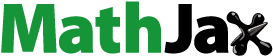
ABSTRACT
Many types of fractional stochastic differential equation (FrSDE), such as Caputo, fractional Brown motion derivatives, and Mittag-Later functions, exist. In recent decades, FrSDE has been a hot topic and can be applied to many fields of research, such as disease transmission, option pricing, and quantitative finance. FrSDEs have various research and applications in financial markets. After comparing many internationally known articles, the fractional order stochastic differential equation proposed in 2016 is most suitable for European option pricing. Over the years, many scholars have studied fractional Brownian motion, fractional ordinary differential equations, and backward differential equations, and no one has studied the application of fractional backward equations in the financial field. Therefore, in this article, to adapt to the financial market more accurately, we construct a backward equation for this kind of FrSDE and construct a new mapping and use the norm method to prove the existence, uniqueness, and stability of the solution to the backward equation. Finally, considering European call options, the Euler Maruyama simulation example of FrSDE is investigated.
1. Introduction
Time series with memory have been widely used in the fields of physics, chemistry, finance and biology. In this paper, we mainly consider that the time series of financial variables must be the memory dependent in the actual financial market. The financial variables here include a region's GDP, interest rate, foreign exchange, stock price, option price, and so on. The conditional probability method can be used to prove the existence of memory effect in stock price series, and the degree of long memory auto-correlation can also be measured [Citation1,Citation2].
Backward stochastic differential equation (BSDE) has developed rapidly in recent years, has been studied and applied in different fields such as probability and statistics, partial differential equation (PDE), function analysis, numerical analysis and stochastic calculation, engineering, economy and mathematical finance. It is impossible for us to give a complete review of all important developments in the past 20 years. The most classic work is the literature [Citation3], in which readers can consult on their own. Therefore, we mainly introduce the method of fractional differential equation, analyse the existence and uniqueness of its solution by using the backward thinking, and finally attempt to evaluate the effect of its numerical solution.
In recent decades, fractional order stochastic differential equation has been a popular topic and can be applied to many fields of research, such as disease transmission, option pricing, and quantitative finance [Citation4–7]. There are two primary research directions: stochastic differential equation driven by fractional Brownian motion (FbmSDE) and stochastic differential equation with Caputo fractional derivatives (Caputo-FrSDE). The form of FbmSDE [Citation8] is represented as follows Equation(Equation1(1)
(1) ):
(1)
(1) In the formula,
represents the stock price in time t,
represents drift coefficient that changes with stock price and time,
represents the volatility that changes with time, and
represents the Fractional Brownian motion.
Although fractional Brownian motion has lost its martingale property, it will obtain Brownian motion with memory trend according to the Hurst exponent(H) [Citation1]. FbmSDE also has many applications in finance [Citation8]. After Peng [Citation3] proposed the backward equation of FbmSDE: , ELS options can now be priced [Citation9].
The second type is Caputo-SDE. A new derivative is defined by Caputo as Equation(Equation2(2)
(2) ) [Citation10]:
(2)
(2) In the equatoion,
represents the gamma function.
Because the processes of the financial market have random effects, Li et al. [Citation9] added the random process to fractional ordinary differential equation (Caputo-Li FrSDE) and proposed a new model constructed from fractional stochastic differential equation [Citation11]. The stochastic process of asset price expressed by the fractional stochastic differential equation is as follows:
(3)
(3) In Equation(Equation3
(3)
(3) ), H is the Hurst index, which describes the memory of the time series and can be calculated by R/SD. In the special case of
, the equation is simplified to a classical stochastic differential equation. H will change the integral form of SDE. In other papers, the finite time stability of solutions of linear stochastic fractional order systems with time delay is also studied when
[Citation12]. However, only
can be considered in the financial market. Inspired by the above literature, we proposes a backward SDE in this range in the form of:
(4)
(4) We mainly introduce a new norm to verify the existence and uniqueness of solutions of above backward fractional differential equations. In the current academic research process, some people have studied the backward equation and fractional differential equation, but no one has studied the subject of this paper, so it is a relatively new idea.
