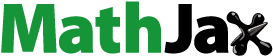
Abstract
The potential of this study for estimating the finite population variance of the study variable of a class of estimators by utilizing an auxiliary variable in simple random sampling is enormous. The asymptotic properties of the proposed class of estimation procedure have been examined. The best fixed values are determined for which the mean squared error of the freshly suggested estimator is lowest. Several well-known existing estimators and class of suggested estimators are identified. To back up the theoretical results, a real population and an extensive simulation study using R software are performed, which demonstrate the dominance of the suggested estimator against all competitors. Appropriate suggestions have been made to the survey statisticians for their real-life implementation.
1. Introduction
An appropriate utilization of auxiliary variables plays a key role in obtaining better estimates of population parameters, as identified in survey studies. It is supplementary information, usually referred to as a benchmark variable or auxiliary variable, and is frequently available through prior experience, census data, or organizational databases. For instance, to analyze the number of trees in a forest, the auxiliary variable will be the diameter of the tree; to study the sales of a book title at a book store in a certain city, the size of the book store is an auxiliary variable, among others. According to Cochran (Citation2007), the inclusion of supplemental information offered by auxiliary variables is widely utilized to increase the precision of the estimators by taking advantage of the correlation between the study and auxiliary variable. In this regard, regression, ratio, and product estimators are excellent examples. Although Cochran (Citation1940) discovered the ratio estimator to be best suited when the study and auxiliary variables are significantly positively correlated, the product estimator is preferable when the variables are highly negatively associated.
In numerous practical situations, survey practitioners are curious not only in the computation of population mean but also in the computation of population variance to enhance certain decision-making policies in the fields of agriculture, production planning in manufacturing industries, business, services industries, ecology, stock investments, seismology, and medical sciences. Thus, improved estimation of the population mean and variance are equally important for effective decision-making procedures. Plenty of situations rises in reality where the of population variance of the study variable is crucial. For these reasons, multiple authors such as Das and Tripathi (Citation1978), Upadhyaya et al. (Citation2004), Singh and Vishwakarma (Citation2008), Gupta and Shabbir (Citation2008), Shabbir and Gupta (Citation2010), Singh et al. (Citation2010), Singh and Solanki (Citation2013), Sisodia and Dwivedi (Citation1981), Kadilar and Cingi (Citation2006), Yadav and Kadilar (Citation2014), Yaqub and Shabbir (Citation2016), Singh and Pal (Citation2017), Cekim and Kadilar (Citation2020), Singh et al. (Citation2021), Singh and Usman (Citation2021), Soni and Pandey (Citation2022), Bhushan et al. (Citation2023), Ahmad et al. (Citation2023), Ali et al. (Citation2024), Pandey et al. (Citation2024a, Citation2024b) are investigated the population variance for conventional and modified estimators based on auxiliary information. Moreover, the extended exponential weighed moving average (EEWMA) statistic is a memory-type statistics that uses past observations along with current information for the estimation of population parameter to improve the efficiency of the estimators. Several authors, Qureshi et al. (Citation2022, Citation2023, Citation2024), Zahid et al. (Citation2023) and so on, have studied the memory type ratio and product estimators in different sampling schemes.
As we know variance estimation is a well-studied topic, so inspired by above-mentioned researchers, the main intent of this work is to propose a family of efficient estimators for the estimation of the population variance of the study variable by utilizing the knowledge of an auxiliary variable in simple random sampling. The leftovers of the article are intended in some sections. Section 2 described some relevant work of population variance estimation. The recommended family of estimators is addressed in Section 3. In Section 4, we have studied the problem of efficiency comparisons. To validate the theoretical findings, an empirical study is performed in Section 5. An ultimate conclusion is given in Section 6.
2. Some pertinent work
Observe that denotes the population, which is composed of N discrete units. Let (Yi, Xi) be the study and the auxiliary variables (Y, X) on the ith unit Ui;
of the population U, respectively. Let us take a sample of size n from the population U and evaluate it using simple random sampling without replacement (SRSWOR).
