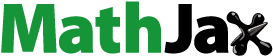
Abstract
A new exponentiated generalized linear exponential distribution (NEGLED) is introduced, which poses increasing, decreasing, bathtub-shaped, and constant hazard rate. Its various mathematical properties such as moments, quantiles, order statistics, hazard rate function (HRF), stress–strength parameter, etc. are derived. Five distributions, exponential distribution (ED), generalized linear exponential distribution (GLED), Rayleigh distribution (RD), Weibull distribution (WD), and generalized linear failure rate distribution (GLFRD) were used for comparison with NEGLED model using a dataset of blood cancer patients. Estimation of the parameters using the maximum likelihood estimation (MLE) method was obtained and to evaluate their performance a simulation study has been carried out. Finally, a dataset of 40 Leukemia patients was analysed for illustrative purpose proving that the NEGLED outperforms compared distributions.
PUBLIC INTEREST STATEMENT
Understanding the need for complex data in all sciences fields, the extension of existing distributions is necessary and timely. We have introduced a new probability distribution known as New Exponentiated Generalized Linear Exponential distribution. This distribution extends the Generalized Linear Exponential Distribution, which accommodates increasing, decreasing, bathtub shaped, and constant hazard rate. Proposed distribution models appear superior than Exponential, Weibull, Rayleigh, Generalized Linear Exponential, and Generalized Failure Rate distributions for the Leukemia dataset.
1. Introduction
Distribution theory plays a vital role in modelling lifetime data not only in life insurance but also in various fields like reliability, queuing theory, and other related areas. For illustration, the standard distributions including Normal, Gamma, and Weibull distributions have attracted wide attention among scientists and attracted very important applications in every branch of science, engineering, technology, demography, etc. These conventional distributions may not provide a satisfactory fit to the real datasets in some cases. Non-monotone hazard rate, for example, cannot be modelled using the above distributions. The Normal distribution has only increasing hazard rate while the Weibull and Gamma distributions show the increasing, decreasing, and constant hazard rate. Therefore, the distributions are modified or extended in the literature for further use. In this article, we use the same method to generate NEGLED, which was used by Gupta et al. (Citation1998) to introduce exponentiated exponential distribution.
Suppose is any positive constant and
be a random variable with distribution function
. Then
,
is known as exponentiated distribution and
is baseline distribution. For instance,
is known as exponentiated Teissier distribution (ETD), see Sharma et al. (Citation2020). In recent time, many distributions are extended to the class of the exponentiated distributions. Sharma et al. (Citation2020) introduced ETD, this new generation made the Teissier distribution compatible with increasing, decreasing and bathtub shape hazard rate. Exponentiated Weibull distribution (EWD) was introduced by Mudholkar and Srivastava (Citation1993) to make WD compatible with non-monotone hazard rates. Later, Nassar and Eissa (Citation2003) again studied the EWD and gave some new statistical measures. Louzada et al. (Citation2014) proposed the exponentiated generalized Gamma distribution. S. Lee and Kim (Citation2019) introduced exponentiated generalized Pareto distribution. For other families of the exponentiated distributions and related studies, see Agarwal et al. (Citation2020), Biçer (Citation2019), C.-S. Lee and Tsai (Citation2017), De Andrade and Zea (Citation2018), Elbatal et al. (Citation2013), Handique et al. (Citation2019), Ghosh et al. (Citation2019), Louzada et al. (Citation2014), Mahmoud and Alam (Citation2010), Okasha and Kayid (Citation2016), Rajchakit et al. (Citation2021), Sarhan et al. (Citation2013), Shakhatreh et al. (Citation2016) Tian et al. (Citation2014a), Tian et al. (Citation2014b), and Wu et al. (Citation2021).
