Abstract
Purpose
Motor symptoms of patients suffering from Parkinson’s disease (PD) are currently mainly treated with dopaminergic pharmacology, and where indicated, with deep brain stimulation. In the last decades, a substantial body of literature has described neurophysiological correlates related to both motor symptoms and treatment effects. These mechanistic insights allow, at least theoretically, for precise targeting of neural processes responsible for PD motor symptoms.
Materials and methods
Literature search was conducted to identify electrophysiological and hemodynamic signals that may serve as neural targets for future neurofeedback training protocols.
Results
In particular alpha, beta and gamma oscillations over the motor cortex show high potential as neural targets for electrophysiological neurofeedback training. Hemodynamic functional magnetic resonance imaging (fMRI) with higher spatial resolution provides additional insights about network activity between cortical and subcortical brain regions in response to established treatments. fMRI based neurofeedback training (NFT) further allows targeting involved networks. Hemodynamic functional near infrared spectroscopy (fNIRS) may be a suitable transfer technology for more and cost-efficient hemodynamic NFT.
Conclusions
This scoping review presents summarises neural markers that may be promising for NFT interventions that are informed by validated neural circuit models. Recommendations for best practice in study design and reporting are provided, highlighting the importance of adequate control conditions and statistical power.
1. Introduction
Idiopathic Parkinson’s disease (PD) is the second most common neurodegenerative disease, affecting 1% of people over the age 60 and 3% of people over 80 years [Citation1]. Cardinal symptoms in early PD stages include tremor (shaking of limbs or the head), rigidity (stiffness of joints), bradykinesia (slowing of movements) and in later stages akinesia (absence of movement), as well as postural instability. These motor symptoms form the basis of the clinical diagnosis of Parkinson’s disease. Other highly prevalent motor symptoms include motility dysfunction of the gastrointestinal system and dysphagia (swallowing difficulties) [Citation2,Citation3], which have been suggested to indicate prodromal PD stages, and also worsen with disease progression [Citation4]. Besides motor symptoms, PD patients are also affected by severe non-motor symptoms that increase with disease progression. These include neuropsychiatric symptoms such as depression, sensory deficits and rapid eye movement sleep behaviour disorder (RBD) as a prodromal syndrome [Citation5,Citation6]. Cognitive deficits, including memory loss, attention deficits and dementia, manifest in 80–90% of late-stage PD patients [Citation7,Citation8]. More recent work has further investigated the relationship between different motor phenotypes and non-motor symptoms [Citation7,Citation9]. Taken together, the neurodegenerative processes occurring in PD affect multiple systems that impact patients’ quality of life substantially [Citation10]. Current established treatment options mainly consist of physiotherapy, pharmacotherapy targeting the dopaminergic system and deep brain stimulation (DBS) of certain basal ganglia structures [Citation11–13]. Electrophysiological studies following pharmacotherapy or DBS treatment have provided rich insights into the circuit pathophysiology of PD. Here, I will describe electrophysiological markers that have been reported to indicate improvements of motor symptoms following established PD treatment. Some of these have been targeted with brain-machine interfaces, including both invasive and non-invasive neurofeedback training (NFT). A recent systematic review on NFT for PD found that most patients were able to self-regulate their own brain activity. However, published studies thus far often lack rigorous study designs (e.g., control conditions), as well as adequate outcome assessment and statistical reporting [Citation14]. The NFT literature for PD is thus likely too premature to evaluate advantages and disadvantages of different approaches based on empirical data. The available literature on established therapies offers many lessons that neurorehabilitation interventions such as NFT can learn from to determine promising neural targets. Hence, the focus of this scoping review will be to provide an overview of identified (pathophysiological) mechanisms with the aim to provide Bacomicists with suggestions for potential neural targets.
2. Revisiting neuroanatomy – a three-way conversation
The underlying molecular neuropathology of PD is complex and affects multiple neurotransmitter systems including the serotonergic raphe nuclei, the cholinergic nucleus basalis, as well as the dopaminergic midbrain [Citation15]. Central to the study of key motor symptoms and established pharmacological treatment are changes of the dopaminergic midbrain, which occur in consequence of cell death of dopaminergic cells in the substantia nigra pars compacta (SNc). The SNc forms an integral part of the basal ganglia circuits (). Two pathways between the SNc and the putamen have been identified that act via (D1 receptor mediated) excitatory and (D2 receptor mediated) inhibitory pathways on the putamen. In healthy individuals, excitatory dopaminergic simulation from the SNc to the putamen leads to inhibition of the globus pallidus internus (GPi) and the disinhibition of the thalamus. In consequence, the thalamus sends more glutamatergic excitatory projections to the cortex (conventionally labelled the direct pathway). With increasing loss of dopaminergic cells in progressing PD, this pathway fails to disinhibit the thalamus and reduces excitatory stimulation of the cortex.
Figure 1. Schematic diagram of basal ganglia circuitry in healthy (A) and PD patients (B). Arrows indicate excitatory projections, dots inhibitory projections, their respective width indicates the relative strength of these projections. Green: direct pathway; orange: indirect pathway; brown: hyperdirect pathway; grey: ubiquitous projections.
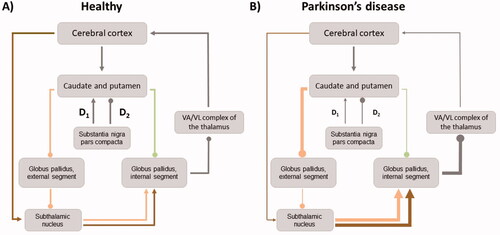
A second pathway (conventionally labelled the indirect pathway) comprises inhibitory projections from the SNc to the putamen, which disinhibit globus pallidus externus (GPe). As a result, the GPe disinhibits the thalamus, which itself is suppressed in its activity via the GPi (as described above). Loss of dopaminergic projections from the SNc result in an imbalance such that the (movement suppressing) indirect pathway becomes hyperactive and the (movement disinhibiting) direct pathway becomes hypoactive (). Moreover, projections between the STN and motor cortices mediate the activation and suppression of motor programmes by interacting with the GPi and SNr [Citation16]. This third pathway (conventionally labelled the hyperdirect pathway) comprises projections from the supplementary motor area (SMA) and the primary motor cortex (M1) to the STN. Although the role of the hyperdirect pathway in the development of motor deficits is currently less well understood than that of the direct and indirect pathway [Citation17], it likely promotes voluntary movements in healthy individuals dependent on dopamine availability [Citation18]. For instance, disturbances of the pathway due to dopaminergic loss contribute to poverty, slowness and eventually absence of movement in PD patients [Citation19,Citation20]. Moreover, it is involved in context specific cognitive adaptation of motor execution [Citation21–23].
3. Established treatment for motor symptoms in PD
Physiotherapy treatment aims to improve key physical capacities in PD patients. Besides standard physiotherapy, several other techniques, including treadmill training, dance or tai chi have been found effective. Main improvements have been reported for reducing rigidity, bradykinesia, disbalance, but also non-motor symptoms in both short- and long-term follow-up assessments [Citation11,Citation24,Citation25]. As an active intervention that involves physical activity, individual treatment regimens require adjustment to the specific disease stage. Physiotherapy is thus used to complement pharmacotherapy and neurosurgical (DBS) treatment, in particular to maintain activities of daily living and alleviate disease burden for patients.
Pharmacological treatment represents the main treatment for PD and focuses predominantly on compensating for the loss of dopaminergic SNc neurons. Different pharmacological agents that act on the dopaminergic circuitry from different angles are available. These include dopamine receptor agonists that mimic dopamine effects, monoamine oxidase inhibitors (MAOI) that prolong the presence of available dopamine, and Levodopa (L-Dopa) as a precursor amino acid of dopamine that passes through the blood-brain barrier and is converted into Dopamine in the brain. L-Dopa treatment, however, may lead to involuntary excessive movements (dyskinesia). Up to 40% of patients may experience dyskinesia after 4–6 years of L-Dopa treatment [Citation26]. Moreover, apathy is observed in up to over 20% of patients [Citation27]. To minimise the risk for such motor complications, guidelines recommend using receptor agonists or MAOI in early disease stages, thereby postponing treatment with L-Dopa to later disease stages [Citation12]. On the other hand, dopamine agonists may have adverse side effects including somnolence in up to 50%, and neuropsychiatric symptoms such as hallucinations and impulse control disorder in up 7% [Citation28]. Overall, pharmacological treatment is an essential component in managing motor symptoms in PD patients. However, with increasing neurodegeneration, pharmacotherapy and in particular L-dopa, becomes less effective in reducing motor symptoms, and dyskinesia increasingly develops as a side effect [Citation29]. Therefore, additional treatment options that allow managing motor symptoms while limiting the need for pharmacotherapy dosages would be beneficial.
