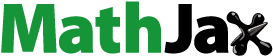
Abstract
Mathematical models based on computational fractional orders, employed for accurate modelling of complex dynamic systems, can ensure the implementation of various analytical, numerical and computing methods encompassing their applications to emerging and ever-varying real-world problems. Tracking, managing and controlling communicable diseases, one being monkeypox with different features, virological and taxonomic attributes, are oriented towards high-risk groups concerning global public health. This study, accordingly, is devoted to the presentation of the piecewise global derivative model of the monkeypox virus by applying the Caputo and Atangana Baleanu fractional-order derivatives in the partitioned two sub-intervals. The model includes eight compartments with two categories of human and rodent populations. The cases which take part in some sense for the said infection are investigated along with connection in this format. The existence and uniqueness of the solution in the framework of the piecewise global derivative are analyzed for both sub-intervals using fixed point theory. The detailed investigation of the dynamics of fractional-order systems and among many other dynamic features, stability is addressed. The stability of the solution is, thus, examined using the idea of Ulam Hyers concept. For the best fitting values of the parameters, the results are simulated using the monkeypox data. Using the method of Newton polynomial, different piecewise dynamics of each compartment are simulated on different fractional orders and time durations. This kind of a proposed approach is thought to lay a foundation where the transmission takes place to control epidemic events and other infectious medical conditions through vaccines or taking preventive measures to maintain and advance global public health while fully optimizing the clinical care of the diseases to manage complications, alleviate symptoms as well as prevent the long-term sequelae. This analysis also deals with sudden variation in monkeypox dynamics and also for crossover dynamics along with removal of discontinuity through modification of piecewise global analysis.
1. Introduction
Modern calculus derivatives, with their extensive applications in mathematical models, help for the understanding of viral infections, epidemic events and other unpredictable phenomena since they have more degrees of freedom than integer-order models. For this reason, fractional-order models are considered to be more precise and reliable, with fractional-order differential equation models appearing to be more compatible with the varying conditions compared to the integer-order ones [Citation1]. Compartmental models, furthermore, are regarded as one of the most frequently employed epidemic model types, related to both experimental and observation data. In these kinds of models, having a finite number of discrete states means that individuals manifest varying aspects, with some of the related states being merely labels which identify the various traits of the individuals affected under epidemiologically relevant conditions [Citation2]. Another perspective is that models based on computational fractional orders can be employed for accurate modelling of complex dynamic systems, which allows one to implement different analytical, numerical and computing methods that include the related applications to emerging and ever-varying real-world problems. As such, tracking, managing and controlling communicable diseases, one being monkeypox (Mpox) which has different features, virological and taxonomic attributes, inclined towards high-risk groups concerning global public health conditions.
The monkeypox is a disease present in animals which has spread the monkeypox infections into human society. As animal species are present all over the globe, it can be found and is communicable over the globe. It was first discovered in the forests of Africa, where forest species specially monkeys are higher compared to the number in the rest of the world. Subsequently, two infections of the said disease matching the pox in community of monkeys, which were then used for the investigation, led to the testing of monkeypox in 1958. For about 6 months of that year, many countries tested different cases of the monkeypox reporting to the World Health Organization (WHO). On 15 June 2022, 2104 cases were tested with clinical records having one death occurred and reported by WHO [Citation3,Citation4]. The said virus may be transmitted directly with contact of an infected scab, rash or fluids of the body are other means the virus may be transmitted from one body of human to another one. The transmission process occurs also through respiration secretion at the time of prolonged face-to-face connection or in sexual activities or any other abrupt physical connections [Citation3,Citation5,Citation6]. Initially, forest animals in Africa like rats and monkeys spread the said viruses to human population, and yet, person-to-person transmission is also mostly present in the reported cases. The transmission from the species to human population occurred as a result of bites or scratches, the process of bush meat, directly connected with body fluids, or used food eaten by rodents seem to be the main causes of the spread of the disease. Direct connection with sores and body fluids from infectious persons can also lead to the transmission of the disease in question. Accordingly, substantial amount of research has analyzed different approaches for the transmission of the said infection by semen and vaginal fluids or in respiration process drops [Citation3,Citation7]. While the signs of monkeypox indicate human to human transmission, it also includes other main signs such as headache, muscle pain, fever, backbone pain, swollen lymph nodes, chills and exhaustion. Mostly, the situation of monkeypox is said to be a commonly mature illness and most people are able to recover on their means after several weeks. Those who have a weak immune defensive system may have more challenging and tougher signs though [Citation3,Citation8]. The smallpox vaccines, anti-viral and vaccine immune globulin established to provide protection against smallpox may also be used as a cure to stop the transmission of monkeypox but there is currently no proven on time curve for the monkeypox virus disease. The vaccine is presently unavailable due to the fact that smallpox has been extinct worldwide [Citation9,Citation10].