The linear exponential distribution (LED) constitutes the constant or increasing hazard rate shape and decreasing or unimodal density function (Sarhan and Kundu, Citation2009), which is unable to model the phenomenon with non-monotone, decreasing, and bathtub shape hazard rates. Bathtub-shaped hazard rates are very common in reliability studies and researchers use them extensively. Mahmoud and Alam (Citation2010) generalized LED to make it compatible with decreasing, increasing and bathtub shaped hazard rate and denoted by GLED. The cumulative distribution function of GLED is given by
where if
, 0, otherwise and
. Further, Tian et al. (Citation2014a) gave new generalization of LED. Meanwhile, GLED does not provide reasonable fit to modelling phenomenon with bimodal density and constant hazard rate. We have introduced a five parameter NEGLED model, which is generalization of GLED (Mahmoud and Alam, Citation2010). The proposed model in this study provides increasing, decreasing, constant and bathtub shape hazard rate. It has right-skewed, unimodal and bimodal density function. Also, NEGLED includes LED, generalized exponential distribution (GED) (Gupta and Kundu, Citation1999), GLED (Sarhan and Kundu, Citation2009, Mahmoud and Alam, Citation2010; and Tian et al., Citation2014a), generalized Rayleigh distribution (GRD) (Kundu and Raqab, Citation2005), exponentiated generalized linear exponential distribution (EGLED) (Sarhan et al., Citation2013), and many other well-known distributions as sub-models which are extensively used in modelling lifetime datasets. It is easy to discuss various statistical properties of the GED, GLED, GRD, etc. on a single platform through NEGLED. Due to flexibility of NEGLED model, one can anticipate its application in different areas of research.
In Section 2, we have introduced the proposed distribution and some of its reliability expressions such as survival function, HRF, and reversed HRF are derived. Section 3 provides the statistical characteristics, i.e. raw moments, quantiles, and order statistics of the newly proposed distribution. The stress–strength parameter that measures the reliability of the component has been discussed for NEGLED in the same section. In Section 4, MLE of unknown parameters for the proposed distribution has been derived, and their performance was evaluated using simulation. For simulation, different sample sizes have been considered. A real-life application is also discussed in Section 5.
2. The NEGLED
The probability density function (PDF) of NEGLED with parameter vector is given by
where ;
and
. The
and
are the scale parameters,
is the shape parameter, and
is the exponentiation parameter. The nature of the truncation parameter
depends on
,
if
. The PDF defined in (2.1) can also be written in simplified manner as
where and
.
The corresponding cumulative distribution function (CDF) of NEGLED is expressed in the following form
It may be notice that if (the set of natural numbers), then (2.2) denotes the CDF of the largest order statistic having a random sample of size
from
, where
. As a result,
can be used to describe a parallel system with
components, each of which is distributed independently as
. In actuarial science, it can also be considered as the distribution function of independently distributed
insured that has
as individual distribution. The proposed distribution includes several known distributions as special cases, some of the most widely used distribution are shown in .
Table 1. Some well-known distributions derived from
The survival function, , HRF,
, and the reversed HRF,
for NEGLED (
) is given by (2.3), (2.4), and (2.5), respectively
and
It is immediate from the that the density of NEGLED can be decreasing, decreasing-increasing type, unimodal or bimodal depending upon the different values of the parameters.
From the , we observe the hazard rate of NEGLED model can be decreasing, increasing, constant or bathtub shaped. Therefore, NEGLED can be used to model the phenomena with constant or non-monotone hazard rates.
It can easily be shown that through specific parametric substitution, one can get the HRF for ED, RD, GRD, LED, and WD from (2.4). Since GLED is a sub-model of that has the same characteristics of increasing, bathtub-shaped or constant among others HRF for specific values of the parameters. Therefore, in dealing with a diverse range of hazard functions to analyse lifetime data, the NEGLED model demonstrates greater versatility than current literature models.
3. Statistical properties of NEGLED
In this section, we discuss various statistical characteristics of proposed NEGLED model. First, we begin with quantile function as well as random sample generation from NEGLED model. Later on, moments, stress–strength parameters, and order statistics will be discussed consecutively.