Invasive deep brain stimulation (DBS) is particularly suitable for individuals for whom pharmacological treatment (and adjunctive physiotherapy) do not provide sufficient improvement of motor symptoms, or where medication associated dyskinesia becomes unbearable [Citation30]. DBS involves the implantation of electrodes whose activity is controlled by a battery-operated implantable pulse generator. The procedure involves stereotactic neurosurgery, which is mainly feasible for patients who will tolerate it. DBS stimulation protocols mostly operate with high-frequency stimulation (∼140Hz) of the STN, but also the GPi and thalamus may be targeted and provide good clinical outcomes in reducing resting tremors. Gait and posture symptoms can be effectively treated via DBS of the penduculopontine nucleus [Citation31]. Optimal stimulation frequencies are determined in patients individually during follow-up phase [Citation30]. DBS has been found highly effective in reducing motor symptoms with 30–70% improvement for rigidity, 60–90% improvement of resting tremor, and 30–70% on global scale of motor impairment (the modified Unified Parkinson’s Disease Rating Scale motor subscale; UDPRS-III), which comprises also other symptoms besides rigidity and resting tremor. As a result, patients’ medication dose (measured in the equivalence of L-Dopa) can be decreased substantially (20–65%), and hence remarkably fewer patients (40–90%) are affected by medication related dyskinesia [Citation30]. With regards to side effects following DBS treatment, haemorrhages are a leading implantation related complication, and prevalence varies between centres with less than 1 and up to 12% [Citation30]. Stimulation related neurological side-effects such as dyskinesia, but also rigid-hypokinetic dysarthria (poor articulation of phonemes) or hypophonia (soft speech due to a lack of coordination in the vocal musculature), have been reported to occur in 4–17% of patients [Citation30]. However, they are mostly transient and can be reversed, e.g., by adjusting the stimulation protocol. Another frequent stimulation side-effect of STN DBS in particular is acute depression with a prevalence in 25–35% [Citation30,Citation32]. Mood disturbances remain difficult to predict, besides the stimulation, also the presurgical psychological well-being of patients and changes in dopaminergic medication are main factors [Citation32]. With regards to potential mechanism driving mood changes, simulation of non-motor areas may be one main cause. For instance, one recent study reported an association between worsening of symptoms and the structural connectivity between the STN and the left prefrontal cortex [Citation33]. More precise targeting via segmented electrodes and intermittent stimulation based on patients’ needs via adaptive DBS may reduce DBS side effects [Citation32]. Altogether, DBS treatment provides effective reduction of key PD motor symptoms and can reduce motor complications that result from dopamine replacement therapy. However, the risk of side effects and potential contraindications against the neurosurgical procedure needs to be considered individually.
Pharmacological and electrophysiological studies have provided important mechanistic insights into the pathophysiology of PD. For instance, pharmacological studies in PD patients and lesion studies in PD animal models allowed assessing the relationship between dopamine depletion, motor deficits typically observed in PD patients, and their (cortical) neural correlates. DBS studies that report simultaneous electrophysiological recordings further allowed probing changes of subcortical and cortical neural oscillations in response to DBS. The DBS literature thus allows refining available mechanistic models of the pathophysiology in PD, incorporating brain network dynamics, and allowing to formulate directed hypotheses [Citation34]. Identified circuit mechanisms of PD can thus substantially inform the design of other interventions that aim to reduce dysfunctional or promote functional neural activity, including NFT.
4. Oscillations that (may) work – three candidates
Beta band oscillations (13–30Hz) have received particular interest in PD research and are linked to both motor symptom severity and pharmacological treatment effects. In healthy and PD patients, beta oscillation measured over central electrodes (C3/C4) show a desynchronisation during motor execution, which is reflected in a power/amplitude decrease. However, the reduction in beta amplitude is less in PD patients compared to healthy individuals, resulting in exaggerated beta synchronisation [Citation35]. Beta synchronisation facilitates stopping behaviour via the hyperdirect and indirect pathway [Citation36,Citation37] and may hence explain PD symptoms of bradykinesia and rigidity [Citation35,Citation38]. Animal models and electrophysiological recordings in PD patients have linked dopamine depletion to excessive synchronisation in the beta band within the basal ganglia and thalamocortical circuits [Citation38,Citation39]. In nonhuman primates [Citation40] and mice [Citation41], dopamine depletion and Parkinson like symptoms can be induced via MPTP (1-methyl-4-phenyl-1,2,3,6-tetrahydropyridine) injections. Excessive beta synchrony after MPTP injection has been repeatedly reported for the motor cortex and parts of the basal ganglia including the STN and pallidum [Citation42,Citation43]. Non-invasive brain stimulation studies in healthy participants have provided further causal evidence for the relationship between increased beta oscillations and slowing of movements [Citation44–46]. Altogether, data from lesion and entrainment studies suggest that dopamine depletion results in increased beta synchronisation, which is associated with slowed movements as one key symptom observed in PD patients. Moreover, clinical studies have repeatedly reported that PD patients show higher amplitudes of beta frequencies at rest in particular within the STN, compared to healthy controls [Citation47–50]. Higher amplitudes in beta frequency, as well as the spatial extent of exaggerated beta synchronisation, have been related to the severity of symptoms, in particular with bradykinesia and rigidity [Citation51–54].
While these studies remain observational and thus largely correlational, intervention studies in PD patients have provided more direct evidence for the involvement of exaggerated beta oscillations in the manifestation of motor symptoms in PD patients. DBS treatment of the STN [Citation51,Citation55,Citation56] and pallidum [Citation57], as well as L-Dopa therapy, result in a suppression of beta activity (13–30Hz) that is proportional to observed clinical improvements [Citation39]. Further, beta coherence between the STN and (pre) motor cortices (i.e., the hyperdirect pathway) likely play a central role in both the pathophysiology of PD and treatment effects of DBS [Citation58–60]. Specifically, motor improvements for rigidity and bradykinesia correlate with observed decreases in beta amplitudes across DBS treatment techniques [Citation50,Citation51,Citation55–57]. Moreover, treatment response to DBS of the STN has been predicted by the spatial extent of beta oscillatory activity within the STN [Citation61]. Likewise, treatment with L-Dopa reduces beta oscillations [Citation48], and the extent of clinical improvements has been found correlated with the change in beta oscillatory activity [Citation62]. Besides changes in amplitude, correlations between activity of different brain areas within specific frequency bands (i.e., coherence) have been documented. For instance, two studies reported that L-dopa treatment reduced coherence in beta frequencies between the GPi and STN, and between the STN and SMA during rest and during motor tasks [Citation48,Citation63]. In light of these findings it has been speculated whether beta oscillations maintain a status quo of the motor system by inhibiting movement [Citation38,Citation64]. Attenuating beta oscillation amplitude in beta local field potentials (LFPs) may thus represent a potential target for non-invasive electroencephalography (EEG) neurofeedback training in PD patients. EEG uses scalp electrodes to measure LFPs, which represent the summed activity of local neural populations [Citation65]. Possibilities for tuning pathological brain oscillations have been discussed in depth, in particular dynamical systems theory provides an attractive framework to design EEG-NFT interventions [Citation66]. Further, EEG is a cost-efficient and widely available technique routinely used in neurological practice, rendering it particularly suitable for NFT delivered by health practitioner, but potentially also for use at home training [Citation67].