Analysis of the new Monkeypox virus for the forecast and infectivity, the authors in [Citation11] established a deep learning algorithm and found the two animals of this virus which is bat and minks. Mostly, mathematical models have been an important role in the direct transmission between humans-to-humans in the outbreak. The infected people have a long incubation period, yet, it is likely that they may not be aware of the symptoms and do not know about the quarantine time. This new disease can easily spread to other people and many researchers have documented models on monkeypox on different levels [Citation12–14].
Much of the research has been geared towards the investigation of the dynamics of fractional-order systems and among many other dynamic features, stability has been reported [Citation15]. Concerned upon our work, we have re-considered the monkeypox mathematical model [Citation16], which has not been investigated under novel piecewise derivative and integrals operator before. The considered model has eight compartments having two categories namely: susceptible humans , exposed humans
, infected humans
, clinical infected humans
, recovered human
, susceptible rodents
, exposed rodents
, infected rodents
.
The monkeypox model is taken from [Citation16] which has the following form as indicated below: (1)
(1) along with initial conditions
where the given parameters in the model with descriptions are given in Table . The calculus of fractional order (FC) has attracted more interesting results from the researchers and scientist over the last 20 years [Citation17,Citation18]. FC, when compared with traditional integer-order models, is said to provide novel, accurate and deeper information on the complex activities of many infectious diseases and epidemic events with the mathematical models used as applicable schemes [Citation19–24]. Because of genetic properties and memory behaviours, integer order problems are not superior than FC problems, and fractional order differential equations employ nonlocal operators, which can be considered to be ideal tools in the mathematical modelling of systems with memory. Many kinds of integer order equations are used in mathematical models of the real world with the real phenomena possible to be analyzed for a higher degree of choices and precision by applying the fractional differential equations [Citation25–31]. The superiority of fractional-order models to classical ones has been demonstrated by several researchers from various branches of science, engineering and life sciences [Citation32–37]. Correspondingly, authors in [Citation38] used the Mittag–Leffler derivative and investigated the fractional infectious disease model. In [Citation39], the authors used Atangana–Baleanu–Caputo fractional derivative and studied a mathematical model of COVID-19. The authors in [Citation40] applied the Caputo–Fabrizio operator along with double Laplace transform and find the series solution for the fractional biological model. The scholars in [Citation41] applied a novel fractional order Lagrangian scheme to show the motion of the beam on nanowire. Liaqat et al. [Citation42] also established a new scheme to obtain the approximate and exact solution in the sense of Caputo fractional partial differential equation along with variable. Odibat and Baleanu [Citation43] studied a novel system of fractional differential equations with involving generalized fractional Caputo operator. The researchers have investigated many number of groundbreaking works employing various operators and applied them to infectious disease and real models [Citation44–50]. The framework of piecewise Caputo and Atangana–Baleanu operator is as follows: A novel operator for piecewise global derivative and integrals was given by Atangana and Araz [Citation51].
Table 1. Parameters and their description according to model Equation1(1)
(1) .
The piecewise derivative is divided into two subintervals, the first interval solution is find out in the sense of one fractional operator while the second interval solution is under the other fractional operator. To overcome cross-over behaviour challenges, one of the unique ways of piece-wise derivative has been proposed in [Citation51]. A new window of cross-over behaviours using these operators has been studied by researchers. Several uses of the fractional operators are investigated in the literature by different researchers [Citation52–56]. Inspired from the above novel operator, we will investigate the model taken from [Citation16] under the framework of piecewise Caputo and Atangana–Baleanu operator in the current study as follows: (2)
(2) In more depth, Equation (Equation2
(2)
(2) ) can be expressed as follows:
(3)
(3) The organization of the rest of this paper is as follows. The preliminaries and basic definitions are introduced in Section 2. Section 3 presents qualitative analysis: existence and uniqueness. Stability analysis with varying forms of function is handled in Section 4. Section 5 describes the numerical scheme for the fractional piecewise for the monkeypox model and Section 6 focuses on numerical simulations and analyses. Finally, Section 7 concludes the study with future directions.
