Abstract
To optimize regional crop rotation systems to mitigate soil organic carbon (SOC) depletion, monitoring the spatial and temporal variation of the SOC budget is of major importance. This study evaluated the influence of crop rotation and topography on the spatial and temporal variation of the SOC budget under cereal cropping in northern Kazakhstan using a geostatistical method, predicted equations of CO2 emission and Moderate Resolution Imaging Spectroradiometer satellite images. The study was carried out in large-scale upland fields (7,915 ha) located in Shortandy, Aqmorinskaya Oblast, from 2001 to 2005. Under the assumption that all crop residue was incorporated into the soil, average annual C input as plant residues in cereal and abandoned fields ranged from 1.0 to 1.6 Mg C ha−1 year−1, with higher values in wet years. Average annual CO2 emission in the cereal fields and the fallow fields ranged from 1.0 to 1.2 Mg C ha−1 year−1 and from 0.7 to 0.8 Mg C ha−1 year−1, respectively. In relation to topography, CO2 emission was higher on the north-facing slope than the south-facing slope, with the highest values on the higher plateau, and the average annual carbon budget ranged from –0.1 to 0.3 Mg C ha−1 year−1. It should be noted that all fallow fields consistently had a negative carbon budget. The 5-year carbon budget for different crop rotation systems ranged from –0.5 (fallow-based rotation) to 0.6 (wheat-based 5-year continuous cereal cropped) Mg C ha−1. These results have some uncertainly because of the low predictive ability of the regression; however, the results indicated that fallow-based and barley-based crop rotation systems were depletive and conversion of the wheat-fallow system to continuous wheat cropping would sequestrate 0.1–0.3 Mg ha−1 year−1 of carbon into the soil.
INTRODUCTION
Central Asia, covering a total arable area of 31 Mha, produces wheat as the major crop, especially in rainfed agricultural zones (CitationFood and Agriculture Organization 2005). The main rainfed, grain-farming region is distributed in northern Kazakhstan, which is covered with fertile Chernozem soils (CitationMain management of geology and cartography, USSR (GUGK) 1982) that are also considered to store a substantial amount of organic carbon, functioning as a huge source and sink of CO2. The Chernozem soils in northern Kazakhstan are, therefore, a vital resource from both an agricultural and an environmental viewpoint.
The conversion of grasslands into croplands and poor soil management, including disturbance of the soil surface by conventional tillage and the resulting soil erosion, have caused various environmental problems in Central Asia (CitationLal and Bruce 1999). The loss of soil organic carbon (SOC) is accentuated by deforestation, land reclamation, attendant soil degradation and desertification, all of which are severe problems in this region (CitationLal 2004). The CitationWorld Bank (2003) reported that 10–30% of soil humus was lost in the arable surface soil of Kazakhstan between 1960 and 1995, with the rainfed agricultural region in northern Kazakhstan most critically affected.
For nearly 50 years of wheat monoculture in this region, crop rotations included summer fallows to retain moisture, accumulate nutrients through mineralization and control weed infestation. Fields under fallow are usually mechanically harrowed several times to keep the land bare and to minimize evapotranspiration during the cropping season. This practice was also commonly applied in the steppe area of North America in which the annual precipitation is below 500 mm. CitationFunakawa et al. (2004a,Citationb) reported high cereal yields from post-fallow fields at the expense of soil organic matter depletion. In addition, CitationKarbozova-Saljinikov et al. (2004) found an inverse relationship between the amount of potentially mineralizable carbon (PMC) in soil and the frequency of fallow in northern Kazakhstan, suggesting that a fallow-based crop rotation system would lead to depletion of the SOC. To fully understand such information in terms of agricultural practices, it is essential to determine the influence of the crop rotation system on the SOC budget in northern Kazakhstan.