3.1. Quantile function and random sample generation
The quantile function which represents the inverse of CDF is given by
Mathematically, the quantile function of can be written as
where Uniform(0,1). The median of
model can be derived by putting
in EquationEquation (3.1)
(3.1)
(3.1) . To generate random sample from
model, one can generate it by using the quantile function given in EquationEquation (3.1)
(3.1)
(3.1) and a random sample from Uniform(0, 1) distribution. For example, the random sample generation of size
from NEGLED, first generate a random sample
(say) of size
from Uniform(0, 1) distribution. Now replace
with
as given in the following formula
3.2. Moments
In pragmatic sciences, moments are important tools for statistical analysis. It can be used to investigate a distribution’s most essential properties (e.g. tendency, dispersion, skewness, and kurtosis). The expression for the moment generating function (MGF), variance, and the th moment of
model have been derived in this sub-section.
Theorem 3.1. For the random variable with
, i.e.
, the
th raw moment of
is given by
Proof. We have
Substituting , we get
.
Since
we have
Suppose , it is easy to confirm the below expressions
So, we have
where and
, respectively, denotes the upper incomplete gamma function and lower incomplete gamma function.□
Remark 3.1. The raw moment, with shape parameter
and scale parameter
, of the Weibull distribution can be found by putting
and
in (3.2).
Lemma 3.1. Let . Then we have
Proof.
Substituting , on taking derivative we get
. So,
Using binomially expansion in the left hand side of (3.4), we have
which proves the result.□
Theorem 3.2. The variance of NEGLED model is derived as follow
where is the mean of
, which can be derived by substituting
in (3.2).
Proof. Simply placing in (3.3), we get
where is the mean of the random variable
. Also, it is easy to get
on putting
in (3.4) as follow
So, the variance of distribution is derived as follow
□
Theorem 3.3. Suppose has a
distribution, then the MGF, i.e.
, of
is given by
Proof. By definition of the MGF of , we have
□
3.3. Stress–strength parameter
Let random stress and strength of the component are denoted by and
, respectively. Then
is known as the stress–strength parameter, which describes the measure of component’s reliability. Let
and
be two independent random variables. Then
Proof.
Consider the transformation , which implies that
. So
Now, take , we get
. So
3.4. Order statistics
Let denote the order statistics of the random sample
from
model. Then, using the standard formula of the PDF of
order statistics see Arnold et al. (Citation1992)), the PDF of the
order statistic
is
Thus, the PDF of (the smallest order statistic) is
and the PDF of (the largest order statistic) is
Theorem 3.4. Let for
be independent r.v.’s. Then
.
The proof of Theorem 3.4 is simple and hence omitted.
The joint PDF of and
is now calculated using the standard formula of the joint PDF of two order statistics (see Arnold et al., Citation1992) as
where . Then, for NEGLED, the joint density of
and
becomes of the form
4. Statistcal inference
Now, we discuss the estimation of the model parameters by using the method of maximum likelihood estimation. Let draw a random sample of size , i.e.
, from
. The likelihood function
for
is given by
and corresponding log-likelihood functions of above equation is
First, differentiate the log-likelihood function with respect to unknown parameters and equate it to 0. The normal equations are given as
Since the MLEs of cannot be obtained in a closed form, one can use iterative procedures like Newton–Raphson method to compute them. It would be impossible to determine the exact distributions of the MLE’s of the parameters due to lack of closed form solution.
The solution to the aforementioned non-linear EquationEquations (4.1(4.1)
(4.1) )–(Equation4.5
(4.5)
(4.5) ) are determined using simulation in R software, assuming asymptotic distribution based on large sample approximations. In our case,
asymptotically follows
. Where
is the vector of MLE’s and denotes the mean vector
meanwhile
denotes the dispersion matrix. Particularly, based on a sample of size
, as
, we have
where
and
, the inverse of the observed information matrix
for
. Suppose
. Then, for
, we obtain
,
and
. Also,
,
and
, where
. Thus, from normal EquationEquations (4.1
(4.1)
(4.1) )–(Equation4.5
(4.5)
(4.5) ), we can calculate the elements of
. The quantity
represents the
confidence interval of
, where
denotes the upper
-th percentile of the standard normal distribution and
denotes the level of significance.