2. Preliminaries and basic definitions
Certain definitions regarding Caputo and ABC fractional as well as piecewise derivatives and also integration are provided in this section of the study.
Definition 2.1
The definition of operator of function
with condition
[Citation14] is
(4)
(4) One can replace
by
in (Equation4
(4)
(4) ), obtain the Caputo–Fabrizio operator.
Definition 2.2
Consider , then
integral [Citation14] is
(5)
(5)
Definition 2.3
The Caputo operator of function [Citation15] is
Definition 2.4
Suppose is piecewise differentiable, then piecewise derivative with Caputo and ABC operators [Citation38] is
where
represents piecewise differential operator, where Caputo operator is in interval
and ABC operator in interval
.
Definition 2.5
Suppose is piecewise integrable, then piecewise derivative with Caputo and ABC operators [Citation38] is
where
represents piecewise integral operator, where Caputo operator is in interval
and ABC operator in interval
.
3. Qualitative analysis: existence and uniqueness
The qualitative analysis of the proposed model in the format of piecewise derivative can be found in this section of the paper where we shall now determine whether a solution exists for the hypothetical piecewise derivable function as well as its specific solution attribute or not. To do this, we may use the system (Equation3(3)
(3) ), and we can also write the following by way of further explanation:
is defined as
(6)
(6) where
(7)
(7) where
. Taking
and the Banach space
with a norm
We assume the following growth condition:
∃
; ∀
we have
∃
;
Theorem 3.1
If be piece-wise continuous on
and
on
, also satisfy
, then (Equation3
(3)
(3) ) has at least one solution.
Proof.
Let's use the Schauder theorem to define a closed sub-set as and X in both subintervals of
.
Suppose
and using (Equation6
(6)
(6) ) as
(8)
(8) Any
, we have
As we derived that
, so
This shows that
is bounded. For complete continuity, we proceed as by taking
, the first interval in the Caputo sense as
(9)
(9) Next (Equation9
(9)
(9) ), we obtain
, then
So
is equi-continuous in
. Consider
in ABC sense for the second interval as
(10)
(10) If
, then
This shows that
is equi-continuous, so by Arzel'a theorem
is completely continuous and bounded. Thus our derivation shows that the proposed piecewise model has at least one solution in both subintervals.
Theorem 3.2
If the operator is contraction along with assumption
then the proposed system has unique solution.
Proof.
As piece-wise continuous, consider
and
on
in sense of Caputo as
(11)
(11) From (Equation11
(11)
(11) ), we have
(12)
(12) This shows that
is contraction and the proposed system has unique solution.
Also in the sense of ABC derivative as
(13)
(13) or
(14)
(14) This is why
is contraction. As a result, the issue under consideration has a singleton solution in the provided subinterval in light of the Banach result. So (Equation12
(12)
(12) ) and (Equation14
(14)
(14) ) the suggested problem has unique solution on each sub-intervals.
4. Stability analysis with varying forms of function
The proving of the H–U stability and different forms is provided herein for our considered model.
Definition 4.1
Our proposed model (Equation1(1)
(1) ) is U–H stable, if for each
, and the inequality
(15)
(15) unique solution
exists with a constant
,
(16)
(16) In addition, if a nondecreasing function
for the inequality presented above
(17)
(17) it is fated
, then obtained solution is generally U–H stable.
Definition 4.2
Our considered model Equation2(2)
(2) is H–U–R stable if
, for each
, and inequality
(18)
(18) unique solution
with constant
, so that
(19)
(19) Anew, if
is existent, for the inequality
(20)
(20) unique solution exists
with constant
, so
(21)
(21) then the obtained solution is generally H–U–R stable.
Remark 4.1
Suppose a function does not depend upon
, and
, then
Lemma 4.3
Suppose the function
(22)
(22) The solution of (Equation22
(22)
(22) ) is
(23)
(23)
(24)
(24)
Theorem 4.4
In the light of Lemma (4.3) if the condition satisfies, then the solution of our considered model (Equation2
(2)
(2) ) is H–U as well as generalized H–U stable.
Proof.
Let us suppose is the solution of Equation2
(2)
(2) also
is a unique solution of (Equation2
(2)
(2) ), so we have
Case:1 for , we have
On further simplification
(25)
(25) Case:2
By further simplification and using
we have
we have
using
At this point, from Equations (Equation25
(25)
(25) ) and (Equation26
(26)
(26) ), we have
(26)
(26) So the solution of model Equation2
(2)
(2) is H–U stable. Also if we replace Φ by
then from (Equation26
(26)
(26) ),
Now
shows that the solution of our proposed model Equation2
(2)
(2) is generalized H–U stable.
