Abstract
Introduction
The Positive and Negative Affect Schedule (PANAS) is a self-report questionnaire that consists of two scales to measure positive and negative affective states. An unresolved debate exists whether positive and negative affective states can co-exist or are mutually exclusive. Furthermore, it is unclear how the presence of a dysmetabolic state that affects cognition, such as chronic kidney disease (CKD), impacts these states.
Aims
We approach this problem using a non-linear method of dimensionality reduction, the Uniform Manifold Approximation and Projection (UMAP), which has the advantage of preserving the data’s global structure.
Results
We show its application in a cohort of 42 CKD patients and 100 age- and gender-matched healthy volunteers (age 57 ± 20 years). UMAP algorithm allowed to identify two mutually exclusive affective states. The presence of CKD impacts both states.
Conclusion
This new methodology and its exemplificative application to CKD are discussed here for the first time.
1. Introduction
Although classical psychology would distinguish the cognitive and affective domains, this separation seems far less clear today [Citation1]. Affective states are subjective experiences that can be studied by questionnaires (self-reported or interviews) or by objective measures such as the facial electromyography (EMG) and autonomic activity [Citation1].
The term “state” as an adjunct of “affect” (“affective state”) underlines that this subjective experience is an unstable property of subjects and varies with poorly described dynamics, albeit often assumed to be cyclic [Citation2]. To quantify and study affect, three American psychologists David Watson, Lee Anna Clark and Auke Tellegen have developed the Positive and Negative Affective State (PANAS) scale in 1988 [Citation3].
A general problem of the quantification of the affective state is that it might be a complex multidimensional phenomenon; thereby, the attempt to reduce it into one or two variables, such as the positive/negative valence, motivational intensity, and arousal may not well represent the phenomenon [Citation4]. Therefore, it would be desirable to use (non-linear) techniques of dimensional reduction that are able to maintain the global structure of the data.
In addition, when generating synthetic or summary variables from a multidimensional space, the resulting “affective variable” may have a chaotic dynamic, as it is generated from latent variables each with a specific complex dynamic. Furthermore, the synthetic variable should be expected to exhibit a more complex dynamical behavior over time than the latent variables it derives from.
2. PANAS
The PANAS is a self-report questionnaire divided into positive affect (PA) and negative affect (NA) [Citation3]. The Positive Affect is constituted of several affects such as: Attentive, Active, Alert, Excited, Enthusiastic, Determined, Inspired, Proud, Interested, and Strong. The Negative Affect subsumes a broad range of aversive affects including fear, nervousness, guilt, and shame. The PANAS scales show good psychometry properties and they have been translated into several languages [Citation5].
There are two versions of Panas: Panas–SF (short-form) with 10 items and an expanded version of the PANAS (the PANAS-X) with 60 items [Citation6–8].
An unresolved debate exists regarding the mutual exclusion or partial overlap of positive and negative states [Citation3,Citation9–11]. The answer to this question might depend upon a problem of definition. On one side, it is possible that the definition of some of the positive and negative items is different among subjects. Some items, such as “excited” or “proud” might have positive or negative value according to the state of the subject. As discussed above, the definition of positive and negative affective states might be regarded as an attempt to reduce the dimensionality of a multidimensional phenomenon into two variables. This reduction should, however, maintain the global structure of the data.
We approach this problem from this perspective. We consider each item of the PANAS test as a variable carrying an information not completely overlapping with the others. Therefore, the problem is trying to reduce the multidimensional structure of the PANAS into the smallest number of variables. Several approaches are possible, and here we adopt for the first time the use of the UMAP algorithm which tries to preserve the overall structure of the data [Citation12]. Indeed, UMAP is a technique to reduce the dimensionality of data and is widely used in the fields of “omics” (proteomic, transcriptome data) where hundreds or thousands of data are gathered for each sample. This algorithm allows reducing the number of dimensions while maintaining the structure of the data. It is useful both for the representation of high dimensional data structures and for their analysis.
Compared with other algorithms such as Principal Component Analysis, UMAP is not a linear technique and has a better preservation of the overall structure of the data.