Let and
be respectively the population means corresponding to the sample means
and
of variables X and Y. Also, consider
and
be the population variances corresponding to the sample variances
and
, respectively. The finite population correction (fpc) is responsible for changing the variance of sample mean when the sample is drawn from a finite population in comparison to an infinite population. Thus, we are omitting the finite population correction (fpc) from the beginning to the end of this article, i.e., we take n−1 instead of
. The purpose is to determine the population variance Sy of the study variable Y based on information from an auxiliary variable X that is highly correlated with the study variable Y.
To obtain the bias and mean squared error (MSE) of the considered estimators, we write
and
such that and to the first degree of approximation,
,
,
,
where ,
,
;
; (p, q) are non-negative integers.
The list of existing estimator(s) along with their mean squared error up to first order of approximation, are given in of Appendix.
Table 1. Several members of the proposed family of estimator (THS) for different values of constant .
3. Recommended family of estimator
Enormous literature has been published on survey sampling from a finite population to tackle the challenges of efficient variance estimation of study variable when information on an auxiliary variable is available. Under certain efficiency conditions, exponential-type estimators are known to outperform the related customary ratio and product-type estimators in terms of lesser mean square errors. Therefore, the question arises as to what happens when the conditions that favor exponential-type estimators over the customary estimators are not readily met. The answer, of course, lies in the use of other efficient estimators that would perform better than both the existing exponential and customary estimators. So, we examine logarithmic-type estimator in our search for such efficient estimators because logarithm is the inverse operation to exponentiation. The logarithmic function has some helpful qualities and is used extensively in a variety of scientific and non-scientific domains. So, motivated by Singh and Solanki (Citation2013) and Bhushan et al. (Citation2023), we solely concentrate on establishing a generalized class of estimators for population variance that can be more efficient than the other conventional estimators for population variance that we have studied earlier in Section 2 only adopt simple random sampling without replacement (SRSWOR) and considers only a single auxiliary variable for convenience.
The suggested class of estimators for population variance of the study variable y as
(1)
(1)
where (ω1, ω2) are constants to be determined such that the mean squared error (MSE) of the proposed class of estimator THS is least,
being constants that take finite values for designing the various estimators and
are either constants or functions of known parameters of the auxiliary variable x, such as
, Cx, β1(x), β2(x) and ρyx.
Various additional estimators can be developed from the suggested estimator for distinct values of (). illustrates how the suggested family of estimators (THS) reduces to an extended form of existing estimators. Expressing (1) in terms of e0 and e1, we have
where ,
,
.
We assume ,
and
; so that
,
and
are expandable. Further, we assume
so that
is expandable.
Now, expanding the right hand side of (2), multiplying and neglecting terms of e′s having power greater than two, we have
(3)
(3)
where
,
,
,
.
Taking expectation of both sides of (3) we get, the bias of THS to the first degree of approximation, as
(4)
(4)
where
,
.
Squaring both sides of (3) and neglecting terms of e′s having power greater than two we have
(5)
(5)
Taking expectation of both sides of (5) we get, the MSE of THS to the first degree of approximation, as
(6)
(6)
where
,
,
. The MSE(THS) is minimized for
(7)
(7)
Thus, the resulting minimum MSE of THS is given by
(8)
(8)
which is true only when
(9)
(9)
For η = 0, the proposed class of estimator THS reduces to Singh and Solanki (Citation2013) estimator, i.e.,
(10)
(10)
4. Efficiency comparisons
To evaluate the mean squared error of the suggested estimator THS over the mean squared error of competitors that are expressed in . So, in this portion of the article, we obtain the efficiency condition as follows
(i)
(11)
(11)
(ii) minMSE(THS) < minMSE(Ti);
(12)
(12)
(iii) minMSE(THS) < MSE(T3)
(iv) minMSE(THS) < minMSE(T5)
(v) minMSE(THS) < MSE(Ti);
(15)
(15)
(vi) minMSE(THS) < minMSE(T14);
(16)
(16)
(vii) minMSE(THS) < minMSE(T15)
(viii) minMSE(THS) < minMSE(T16)
(ix) minMSE(THS) < minMSE(T17)
(x) minMSE(THS) < minMSE(T18)
(xi) minMSE(THS) < minMSE(T19)
(xi) minMSE(THS) < minMSE(T20)
The suggested family of estimator outperforms the other conventional estimators listed in when the conditions from (11-22) are met. In the next section, we conducted a numerical analysis to judge the theoretical results by using real and hypothetical population.