Invasive electrophysiological recordings have shown that kinesthetic motor imagery can reduce beta oscillations in the STN [Citation68]. These early findings were replicated and extended in a motor imagery paradigm that studied changes of STN activity in response to different levels of imagined movements, reporting reductions in beta LFP amplitude in PD patients who imagined different intensity levels of grip force [Citation69]. Although the study did also not include neurofeedback training, reported findings suggest that motor imagery alone resulted in parametric suppression of oscillations in the STN within the beta frequency band, providing a promising neural target for future invasive NFT protocols. Such modulation was indeed first reported in a two-group design study with eight DBS-treated PD patients, who either trained beta LFP down- or upregulation [Citation70]. Patients were able to voluntarily control resting beta-band activity in the STN in the intended direction without explicit cognitive strategies. In particular the group that trained beta downregulation showed significant decrease in beta band oscillations. However, the study only included a very short training interval (10 minutes) and did not assess behavioural or clinical outcomes. A more recent single-blind, within-subject controlled study replicated and extended these findings [Citation71]. The authors demonstrated bidirectional control of continuous STN activity over a one-hour training period of motor-imagery based NFT. Further, they observed that patients’ self-regulation performance increased over time, suggesting that NFT helped to learn the voluntary suppression of beta. Self-regulation was further maintained when visual feedback was subsequently withdrawn, suggesting a transfer of learned strategies. Importantly, motor improvements with regards to bradykinesia were observed two days following the training. This work has laid the ground for a recently registered larger (open label) clinical trial (https://clinicaltrials.gov/ct2/show/NCT05101161). The first demonstration of accelerated movements in a cued reaction time task were shown by another group that trained PD patients in suppressing STN beta LFPs [Citation72]. This study, however, was limited by a within-subject design where the control condition was “no training” and patients were merely asked to pay attention to the visual feedback without engaging in motor imagery. Besides decreases in beta band synchrony, the authors also found increases in gamma activity within the STN, and decreased beta band coupling between the STN and motor cortices (i.e., the hyperdirect pathway). Of note, this study was also the first to report side effects following NFT. Patients who had pre-existing tremor showed increased Parkinsonian tremor (3–7Hz) amplitudes, which were correlated with increased theta band (3–7Hz) activity in the STN. The authors discussed that reduction in beta oscillations may increase tremor band activities in the STN and that PD patients suffering from tremor may rather benefit from NFT protocols that target tremor-related frequency bands, e.g., aim to decrease theta synchrony. This conclusion is in line with a narrative review on NFT in PD [Citation73]. Of interest, theta oscillations have also been linked to gait disturbances in PD patients. Increased frontal theta oscillations seem related to the transition to freeze of gait, possibly reflecting a cognitive compensatory mechanism [Citation74–76]. Decreased occipital theta oscillations, on the other hand, have been linked to gait disbalance and recently successfully targeted using NFT [Citation77]. Hence, in the context of PD, thinking of theta oscillations as exclusively movement-promoting or -inhibiting is likely misleading. Further studies will be needed to understand its role in the underlying pathophysiology and make informed suggestions for symptom specific targeting via NFT.
A second potential candidate for neurofeedback are high gamma band oscillations. Gamma oscillations (60–90Hz) have been linked to promoting movement, but also to PD motor symptoms. For instance, non-invasive brain stimulation studies reported increased movement speeds after the entrainment of motor cortices in the gamma frequency band for healthy participants [Citation45,Citation46]. In PD patients, dopaminergic treatment has been found to increase the amplitude of gamma oscillations measured over central electrodes proportional to the degree that movements were facilitated [Citation78]. Other recent work suggests that the interplay between beta and gamma oscillations may represent a key coupling mechanism that sub-serves maintaining motor programmes and mediates treatment effects. For instance, exaggerated coupling between low frequency beta band (13–22 Hz) phase-and-amplitude of high frequency gamma (300 Hz) band amplitude within the STN correlated with bradykinesia and rigidity severity [Citation79]. Moreover, STN DBS treatment reduces phase-and-amplitude remarkably, and this measure has been shown to correlate with rigidity scores before and after DBS [Citation80]. In order to use gamma oscillations as a target signature for EEG-NFT training, participants would need to be able to modulate its amplitude (e.g., via motor imagery). Indeed, similar to beta band frequencies, gamma band frequencies (55–85Hz) can also be modulated using motor imagery as revealed by invasive recordings from STN of DBS treated PD patients [Citation69]. Specifically, these data showed increased amplitudes (in contrast to decreased amplitude in the beta band) that patients could modulate via motor imagery tasks of different intensity. Overall, the presented literature suggests that gamma rhythms likely promote movement in PD. However, beyond physiological movements, exaggerated gamma oscillations have been linked to pathological movements such as dyskinesia, which represents one common motor complication following long-term treatment with L-Dopa. Although the neural mechanisms of dyskinesia are still poorly understood, gamma oscillations likely contribute (see for review [Citation73]). For instance, gamma oscillations (60–90 Hz) in the motor cortex have been linked to dyskinetic symptoms, as reported for the 6-OHDA PD model in rodents [Citation81], and more recently also in PD patients [Citation82]. Therefore, although EEG-NFT training of gamma frequencies may promote movement, it may also promote dyskinetic brain states.
Another target for EEG-NFT motor rehabilitation in PD may be reducing power in the alpha frequency band (8–12 Hz). Event Related De-synchronisation (ERD) in the alpha bands over central (C3, C4, Cz, Fz) electrodes precedes voluntary self-paced movements for a short period (∼2 seconds) [Citation83]. The onset of ERD during voluntary movement is delayed in PD patients compared to healthy controls [Citation84], which may result from a later initiation of motor programming due to sustained movement inhibition in consequence to elevated beta rhythms. L-Dopa replacement therapy reduces delayed ERD in PD patients, as reported for different motor tasks including self-paced button press [Citation85] and wrist flexion movements [Citation86]. Further, studies have reported correlations between ERD delay and PD severity [Citation86], as well as between differences in ERD delays “ON” vs. “OFF” medication and changes in movement speed “ON” vs. “OFF”, including self-paced elbow flexions and hand squeezes [Citation87]. Further evidence for the relationship between successful treatment for PD symptoms and increases in alpha ERD latencies stems from DBS studies. For instance, STN DBS in combination with L-Dopa was associated with an increase in alpha ERD latency during movement preparation over the contralateral central region, as well as an increase in alpha ERD during movement execution over central electrodes [Citation88]. The same study also reported that more advanced PD patients showed a larger decrease in alpha ERD latency. Of interest, the same study could not find such relationships for pallidal DBS. This dissociation may indicate that ERD latency modulation during movement preparation (and motor imagery) is mainly influenced by the hyperdirect pathway [Citation16]. A more recent STN DBS study extended the focus on lower frequencies, investigating amplitude changes within the alpha band (mu rhythm) and lower beta band frequencies over sensorimotor cortices. The authors reported similar reductions in amplitude for both frequency bands after unilateral STN DBS [Citation89]. Noteworthy, high synchronisations between motor cortices in the lower and higher alpha band have previously been reported for early-stage (i.e., mostly Hoehn-Yahr stages I and II) PD patients compared to controls [Citation90]. Further, decreases in alpha amplitude during STN DBS were correlated with improvements of patient’s UPDRS rigidity scores [Citation91]. Taken together, these observational and interventional studies suggest that the alpha ERD degenerates with disease progression, and it may indicate that PD treatment enhances movement speed by normalising pre-movement alpha ERD. Further, these findings are in line with the notion that the basal ganglia promote alpha ERD by releasing the motor regions from an idling state, thereby permitting effective motor response selection and programming [Citation92]. With regards to motor imagery-based EEG-NFT training in PD patients, alpha rhythms may thus provide a promising target to facilitate voluntary movement initiation. Importantly, previous EEG studies suggested alpha rhythms decrease during motor imagery in healthy participants [Citation93,Citation94]. Further, BCI and EEG-NFT studies in healthy participants have shown that reduction of premovement alpha desynchronisation (11–13 Hz) is trainable [Citation95,Citation96]. Based on this study, a proof-of-concept study protocol with PD patients has been developed and registered (https://doi.org/10.1186/ISRCTN16783092) and interim results reported [Citation97].
5. Hemodynamic NFT targets
One main limitation of EEG is its relatively low spatial resolution, limiting recordings only to the neocortex. The non-invasive brain imaging technique functional magnetic resonance imaging (fMRI), on the other hand, allows recording activity in deep brain structures, including the basal ganglia. The image contrast of fMRI is based on the blood oxygenation level dependent signal (BOLD), which is inversely proportional to the local level of deoxyhaemoglobin [Citation98]. BOLD represents an indirect measure of neural activity, its neural origin lies in LFPs [Citation99]. Of interest, the frequency bands discussed above (alpha, beta and gamma) have been identified as being most informative with regards to variations in the BOLD signal [Citation100]. fMRI offers additional insights into neural correlates of established treatments, including network effects during DBS stimulation. Published task-based fMRI and resting-state fMRI studies conducted in PD patients treated with STN DBS were recently reviewed [Citation101]. Local patterns of modulations in the BOLD signal during rest and motor tasks were mostly observed in areas involved that form part of the direct and indirect pathway, including basal ganglia structures such as the GP and the putamen, thalamus, motor cortex, as well as the cerebellum. Of note, distant effects in the primary motor cortex (M1) showed a mixed pattern of either, activation, deactivation or no effect. The authors noted that this variability may be explained by different phenotypes of PD (such that akinetic-rigid type PD patients showed an increase in resting-state M1 activity, while patients with tremor-dominant PD showed a decrease) [Citation101]. Caution is required, however, when drawing reverse inferences [Citation102] from fMRI data about potential pathological vs. compensatory mechanisms [Citation101]. To investigate large-scale network effects of DBS and relate these to classical circuit models of PD (), computational approaches such as dynamic causal modelling (DCM) [Citation103] can provide further insights about network interactions between subcortical and cortical brain regions. For instance, one fMRI study included in this review [Citation101] reported that sensitizing the thalamus to direct pathway afferents, while simultaneously desensitizing the STN to its afferents from the hyperdirect and indirect pathway was related to better clinical outcome of STN DBS [Citation104].