The paper is organized as follows. In Second 2, we introduce the transformation from Caputo fractional reciprocal to fractional differential equation. In Section 3, some hypotheses and lemmas are proposed to facilitate the later theorem citation. In Section 4, the existence and uniqueness of mild solutions of backward fractional differential equations are proven, and the interval conditions for the existence of solutions are given. In Section 5, stability conditions of the backward fractional differential equations are given. In Section 6, the Euler simulation solution of fractional SDE is simply compared with the real solution under specific circumstances. In Section 7, we conclude our investigation.
2. Fractional backward stochastic integral equation
In the real financial market, the Hurst index typically only considers the case that , the following research is based on this premise. In this interval, the Caputo fractional derivative is in the following form [Citation11]:
(5)
(5) In addition, let
, X is the stock price. The drift coefficient is changed from
to
, and the fluctuation coefficient is changed from
to
. We substitute this variable into Equation(Equation3
(3)
(3) ) and obtain:
(6)
(6) Combining with Equations(Equation5
(5)
(5) ) and(Equation6
(6)
(6) ), we obtain:
(7)
(7) Notice that
represents the daily returns of stock
, and
is the returns of one year; thus,
, and Equation(Equation7
(7)
(7) ) can be written as follows:
(8)
(8) Integrating Equation(Equation8
(8)
(8) ) in the interval
and noting that
we take the backward equation according to the method from [Citation13], to obtain the result:
(9)
(9)
3. Assumptions and conditions
In this section, we primarily build some lemmas and conditional assumptions to facilitate the proof of theorems in the next sections.
Assumption 3.1
First of all, we denote by the set of
-measurable random variables X:
which are square integrable, and by
the set of predictable processes
such that
where
the standard Euclidean norm in the Euclidean space
, or q). The terminal condition
in Equation(Equation9
(9)
(9) ) is
-measurable and square integrable. Moreover, we need the following assumptions [Citation14]:
(A1) | For all | ||||
(A2) | The functions | ||||
(A3) | The generator |
Lemma 3.1
Bohr's inequality [Citation15]
If is a normed linear space, when p, q>1 and
. Then,
Also,
Lemma 3.2
Jesson's inequality [Citation15]
Suppose φ is a convex function in , f, p are integrable in
, α
, and
.Then
Specially,
Lemma 3.3
Holder's inequality [Citation15]
Suppose or
is a real or imaginary column. Let
, when p = 1, it is stipulated that
, then
Specially,
Lemma 3.4
Banach's theorem [Citation16]
Suppose X is a metric space. is a mapping (It does not have to be linear). There exists a constant
such that
Then, there exists
makes Tx = x, where T is a compression map.
Lemma 3.5
Martingale representation theorem [Citation17]
Let , be a local martingale adapted to the Brownian filtration
. Then there exists a predictable process
such that
with probability 1, and the following equation holds
Moreover, if Y is an integrable
-measurable random variable,
, then
If in addition, Y and B have jointly a Gaussian distribution, then the process
is deterministic.
Lemma 3.6
[Citation15]
Suppose , and
. Thus
Definition 3.1
Let and
be fixed. We say that a measurable function
belongs to
if and only if the quantity
Definition 3.2
The trivial solution of Equation(Equation9(9)
(9) ) is said to be exponentially stable [Citation18] in the quadratic mean if there exist positive constants C, v such that
Lemma 3.7
Let and
. Then,
is a Banach space.
Proof.
Obviously, the defined weighted norm satisfies the conditions of norm in space. It is only left to show that it is complete. Hence, a proof of this part is similar to the proof of Theorem 3.4 in [Citation19], so we omit it here.