To study various properties of MLE, the estimates of parameters of NEGLED model are derived using simulation. Samples of size are considered with iteration of 10,000 from
using optim command in R software. The bias, standard error, and coverage length (length of
confidence interval) for the MLE of each parameter are evaluated for each case. Findings are presented in the .
Table 2. Bias, Standard error (SE), and Coverage length (CL) for MLEs from NEGLED model
We noticed that perhaps the standard errors, coverage lengths, and absolute biases of each of the ,
,
,
and
MLE’s decrease as increasing the size of the sample, see . This indicates that the MLE method provides good estimates of the parameters for the NEGLED model.
Further, we estimate the stress–strength parameter i.e. . In the context of the reliability of a system, it is very important to study the system performance referred to as the stress–strength parameter. The system will only survive if the applied stress is lesser than the strength. In practise, a good design is one in which the strength is always greater than the expected stress. In the statistical sciences, inferring the stress–strength parameter from a complete or censored sample has piqued the interest of many scientists over years, and the challenge of estimating
under various scenarios has been extensively researched. Many research on the inference of stress–strength parameter
from various perspective have recently been published in the literature. For example, half logistic distribution (Ratnam et al., Citation2000), Burr type X distribution (Kim et al., Citation2000), and normal distribution (Guo and Krishnamoorthy (Citation2004), Barbiero (Citation2011)).
Let we draw two independent random samples, i.e. and
, from
and
of sizes
and
respectively. Then the log-likelihood function
of
is
EquationEquations (4.6(4.6)
(4.6) )–(Equation4.11
(4.11)
(4.11) ) below are the normal equations for the log-likelihood function
.
The non-linear EquationEquations (4.6(4.6)
(4.6) )–(Equation4.11
(4.11)
(4.11) ) can also be solved using iterative procedures like discuss earlier. Thus the MLE of stress–strength parameter i.e.
is
where the MLEs of and
are denoted by
and
, respectively. The general scenario when different multiple parameters of NEGLED model are considered, in such cases, we can compute
but to obtain a closed form is difficult.
5. Statistical data analysis
In the present section, to elucidate the application of NEGLED model, we considered a dataset of 40 patients suffering from Leukemia (a type of blood cancer). Also, the log-likelihood values, Akaike information criterion (AIC) values, log-likelihood ratio (LR) test statistic, and Kolmogorov–Smirnov (KS) test statistic are calculated. These values will help to test the goodness-of-fit of the NEGLED model compare to more familiar distribution models, namely GLFRD, GLED, RD, WD, and ED. At last, a graphical representation provides the empirical and estimated survival functions of the NEGLED, GLFRD, GLED, RD, WD, and ED models for Leukemia dataset.
shows the dataset of the lifetime (in days) of 40 patients suffering from Leukemia from one of the Ministry of Health Hospitals in Saudi Arabia, studied by Abouammoh et al. (Citation1994). Taking into account the computational ease, all the data points was divided by 100 in . Six distribution models GLFRD, GLED, RD, WD, ED along with NEGLED are considered for fitting the dataset. To implement the LR test GLFRD, GLED, RD, WD, and ED have been considered as the null distributions, meanwhile, the NEGLED model has been taken as the alternative distribution. Furthermore, let H = 0 and H = 1 denotes the rejection and acceptance of the null hypotheses respectively. presents the MLEs of the parameters, KS measurements and associated p-values for the Leukemia dataset. And furnishes the AIC values, log-likelihood values, H values and LR test statistic for the compared distribution models. Additionally, gives the simple quartile summary of Leukemia data along with quartile summary based on NEGLED model.