Feasibility for fMRI-NFT in PD has been shown with initial promising clinical outcomes; PD was indeed the first clinical application of this relatively new technique [Citation105]. To date, only a few fMRI-NFT studies have been conducted in mostly early-stage (Hoehn-Yahr I and II) PD patients. Thus far published studies have mostly trained patients to increase the BOLD signal in the SMA [Citation105–107] using motor imagery. The SMA is a premotor region that forms a key structure in the hyperdirect pathway [Citation16] between motor cortex and STN. Given their initial promising clinical findings in an earlier study [Citation105], the authors conducted a single-blind, randomised controlled trial with a similar paradigm, reporting clinically meaningful improvement of about 4.5 points on a global score (UDPRS-III) in their experimental group (N = 13) [Citation106]. However, the study did not find superiority compared to their active control group (N = 13) that performed high-technology supervised exercise training that involved feedback-based motor exercises via an immersive game console. As the authors also note, however, this group comparison was likely conservative as patients in the control group, too, took part in a high-intensity intervention protocol with feedback learning [Citation108,Citation109]; the study would thus have required a substantially larger sample (and/or more training sessions) to detect relevant differences in the clinical outcome. With regards to other potential target areas, the spatial resolution of fMRI allows training activity of both cortical and subcortical areas in the context of motor imagery and motor execution based NFT. For motor imagery based fMRI-NFT, premotor cortices such as the ventral premotor cortex but also subcortical areas such as basal ganglia structure show consistent (kinesthetic) motor imagery task activation and may serve as possible targets [Citation110]. However, in particular basal ganglia structures also play a role in the learning to self-regulate brain activity for a range of NFT paradigms [Citation111,Citation112], rendering the design of appropriate control conditions for such fMRI more challenging [Citation108,Citation113]. With regards to the primary cortex (M1), activation during motor imagery has not been consistently found with and without NFT [Citation110,Citation114–116]. It may, however, provide a suitable target region for motor execution based NFT. With regards to other motor symptoms in PD, difficulties in swallowing are highly prevalent with up to 90% in late disease stages. Proof of concept for fMRI-NFT of the left precentral gyrus to guide swallowing has been reported for healthy individuals [Citation117]. Similar paradigms could be adapted to reinforce compensatory activity identified in non-symptomatic PD patients [Citation4] or improve swallowing in symptomatic PD patients.
Moving beyond mean-field activity models of specific brain regions, connectivity-based models and multivariate statistical techniques such as multivariate pattern analysis (MVPA) and representational similarity analysis (RSA) may provide promising alternative approaches. Network dynamics or voxel patterns associated with therapeutic effects of established treatments could be reinforced, emulated, or possibly test for their causal influence [Citation118]. For instance, DCM based NFT [Citation119] could be applied to reinforce positive and negative modulatory effects identified to emulate therapeutic DBS effects [Citation104]. Further, real-time implementations of advanced statistical approaches such as MVPA and RSA allow exploiting condition specific information encoded in high-dimensional fMRI data. For instance, they provide higher sensitivity for movement-specific differences in neural activity patterns of both executed and imagined hand movements, including M1 [Citation120–122]. When combined with multimodal approaches, respective spatial and temporal neural profiles can be comprehensively characterised and compared across modalities [Citation123]. Further, when applied to fMRI data recorded during highly effective established treatment such as DBS, MVPA can identify network patterns related to treatment success [Citation124]. These examples illustrate how multivariate approaches can inform future NFT targets selections fMRI-NFT protocols. Altogether, multivariate real-time fMRI-NFT protocols allow targeting relevant neural signatures more precisely compared to standard mean field approaches [Citation125,Citation126], yielding new opportunities for self-regulation based NFT in PD and motor rehabilitation more generally.
To improve the transfer towards more mobile interventions, multi-channel functional near-infrared-spectroscopy (fNIRS) may be used. fNIRS is a non-invasive optical neuroimaging technique that measures concentration changes of oxygenated hemoglobin and deoxygenated hemoglobin in cerebral vessels. fNIRS is conceptually comparable to fMRI because it captures hemodynamic responses of brain activity [Citation127,Citation128]. fMRI BOLD and optical fNIRS signals have shown high correspondence for a variety of tasks [Citation129]. Compared to fMRI, fNIRS has no environmental or population-specific restrictions and is thus suitable for fully mobile use. Further, fNIRS devices are substantially less costly and thus more scalable, allowing for adequately powered clinical trials. The spatial resolution of fNIRS, however, is constrained to measuring cortical activity. fNIRS brain mapping studies have provided further insights into cortical responses following established treatment in PD patients [Citation130,Citation131]. Further, a recent systematic review provides an overview of fNIRS-NFT studies conducted in healthy participants and patients [Citation132], which are mainly confined to proof-of-concept studies. Of interest for motor rehabilitation, it also reviewed one randomised clinical trial (RCT) that reported improved hand/finger motor symptoms in stroke patients when comparing real fNIRS-NFT vs. sham NFT [Citation133]. A more recent larger, two-center RCT of motor imagery based SMA fNIRS NFT by the same group resulted in greater improvements for post stroke gait and balance disturbances when comparing real vs. sham [Citation134]. Further, recent validation work showed that fNIRS can measure motor imagery and motor execution related SMA activity with high spatial specificity and task sensitivity when compared to gold standard fMRI recordings [Citation135]. Hence, fNIRS-NFT based on (pre) motor cortices such as the SMA may be a promising approach for mobile, scalable fNIRS-NFT. Further, building up on previous reports of parametric modulations in relevant neural circuits during motor imagery [Citation69,Citation70], more recent developments for hemodynamic NFT protocols allow to provide more precise and resource-efficient measures of self-regulation success [Citation115,Citation136]. Currently, one ongoing fNIRS-NFT trial is registered with PD patients that targets the SMA (https://www.drks.de/drks_web/navigate.do?navigationId=trial.HTML&TRIAL_ID=DRKS00022997). Moreover, future applications may benefit from wearable EEG-fNIRS technologies that can integrate information from both modalities [Citation137].
6. Targeting non-motor symptoms
To-date, NFT studies conducted in PD patients have primarily focused on improving motor symptoms. Non-motor symptoms, however, including depression, attention and cognitive deficits become dominant symptoms in late disease stages. A recent review of NFT to treat cognitive impairment reported evidence for the feasibility of EEG- and fMRI-NFT paradigms that aim to treat dementia and cognitive impairment, reporting first promising clinical findings [Citation138]. Cognitive symptoms have also been successfully targeted in stroke patients [Citation139]. With regards to attention and mood, currently available literature largely suggests that a substantial proportion of reported symptom improvements may be less specific about the neural circuitry that is being targeted [Citation140,Citation141]. “Active ingredients” of non-specific effects that can be enhanced with NFT include sense of control and self-efficacy, which are central to motor related self-management in PD patients [Citation142,Citation143]. One could therefore expect that NFT protocols that target motor cortices or basal ganglia structures and allow patients to self-regulate their brain activity may also likely result in clinically meaningful improvements with regards to mood and attention. Hence, at least including respective measures as secondary outcomes may hence be worthwhile considering for future PD NFT studies. This seems particular relevant given that improvements in motor symptoms have also been linked to mood improvements in place control groups in the context of antidepressive medication [Citation144].