We define the remark to conclude the Rassias stability results and also the generalized form.
Remark 4.2
Suppose a function does not depend upon
, and
, then
Lemma 4.5
Solution to the model
hold the relation given below
(27)
(27) where
.
With the help of Remark 4.2, one can get Equation (Equation27(27)
(27) ).
Theorem 4.6
The solution of model (Equation27(27)
(27) ) is H–U–R stable if the following conditions hold:
For each
and a constant
, we get
For each
and constant
, we get
Proof.
We prove these results in two cases.
Case:1 for , we have
On further simplification,
(28)
(28) Case:2
By further simplification and using
we have
(29)
(29) we have
using
At that point, from equations (Equation28
(28)
(28) ) and (Equation29
(29)
(29) ), we have
So the solution of model Equation2
(2)
(2) is H–U–R stable.
Remark 4.3
Suppose a function does not depend upon
, and
, then
Theorem 4.7
In light of ,
, Remark 4.3 and 4.5, the solution of model Equation2
(2)
(2) is generalized H–U–R stable, if
.
Where
For each
and constant
, we get
and
For each
and constant
, we get
Proof.
We have obtained our results in two cases:
Case 1 for , we have
On further simplification,
(30)
(30) Case 2
By further simplification and using
we have
(31)
(31) we have
using
At this juncture, from Equations (Equation30
(30)
(30) ) and (Equation31
(31)
(31) ), we have
So the solution of the model (Equation2
(2)
(2) ) is generalized H–U–R stable.
5. Numerical scheme for the fractional piecewise for the monkeypox model
In this section, the numerical scheme for the following monkeypox according to model (Equation2(2)
(2) ) is given.
(32)
(32) By applying the piece-wise integral to the Caputo and ABC derivative, we obtain
(33)
(33) At
(34)
(34) We put the Newton polynomials, so the following is obtained:
(35)
(35)
Similarly, for the remaining compartments we get (36)
(36)
(37)
(37)
(38)
(38)
(39)
(39)
(40)
(40)
(41)
(41)
(42)
(42)
Here
and
With the aforementioned application steps in line with the considered model, we have obtained our results accordingly. All the analyses and numerical simulation results obtained have been performed with MATLAB software [Citation57].
6. Numerical simulations and analysis
In this section, the development of the numerical simulation is provided for all the eight agents of the considered problem along with the initial data and parameters values mentioned in Table and taken from [Citation16]. The initial population is . The graphical representation of all the eight quantities of the considered problem has been shown on three different data of fractional orders and time under piecewise Caputo and ABC derivative. The first agent of susceptible human population is shown in Figure (a)–(c) respectively, on two sub intervals with the bending terminal point of the first interval. The said class population decreases slowly in the first interval while in second interval it becomes stable. On small fractional orders, it becomes stable quickly and piece wise behaviours are negligible in this case as shown in Figure (c). The second agent of expose human population is shown in Figure (a)–(c), respectively on two subintervals with the bending terminal point of the first interval. The said class population first increases slowly in the first interval reaching to its peak value and then moving towards stability. On small fractional orders, it becomes stable quickly and piecewise behaviours are negligible in this case as shown in Figure (a) and (c). The third quantity of infected human population is shown in Figure (a)–(c), respectively on two subintervals with the bending terminal point of the first interval. The said class population first increases slowly in the first interval reaching to its peak value and then moving towards stability. On small fractional orders, it becomes stable quickly and piece wise behaviours are negligible in this case as shown in Figure (c). On large fractional orders, the curves are far away from each other while on small they are close to each other. Next we simulate the fourth quantity of clinically human infected population is shown in Figure (a)–(c), respectively on two subintervals with the bending curves on terminal point of the first interval. The said class population first increases quickly in the first interval reaching to its peak value and then moving towards stability. On small fractional orders, it becomes stable quickly and piece wise behaviours are negligible in this case as shown in Figure (c). On large fractional orders, the curves are far away from each other while on small they are close to each other.
Table 2. Parameters and their description according to model (Equation2(2)
(2) ).
Figure 1. Dynamical behaviours of susceptible human individuals on different arbitrary fractional orders
and time durations on sub interval
and
of
.