Our reasoning is that unsupervised reduction of PANAS data could identify mutually exclusive states. If this premise holds true, the oscillation between these two states may be used as a strategy to analyze the temporal dynamics of affective states.
A vast amount of literature describes a change in cognitive abilities and mood in patients with chronic kidney disease (CKD) at various stages and in patients in dialysis [Citation13,Citation14].
Specifically, a large percentage of patients with CKD (about 25%) show a mild cognitive impairment already at early stages of CKD [Citation15–17]. Furthermore about 15% of patients with CKD show signs of depression [Citation15]. Although it may seem tempting to explain the cognitive and mood changes as due to the psychological burden of a diagnosis of a chronic disease at risk of progression, organic factors (e.g., uremic toxins, anemia, electrolytes and water unbalance) are likely to play a major role in this brain-body link [Citation18,Citation19]. Specifically, animal models of CKD also show cognitive dysfunction and depression, compared with sham-operated animals [Citation20]. Furthermore, patients with CKD also show subtle motor alterations (e.g., tremor and gait dysfunctions) [Citation21,Citation22]. Finally, the progression of cognitive and mood seems to follow the amount of kidney dysfunction and these are not fully reversed by kidney transplantation [Citation23].
A recent meta-analysis reports a 59% increased risk for death in adults with CKD and comorbid depression when compared with those with little or no depressive symptoms. Surprisingly though, the literature on the influence of positive psychological attributes in patients with CKD is virtually non-existent [Citation24].
Knowing the emotional state of patients may help healthcare professionals to establish therapeutic relationships. Therefore, the use of tools for affective states (such as PANAS) may represent a step forward in improving the overall care and quality of life of patients with kidney disease.
The present paper analyses the positive and negative affect in several time periods, showing the possibility to reduce affect states in only two-dimension through Uniform Manifold Approximation and Projection (UMAP). UMAP is a powerful algorithm to perform dimension reduction while accurately representing local structure of data and incorporating the global structure. There are several dimension reduction techniques including those performing a linear transformation of data like the well-known Principal Component Analysis (PCA) [Citation25]. However, PCA is a linear method and does not perform well in cases where relationships are non-linear. Nonlinear and high dimensional data can be difficult to interpret, and therefore UMAP makes it easier to view data. This approach is used to analyze the temporal trajectories of mutually exclusive states.
3. Materials and methods
3.1. Participants
Patients with chronic kidney disease (CKD) were recruited from the UOC Nephrology and Dialysis of the University of Campania. Control subjects consisted of age- and gender-matched healthy volunteers; 42 CKD patients and 100 controls was enrolled for this study. Although sample unbalance may result in lower statistical power, the analysis carried out here is based on a large number of variables (20 items from the PANAS). A large dataset of control patients makes the UMAP algorithm more stable regarding the solution found. The CKD patients were then projected in the same space of the control patients to have comparable structural information of the starting dataset.
Clinically relevant variables of the populations under study are reported in . This retrospective study was approved by the Ethics Committee of the Second University of Naples, Italy [Citation26].
Table 1. Clinically relevant variables of the populations under study.
3.2. Procedure
The Positive and Negative Affective State (PANAS) test [Citation3] is a measure of affect (20 items such as “excited,” “scared,” etc.) [Citation27]. The PANAS reliability and construct validity have been already validated in a large sample [Citation27].
The test was administered to the participants, instructing them to indicate how much they felt the items, on a 5-point Likert scale (1 = Very slightly or not at all, 5 = Extremely) [Citation28]. The results were analyzed according to the original method consisting of summing all positive items and, separately, all negative items, thereby giving two different scores (PA score and NA score).
Depression was tested using the Geriatric Depression Scale (GDS). None of the patients showed signs of depression.
3.3. Uniform manifold approximation and projection for dimension reduction (UMAP) analysis
To perform UMAP analysis of PANAS data, a custom script written in R language has been used (freely available from https://cran.r-project.org/). The library umap has been implemented (https://cran.r-project.org/web/packages/umap/index.html).