5. Empirical study
To test, the efficiency of the suggested family of estimators over other competing estimator(s), we conducted an empirical study by using real and simulated population. The percent relative efficiency (PRE) of all considered estimators is defined as
(23)
(23)
where
.
The PRE of the proposed estimator THS with respect to is defined as
(24)
(24)
where
.
The is to be computed for different values of the constants (
).
5.1. Real dataset
To validate the theoretical results, we execute a numerical analysis with a real population, which is based on the literacy of persons in the village (refer to Mukherjee Citation1998). Let Y denotes the literate persons in the village and X denotes the number of workers in the village, respectively. The specifics of real population is defined in where population size N = 64 and sample size is 19.
Table 2. Data statistics.
and show the bias and percent relative efficiency of the estimators using the real population. From , it is clear that the estimator which utilizes information on mean
and coefficient of kurtosis β2(x) having minimum bias as compared to other family of suggested estimator. It is observed from that all estimators
, (
) which are members of the suggested family of estimators THS perform better than the usual unbiased estimator, Das and Tripathi (Citation1978), Isaki (Citation1983), Singh et al. (Citation1988), Upadhyaya and Singh (Citation1999), Kadilar and Cingi (Citation2006) ratio type estimators, Shabbir and Gupta (Citation2007), Singh and Solanki (Citation2013) class of estimators, Yadav and Kadilar (Citation2014), Yaqub and Shabbir (Citation2016), Singh and Pal (Citation2017), Cekim and Kadilar (Citation2020) and Bhushan et al. (Citation2023) class of estimators. Besides this, among all the estimators and classes of estimators discussed here, estimator
which utilizes information on the population coefficient of variation Cx is the best in terms of having the highest percent relative efficiency.
Table 3. Bias of proposed family of estimators using real population.
Table 4. Percent relative efficiency of distinct estimators using real population.
5.2. Hypothetical population
Making use of R software, a Monte Carlo simulation study is carried out to compare the percent relative efficiency (PRE) of the proposed class of estimator against other conventional estimator(s) that are considered in our article. We assume three finite populations of size N = 2000, N = 3000, and N = 4500 generated from a normal distribution, and from the population N = 2000 we take different samples of sizes, i.e., n = 200, n = 300, and n = 400. From N = 3000, we pick a sample of distinct sizes, i.e., n = 300, n = 400, and n = 500. Moreover, from a population N = 4500, we choose the sample of sizes n = 400, n = 500, and n = 600. The study and auxiliary variable are also generated from a normal distribution, i.e., and
, respectively.
represents the bias of the recommended family of estimator(s) with three hypothetical populations N and samples n. It is envisaged from that for increase in the value of sample size from 200 to 400, the ;
of the family of recommended estimator(s) decreases. The estimator
which utilizes information on mean
having minimum bias when population size i.e., N = 2000 and N = 4500 and when population size i.e., N = 3000, the estimator
which utilizes information on coefficient of variation Cx, correlation coefficient ρyx, coefficient of kurtosis β2(x) and mean
having minimum bias among all the family of estimators/classes of estimators addressed here.
Table 5. Bias of proposed family of estimators using simulated population with different values of N and n.
illustrates the percent relative efficiency of the proposed family of estimators over other competing estimators by using three hypothetical populations, i.e. . Following points are noted from that
Table 6. Percent relative efficiency of different estimators using simulated population when N = 2000.
Table 7. Percent relative efficiency of different estimators using simulated population when N = 3000.
Table 8. Percent relative efficiency of different estimators using simulated population when N = 4500.
1. From , the estimator which utilizes information on mean
, coefficient of kurtosis β2(x), coefficient of variation Cx, and correlation coefficient ρyx having the highest percent relative efficiency amongst all the estimators/classes of estimators addressed here.
2. From , the estimator that utilizes information on the population coefficient of variation Cx has the highest percent relative efficiency over the other considered estimators.