7. Turning neural targets into informative intervention studies
Published literature of PD brain mapping studies provide a solid mechanistic basis to understand pathology and treatment effect. It can thus substantially inform hypotheses and study designs for targeting specific symptoms with neuromodulatory interventions such as NFT. To put suggested neural targets at a fair test and find out about their therapeutic potential, the PD NFT field needs to improve on design and reporting practices as also concluded by a recent systematic review on NFT in PD [Citation14]. Specifically, they concluded that NFT studies should report more clinical information (including potential side effects) and use appropriate numerical reporting (e.g., inferential statistics and effect sizes). To move the field beyond mere feasibility studies, it will require adequate control conditions, blinding suited to the respective study design (e.g., double blind for NFT vs. sham NFT [Citation134], single-blind for NFT vs. active control comparison Citation108] and patient randomisation. Of note, placebo effects, which implicate dopamine release [Citation145], are largely preserved in PD patients despite the loss of dopaminergic function [Citation146]. For instance, substantial placebo effects have been observed for other neuromodulatory interventions such as brain stimulation during both “ON” and “OFF” medication [Citation59]. Hence, NFT interventions in PD will require controlling for non-specific NFT effects. Their design should take into account that NFT is a complex, reward learning based task. Associated motivational processes [Citation113] involve several brain regions, e.g., basal ganglia structures such as the putamen [Citation111,Citation112], that are damaged by the PD pathology. Counteracting such pathology may provide one rationale to target these areas in the first place. However, researchers should consider controlling for involved motivational processes to discern specific effects related to their target process [Citation108,Citation113]. Further, depending on the disease stage, pathology may also affect motivational and learning to a degree that PD patients show impaired NFT acquisition, in particular when being “OFF” medication. Including a healthy, age-matched control group should thus be considered. RCTs will further require sufficient statistical power to increase the chances for informative trial outcomes. Powering studies for smallest effect sizes of interest would be desired [Citation147] and can be informed by minimal clinically important differences [Citation148,Citation149]. However, sample size calculation to detect small effect sizes may exceed resource constraints. Means to improve trials’ efficacy can involve increasing the number of training sessions, conducting multi-centre study and/or employing sequential sampling plans [Citation150]. To increase replicability and reproducibility, study preregistration and data sharing are strongly encouraged. Comprehensive study preregistration includes specific hypotheses mapped to respective sampling and analysis plans. Of note, the publishing format Registered Reports (RRs) provides pre-acceptance for publication following peer-review of the study protocol. First data indicates that they mitigate excess significance when compared to traditional literature in the life sciences [Citation151,Citation152]. The recently published CRED-nf checklist provides guidance and suggestions regarding good design and reporting practices [Citation153].
8. Conclusion
Neurofeedback training has been suggested as a potential add-on therapy in the treatment of motor symptoms in PD. The development of successful interventions can benefit from neural markers reported for established therapies such as pharmacological treatment and DBS. This scoping review highlights three electrophysiological markers that may provide suitable neural targets for non-invasive (i.e., EEG) and invasive (i.e., DBS electrodes), symptom-specific NFT in PD patients: 1) reinforcing beta-band ERD (13–30Hz) to reduce exaggerated beta synchronisation, 2) increasing the amplitude of pro-kinetic gamma-band (30–80Hz) frequencies, and 3) reinforcing pre-movement alpha ERD (8–12Hz) to facilitate voluntary movement initiation. Their individual therapeutic potential may mainly improve symptoms of bradykinesia and rigor, but also induce possible side effects (e.g., tremor and dyskinesia) that require careful monitoring and reporting. Research on PD phenotypes should be considered in inclusion criteria. For the first generation of NFT trials it is likely advisable to test for the clinical potential of these three potential NFT targets separately. Once shown to be effective in reducing motor symptoms, this will allow to formulate clear indications and contraindications for NFT. Next, NFT protocols could also combine training targets to provide individually tailored network based NFT. Moreover, future research on the relation between cognitive and motor symptoms may provide additional targets for NFT. Hemodynamic fMRI offers higher spatial resolution and thus complementary mechanistic insights. Regarding promising target areas for fMRI NFT, premotor cortices including the SMA have shown promise and can be extended to training of subcortical structures involved in motor imagery or motor execution. fMRI NFT paradigms can further benefit from exploiting high dimensional neural information using network based approaches and multivariate statistical methods. Hemodynamic fNIRS NFT may provide a promising approach to translate protocols for mobile and cost-efficient use. The NFT field can only advance with adequately designed reported clinical NFT trials that include adequate controls and statistical power [Citation153]. Involved resource demands will likely put mobile technologies such as EEG and fNIRS in an advantageous position. Altogether, despite remaining unknowns of involved mechanisms [Citation34], PD is a disorder that provides Bacomicists with informative neural priors to design NFT interventions based on validated neural circuit models. Although NFT will likely not replace established therapies, it may serve as an add-on treatment to help reduce dopaminergic dosages or stimulation intensities in patients using DBS.
Acknowledgements
Part of this manuscript is based on a Ph.D. thesis chapter [Citation154]. The author would like to thank Dr. David Linden, Mr. Po Liu and Ms. Franziska Klein for helpful comments on an earlier version of this manuscript.
Disclosure statement
The author has in the past received payments for consulting for a neurofeedback start-up company (Mendi Innovations AB) and is co-founder of the science consultancy kop science + consulting GbR (exited in 2021).
Additional information
Funding
References
- Lau L. D, Breteler MMB. The epidemiology of Parkinson’s disease. Lancet Neurol. 2006;5(6):525–535.
- Cersosimo MG, Raina GB, Pecci C, et al. Gastrointestinal manifestations in Parkinson's disease: prevalence and occurrence before motor symptoms. J Neurol. 2013;260(5):1332–1338.
- Suttrup I, Warnecke T. Dysphagia in Parkinson's disease. Dysphagia. 2016;31(1):24–32.
- Suntrup S, Teismann I, Bejer J, et al. Evidence for adaptive cortical changes in swallowing in Parkinson's disease. Brain. 2013;136(Pt 3):726–738.
- Chaudhuri RK, Healy DG, Schapira AV. Non-motor symptoms of Parkinson’ s disease: diagnosis and management related papers management. Lancet Neurol. 2006;5(3):235–245.
- Barber TR, Lawton M, Rolinski M, et al. Prodromal parkinsonism and neurodegenerative risk stratification in rem sleep behavior disorder. Sleep. 2017;40(8):11–13.
- Gratwicke J, Jahanshahi M, Foltynie T. Parkinson's disease dementia: a neural networks perspective. Brain. 2015;138(Pt 6):1454–1476.
- Biundo R, Weis L, Antonini A. Cognitive decline in Parkinson's disease: the complex picture. NPJ Parkinsons Dis. 2016;2(1):16018.
- Ba F, Obaid M, Wieler M, et al. Parkinson disease: the relationship between non-motor symptoms and motor phenotype. Can J Neurol Sci. 2016;43(2):261–267.
- Muslimović D, Post B, Speelman JD, et al. Determinants of disability and quality of life in mild to moderate Parkinson disease. Neurology. 2008;70(23):2241–2247.
- Tomlinson CL, Patel S, Meek C, et al. Physiotherapy intervention in Parkinson’s disease: systematic review and meta-analysis. BMJ. 2012;345(1):e5004–14.
- Connolly BS, Lang AE. Pharmacological treatment of Parkinson disease: a review. JAMA - J Am Med Assoc. 2014;311(16):1670–1683.
- Chiken S, Nambu A. Mechanism of deep brain stimulation: inhibition, excitation, or disruption? Neuroscientist. 2016;22(3):313–322.
- Anil K, Hall SD, Demain S, et al. A systematic review of neurofeedback for the management of motor symptoms in Parkinson’s disease. Brain Sci. 2021;11(10):1292.
- Losseff N, Brown M, Grieve J. Stroke and cerebrovascular disease. In: Clarke C, Howard R, Rossor M, Shorvon S, eds. Neurology: a queen square textbook. London: Wiley-Blackwell. 2009. p. 109–154.
- Nambu A, Tokuno H, Takada M. Functional significance of the cortico- subthalamo- pallidal ‘hyperdirect’ pathway. Neuro Res. 2002;43(2):111–117.
- Nambu A. Seven problems on the basal ganglia. Curr Opin Neurobiol. 2008;18(6):595–604.
- Chu HY, McIver EL, Kovaleski RF, et al. Loss of hyperdirect pathway cortico-subthalamic inputs following degeneration of midbrain dopamine neurons. Neuron. 2017;95(6):1306–1318.e5.
- Nambu A, Tachibana Y, Chiken S. Cause of parkinsonian symptoms: firing rate, firing pattern or dynamic activity changes? Basal Ganglia. 2015;5(1):1–6.
- Rodriguez-Oroz MC, Jahanshahi M, Krack P, et al. Initial clinical manifestations of Parkinson’s disease: features and pathophysiological mechanisms. Lancet Neurol. 2009;8(12):1128–1139.
- Neumann W, Schroll H, Luisa A, et al. Functional segregation of basal ganglia pathways in Parkinson’s disease. 2018;141(9):2655–2669.
- Aron AR, Herz DM, Brown XP, et al. Frontosubthalamic circuits for control of action and cognition. J Neurosci. 2016;36(45):11489–11495.
- Herz DM, Little S, Pedrosa DJ, et al. Mechanisms underlying decision-making as revealed by deep-brain stimulation in patients with Parkinson's disease. Curr Biol. 2018;28(8):1169–1178.e6.
- Mak MK, Wong-Yu IS, Shen X, et al. Long-term effects of exercise and physical therapy in people with Parkinson disease. Nat Rev Neurol. 2017;13(11):689–703.
