15 stimulates rapamycin-sensitive ovarian cancer cell growth and invasion. Biochem Pharmacol. 85(1):46–58. doi: 10.1016/j.bcp.2012.10.007.
- Grisaru D, Hauspy J, Prasad M, Albert M, Murphy KJ, Covens A, Macgregor PF, Rosen B. 2007. Microarray expression identification of differentially expressed genes in serous epithelial ovarian cancer compared with bulk normal ovarian tissue and ovarian surface scrapings. Oncol Rep. 18:1347–1356.
- Halari CD, Zheng M, Lala PK. 2021. Roles of two small leucine-rich proteoglycans decorin and biglycan in pregnancy and pregnancy-associated diseases. Int J Mol Sci. 22(19):10584. doi: 10.3390/ijms221910584.
- Harrow J, Frankish A, Gonzalez JM, Tapanari E, Diekhans M, Kokocinski F, Aken BL, Barrell D, Zadissa A, Searle S. 2012. GENCODE: the reference human genome annotation for The ENCODE project. Genome Res. 22(9):1760–1774. doi: 10.1101/gr.135350.111.
- Holschneider CH, Berek JS. 2000. Ovarian cancer: epidemiology, biology, and prognostic factors. Semin Surg Oncol. 19(1):3–10. doi: 10.1002/1098-2388(200007/08)19:1<3::AID-SSU2>3.0.CO;2-S.
- Javdan P, Reiisi S, Mohammadi Nejad P. 2018. Overexpression of TRAF4 gene in ovarian cancer samples and association with metastasis and poor prognosis in patients. J Arak Uni Med Sci. 21:21–30.
- Jiang H, Feng Y. 2004. Inhibition of hypoxia-inducible factor 1alpha expression and tumor growth in SKOV3 ovarian cancer model by sirolimus. Zhonghua fu Chan ke za zhi. 39:474–477.
- Jiang Y-P, Wu X-H, Shi B, Wu W-X, Yin G-R. 2006. Expression of chemokine CXCL12 and its receptor CXCR4 in human epithelial ovarian cancer: an independent prognostic factor for tumor progression. Gynecol Oncol. 103(1):226–233. doi: 10.1016/j.ygyno.2006.02.036.
- Jiang Z, Xu Y, Cai S. 2010. CXCL10 expression and prognostic significance in stage II and III colorectal cancer. Mol Biol Rep. 37(6):3029–3036. doi: 10.1007/s11033-009-9873-z.
- Kanehisa M, Goto S. 2000. KEGG: Kyoto encyclopedia of genes and genomes. Nucleic Acids Res. 28(1):27–30. doi: 10.1093/nar/28.1.27.
- Koop G. 2017. Bayesian methods for empirical macroeconomics with big data. Rev Economic Anal. 9(1):33–56. doi: 10.15353/rea.v9i1.1434.
- Li T, Wernersson R, Hansen RB, Horn H, Mercer J, Slodkowicz G, Workman CT, Rigina O, Rapacki K, Stærfeldt HH. 2017. A scored human protein–protein interaction network to catalyze genomic interpretation. Nat Meth. 14(1):61–64. doi: 10.1038/nmeth.4083.
- Liou J-T, Yuan H-B, Mao C-C, Lai Y-S, Day Y-J. 2012. Absence of C–C motif chemokine ligand 5 in mice leads to decreased local macrophage recruitment and behavioral hypersensitivity in a murine neuropathic pain model. Pain. 153(6):1283–1291. doi: 10.1016/j.pain.2012.03.008.
- Long H, Xiang T, Qi W, Huang J, Chen J, He L, Liang Z, Guo B, Li Y, Xie R. 2015. CD133+ ovarian cancer stem-like cells promote non-stem cancer cell metastasis via CCL5 induced epithelial-mesenchymal transition. Oncotarget. 6(8):5846–5859. doi: 10.18632/oncotarget.3462.
- Long H, Xie R, Xiang T, Zhao Z, Lin S, Liang Z, Chen Z, Zhu B. 2012. Autocrine CCL5 signaling promotes invasion and migration of CD133+ ovarian cancer stem-like cells via NF-κB-mediated MMP-9 upregulation. Stem Cells. 30(10):2309–2319. doi: 10.1002/stem.1194.
- Nash MA, Deavers MT, Freedman RS. 2002. The expression of decorin in human ovarian tumors. Clin Cancer Res. 8:1754–1760.
- Norouzi-Barough L, Sarookhani MR, Sharifi M, Moghbelinejad S, Jangjoo S, Salehi R. 2018. Molecular mechanisms of drug resistance in ovarian cancer. J Cell Physiol. 233(6):4546–4562. doi: 10.1002/jcp.26289.
- Obermajer N, Muthuswamy R, Odunsi K, Edwards RP, Kalinski P. 2011. PGE2-induced CXCL12 production and CXCR4 expression controls the accumulation of human MDSCs in ovarian cancer environment. Cancer Res. 71(24):7463–7470. doi: 10.1158/0008-5472.CAN-11-2449.
- Pandis N. 2016. Linear regression. Am J Orthod Dentofacial Orthop. 149(3):431–434. doi:10.1016/j.ajodo.2015.11.019.
- Pintoleite R, Botelho P, Ribeiro E, Oliveira PA, Santos L. 2009. Effect of sirolimus on urinary bladder cancer T24 cell line. J Exp Clin Cancer Res. 28(1):3–3. doi: 10.1186/1756-9966-28-3.
- Pletscher-Frankild S, Pallejà A, Tsafou K, Binder JX, Jensen LJ. 2015. DISEASES: text mining and data integration of disease–gene associations. Methods. 74:83–89. doi: 10.1016/j.ymeth.2014.11.020.