3. From , the estimator when the constants a = 1, b = 0, c = 0 and d = 1 having the highest percent relative efficiency among others.
According to the results from , the PRE of the proposed family of estimators ;
performs better than all the other estimators that are considered in our work. As a result, the proposed class of estimators explains its significance and is strongly advisable for survey practitioners.
6. Conclusion
For the population variance of a study variable, we have developed a generalized family of estimators where information on an auxiliary variable is available in this article. Additionally, some well-known estimators of population variance, such as the usual unbiased estimator, Das and Tripathi (Citation1978), Isaki (Citation1983), Singh et al. (Citation1988), Upadhyaya and Singh (Citation1999), Kadilar and Cingi (Citation2006) ratio type estimators, Shabbir and Gupta (Citation2007), Singh and Solanki (Citation2013) class of estimators, Yadav and Kadilar (Citation2014), Yaqub and Shabbir (Citation2016), Singh and Pal (Citation2017), Cekim and Kadilar (Citation2020) and Bhushan et al. (Citation2023) class of estimators are also advised. Several families of estimators are produced from the suggested estimator by using different parametric of
. Up to the first degree of approximation, we have established the bias and mean square error of the proposed estimator. A comparison study is conducted to determine the efficiency conditions under which the proposed family of estimators outperforms the other conventional estimators. A simulation study is also conducted to test the theoretical results using real and three hypothetical populations, and it is obvious from the simulation study that the PRE of the proposed family of estimators
;
performs better than all the other estimators. As a result, in practice, we prefer the proposed class of estimators.
Disclosure statement
All authors have no conflict of interest to declare.
References
- Ahmad S, Adichwal N K, Aamir M, Shabbir J, Alsadat N, Elgarhy M, Ahmad H. 2023. An enhanced estimator of finite population variance using two auxiliary variables under simple random sampling. Sci Rep. 13:10698202.
- Ali H, Asim SM, Ijaz M, Zaman T, Iftikhar S. 2024. Improvement in variance estimation using transformed auxiliary variable under simple random sampling. Sci Rep. 14:8117. doi:10.1038/s41598-024-58841-x. PMC: 38582765.
- Bhushan S, Kumar A, Alsubie A, Lone SA. 2023. Variance estimation under an efficient class of estimators in simple random sampling. Ain Shams Eng J. 14:102012. doi:10.1016/j.asej.2022.102012.
- Cekim H, Kadilar C. 2020. ln-type variance estimators in simple random sampling. Pak J Stat Oper Res. 16:689–696. doi:10.18187/pjsor.v16i4.3072.
- Cochran WG. 1940. The estimation of the yields of cereal experiments by sampling for the ratio of grain to total produce. J Agric Sci. 30:262–275. doi:10.1017/S0021859600048012.
- Cochran, WG. 2007. Sampling techniques. Hoboken, NJ: John Wiley & Sons.
- Das AK, Tripathi TP. 1978. Use of auxiliary information in estimating the finite population variance. Sankhya C. 40:139–148.
- Gupta S, Shabbir J. 2008. Variance estimation in simple random sampling using auxiliary information. Hacet J Math Stat. 37:57–67.
- Isaki CT. 1983. Variance estimation using auxiliary information. J Am Stat Assoc. 78:117–123. doi:10.1080/01621459.1983.10477939.
- Kadilar C, Cingi H. 2006. Ratio estimators for the population variance in simple and stratified random sampling. Appl Math Comput. 173:1047–1059. doi:10.1016/j.amc.2005.04.032.
- Mukherjee, C, White, H, Wuyts, M. 1988. Econometrics and data analysis for developing countries. London: Routledge. doi:10.4324/9781315003580.
- Pandey MK, Singh GN, Zaman T, Mutairi AA, Mustafa MS. 2024a. Improved estimation of population variance in stratified successive sampling using calibrated weights under non-response. Heliyon. 10:e27738. doi:10.1016/j.heliyon.2024.e27738. PMC: 38545218.
- Pandey M K, Singh G N, Zaman T, Mutairi A A, Mustafa MS. 2024b. A general class of improved population variance estimators under non-sampling errors using calibrated weights in stratified sampling. Sci Rep. 14:2948.