UMAP parameters used for analysis (umap command in umap library of R software) were as follows:Number of neighbors: 15; number of UMAP components: 2; metric: Euclidean; number of epochs: 200; minimum distance: 0.1, local connectivity: 1; bandwidth: 1; alpha: 1; gamma: 1; negative sample rate: 5.
3.4. Statistics
Data have been plotted using the ggplot libraries in R language. Clusters of data have been identified using k-means algorithm. Statistical differences between groups (CKD and healthy volunteers) have been analyzed using Student’s t-test.
4. Results
To calibrate the data for the UMAP algorithm, we have first analyzed temporal data from one healthy volunteer who collected PANAS scores twice per day over a period of one month. The resulting dynamics of classical positive- and negative-affective scores (PA, NA respectively) are shown in . There is no clear temporal evolution of the PA and NA scores. Conversely, the UMAP representation of the PANAS items shows two different states and the subject alternates between these two states. These states do not correspond to PA or NA scores: they are embedded in the structure of the PANAS items but have no simple relationship with the two classical affective scores. The ability of UMAP to extract two different and mutually exclusive affective states from the PANAS items is then exploited to analyze the population of CKD patients and healthy volunteers. As shown in , both populations show two different clusters in the UMAP representation. Within each cluster, the CKD and the healthy volunteers can be differentiated. A Students’ t-test for UMAP1 and UMAP 2 variables differences between CKD and healthy volunteers shows a significant difference between the two groups (p ≪ 0.05).
Figure 1. Dynamical aspects of PANAS. Left: using the standard NA and PA scores, mood states are poorly differentiated. Right: using PANAS two major states are evident within the subject, with a clear oscillation over the days. Nodes are individual PANAS tests. Lines connect temporally successive PANAS tests. Colors change with time and give an overview of the time trajectories.
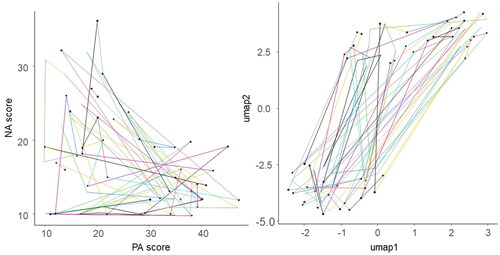
Figure 2. PANAS states are best described by UMAP analysis with good discrimination of CKD and control subjects. Left: Positive and Negative affective scores (PA, NA respectively) do not discriminate different states in the subjects. Right: UMAP analysis shows two distinct clusters in the affective states and within each cluster controls and CKD patients can be differentiated. Nodes are individual PANAS tests.
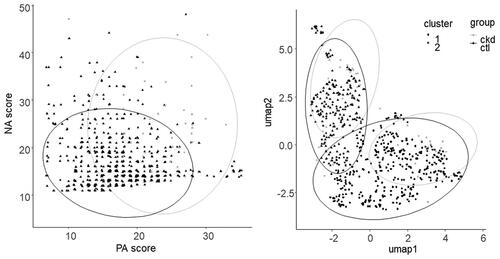
5. Discussion
The main result of the present study is that a UMAP representation of the PANAS items shows the presence of two different affective states, which appear mutually exclusive when temporal data are considered. However, these two affective states do not correspond to the Negative and Positive affective states that have been discussed in the literature.
The interpretation of UMAP states in terms of the original PANAS items is not simple. Other dimensionality reduction methods, such as Principal Component Analysis (PCA) are based on a linear combination of the original variables, and therefore the “synthetic variables” (principal components) can be expressed in terms of combination of an initial set of variables. UMAP, instead, is based on a different concept.
The original variables (in our case the PANAS items such as “excited,” “scared,” etc.) are plotted in the 20-dimensional space (one dimension for each item). Therefore, each subject will be a point in this 20-dim space. Some subjects will be closer in this 20-dim space and others will be far apart. UMAP tries to project these points in a 2D space (with axes UMAP1 and UMAP2) so that points that were neighbors will remain neighbors in the plane, therefore preserving the structure of the original data. To have an intuitive understanding of this process, suppose we have a 3D model, such as points on the edges of a cube, and we want to represent them on a flat, 2D, surface. PCA would fail to maintain the structure of the initial data. Conversely, UMAP tries to project the initial 3D model on a surface so that the vertices will remain vertices, and the sides of the cube will remain sides in the 2D drawing.