![Figure 1. Dynamical behaviours of susceptible human individuals Sh(t) on different arbitrary fractional orders κ and time durations on sub interval [0,t1] and [t1,T] of [0,T].](/cms/asset/6f86ec11-f7de-49a1-bdf6-16f51a00b5ee/gipe_a_2300330_f0001_oc.jpg)
Figure 2. Dynamical behaviours of Exposed human individuals on different arbitrary fractional orders
and time durations on sub interval
and
of
.
![Figure 2. Dynamical behaviours of Exposed human individuals Eh(t) on different arbitrary fractional orders κ and time durations on sub interval [0,t1] and [t1,T] of [0,T].](/cms/asset/7015be6e-d168-4270-be5b-7c0e6445cfee/gipe_a_2300330_f0002_oc.jpg)
Figure 3. Dynamical behaviours of infected human individuals on different arbitrary fractional orders
and time durations on sub interval
and
of
.
![Figure 3. Dynamical behaviours of infected human individuals Ih(t) on different arbitrary fractional orders κ and time durations on sub interval [0,t1] and [t1,T] of [0,T].](/cms/asset/bc05cbd9-32cd-4039-ab85-fcd01c04b93d/gipe_a_2300330_f0003_oc.jpg)
Figure 4. Dynamical behaviours of clinical ill human individuals on different arbitrary fractional orders
and time durations on sub interval
and
of
.
![Figure 4. Dynamical behaviours of clinical ill human individuals Ch(t) on different arbitrary fractional orders κ and time durations on sub interval [0,t1] and [t1,T] of [0,T].](/cms/asset/6da5156a-9ccb-41f3-b287-e1b2d61a2893/gipe_a_2300330_f0004_oc.jpg)
Next we simulate the fifth quantity of the Recovered human population is shown in Figure (a)–(c) respectively on two subintervals with the bending curves on terminal point of the first interval. The said class population increases gradually in the first as well as in the second interval when the infected human recovered. On large fractional orders, the curves are far away from each other while on small they are close to each other. As the last related stage, we simulate the last quantity of infected rodent population as shown in Figure (a)–(c) respectively on two subintervals with bending curves on terminal point of the first interval. The said class population first increases quickly in the first interval reaching to its peak value and then decreasing moving towards stability. On small fractional orders, it becomes stable quickly and piecewise behaviours are negligible in this case as shown in Figure (c). On large fractional orders, the curves are far away from each other while on small they are close to each other. Next, we simulate the sixth quantity of susceptible rodent population is shown in Figure (a)–(c), respectively on two subintervals with the bending curves on terminal point of the first interval. The said class population first declines quickly in the first interval and in the second interval and then moves towards stability. On small fractional orders it becomes stable quickly and piecewise behaviours are negligible in this case as shown in Figure (c). On large fractional orders, the curves are far away from each other while on small they are close to each other. Next, we simulate the seventh quantity of exposed rodent population as shown in Figure (a)–(c), respectively on two subintervals with the bending curves on terminal point of the first interval. The said class population first increases quickly in the first interval reaching to its peak value and then moving towards stability. On small fractional orders, it becomes stable quickly and piecewise behaviours are negligible in this case as shown in Figure (c). On large fractional orders, the curves are far away from each other while on small they are close to each other.
Figure 5. Dynamical behaviours of Recovered human individuals on different arbitrary fractional orders
and time durations on sub interval
and
of
.
![Figure 5. Dynamical behaviours of Recovered human individuals Rh(t) on different arbitrary fractional orders κ and time durations on sub interval [0,t1] and [t1,T] of [0,T].](/cms/asset/6c201716-cb44-43bb-af16-1235857faa33/gipe_a_2300330_f0005_oc.jpg)
7. Conclusion and future directions
The investigated work developed for addressing the considered problem of piecewise monkeypox infection, which is composed of eight agents for monkeypox virus under the fractional derivatives of Caputo and Atangana Baleanu in the sub-partitioned scheme. Through our study, we have proven that all the quantities are converging and quickly stabilized on small fractional orders as given in the numerical simulation section. The important theoretical, numerical and simulation-related properties have been presented for the considered model. By the application of fixed point results, we have derived results which deal with existence and uniqueness of solution for both sub intervals in the sense of Caputo and Atangana Baleanu operators. The Ulam–Hyers stability concept on both interval has also been derived. The Newton Polynomial technique has been utilized for computing numerical solution of the piecewise fractional model of monkeypox virus. Consequently, we have observed that the piecewise data provide more information describing crossover dynamics for different fractional orders, and the graphical results present thought-provoking information regarding both piecewise and fractional order analysis. Based on these derived results, control measures and strategies to curb the disease and prevent future occurrences can be proposed as one of the future directions. Another direction can be concerned with the further investigation of the new dynamics of the viruses under examination through newly designed computational fractional models. Furthermore, the efficient numerical techniques can be sought and adapted to verify the theoretical aspects as well as findings related to the dynamic behaviour of diseases. The kind of schemes we used can allow one to implement various analytical, numerical and computing methods by including their applications in emerging real-world problems. The effective management and control of communicable diseases, like monkeypox handled in this study, with different features, virological attributes and varying characteristics concern high-risk groups concerning broader society and global public health.