Table 3. A dataset of lifetimes (in days) for 40 patients suffering from leukemia type blood cancer
Table 4. The MLEs of the parameters, KS measurements and associated -values for the leukemia data
Table 5. Information criteria for the leukemia data
Table 6. Quartile summary of the leukemia dataset
The approximate 95% confidence intervals for , and
are (0.2594, 0.2877), (0.2703, 0.2768), (0.1638, 0.3833), (−1.0103, 1.5575), and (−0.1156, 0.6628), respectively. The observed Fisher information matrix for Leukemia data under NEGLED is given by
In , KS test statistic values accompanying their -values are shown for various modelling distributions. Again from the , for all distribution models except the ED, the
-values corresponding to the KS test statistics are higher than
level of significance. It is therefore clearly evident that at 5% level of significance we reject ED and none of the five models GLFRD, GLED, RD, WD, and NEGLED are rejected at the considerable level of significance. The NEGLED model is best model in the sense that it has the largest
-value among all the used models here to fit the Leukemia dataset.
Comparing AIC values from , we mention that the NEGLED model has the smallest AIC value among all the considered distribution models. Therefore, the NEGLED model is chosen as the model with the best fit among all the distributions considered. Moreover, provides the proof in the support of NEGLED model for the given dataset compare to the all considered models. As we can see the theoretical reliability function of the NEGLED model is better fitted to empirical reliability function.
Figure 8. The empirical and estimated survival functions of the NEGLE, GLFRD, GLED, RD, WD, and ED models focused on the data in
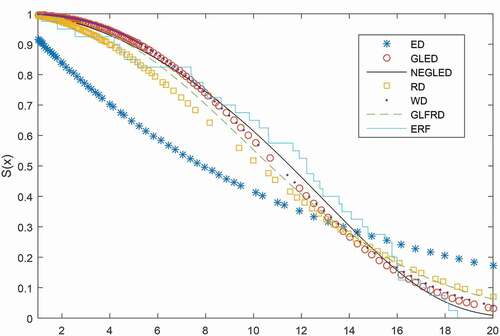
The log-likelihood value of NEGLED model is largest compare to the considered models which indicates the best fit of NEGLED to the given dataset, see . At 5% significance level the critical values for 1, 2, 3, and 4 d.f. are 3.841, 5.991, 7.815, and 9.488, respectively. Next, the LR test statistic for all the models are greater than
critical values for corresponding d.f., see . Consequently, at 5% level of significance, we reject all the null hypotheses i.e. GLFRD, GLED, RD, WD, and ED. Considering all the above results, we may conclude that the NEGLED model is superior competitor for lifetime datasets than the ED, RD, WD, GLFRD, and GLED models.
6. Conclusion
In this article, a new distribution named NEGLED has been introduced which generalizes the GLED model studied by Mahmoud and Alam (Citation2010) and several other well-known distributions. We investigated some statistical properties of the proposed distribution like HRF, quantile function, random sample generation, moments, stress–strength parameter, and order relations. To illustrate the MLEs behavior with increasing sample size, MLE and inference for the NEGLED model using simulation were obtained. It was found that the MLE method provides good estimates for the NEGLED model. At last, using the proposed distribution and some well-known distributions, a real-life dataset is fitted. It was found that, compared to the other distributions (ED, RD, WD, GLED, and GLFRD), the NEGLED model offers a better fit to the Leukemia dataset. Therefore, accounting the flexibility of PDF and HRF, the NEGLED model can be utilized as an effective model for lifetime data applications.
Acknowledgements
We are very thankful to the Editorial board and the reviewers for their valuable comments and suggestions which helped to improve the manuscript significantly.
Disclosure statement
No potential conflict of interest was reported by the authors.
Additional information
Funding
Notes on contributors
Neeraj Poonia
Neeraj Poonia obtained his M.Sc. in Statistics (2017) from the Central University of Punjab, Bathinda, India. He is currently pursuing his Ph.D. in Statistics in the School of Basic Sciences at the Indian Institute of Technology Mandi, Himachal Pradesh, India. His research interest includes probability distribution theory and applied statistics.