The SOC budget can be evaluated from the carbon input as crop residues and the carbon output as CO2 emission. CitationFunakawa et al. (2004b) suggested that carbon input as crop residues had a positive correlation with soil water content on seeding time in northern Kazakhstan, and that soil water content was affected by the crop rotation phase. In addition, the spatial pattern of soil water content depends on the agro-landscape in this region (CitationYanai et al. 2005). Thus, the spatial pattern of carbon input as plant residues could be affected by the crop rotation phase and topography in northern Kazakhstan. The widely accepted method of remote sensing has been used to evaluate this spatial pattern precisely (CitationGoward et al. 1991; CitationInoue and Olioso 2006; CitationJackson and Pinter 1986; CitationJustice et al. 1985; CitationRunning et al. 1994; CitationTucker and Sellers 1986). Many studies have shown relationships of red and near-infrared reflected energy to the amount of vegetation present on the ground (CitationColwell 1974). The Normalized Difference Vegetation Index (NDVI) is the most popular index and the most used index in various remote sensing applications (CitationDeering 1978; CitationHuete et al. 1999; CitationLabus et al. 2002; CitationNicholson and Farrar 1994). This index, however, is subject to the influences of soil and air conditions (CitationHuete 1988). CitationHuete (1988), therefore, developed the Soil Adjusted Vegetation Index (SAVI) to remove much of the ground contamination associated with soil brightness variations. The basis of SAVI in minimizing “soil noise” has been corroborated by numerous studies in grassland and agricultural areas (CitationBausch 1993; CitationHuete et al. 1992; CitationQi et al. 1993). Moreover, new satellite sensors, such as the Moderate Resolution Imaging Spectroradiometer (MODIS), have enabled the spatial and temporal variation of plant properties to be measured at an agro-landscape level because the sensor's red and infrared band resolutions are 250 m. However, very few studies have examined the spatial and temporal variation of carbon input as plant residues in the important rainfed agricultural regions of northern Kazakhstan.
Carbon output as CO2 emission is another key factor for evaluating the SOC budget. CitationYanai et al. (2005) showed that PMC content was affected by topography, and mentioned the need for estimating absolute values of CO2 emission under field conditions. In addition, CitationTakata et al. (2007a) reported that CO2 emission under field conditions in this region could be estimated using soil temperature, precipitation and PMC data of soil, and further suggested that carbon output as CO2 emission depended on land use as well as field condition. To optimize the regional crop rotation system to mitigate SOC depletion, it is important to understand the influence of the crop rotation system on the spatial and temporal variation of the SOC budget. Therefore, this study sought to evaluate the influence of crop rotation and topography on the spatial and temporal variation of the SOC budget. To this end, the present study attempts to: (1) predict the spatial and temporal variability of carbon input as crop residues using multi-temporal MODIS satellite images, (2) clarify the spatial and temporal variability of field properties and SOC output as CO2 emission using geostatistics and model equations, (3) clarify the spatial and temporal variability of the SOC budget using the results from (1) and (2).
MATERIALS AND METHODS
Study site
The study was conducted in large-scale, rainfed agricultural fields (N51°30-37′, E70°08-17′) located near the Barayev Kazakh Research and Production Center of Grain Farming in Shortandy, Aqmorinskaya Oblast, northern Kazakhstan (). The area is characterized by a continental climate with hot summers and cold winters, and mean annual precipitation is 323 mm with large variations between years. The average annual air temperature is 1.6°C, and the cultivation season is from mid-May to mid-September. A preliminary study showed that the general properties of the surface soil in this area were as follows: pH (H2O), 7.9–8.1; electrical conductivity, 0.15–0.24 dS m−1; texture, light clay to silty clay; cation exchange capacity, 27.8 cmolc kg−1. The soil of the study site was, therefore, classified as Typic Haplustolls (CitationSoil Survey Staff 2003), Haplic Chernozem (CitationFood and Agriculture Organization, International Soil Reference and Information Centre (ISRIC) and International Union of Soil Sciences (IUSS) 1998) or Southern Chernozem based on the classification system of the former Soviet Union.