Disclosure statement
No potential conflict of interest was reported by the author(s).
References
- Okyere S, Ackora-Prah J. Modeling and analysis of monkeypox disease using fractional derivatives. Results Eng. 2023;17:100786. doi:10.1016/j.rineng.2022.100786
- ur Rahman M, Alhawael G, Karaca Y. Compartmental analysis of middle eastern respiratory syndrome coronavirus model under fractional operator with next-generation matrix methods. Fractals. 2023.
- Centers for Disease Control and Prevention 2021 National Center for Emerging and Zoonotic Infectious Diseases(NCEZID), Division of High-Consequence Pathogens and Pathology (DHCPP), Monkeypox, accessed: November 10, 2021.
- Bunge EM, Hoet B, Chen L, et al. The changing epidemiology of human monkeypox potential threat a systematic review. PLoS Negl Trop Dis. 2022;16(2):e001014. doi:10.1371/journal.pntd.0010141
- Durski KN, McCollum AM, Nakazawa Y, et al. Emergence of monkeypox West and Central Africa, 1970–2017. Morb Mortal Wkly Rep. 2018;67(10):306–310. doi:10.15585/mmwr.mm6710a5
- Jezek Z, Szczeniowski M, Paluku K, et al. Human monkeypox: confusion with chickenpox. Acta Trop. 1988;45:297–307.
- Alakunle E, Moens U, Nchinda G, et al. Monkeypox virus in Nigeria: infection biology, epidemiology, and evolution. Viruses. 2020;12(11):1257. doi:10.3390/v12111257
- Nguyen PY, Ajisegiri WS, Costantino V, et al. Reemergence of human monkeypox and declining population immunity in the context of urbanization, Nigeria. Emerg Infect Dis. 2021;27:1007.
- Nigeria Centre for Disease Control, Monkeypox outbreak situation report, accessed: November 10, 2021, 2021.
- Centers for Disease Control and Prevention, National Center for Emerging and Zoonotic Infectious Diseases (NCEZID), Division of High-Consequence Pathogens and Pathology (DHCPP), Monkeypox, accessed: November 11, 2021, 2021.
- Guo Q, Li M, Wang C, et al. Host and infectivity prediction of Wuhan 2019 novel coronavirus using deep learning algorithm, BioRxiv, 2020.
- Peter OJ, Oguntolu FA, Ojo MM, et al. Fractional order mathematical model of monkeypox transmission dynamics. Phys Scr. 2022;97(8):084005. doi:10.1088/1402-4896/ac7ebc
- Majee S, Jana S, Barman S, et al. Transmission dynamics of monkeypox virus with treatment and vaccination controls: A fractional order mathematical approach. Phys Scr. 2023;98(2):024002. doi:10.1088/1402-4896/acae64
- Liu P, ur Rahman M, Din A. Fractal fractional based transmission dynamics of COVID-19 epidemic model. Comput Methods Biomech Biomed Engin. 2022;25:1–18.
- Wang W, Qiao Y, Miao J, et al. Dynamic analysis of fractional-order recurrent neural network with caputo derivative. Int J Bifurc Chaos. 2017;27(12):1750181. doi:10.1142/S0218127417501814
- Peter OJ, Oguntolu FA, Ojo MM, et al. Fractional order mathematical model of monkeypox transmission dynamics. Phys Scr. 2022;97(8):084005. doi:10.1088/1402-4896/ac7ebc
- Podlubny I. Fractional differential equations. New York: Academic Press; 1999. (Mathematics in Science and Engineering; Vol. 340).