- Rainczuk A, Rao JR, Gathercole JL, Fairweather NJ, Chu S, Masadah R, Jobling TW, Deb-Choudhury S, Dyer J, Stephens AN. 2014. Evidence for the antagonistic form of CXC-motif chemokine CXCL10 in serous epithelial ovarian tumours. Int J Cancer. 134(3):530–541. doi: 10.1002/ijc.28393.
- Richardson A, Kaye SB. 2005. Drug resistance in ovarian cancer: the emerging importance of gene transcription and spatio-temporal regulation of resistance. Drug Resist Updates. 8(5):311–321. doi: 10.1016/j.drup.2005.09.001.
- Righi E, Kashiwagi S, Yuan J, Santosuosso M, Leblanc P, Ingraham R, Forbes B, Edelblute B, Collette B, Xing D. 2011. CXCL12/CXCR4 blockade induces multimodal antitumor effects that prolong survival in an immunocompetent mouse model of ovarian cancer. Cancer Res. 71(16):5522–5534. doi: 10.1158/0008-5472.CAN-10-3143.
- Ritchie ME, Phipson B, Wu D, Hu Y, Law CW, Shi W, Smyth GK. 2015. Limma powers differential expression analyses for RNA-sequencing and microarray studies. Nucleic Acids Res . 43(7):e47. doi:10.1093/nar/gkv007.
- Samuel P, Mulcahy LA, Furlong F, McCarthy HO, Brooks SA, Fabbri M, Pink RC, Carter DRF. 2018. Cisplatin induces the release of extracellular vesicles from ovarian cancer cells that can induce invasiveness and drug resistance in bystander cells. Philos Trans R Soc B. 373(1737):20170065. doi: 10.1098/rstb.2017.0065.
- Shah ED, Fisch BM, Arceci RJ, Buckley JD, Reaman GH, Sorensen PH, Triche TJ, Reynolds CP. 2014. Drugpath: a database for academic investigators to match oncology molecular targets with drugs in development. Cancer Chemother Pharmacol. 73(5):1089–1093. doi: 10.1007/s00280-014-2433-9.
- Shannon P, Markiel A, Ozier O, Baliga NS, Wang JT, Ramage D, Amin N, Schwikowski B, Ideker T. 2003. Cytoscape: a software environment for integrated models of biomolecular interaction networks. Genome Res. 13(11):2498–2504. doi: 10.1101/gr.1239303.
- Stippel D, Kasper H, Schleimer K, Töx U, Bangard C, Hölscher A, Beckurts K. 2005. Successful use of sirolimus in a patient with bulky ovarian metastasis of hepatocellular carcinoma after liver transplantation. Transplant Proc. 37(5):2185–2187. doi: 10.1016/j.transproceed.2005.03.013.
- Tang Z, Kang B, Li C, Chen T, Zhang Z. 2019. Structural and functional insight into the mycobacterium tuberculosis protein PrpR reveals a novel type of transcription factor. Nucleic Acids Res. 47(18):9934–9949. doi: 10.1093/nar/gkz724.
- Torre LA, Trabert B, DeSantis CE, Miller KD, Samimi G, Runowicz CD, Gaudet MM, Jemal A, Siegel RL. 2018. Ovarian cancer statistics, 2018. CA Cancer J Clin. 68(4):284–296. doi:10.3322/caac.21456.
- Türei D, Korcsmáros T, Saez-Rodriguez J. 2016. Omnipath: guidelines and gateway for literature-curated signaling pathway resources. Nat Meth. 13(12):966–967. doi: 10.1038/nmeth.4077.
- Uhlén M, Fagerberg L, Hallström BM, Lindskog C, Oksvold P, Mardinoglu A, Sivertsson Å, Kampf C, Sjöstedt E, Asplund A. 2015. Tissue-based map of the human proteome. Science. 347(6220):1260419. doi: 10.1126/science.1260419.
- Wang Z, Jensen MA, Zenklusen JC. 2016. A practical guide to the cancer genome atlas (TCGA). Meth Mol Biol. 1418:111–141. doi:10.1007/978-1-4939-3578-9_6.
- Weitzenfeld P, Benbaruch A. 2014. The chemokine system, and its CCR5 and CXCR4 receptors, as potential targets for personalized therapy in cancer. Cancer Lett. 352(1):36–53. doi: 10.1016/j.canlet.2013.10.006.
- Yaalhahoshen N, Shina S, Leidertrejo L, Barnea I, Shabtai E, Azenshtein E, Greenberg I, Keydar I, Benbaruch A. 2006. The chemokine CCL5 as a potential prognostic factor predicting disease progression in stage II breast cancer patients. Clin Cancer Res. 12(15):4474–4480. doi: 10.1158/1078-0432.CCR-06-0074.
- Zhou B, Sun C, Li N, Shan W, Lu H, Guo L, Guo E, Xia M, Weng D, Meng L. 2016. Cisplatin-induced CCL5 secretion from CAFs promotes cisplatin-resistance in ovarian cancer via regulation of the STAT3 and PI3K/Akt signaling pathways. Int J Oncol. 48(5):2087–2097. doi: 10.3892/ijo.2016.3442.
- Zhou Y, Zhou B, Pache L, Chang M, Khodabakhshi AH, Tanaseichuk O, Benner C, Chanda SK. 2019. Metascape provides a biologist-oriented resource for the analysis of systems-level datasets. Nat Commun. 10(1):1523. doi:10.1038/s41467-019-09234-6.