- Qureshi M N, Alamri O A, Riaz N, Iftikhar A, Tariq M U, Hanif M. 2023. Memory-type variance estimators using exponentially weighted moving average statistic in presence of measurement error for time-scaled surveys. PLoS One. 18:277697.
- Qureshi M N, Faizan Y, Shetty A, Ahelali M H, Hanif M, Alamri O A. 2024. Ln-type estimators for the estimation of the population mean of a sensitive study variable using auxiliary information. Heliyon. 10:23066.
- Qureshi M N, Tariq M U, Hanif M. 2022. Memory-type ratio and product estimators for population variance using exponentially weighted moving averages for time-scaled surveys. Commun Stat Simul Comput. 53:1484–1493. doi:10.1080/03610918.2022.2050390.
- Shabbir J, Gupta S. 2007. On improvement in variance estimation using auxiliary information. Commun Stat Theory Methods. 36:2177–2185. doi:10.1080/03610920701215092.
- Shabbir J, Gupta S. 2010. Some estimators of finite population variance of stratified sample mean. Commun Stat Theory Methods. 39:3001–3008. doi:10.1080/03610920903170384.
- Singh C, Singh GN, Kim J-M. 2021. A randomized response model for sensitive attribute with privacy measure using Poisson distribution. Ain Shams Eng J. 12:4051–4061. doi:10.1016/j.asej.2021.03.006.
- Singh GN, Usman M. 2021. Enhanced estimation of population distribution function in the presence of non-response. Ain Shams Eng J. 12:3109–3119. doi:10.1016/j.asej.2021.02.002.
- Singh HP, Pal SK. 2017. Improvement over variance estimation using auxiliary information in sample surveys. Commun Stat Theory Methods. 46:7732–7750. doi:10.1080/03610926.2016.1161799.
- Singh HP, Solanki RS. 2013. Improved estimation of finite population variance using auxiliary information. Commun Stat Theory Methods. 42:2718–2730. doi:10.1080/03610926.2011.617485.
- Singh HP, Singh S, Kim J-M. 2010. Efficient use of auxiliary variables in estimating finite population variance in two-phase sampling. Commun Stat Appl Methods. 17:165–181. doi:10.5351/CKSS.2010.17.2.165.
- Singh HP, Upadhyaya LN, Namjoshi UD. 1988. Estimation of finite population variance. Curr Sci. 57:1331–1334.
- Singh HP, Vishwakarma GK. 2008. Some families of estimators of variance of stratified random sample mean using auxiliary information. J Stat Theory Pract. 2:21–43. doi:10.1080/15598608.2008.10411858.
- Sisodia BVS, Dwivedi V K. 1981. A modified ratio estimator using coefficient of variation of auxiliary variable. J Indian Soc Agric Stat. 33:13–18.
- Soni SS, Pandey H. 2022. Improved estimation of population variance utilizing known auxiliary parameters. Sch J Phys Math Stat. 9:92–101. doi:10.36347/sjpms.2022.v09i06.001.
- Upadhyaya LN, Singh HP. 1999. An estimator of population variance that utilizes the kurtosis of an auxiliary variable in sample surveys. Vikram Math J. 19:14–17.
- Upadhyaya LN, Singh HP, Singh S. 2004. A class of estimators for estimating the variance of the ratio estimator. J Jpn Stat Soc. 34:47–63. doi:10.14490/jjss.34.47.
- Yadav SK, Kadilar C. 2014. A two parameter variance estimator using auxiliary information. Appl Math Comput. 226:117–122. doi:10.1016/j.amc.2013.10.044.
- Yaqub M, Shabbir J. 2015. An improved class of estimators for finite population variance. Hacet J Math Stat. 45:1–1. doi:10.15672/HJMS.20156310746.
- Zahid R, Noor-Ul-Amin M, Khan I, Salman AA, Pathak PK, Rahimi J. Combination of memory type ratio and product estimators under extended EWMA statistic with application to wheat production. Sci Rep. 13. doi:10.1038/s41598-023-40687-4.
Appendix
Appendix
Table A.1. Existing estimators with their mean square errors under SRS.