- Radder DLM, Lígia Silva de Lima A, Domingos J, et al. Physiotherapy in Parkinson's disease: a meta-analysis of present treatment modalities. Neurorehabil Neural Repair. 2020;34(10):871–880.
- Ahlskog J, Muenter M. Frequency of levodopa-related dyskinesias and motor fluctuations as estimated from the cumulative literature. Mov Disord. 2001;16(3):448–458.
- Pérez-Pérez J, Pagonabarraga J, Martínez-Horta S, et al. Head-to-head comparison of the neuropsychiatric effect of dopamine agonists in Parkinson's disease: a prospective, cross-sectional study in non-demented patients. Drugs Aging. 2015;32(5):401–407.
- Antonini A, Tolosa E, Mizuno Y, et al. A reassessment of risks and benefits of dopamine agonists in Parkinson’s disease. Lancet Neurol. 2009;8(10):929–937.
- Huot P, Johnston TH, Koprich JB, et al. The pharmacology of L-DOPA-induced dyskinesia in Parkinson's disease. Pharmacol Rev. 2013;65(1):171–222.
- Benabid AL, Chabardes S, Mitrofanis J, et al. Deep brain stimulation of the subthalamic nucleus for the treatment of Parkinson’s disease. Lancet Neurol. 2009;8(1):67–81.
- Wang JW, Zhang YQ, Zhang XH, et al. Deep brain stimulation of pedunculopontine nucleus for postural instability and gait disorder after Parkinson disease: a meta-analysis of individual patient data. World Neurosurg. 2017;102:72–78.
- Accolla EA, Pollo C. Mood effects after deep brain stimulation for Parkinson’s disease: an update. Front Neurol. 2019;10:617–613.
- Irmen F, Horn A, Mosley P, et al. Left prefrontal connectivity links subthalamic stimulation with depressive symptoms. Ann Neurol. 2020;87(6):962–975.
- McGregor MM, Nelson AB. Circuit mechanisms of Parkinson's Disease. Neuron. 2019;101(6):1042–1056.
- Jenkinson N, Brown P. New insights into the relationship between dopamine, beta oscillations and motor function. Trends Neurosci. 2011;34(12):611–618.
- Chambers CD, Garavan H, Bellgrove MA. Insights into the neural basis of response inhibition from cognitive and clinical neuroscience. Neurosci Biobehav Rev. 2009;33(5):631–646.
- Jahfari S, Waldorp L, van den Wildenberg WPM, et al. Effective connectivity reveals important roles for both the hyperdirect (Fronto-Subthalamic) and the indirect (Fronto-Striatal-Pallidal) Fronto-Basal ganglia pathways during response inhibition. J Neurosci. 2011;31(18):6891–6899.
- Engel AK, Fries P. Beta-band oscillations-signalling the status quo? Curr Opin Neurobiol. 2010;20(2):156–165.
- Little S, Brown P. The functional role of beta oscillations in Parkinson’s disease. Park Relat Disord. 2014;20(SUPPL.1):S44–S48.
- Wichmann T, DeLong M. Pathophysiology of Parkinson's disease: the MPTP primate model of the human disorder. Ann NY Acad Sci. 2003;(991):199–213.
- Jackson-Lewis V, Przedborski S. Protocol for the MPTP mouse model of Parkinson's disease. Nat Protoc. 2007;2(1):141–151.
- Bergman H, Wichmann T, Karmon B, et al. The primate subthalamic nucleus. II. Neuronal activity in the MPTP model of parkinsonism. J Neurophysiol. 1994;72(2):507–520.
- Moran A, Stein E, Tischler H, et al. Bar-Gad I. Decoupling neuronal oscillations during subthalamic nucleus stimulation in the parkinsonian primate. Neurobiol Dis. 2012;45(1):583–590.
- Pogosyan A, Gaynor LD, Eusebio A, et al. Boosting cortical activity at beta-band frequencies slows movement in humans. Curr Biol. 2009;19(19):1637–1641.
- Joundi RA, Jenkinson N, Brittain J-S, et al. Driving oscillatory activity in the human cortex enhances motor performance. Curr Biol. 2012;22(5):403–407.
- Moisa M, Polania R, Grueschow M, et al. Brain network mechanisms underlying motor enhancement by transcranial entrainment of gamma oscillations. J Neurosci. 2016;36(47):12053–12065.
- Pollok B, Krause V, Martsch W, et al. Motor-cortical oscillations in early stages of Parkinson's disease. J Physiol. 2012;590(13):3203–3212.
- Brown P, Oliviero A, Mazzone P, et al. Dopamine dependency of oscillations between subthalamic nucleus and pallidum in Parkinson’s disease. J Neurosci. 2001;21(3):1033–1038.
- Marsden JF, Limousin-Dowsey P, Ashby P, et al. Subthalamic nucleus, sensorimotor cortex and muscle interrelationships in Parkinson’s disease. Brain. 2001;124(2):378–388.
- Kühn AA, Tsui A, Aziz T, et al. Pathological synchronisation in the subthalamic nucleus of patients with Parkinson's disease relates to both bradykinesia and rigidity. Exp Neurol. 2009;215(2):380–387.
- Hammond C, Bergman H, Brown P. Pathological synchronization in Parkinson's disease: networks, models and treatments. Trends Neurosci. 2007;30(7):357–364.
- Gatev P, Darbin O, Wichmann T. Oscillations in the basal ganglia under normal conditions and in movement disorders. Mov Disord. 2006;21(10):1566–1577.
- Zaidel A, Arkadir D, Israel Z, et al. Akineto-rigid vs. tremor syndromes in parkinsonism. Curr Opin Neurol. 2009;22(4):387–393.
- Pogosyan A, Yoshida F, Chen CC, et al. Parkinsonian impairment correlates with spatially extensive subthalamic oscillatory synchronization. Neuroscience. 2010;171(1):245–257.
- van Wijk BCM. Is broadband gamma activity pathologically synchronized to the beta rhythm in Parkinson's Disease? J Neurosci. 2017;37(39):9347–9349.
- Santaniello S, McCarthy MM, Montgomery EB, Gale JT, et al. Therapeutic mechanisms of high-frequency stimulation in Parkinson's disease and neural restoration via loop-based reinforcement. Proc Natl Acad Sci USA. 2015;112(6):E586–E595.
- Wang DD, de Hemptinne C, Miocinovic S, et al. Pallidal deep brain stimulation disrupts pallidal beta oscillations and coherence with primary motor cortex in Parkinson’s disease. J Neurosci. 2018;10:431–418.
- Akram H, Georgiev D, Mahlknecht P, et al. Subthalamic deep brain stimulation sweet spots and hyperdirect cortical connectivity in Parkinson’s disease. Neuroimage. 2017;158:332–345.
- Chou Y, Hickey PT, Sundman M, et al. Effects of repetitive transcranial magnetic stimulation on motor symptoms in Parkinson disease. JAMA Neurol. 2015;72(4):432.
- Oswal A, Cao C, Yeh CH, et al. Neural signatures of hyperdirect pathway activity in Parkinson’s disease. Nat Commun. 2021;12(1).
- Zaidel A, Spivak A, Grieb B, et al. Subthalamic span of β oscillations predicts deep brain stimulation efficacy for patients with Parkinson’s disease. Brain. 2010;133(7):2007–2021.
- Weinberger M, Mahant N, Hutchison WD, et al. Beta oscillatory activity in the subthalamic nucleus and its relation to dopaminergic response in Parkinson's disease. J Neurophysiol. 2006;96(6):3248–3256.
- Cassidy M, Mazzone P, Oliviero A, et al. Movement‐related changes in synchronization in the human basal ganglia. Brain. 2002;125(6):1235–1246.
- Engel AK, Moll CKE, Fried I, et al. Invasive recordings from the human brain: clinical insights and beyond. Nat Rev Neurosci. 2005;6(1):35–47.
- Lopes da Silva F. EEG: Origin and measurement. In: Mulert C, Lemieux L, eds. Eeg-fmri. 1st ed. Berlin, Heidelberg: Springer; 2010. 19–38.
- Ros TJ, Baars B, Lanius RA, et al. Tuning pathological brain oscillations with neurofeedback: a systems neuroscience framework. Front Hum Neurosci. 2014;8:1–22.
- Kranczioch C, Zich C, Schierholz I, et al. Mobile EEG and its potential to promote the theory and application of imagery-based motor rehabilitation. Int J Psychophysiol. 2014;91(1):10–15.
- Kühn AA, Doyle L, Pogosyan A, et al. Modulation of beta oscillations in the subthalamic area during motor imagery in Parkinson's disease. Brain. 2006;129(Pt 3):695–706.