- Sar EY, Giresunlu IB. Fractional differential equations. Pramana J Phys. 2016;87:17. doi:10.1007/s12043-016-1225-7
- Shen WY, Chu YM, ur Rahman M, et al. Mathematical analysis of HBV and HCV co-infection model under nonsingular fractional order derivative. Results Phys. 2021;28:104582. doi:10.1016/j.rinp.2021.104582
- Zhang L, ur Rahman M, Arfan M, et al. Investigation of mathematical model of transmission co-infection TB in HIV community with a non-singular kernel. Results Phys. 2021;28:104559. doi:10.1016/j.rinp.2021.104559
- Baleanu D, Jajarmi A, Mohammadi H, et al. A new study on the mathematical modelling of human liver with Caputo–Fabrizio fractional derivative. Chaos Solit Fractals. 2020;134:109705. doi:10.1016/j.chaos.2020.109705
- Defterli O, Baleanu D, Jajarmi A, et al. Fractional treatment: an accelerated mass-spring system. Rom Rep Phys. 2022;74:1–13.
- Baleanu D, Shekari P, Torkzadeh L, et al. Stability analysis and system properties of Nipah virus transmission: a fractional calculus case study. Chaos Solit Fractals. 2023;166:112990. doi:10.1016/j.chaos.2022.112990
- Owolabi KM, Pindza E. A nonlinear epidemic model for tuberculosis with Caputo operator and fixed point theory. Healthcare Anal. 2022;2:100111. doi:10.1016/j.health.2022.100111
- Li B, Zhang T, Zhang C. Investigation of financial bubble mathematical model under fractal–fractional Caputo derivative. FRACTALS (fractals). 2023;31:1–13.
- Agarwal P, Deniz S, Jain S, et al. A new analysis of a partial differential equation arising in biology and population genetics via semi analytical techniques. Phys A Stat Mech Appl. 2020;542:122769. doi:10.1016/j.physa.2019.122769
- Rashid S, Tul Kubra K, Sultana S, et al. An approximate analytical view of physical and biological models in the setting of Caputo operator via Elzaki transform decomposition method. J Comput Appl Math. 2022;413:114378. doi:10.1016/j.cam.2022.114378
- Chowdhury SMEK, Forkan M, Ahmed SF, et al. Modeling the SARS-CoV-2 parallel transmission dynamics: asymptomatic and symptomatic pathways. Comput Biol Med. 2022;143:105264. doi: 10.1016/j.compbiomed.2022.105264
- Shams M, Kausar N, Samaniego C, et al. On efficient fractional caputo-Type simultaneous scheme for finding all roots of polynomial equations with biomedical engineering applications. Fractals. 2023;31(04):2340075. doi:10.1142/S0218348X23400753
- Hassani H, Avazzadeh Z, Tenreiro Machado JA, et al. Optimal solution of a fractional HIV/AIDS epidemic mathematical model. J Comput Biol. 2022;29(3):276–291. doi:10.1089/cmb.2021.0253
- He Q, Zhang X, Xia P, et al. A comparison research on dynamic characteristics of Chinese and American energy prices. J Glob Inf Manag. 2023;31:1–16.
- Owolabi KM, Shikongo A. Fractal fractional operator method on HER2+ breast cancer dynamics. Int J Appl Comput Math. 2021;7(3):85. doi:10.1007/s40819-021-01030-5
- Durur Hülya, Yokuş Aıf, Yavuz M. Behavior analysis and asymptotic stability of the traveling wave solution of the Kaup-Kupershmidt equation for conformable derivative. Fract Calc New Appl Underst Nonlinear Phenom. 2022;3:162.
- Karaagac B, Owolabi KM, Nisar KS. Analysis and dynamics of illicit drug use described by fractional derivative with Mittag-Leffler kernel. CMC-Comput Mater Cont. 2020;65:1905–1924.
- Martínez-Farías FJ, Alvarado-Sánchez Aí, Rangel-Cortes E, et al. Bi-dimensional crime model based on anomalous diffusion with law enforcement effect. Math Model Numer Simul Appl. 2022;2:26–40.
- Naik PA, Zu J, Owolabi KM. Global dynamics of a fractional order model for the transmission of HIV epidemic with optimal control. Chaos Solit Fractals. 2020;138:109826. doi:10.1016/j.chaos.2020.109826
- Li B, Eskandari Z, Avazzadeh Z. Strong resonance bifurcations for a discrete-time prey–predator model. J Appl Math Comput. 2023;69:1–18.
- Xuan L, ur Rahmamn M, Ahmad S, et al. A new fractional infectious disease model under the non-singular Mittag–Leffler derivative. Waves Random Complex Media. 2022;1–27. doi:10.1080/17455030.2022.2036386.