Therefore, when analyzing the UMAP graph, the reader has a visual simplification of the original dataset, allowing to identify, for example, clusters of data; conversely, the axes UMAP1 and UMAP2 should not be considered as a combination of the original data as in PCA. In , this concept is visually represented.
Figure 3. Different interpretation of UMAP representations and PCA analysis. UMAP tries to identify the structure of the data (in this case the wireframe of a cube) and to represent it in lower dimensional (2D) space. PCA finds synthetic variables as linear combination of the original variables (in our case the x,y,z position of points on a cube) to minimize the variance of the data, ignoring the structure of the dataset.
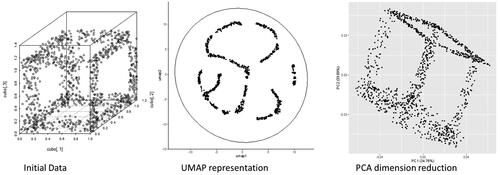
Therefore, the longstanding debate whether positive and negative affective states are mutually exclusive or can be present at the same time and can be solved from a different perspective: there are two different affective states, and these are mutually exclusive, and these affective states can be retrieved using UMAP analysis. However, these states do not correspond to NA and PA states.
Affective analysis has focused on finding two synthetic variables and deciding whether, using these two synthetic variables, there are two mutually exclusive emotional states or just one. We propose to solve this problem using a different approach that analyzes the data structure of the multidimensional test used in the affective analysis. This approach not only shows that there are two mutually exclusive states, but also that they can be used to analyze the temporal trajectories of subjects in future studies. Finally, we show that this approach can be used to better identify the separation between CKD patients and controls. Since the aim of the manuscript is to present a new methodological approach, a great amount of work is opened to scientists willing to explore the structure of this specific psychological test and of other tests in normal subjects and in diseased patients. The presence of an altered affective state in CKD patients is interesting and might be linked to psychological and environmental aspects (such as the knowledge of an incurable chronic disease) and organic factors (such as the presence of uremic toxins, and water and electrolyte imbalances). Future investigations should try to disentangle these two possible explanations.
CKD patients show a significant difference in UMAP affective states compared with healthy volunteers. As we do not have temporal data for CKD patients, we cannot study dynamical changes of the affective states in the CKD population. The transversal nature of this study does not allow us to understand the temporal dynamics of UMAP states.
In conclusion, future studies should use UMAP analysis to identify mutually exclusive affective states and to study their dynamics in both healthy subjects and in patients with CKD.
Disclosure statement
No potential conflict of interest was reported by the author(s).
References
- Duncan S, Barrett LF. Affect is a form of cognition: a neurobiological analysis. Cogn Emot. 2007;21(6):1184–1211.
- Winthorst WH, Bos EH, Roest AM, et al. Seasonality of mood and affect in a large general population sample. PLoS One. 2020;15(9):e0239033.
- Watson D, Clark LA, Tellegen A. Development and validation of brief measures of positive and negative affect: the PANAS scales. J Pers Soc Psychol. 1988;54(6):1063–1070.
- Sander D. Models of emotion. In: Armony J, Vuilleumier P, editors. In: The cambridge handbook of human affective neuroscience. Cambridge: Cambridge University Press; pp. 5–54.
- Terracciano A, McCrae RR, Costa PT. Factorial and construct validity of the Italian positive and negative affect schedule (PANAS). Eur J Psychol Assess. 2003;19(2):131–141.
- Thompson ER. Development and validation of an internationally reliable short-form of the positive and negative affect schedule (PANAS). J Cross Cult Psychol. 2007;38:227–242.
- Sanmartín R, Vicent M, Gonzálvez C, et al. Positive and negative affect schedule-short form: factorial invariance and optimistic and pessimistic affective profiles in Spanish children. Front Psychol. 2018;9:392.