In particular, we consider p = 2 and throughout this paper such that the weighted norm is defined by
To show existence and uniqueness of the solutions to backward fractional differential equation, we consider the following norm:
We define
to be a Banach space endowed with the norm
where
Then
is also Banach space equipped with the norm as below:
4. Existence and uniqueness
To prove the existence and uniqueness theorem of solutions, we must first discuss the existence and uniqueness of solutions and find a range to make Banach's fixed point theorem hold. First, we prove Lemma 4.1 which is important in the next proof.
Lemma 4.1
For any , FrBSDE equation
(10)
(10) admits a unique solution in
.
Proof.
Uniqueness: Take expectations on both sides of Equation(Equation10
(10)
(10) ) at the same time.
represents the information we know at time t. Then we get:
(11)
(11) Assuming that
and
are different solutions of Equation(Equation10
(10)
(10) ), then
Thus
and
Thus
It is obvious that
and this follows that
.
Existence: According to Equation(Equation11(11)
(11) ), we find that
and
are unknown. According to Lemma 3.5, we know that
is a martingale. Thus we have
which make
(12)
(12) Obviously, for all
, we have
when
.
Because
we have
(13)
(13) Similarly,
(14)
(14) Substitute Equations(Equation13
(13)
(13) ) and(Equation14
(14)
(14) ) into Equation(Equation11
(11)
(11) ), we have
Compared with Equation(Equation10
(10)
(10) ), we can get
(15)
(15) Then there exists a solution
of Equation(Equation10
(10)
(10) ) given by
(16)
(16)
Next, let's find out the range that makes Banach's theorem true.
Lemma 4.2
(17)
(17) where
Proof.
Now we estimate the solution given by Equations(Equation16
(16)
(16) ) and(Equation15
(15)
(15) ) in
. From Equation(Equation16
(16)
(16) ) and Lemma 3.1, it follows that
(18)
(18) Easily, we have:
Using expectation property
and conditional expectation property, we have:
Because Lemma 3.2, we get:
We know that
, then:
Easily, we have:
Above of all, we get:
(19)
(19) Next, considering Equation(Equation15
(15)
(15) ) with Lemma 3.1, we can write :
Using Lemma 3.3, we can get:
Let
We have:
(20)
(20) Using Lemma 3.1, and
, we get:
From Equation(Equation12
(12)
(12) ), we invoke the following inequalities for
(21)
(21) So,
And then,
(22)
(22) Combining with Equations(Equation22
(22)
(22) ) and(Equation19
(19)
(19) ), we get:
(23)
(23) Through simplification, we have
Through the above proof, we can simply obtain the existence and uniqueness of solutions to fractional stochastic differential equations from the following theorem.
Theorem 4.1
If
(24)
(24) then Equation (Equation9
(9)
(9) ) admits a unique solution
under Assumption 3.1.
Proof.
For any fixed , it follows from Assumption 3.1 that
By Lemma 4.1, the equation
has a unique solution in
. Thus, the operator
defined by
where
is a solution to Equation(Equation10
(10)
(10) ), is well-defined. Now we prove the contractivity of the operator Ψ. To do so, we apply Lemma 4.2 to obtain that
According to Assumption 3.1,
And then,
So, when condition Equation(Equation24
(24)
(24) ) is true, we get contractivity of operator Ψ on
, which in turn implies the existence and uniqueness of Equation(Equation10
(10)
(10) ).
5. Stability analysis
Mean square stability is a very important kind of stability. Mathematically, the mean square (2-order moment) is the norm in Hilbert space, and the mean square stability is the norm stability. Therefore, this section mainly introduces the second-order moment stability of trivial solutions to fractional backward stochastic differential equations.
Theorem 5.1
There exist two constants and
according to Lemma 3.2 such that Equation (Equation10
(10)
(10) ) is stable.
Proof.