- Thabet STM, Abdo MS, Shah K, et al. Study of transmission dynamics of COVID-19 mathematical model under ABC fractional order derivative. Results Phys. 2020;19:103507. doi:10.1016/j.rinp.2020.103507
- ur Rahman M, Althobaiti A, Riaz MB, et al. A theoretical and numerical study on fractional order biological models with Caputo Fabrizio derivative. Fractal Fract. 2022;6(8):446. doi:10.3390/fractalfract6080446
- Erturk VS, Godwe E, Baleanu D, et al. Novel fractional-order lagrangian to describe motion of beam on nanowire. Acta Phys Pol A. 2021;140(3):265–272. doi:10.12693/APhysPolA.140.265
- Liaqat MI, Etemad S, Rezapour S, et al. A novel analytical Aboodh residual power series method for solving linear and nonlinear time-fractional partial differential equations with variable coefficients. AIMS Math. 2022;7(9):16917–16948. doi:10.3934/math.2022929
- Odibat Z, Baleanu D. Nonlinear dynamics and chaos in fractional differential equations with a new generalized Caputo fractional derivative. Chin J Phys. 2022;77:1003–1014. doi: 10.1016/j.cjph.2021.08.018
- Abidemi A, Owolabi KM, Pindza E. Modelling the transmission dynamics of Lassa fever with nonlinear incidence rate and vertical transmission. Phys A Stat Mech Appl. 2022;597:127259. doi:10.1016/j.physa.2022.127259
- Yavuz M, Ozköse F, Akman Müzeyyen, et al. A new mathematical model for tuberculosis epidemic under the consciousness effect. Math Model Control. 2023;3(2):88–103. doi:10.3934/mmc.2023009
- Karaagac B, Owolabi KM. Numerical analysis of polio model: A mathematical approach to epidemiological model using derivative with Mittag–Leffler kernel. Math Methods Appl Sci. 2023;46(7):8175–8192. doi:10.1002/mma.v46.7
- Gülnur YEL, Kayhan Mç, Ciancio A. A new analytical approach to the (1+ 1)-dimensional conformable fisher equation. Math Model Numer Simul Appl. 2022;2(4):211–220.
- Naik PA, Owolabi KM, Zu J, et al. Modeling the transmission dynamics of COVID-19 pandemic in Caputo type fractional derivative. J Multiscale Model. 2021;12(3):2150006. doi:10.1142/S1756973721500062
- Duran S, Durur H, Yavuz M, et al. Discussion of numerical and analytical techniques for the emerging fractional order murnaghan model in materials science. Opt Quantum Electron. 2023;55(6):571. doi:10.1007/s11082-023-04838-1
- Li B, Eskandari Z, Avazzadeh Z. Dynamical behaviors of an SIR epidemic model with discrete time. Fractal Fract. 2022;6(11):659. doi:10.3390/fractalfract6110659
- Atangana A, Araz SI. New concept in calculus: piecewise differential and integral operators. Chaos Solit Fractals. 2021;145:110638. doi:10.1016/j.chaos.2020.110638
- Sohail A, Yu Z, Arif R, et al. Piecewise differentiation of the fractional order CAR-T cells-SARS-2 virus model. Results Phys. 2022;33:105046. doi:10.1016/j.rinp.2021.105046
- Atangana A, Toufik M. A piecewise heat equation with constant and variable order coefficients: A new approach to capture crossover behaviors in heat diffusion. AIMS Math. 2022;7(5):8374–8389. doi:10.3934/math.2022467
- Heydari MH, Razzaghi M. A numerical approach for a class of nonlinear optimal control problems with piecewise fractional derivative. Chaos Solit Fractals. 2021;152:111465. doi:10.1016/j.chaos.2021.111465
- Shah K, Abdeljawad T, Ali A. Mathematical analysis of the Cauchy type dynamical system under piecewise equations with Caputo fractional derivative. Chaos Solit Fractals. 2022;161:112356. doi:10.1016/j.chaos.2022.112356
- ur Rahman M, Arfan M, Baleanu D. Piecewise fractional analysis of the migration effect in plant-pathogen-herbivore interactions. Bull Biomath. 2023;1(1):1–23. doi:10.59292/bulletinbiomath.2023001
- MATLAB, 2022 version 9.12.0 (R2022a). The Math-Works Inc., Natick, Massachusetts.