- Parrish BP, Zautra AJ, Davis MC. The role of positive and negative interpersonal events on daily fatigue in women with fibromyalgia, rheumatoid arthritis, and osteoarthritis. Health Psychol. 2008;27(6):694–702.
- Cacioppo JT, Berntson GG. Relationship between attitudes and evaluative space: a critical review, with emphasis on the separability of positive and negative substrates. Psychol. Bull. 1994;115(3):401–423.
- Watson D, Clark LA. Negative affectivity: the disposition to experience aversive emotional states. Psychol Bull. 1984;96:465–490.
- Russell JA, Carroll JM. On the bipolarity of positive and negative affect. Psychol Bull. 1999;125(1):3–30.
- McInnes L, Healy J, Saul N, et al. UMAP: uniform manifold approximation and projection. J Open Source Softw. 2018;3(29):861.
- Viggiano D, Wagner CA, Blankestijn PJ, et al. Mild cognitive impairment and kidney disease: clinical aspects. Nephrol Dial Transplant. 2020;35(1):10–17.
- Viggiano D, Wagner CA, Martino G, et al. Mechanisms of cognitive dysfunction in CKD. Nat Rev Nephrol. 2020;16:452–469.
- Liabeuf S, Pepin M, Franssen CFM, et al. Chronic kidney disease and neurological disorders: are uraemic toxins the missing piece of the puzzle? Nephrol Dial Transplant. 2021;37:ii33-ii44.
- Pépin M, Ferreira AC, Arici M, et al. Cognitive disorders in patients with chronic kidney disease: specificities of clinical assessment. Nephrol Dial Transplant. 2021;37:ii23-ii32.
- Bikbov B, Soler MJ, Pešić V, et al. Albuminuria as a risk factor for mild cognitive impairment and dementia—what is the evidence? Nephrol Dial Transplant. 2021;37:ii55–ii62.
- Viggiano D, Bruchfeld A, Carriazo S, et al. Brain dysfunction in tubular and tubulointerstitial kidney diseases. Nephrol Dial Transplant. 2021;37:ii46–ii55.
- Silva I, Unwin PH, Hoorn R, et al. Acidosis, cognitive dysfunction and motor impairments in patients with kidney disease. Nephrol Dial Transplant. 2021;37:ii4–ii12.
- Bobot M, Thomas L, Moyon A, et al. Uremic toxic Blood-Brain barrier disruption mediated by AhR activation leads to cognitive impairment during experimental renal dysfunction. J Am Soc Nephrol. 2020;31(7):1509–1521.
- Tran J, Ayers E, Verghese J, et al. Gait abnormalities and the risk of falls in CKD. Clin J Am Soc Nephrol. 2019;14(7):983–993.
- Otobe Y, Hiraki K, Hotta C, et al. Mild cognitive impairment in older adults with pre-dialysis patients with chronic kidney disease: prevalence and association with physical function. Nephrology. 2019;24(1):50–55.
- Sharma A, Yabes J, Al Mawed S, et al. Impact of cognitive function change on mortality in renal transplant and end-stage renal disease patients. Am J Nephrol. 2016;44(6):462–472.
- Hernandez R, Lash JP, Burrows B, et al. The association of positive affect and cardiovascular health in hispanics/latinos with chronic kidney disease: results from the hispanic community health study/study of latinos (HCHS/SOL). Prev Med Rep. 2019;15:100916.
- Jolliffe IT, Cadima J. Principal component analysis: a review and recent developments. Philos Trans A Math Phys Eng Sci. 2016;374(2065):20150202.
- Viggiano D, De Santo NG, Amruthraj NJ, et al. Renal response to an oral protein load in patients with Central diabetes insipidus before and after treatment with vasopressin. J Nephrol. 2019;32:411–415.
- Crawford JR, Henry JD. The positive and negative affect schedule (PANAS): construct validity, measurement properties and normative data in a large non-clinical sample. Br J Clin Psychol. 2004;43(Pt 3):245–265.
- Merz EL, Malcarne VL, Roesch SC, et al. Psychometric properties of positive and negative affect schedule (PANAS) original and short forms in an African American community sample. J Affect Disord. 2013;151:942–949.