According to Equation(Equation10(10)
(10) ) and Lemma 3.1, we have:
Taking expectations at the same time on both sides of the above inequality, and using Lemma 3.3, we get
Using linear growth of Assumption 3.1
By Lemma 3.6, we have
6. Example
Here, we consider discretizing the backward equation and make an estimate of the stock price . The comparison between the forward and backward FrSDE and the real solution is adopted respectively. First, the forward equation
(25)
(25) and the formula of the true solution Equation(Equation26
(26)
(26) ) [Citation11] are given:
(26)
(26) After reversing the above Equations(Equation26
(26)
(26) ) and(Equation25
(25)
(25) ), we assume that
. Here, we consider discretizing the time into one hundred equal parts, and finally get the knowledge solution and Euler–Maruyama simulation solution of the stock price at each time, as shown in the Figure . The blue discount represents the real solution, and the red dotted line represents the simulation solution [Citation20]. The main difference between the inputs of Figure (a,b) is volatility. When the volatility is relatively small in the short term, the fitting degree will be higher. The effect is shown in Figure (a). If the volatility is too large, it will constantly offset due to the split time points, making it difficult to fit on the way. However, although Figure (b) is difficult to achieve access on the way, the trend and results are roughly the same, with good results. The following data experiment table is based on Figure (b). As Tables – based on
. The errors represents the difference between the EM simulation result and the real simulation value. When the fractional order α changes, it will not have a great impact on the error of the solution, which is in line with the objective fact. Because Hurst index is only related to market rules and should not be a reason to affect the accuracy.
Table 1. Errors between real solution and simulated solution when .
Table 2. Errors between real solution and simulated solution when .
Table 3. Errors between real solution and simulated solution when .
Table 4. Errors between real solution and simulated solution when .
The table shows that the larger the coefficient N is, the better. Thus, a good error can be achieved when it is approximately 1000, and the time consumption is relatively small. The larger N makes the result worse and the longer the running time. To reduce error, we must optimize the algorithm, which is difficult to solve by modifying the coefficient. In literature [Citation20], it is known that the model has only local weak convergence of order 1 and strong convergence of order . This table shows that the choice of order has little effect on the experimental error results, and N has little effect on the error simulation of the model; thus, the model cannot improve the effect of the model by simply modifying the parameters. This result highlights an area for future work to be completed.
For the problem of solving stochastic equations, various disciplines are currently studying this problem and giving many research methods. For example, solve the nonlinear equation by linearizing it [Citation21]; The R function method of Kudriahov, the Jacobian elliptic function method (JEFM) and the improved auxiliary equation method (MAE) are three effective and robust integration methods to help extract the solution of the equation [Citation22]; Even scientists and researchers from different disciplines are dealing with fractional mixed boundary value problems [Citation23]. The proofs of these studies in mathematical theory are excellent, but they are difficult to apply in real life. As can be seen from the table, this paper can find the solution of fractional order equation in a short time by using the backward method, which has certain computational advantages for the above methods, but the above methods are also very worthy of learning, The comparison of numerical experiments with various methods is also an important and interesting direction in the future.
7. Conclusion and future research
Because fractional ordinary differential equations can express the memory effect in the financial system, we establish a backward fractional stochastic differential equation through the fractional differential equation proposed by Tien [Citation8]. At the same time, because the fractional differential equation can express the memory effect of the financial system when floating, we study the problem of integrating the stochastic process into the fractional differential equation, which can facilitate us to analyse the market volatility with memory. Therefore, after proving the existence and uniqueness of the solution, we also make a simple regression of the option price through fractional order stochastic differential equation. Because the memory indexes in the financial market typically, we only consider . Based on the fractional stochastic differential equation, we apply the method of constructing new norms to prove the Banach fixed point theorem and then obtain the existence and uniqueness of its solution. Finally, the comparison between the real solution and the simulated solution is given. It can be seen from the comparison chart that although the final simulation result after parameter adjustment can approximate the real solution, there are many errors in this method if it is the same as the real solution at all times. Based on the study of the existence and uniqueness of the solution of fractional stochastic differential equation, it can be found that if the stock price drift and volatility are driven by fractional order (that is, affected by the past state), it is also theoretically valid to inversely solve the option price according to the expectation at the last moment. Thus, if the neural network is used to solve the option pricing, it can also be used as the input of the neural network according to the past data, and the time length of this past data needs to be determined by continuous experiments. Of course, it will be particularly interesting to use different numerical methods to solve the numerical solution of the pricing of backward fractional stochastic differential options, which is also the main goal of the future work.