Sarita Azad
Sarita Azad obtained her Ph.D. in Mathematics (2008) from Delhi University and the Indian Institute of Science, India. She is currently working as an assistant professor in the School of Basic Sciences at the Indian Institute of Technology Mandi, Himachal Pradesh, India. Her area of research includes climate change modelling, statistical data analysis, time series analysis and forecasting, and distribution theory.
Refereces
- Abouammoh, A., Abdulghani, S., & Qamber, I. (1994). On partial orderings and testing of new better than renewal used classes. Reliab. Eng. Syst. Safety, 43(1), 37–14. https://doi.org/https://doi.org/10.1016/0951-8320(94)90094-9
- Agarwal, P., Hyder, -A.-A., & Zakarya, M. (2020). Well-posedness of stochastic modified kawahara equation. Advances in Difference Equations, (2020(1), 1–10. https://doi.org/https://doi.org/10.1186/s13662-019-2485-6
- Arnold, B. C., Balakrishnan, N., & Nagaraja, H. N. (1992). A first course in order statistics (Vol. 54). Siam.
- Barbiero, A. (2011). Confidence intervals for reliability of stress-strength models in the normal case. Communications in Statistics-Simulation and Computation, 40(6), 907–925. https://doi.org/https://doi.org/10.1080/03610918.2011.560728
- Biçer, H. D. (2019). Properties and inference for a new class of generalized rayleigh distributions with an application. Open Mathematics, 17(1), 700–715. https://doi.org/https://doi.org/10.1515/math-2019-0057
- De Andrade, T. A., & Zea, L. M. (2018). The exponentiated generalized extended pareto distribution. Journal of Data Science, 16(4), 781–800. https://doi.org/https://doi.org/10.6339/JDS.201810_16(4).00007
- Elbatal, I., Diab, L., & Alim, N. A. (2013). Transmuted generalized linear exponential distribution. International Journal of Computer Applications, 83(17), 29–37. https://doi.org/https://doi.org/10.5120/14671-2681
- Ghosh, S., Kataria, K., & Vellaisamy, P. (2019). On transmuted generalized linear exponential distribution. Communications in Statistics-Theory and Methods, 1–23. https://doi.org/https://doi.org/10.1080/03610926.2019.1655577
- Guo, H., & Krishnamoorthy, K. (2004). New approximate inferential methods for the reliability parameter in a stress–strength model: The normal case. Communications in Statistics-Theory and Methods, 33(7), 1715–1731. https://doi.org/https://doi.org/10.1081/STA-120037269
- Gupta, R. C., Gupta, P. L., & Gupta, R. D. (1998). Modeling failure time data by lehman alternatives. Communications in Statistics - Theory and Methods, 27(4), 887–904. https://doi.org/https://doi.org/10.1080/03610929808832134
- Gupta, R. D., & Kundu, D. (1999). Generalized exponential distributions. Aust. N. Z. J. Stat, 41(2), 173–188. https://doi.org/https://doi.org/10.1111/1467-842X.00072
- Handique, L., Chakraborty, S., & de Andrade, T. A. (2019). The exponentiated generalized marshall–olkin family of distribution: Its properties and applications. Annals of Data Science, 6(3), 391–411. https://doi.org/https://doi.org/10.1007/s40745-018-0166-z
- Kim, D.-H., Kang, S.-G., & Cho, J.-S. (2000). Noninformative priors for stress-strength system in the burr-type x model. Journal of the Korean Statistical Society, 29(1), 17–27.