- Fischer P, Pogosyan A, Cheeran B, et al. Subthalamic nucleus beta and gamma activity is modulated depending on the level of imagined grip force. Exp Neurol. 2017;293:53–61.
- Fukuma R, Yanagisawa T, Tanaka M, et al. Real-time neurofeedback to modulate β-band power in the subthalamic nucleus in Parkinson’s disease patients. eNeuro. 2018;5(6):1–8.
- Bichsel O, Stieglitz LH, Oertel MF, et al. Deep brain electrical neurofeedback allows Parkinson patients to control pathological oscillations and quicken movements. Sci Rep. 2021;11(1):1–10.
- He S, Mostofi A, Syed E, et al. Subthalamic beta-targeted neurofeedback speeds up movement initiation but increases tremor in parkinsonian patients. Elife. 2020;9:1–20.
- Esmail S, Linden D. Neural networks and neurofeedback in Parkinson’s disease. NR. 2014;1(3-4):240–272.
- Singh A, Cole RC, Espinoza AI, et al. Frontal theta and beta oscillations during lower-limb movement in Parkinson's disease. Clin Neurophysiol. 2020;131(3):694–702.
- Ardi Handojoseno AM, Shine JM, Nguyen TN, et al. Analysis and prediction of the freezing of gait using EEG brain dynamics. IEEE Trans Neural Syst Rehabil Eng. 2015;23(5):887–896.
- Shine JM, Handojoseno AMA, Nguyen TN, et al. Abnormal patterns of theta frequency oscillations during the temporal evolution of freezing of gait in Parkinson's disease. Clin Neurophysiol. 2014;125(3):569–576.
- Azarpaikan A, Torbati HT, Sohrabi M. Neurofeedback and physical balance in Parkinson's patients. Gait Posture. 2014;40(1):177–181.
- Jenkinson N, Kühn AA, Brown P. γ Oscillations in the human basal ganglia. Exp Neurol. 2013;245:72–76.
- van Wijk BCM, Beudel M, Jha A, et al. Subthalamic nucleus phase-amplitude coupling correlates with motor impairment in Parkinson's disease. Clin Neurophysiol. 2016;127(4):2010–2019.
- De Hemptinne C, Swann NC, Ostrem JL, et al. Therapeutic deep brain stimulation reduces cortical phase-amplitude coupling in Parkinson's disease. Nat Neurosci. 2015;18(5):779–786.
- Halje P, Tamte M, Richter U, et al. Levodopa-Induced dyskinesia is strongly associated with resonant cortical oscillations. J Neurosci. 2012;32(47):16541–16551.
- Swann NC, de Hemptinne C, Miocinovic S, et al. Gamma oscillations in the hyperkinetic state detected with chronic human brain recordings in Parkinson's Disease. J Neurosci. 2016;36(24):6445–6458.
- Pfurtscheller G, Aranibar A. Evaluation of event-related desynchronization (ERD) preceding and following voluntary self-paced movement. Electroencephalogr Clin Neurophysiol. 1979;46(2):138–146.
- Defebvre L, Bourriez J, Dujardin K, et al. Spatiotemporal study of Bereitschaftspotential and event-related desynchronization during voluntary movement in Parkinson’s disease. Brain Topogr. 1994. [cited 2017 May 22]. Accessed from: http://www.springerlink.com/index/T307406857L0664U.pdf.
- Magnani G, Cursi M, Leocani L, et al. Acute effects of L-dopa on event-related desynchronization in Parkinson's disease. Neurol Sci. 2002;23(3):91–97.
- Devos D, Labyt E, Derambure P, et al. Effect of L-Dopa on the pattern of movement-related (de)synchronisation in advanced Parkinson's disease. Neurophysiol Clin. 2003;33(5):203–212.
- Wang HC, Lees A J, Brown P. Impairment of EEG desynchronisation before and during movement and its relation to bradykinesia in Parkinson’s disease. J Neurol Neurosurg Psychiatry. 1999;66(4):442–446.
- Devos D, Defebvre L. Effect of deep brain stimulation and l-Dopa on electrocortical rhythms related to movement in Parkinson's disease. Prog Brain Res. 2006;159:331–349.
- Abbasi O, Hirschmann J, Storzer L, et al. Unilateral deep brain stimulation suppresses alpha and beta oscillations in sensorimotor cortices. Neuroimage. 2018;174:201–207.
- Stoffers D, Bosboom JLW, Deijen JB, et al. Increased cortico-cortical functional connectivity in early-stage Parkinson's disease: an MEG study. Neuroimage. 2008;41(2):212–222.
- Airaksinen K, Butorina A, Pekkonen E, et al. Somatomotor mu rhythm amplitude correlates with rigidity during deep brain stimulation in parkinsonian patients. Clin Neurophysiol. 2012;123(10):2010–2017.
- Brinkman L, Stolk A, Marshall TR, et al. Independent causal contributions of alpha- and beta-band oscillations during movement selection. J Neurosci. 2016;36(33):8726–8733.
- Broetz D, Braun C, Weber C, et al. Combination of brain-computer interface training and goal-directed physical therapy in chronic stroke: a case report. Neurorehabil Neural Repair. 2010;24(7):674–679.
- Llanos C, Rodriguez M, Rodriguez-Sabate C, et al. Mu-rhythm changes during the planning of motor and motor imagery actions. Neuropsychologia. 2013;51(6):1019–1026.
- Mcfarland D, Mcfarland DJ, Sarnacki WA, et al. Effects of training pre-movement sensorimotor rhythms on behavioral performance effects of training pre-movement sensorimotor rhythms on behavioral performance. J Neural Eng. 2015;12(6):1–21.
- Ring C, Cooke A, Kavussanu M, et al. Investigating the efficacy of neurofeedback training for expediting expertise and excellence in sport. Psychol Sport Exerc. 2015;16:118–127.
- Cooke A, MacLeod C, Pritchard A, et al. Training the Brain to Mimic Medication: An Investigation of the Feasibility and Effectiveness of Home-Based “Brain Training” as a Non-Pharmacological Treatment for Motor Symptoms of Parkinson’s Disease. In: Poster Session Presented at International Association of Gerontology and Geriatrics – European Region Congress, Gothenburg, Sweden; 2019.
- Shmuel A. Locally measured neuronal correlates of functional MRI signals. In: Mulert C, Lemieux L, eds. EEG - FMRI. Physiological basis, technique, and applications. 1st ed. Berlin Heidelberg: Springer-Verlag; 2010. 63–82.
- Logothetis NK. The neural basis of the blood-oxygen-level-dependent functional magnetic resonance imaging signal. Philos Trans R Soc Lond B Biol Sci. 2002;357(1424):1003–1037.
- Magri C, Schridde U, Murayama Y, et al. The amplitude and timing of the BOLD signal reflects the relationship between local field potential power at different frequencies. J Neurosci. 2012;32(4):1395–1407.
- Jech R, Mueller K. Investigating network effects of DBS with fMRI. In: Connectomic deep brain stimulation. London, Oxford, Boston, New York und San Diego: Elsevier Inc.; 2022. 275–301.
- Poldrack RA. Can cognitive processes be inferred from neuroimaging data? Trends Cogn Sci. 2006;10(2):59–63.
- Stephan KE, Penny WD, Moran RJ, et al. Ten simple rules for dynamic causal modeling. Neuroimage. 2010;49(4):3099–3109.
- Kahan J, Urner M, Moran R, et al. Resting state functional MRI in Parkinson's disease: the impact of deep brain stimulation on 'effective' connectivity. Brain. 2014;137(Pt 4):1130–1144.
- Subramanian L, Hindle JV, Johnston S, et al. Real-time functional magnetic resonance imaging neurofeedback for treatment of Parkinson's disease. J Neurosci. 2011;31(45):16309–16317.
- Subramanian L, Morris MB, Brosnan M, et al. Functional magnetic resonance imaging neurofeedback-guided motor imagery training and motor training for Parkinson's disease: randomized trial. Front Behav Neurosci. 2016;10:111.
- Buyukturkoglu K, Rana M, Ruiz S, et al. Volitional Regulation of the Supplementary Motor Area with fMRI – BCI neurofeedback in Parkinson ’ s Disease : A Pilot Study. 2013. 6–8.
- Sorger B, Scharnowski F, Linden DEJ, et al. Control freaks: towards optimal selection of control conditions for fMRI neurofeedback studies. Neuroimage. 2019;186:256–265.
- Thibault RT, Lifshitz M, Raz A. The climate of neurofeedback: scientific rigour and the perils of ideology. Brain. 2018; 141(2):e11–2019.
- Hétu S, Grégoire M, Saimpont A, et al. The neural network of motor imagery: an ALE meta-analysis. Neurosci Biobehav Rev. 2013;37(5):930–949.