Disclosure statement
No potential conflict of interest was reported by the authors.
Additional information
Funding
References
- Hurst HE. Long-term storage capacity of reservoirs. Trans Am Soc Civ Eng. 1951;116(1):770–799.
- Garzarelli F, Cristelli M, Pompa G, et al. Memory effects in stock price dynamics: evidences of technical trading. Sci Rep. Mar 27, 2014;4:1–9.
- Hu Y, Peng S. Backward stochastic differential equation driven by fractional brownian motion. SIAM J Control Optim. 2009;48(3):1675–1700.
- Hussain S, Madi EN, Khan H, et al. On the stochastic modeling of COVID-19 under the environmental white noise. J Funct Sp. 2022;2022:1–9.
- Ahmad M, Zada A, Ghaderi M, et al. On the existence and stability of a neutral stochastic fractional differential system. Fractal Fract. 2022;6(4):203.
- Khan H, Alam K, Gulzar H, et al. A case study of fractal–fractional tuberculosis model in China: existence and stability theories along with numerical simulations. Math Comput Simul. 2022;198:455–473.
- Baleanu D, Jajarmi A, Mohammadi H, et al. A new study on the mathematical modelling of human liver with Caputo–Fabrizio fractional derivative. Chaos Solitons Fractals. 2020;134:Article ID 109705.
- Tien DN. Fractional stochastic differential equations with applications to finance. J Math Anal Appl. 2013;397(1):334–348.
- Wang J, Yan Y, Chen W, et al. Equity-linked securities option pricing by fractional brownian motion. Chaos Solitons Fractals. 2021;144:Article ID 110716.
- Caputo M. Linear models of dissipation whose q is almost frequency independent. Ann Geophys. 1966;19(4):383–393.
- Li Q, Zhou Y, Zhao X, et al. Fractional order stochastic differential equation with application in european option pricing. Discrete Dyn Nat Soc. 2014. DOI:10.1155/2014/621895
- McHiri L, Ben Makhlouf A, Baleanu D, et al. Finite-time stability of linear stochastic fractional-order systems with time delay. Adv Differ Equ. 2021;2021(1):1–10.
- Peng S. The backward stochastic differential equations and its application. Adv Math (NY). 1997;26(2):97–112.
- Ding D, Li X, Liu Y. A regression-based numerical scheme for backward stochastic differential equations. Comput Stat. 2017;32(4):1357–1373.
- Kuang J. Applied inequalities. Qingdao (China): Shandong Science and Technology Press; 2010.
- Liu P. Fundamentals of functional analysis. Beijing (China): Science Press; 2001.
- Klebaner FC. Introduction to stochastic calculus with applications. Singapore: World Scientific Publishing Company; 2012.
- Umamaheswari P, Balachandran K, Annapoorani N. Existence and stability results for Caputo fractional stochastic differential equations with Lévy noise. Filomat. 2020;34(5):1739–1751.
- Baghani O. On fractional langevin equation involving two fractional orders. Commun Nonlinear Sci Numer Simul. 2017;42:675–681.
- Urama TC, Ezepue PO. Stochastic ito-calculus and numerical approximations for asset price forecasting in the nigerian stock market. J Math Finance. 2018;8(04):640–667.
- Gençoğlu MT, Agarwal P. Use of quantum differential equations in sonic processes. Appl Math Nonlinear Sci. 2021;6(1):21–28.
- Arshed S, Raza N, Javid A, et al. Chiral solitons of (2+1)-dimensional stochastic chiral nonlinear schrödinger equation. Int J Geom Methods Mod Phys. 2022;19(10):2250149–3991.
- Aghili A. Complete solution for the time fractional diffusion problem with mixed boundary conditions by operational method. Appl Math Nonlinear Sci. 2021;6(1):9–20.