- Kundu, D., & Raqab, M. Z. (2005). Generalized Rayleigh distribution: Different methods of estimations. Comput. Statist. Data Anal, 49(1), 187–200. https://doi.org/https://doi.org/10.1016/j.csda.2004.05.008
- Lee, C.-S., & Tsai, H.-J. (2017). A note on the generalized linear exponential distribution. Statistics & Probability Letters, 124, 49–54. https://doi.org/https://doi.org/10.1016/j.spl.2016.12.016
- Lee, S., & Kim, J. H. (2019). Exponentiated generalized pareto distribution: Properties and applications towards extreme value theory. Communications in Statistics-Theory and Methods, 48(8), 2014–2038. https://doi.org/https://doi.org/10.1080/03610926.2018.1441418
- Louzada, F., Marchi, V., & Roman, M. (2014). The exponentiated exponential–geometric distribution: A distribution with decreasing, increasing and unimodal failure rate. Statistics, 48(1), 167–181. https://doi.org/https://doi.org/10.1080/02331888.2012.667103
- Mahmoud, M. A. W., & Alam, F. M. A. (2010). The generalized linear exponential distribution. Statist. Probab. Lett, 80(11–12), 1005–1014. https://doi.org/https://doi.org/10.1016/j.spl.2010.02.015
- Mudholkar, G. S., & Srivastava, D. K. (1993). Exponentiated weibull family for analyzing bathtub failure-rate data. IEEE Transactions on Reliability, 42(2), 299–302. https://doi.org/https://doi.org/10.1109/24.229504
- Nassar, M. M., & Eissa, F. H. (2003). On the exponentiated weibull distribution. Communications in Statistics-Theory and Methods, 32(7), 1317–1336. https://doi.org/https://doi.org/10.1081/STA-120021561
- Okasha, H. M., & Kayid, M. (2016). A new family of marshall–olkin extended generalized linear exponential distribution. Journal of Computational and Applied Mathematics, 296, 576–592. https://doi.org/https://doi.org/10.1016/j.cam.2015.10.017
- Rajchakit, G., Sriraman, R., Boonsatit, N., Hammachukiattikul, P., Lim, C., & Agarwal, P. (2021). Global exponential stability of clifford-valued neural networks with time-varying delays and impulsive effects. Advances in Difference Equations, (2021(1), 1–21. https://doi.org/https://doi.org/10.1186/s13662-021-03367-z
- Ratnam, R., Rosaiah, K., & Anjaneyulu, M. (2000). Estimation of reliability in multicomponent stress-strength model: Half logistic distribution. IAPQR TRANSACTIONS, 25(1), 43–52.
- Sarhan, A. M., Ahmad, A. E.-B. A., & Alasbahi, I. A. (2013). Exponentiated generalized linear exponential distribution. Applied Mathematical Modelling, 37(5), 2838–2849. https://doi.org/https://doi.org/10.1016/j.apm.2012.06.019
- Sarhan, A. M., & Kundu, D. (2009). Generalized linear failure rate distribution. Comm. Statist. Theory Methods, 38(5), 642–660. https://doi.org/https://doi.org/10.1080/03610920802272414
- Shakhatreh, M. K., Yusuf, A., & Mugdadi, A.-R. (2016). The beta generalized linear exponential distribution. Statistics, 50(6), 1346–1362. https://doi.org/https://doi.org/10.1080/02331888.2016.1230617
- Sharma, V. K., Singh, S. V., & Shekhawat, K. (2020). Exponentiated teissier distribution with increasing, decreasing and bathtub hazard functions. Journal of Applied Statistics, 1–23. https://doi.org/https://doi.org/10.1080/02664763.2020.1813694
- Tian, Y., Tian, M., & Zhu, Q. (2014a). A new generalized linear exponential distribution and its applications. Acta Math. Appl. Sin. Engl. Ser, 30(4), 1049–1062. https://doi.org/https://doi.org/10.1007/s10255-014-0442-4
- Tian, Y., Tian, M., & Zhu, Q. (2014b). Transmuted linear exponential distribution: A new generalization of the linear exponential distribution. Comm. Statist. Simulation Comput, 43(10), 2661–2677. https://doi.org/https://doi.org/10.1080/03610918.2013.763978
- Wu, S., Li, C., & Agarwal, P. (2021). Relaxed modulus-based matrix splitting methods for the linear complementarity problem. Symmetry, 13(3), 503. https://doi.org/https://doi.org/10.3390/sym13030503