- Emmert K, Kopel R, Sulzer J, et al. Meta-analysis of real-time fMRI neurofeedback studies using individual participant data: how is brain regulation mediated? Neuroimage. 2016;124(Pt A):806–812.
- Zhao Z, Yao S, Zweerings J, et al. Putamen volume predicts real-time fMRI neurofeedback learning success across paradigms and neurofeedback target regions. Hum Brain Mapp. 2021;42(6):1879–1887.
- Lubianiker N, Goldway N, Fruchtman-Steinbok T, et al. Process-based framework for precise neuromodulation. Nat Hum Behav. 2019;3(5):436–445.
- Berman BD, Horovitz SG, Venkataraman G, et al. Self-modulation of primary motor cortex activity with motor and motor imagery tasks using real-time fMRI-based neurofeedback. Neuroimage. 2012;59(2):917–925.
- Mehler DMA, Williams AN, Krause F, et al. The BOLD response in primary motor cortex and supplementary motor area during kinesthetic motor imagery based graded fMRI neurofeedback. Neuroimage. 2019;184(September 2018):36–44.
- Blefari ML, Sulzer J, Hepp-Reymond M-C, et al. Improvement in precision grip force control with self-modulation of primary motor cortex during motor imagery. Front Behav Neurosci. 2015;9:18.
- Kober SE, Grössinger D, Wood G. Effects of motor imagery and visual neurofeedback on activation in the swallowing network: a real-time fMRI study. Dysphagia. 2019;34(6):879–895.
- Mehler DMA, Kording KP. The lure of misleading causal statements in functional connectivity research. arXiv. 2018.
- Koush Y, Ashburner J, Prilepin E, et al. Real-time fMRI data for testing OpenNFT functionality. Data Brief. 2017;14:344–347.
- Pilgramm S, de Haas B, Helm F, et al. Motor imagery of hand actions: decoding the content of motor imagery from brain activity in frontal and parietal motor areas. Hum Brain Mapp. 2016;37(1):81–93.
- Zabicki A, de Haas B, Zentgraf K, et al. Imagined and executed actions in the human motor system: Testing neural similarity between execution and imagery of actions with a multivariate approach. Cereb Cortex. 2016;27(9):4523–4536.
- Park C. h, Chang WH, Lee M, et al. Which motor cortical region best predicts imagined movement? Neuroimage. 2015;113:101–110.
- Kolasinski J, Dima DC, Mehler DMA, et al. Spatially and temporally distinct encoding of muscle and kinematic information in rostral and caudal primary motor cortex. Cereb Cortex Commun. 2020;1(1):tgaa009.
- Cho S, Min H, In M, et al. Multivariate pattern classification on BOLD activation pattern induced by deep brain stimulation in motor, associative, and limbic brain networks. Sci Rep. 2020;10(1):1–12.
- Kilmarx J, Oblak E, Sulzer J, et al. Towards a common template for neural reinforcement of finger individuation. Sci Rep. 2021;11(1):1–12.
- Russo AG, Lührs M, Di Salle F, et al. Towards semantic fMRI neurofeedback: navigating among mental states using real-time representational similarity analysis. J Neural Eng. 2021;18(4):046015.
- Cui X, Bray S, Bryant DM, et al. A quantitative comparison of NIRS and fMRI across multiple cognitive tasks. Neuroimage. 2011;54(4):2808–2821.
- Strangman G, Culver JP, Thompson JH, et al. A quantitative comparison of simultaneous BOLD fMRI and NIRS recordings during functional brain activation. Neuroimage. 2002;17(2):719–731.
- Scarapicchia V, Brown C, Mayo C, et al. Functional magnetic resonance imaging and functional near-infrared spectroscopy: insights from combined recording studies. Front Hum Neurosci. 2017;11:419–412.
- Klempir O, Krupicka R, Mehnert J, et al. Reshaping cortical activity with subthalamic stimulation in Parkinson’s disease during finger tapping and gait mapped by near infrared spectroscopy. J Appl Biomed. 2019;17(3):157–166.
- Orcioli-Silva D, Vitório R, Nóbrega-Sousa P, et al. Levodopa Facilitates Prefrontal Cortex Activation During Dual Task Walking in Parkinson Disease. 2020.
- Kohl SH, Mehler DMA, Lührs M, et al. The potential of functional near-infrared spectroscopy-based neurofeedback – a systematic review and recommendations for best practice. Front Neurosci. 2020;14:594.
- Mihara M, Hattori N, Hatakenaka M, et al. Near-infrared spectroscopy-mediated neurofeedback enhances efficacy of motor imagery-based training in poststroke victims: a pilot study. Stroke. 2013;44(4):1091–1098.
- Mihara M, Fujimoto H, Hattori N, et al. Effect of Neurofeedback Facilitation on Poststroke Gait and Balance Recovery: A Randomized Controlled Trial. Vol 96; 2021.
- Klein F, Debener S, Witt K, et al. fMRI ‑ based validation of continuous – wave fNIRS of supplementary motor area activation during motor execution and motor imagery. Sci Rep. 2022;12(1):1–20.
- Mehler DMA, Williams AN, Whittaker JR, et al. Graded fMRI neurofeedback training of motor imagery in middle cerebral artery stroke patients: a preregistered proof-of-concept study. Front Hum Neurosci. 2020;14:226–217.
- Uchitel J, Vidal-Rosas EE, Cooper RJ, et al. Wearable, Integrated EEG – fNIRS Technologies : A Review. 2021.
- Trambaiolli LR, Cassani R, Mehler DMA, et al. Neurofeedback and the aging brain: a systematic review of training protocols for dementia and mild cognitive impairment. Front Aging Neurosci. 2021;13:682683.
- Wang T, Mantini D, Gillebert CR. The potential of real-time fMRI neurofeedback for stroke rehabilitation: a systematic review. Cortex. 2018;107:148–165.
- Trambaiolli L, Kohl SH, Linden DEJ, et al. Neurofeedback training in major depressive disorder: a systematic review of clinical efficacy, study quality and reporting practices. Neurosci Biobehav Rev. 2021;125:33–56.
- Schönenberg M, Wiedemann E, Schneidt A, et al. Neurofeedback, sham neurofeedback, and cognitive-behavioural group therapy in adults with attention-deficit hyperactivity disorder: a triple-blind, randomised, controlled trial. Lancet Psychiatry. 2017;4(9):673–684.
- Chenoweth L, Gallagher R, Sheriff JN, et al. Factors supporting self-management in Parkinson's disease: implications for nursing practice. Int J Older People Nurs. 2008;3(3):187–193.
- Ellis T, Cavanaugh JT, Earhart GM, et al. Factors associated with exercise behavior in people with Parkinson disease. Phys Ther. 2011;91(12):1838–1848.
- Zhuo C, Xue R, Luo L, et al. Efficacy of antidepressive medication for depression in Parkinson disease: a network meta-analysis. Medicine. 2017;96(22):e6698.
- Colagiuri B, Schenk LA, Kessler MD, et al. The placebo effect: from concepts to genes. Neuroscience. 2015;307:171–190.
- de la Fuente-Fernandez R, Stoessl AJ. The placebo effect in Parkinson’s disease. Trends Neurosci. 2002;25(6):302–306.
- Algermissen J, Mehler DMA. May the power be with you: are there highly powered studies in neuroscience, and how can we get more of them? J Neurophysiol. 2018;119(6):2114–2117.
- Horváth K, Aschermann Z, Ács P, et al. Minimal clinically important difference on the motor examination part of MDS-UPDRS. Parkinsonism Relat Disord. 2015;21(12):1421–1426.
- Nijhuis FAP, Esselink R, de Bie RMA, et al. Translating evidence to advanced Parkinson's disease patients: a systematic review and meta-analysis. Mov Disord. 2021;36(6):1293–1307.
- Stefan AM, Gronau QF, Schönbrodt FD, et al. A tutorial on bayes factor design analysis using an informed prior. Behav Res Methods. 2019;51(3):1042–1058.
- Allen CPG, Mehler DMA. Open science challenges, benefits and tips in early career and beyond. PLoS Biol. 2019;17(5):e3000246.
- Scheel AM, Schijen M, Lakens D. An excess of positive results: Comparing the standard psychology literature with registered reports. Preprint. 2020.
- Ros T, Enriquez-Geppert S, Zotev V, et al. Consensus on the reporting and experimental design of clinical and cognitive-behavioural neurofeedback studies (CRED-nf checklist). Brain. 2020;143(6):1674–1685.
- Mehler DMA. Clinical applications of real-time FMRI neurofeedback training – premises, promises, and pitfalls. PhD thesis at Cardiff University 2018.