The boundaries of the arable fields in the study area () were delineated by eye using Aster images taken on 19 June 2004. The total area of extracted arable fields was 7,915 hectares. Land use during the study period from 2001 to 2005 was recorded by field observation (). The 5-year crop rotation system was grouped into six classes as follows: wheat-based 5-year cereal (W-5-yr), wheat-based 4-year cereal with 1-year fallow, (W-4-yr), barley-based 5-year cereal (B-5-yr), barley-based 4-year cereal with 1-year fallow (B-4-yr), fallow-based (F) and others (O). Descriptions of these crop rotation systems are given in .
Topographic characteristics
The digital elevation model data shown in at a resolution of 90 m for the study area was downloaded from the NASA website (STRM-3: http://www2.jpl.nasa.gov/srtm. Elevation ranged from 382 to 435 m; the central plateau showed the highest elevation and the north-facing and south-facing slopes gently stretched away from the plateau. The terrain attributes included elevation (ELEV), slope (SLOPE), plan curvature (PLANC), profile curvature (PROFC) and compound topographic index (CTI). PLANC is the curvature of the corresponding normal section tangential to a contour. Negative PLANC indicates concentration and positive divergence of flow. PROFC is the curvature of the corresponding normal section tangential to a flow-line. PROFC is negative when the normal section concavity is directed up and positive in the opposite case. The CTI represents landscape areas where water accumulates (CitationMoore et al. 1993) and is a quantification of catenary landscape position. The index is defined as:
Table 1 Description of the crop rotation systems
Soil and plant sampling and analytical methods
The soil sampling method of CitationYanai et al. (2005) was used for the 66 sampling plots (1 km × 1 km each) shown in . Surface soil samples (0–15 cm) were collected in May 2001 and 2003 at five points within 10 m of the center of each plot. The samples were mixed and used for analysis. In addition, soil samples (0–90 cm) for the determination of soil water content were collected every 15 cm at the center of each plot using a hand auger during the seeding period (late May to beginning of June) from 2001 to 2004. The soil water content was determined by drying the soil sample at 105°C for 24 h. The PMC content was determined for the surface soil samples by incubating the samples for 19 weeks under conditions of 30°C air temperature and a soil water content of 60% of field capacity for 15 g of soil. Emitted CO2 from the soil samples was collected by alkaline trap and titrated with 0.1 mol L−1 HCl. Titration of the alkali trap was conducted every 2–4 weeks. The PMC was obtained after fitting the data of mineralized carbon to a first-order kinetic model using a non-linear regression model (SPSS, Chicago, USA, 1998):
Plant sampling was carried out during the harvest period (late August to September) from 2001 to 2005. In above-ground and below-ground 1 m × 0.5 m quadrats (2001 and 2002 1 m × 0.92 m quadrats), the plant parts were harvested in representative non-fallow plots. When predicting plant biomass using the satellite sensor, underestimates could occur at a contaminated pixel, for example, the existence of a road in a pixel. As 10 of 66 sampling points were close to a road (within 250 m), we did not collect plant samples at these points. The total numbers of sampling points in 2001 to 2005 were 47, 51, 44, 55 and 36, respectively. Harvested plant samples were separated into ears, stems, leaves and roots, dried at 70°C for 24 h and weighed. For the ear samples, grains were threshed with a hand thresher and weighed to represent the yield. Total carbon content of the dried samples was measured using the dry combustion method (Sumika NC-800-13N, Osaka, Japan).
Mapping of annual CO2 emission
To estimate annual CO2 emission, the daily CO2 emission from both cereal and fallow fields was estimated using two equations provided by CitationTakata et al. (2007a), which were modeled on the measured CO2 emission using the trenching method (exclusion of root respiration) at the same study area in 2002, 2004 and 2005, as follows:
The daily soil temperature maps were computed using MODIS Land Surface Temperature/Emissivity 8-Day L3 Global 1 km products (MOD11A2V4) (The Land Processes Distributed Active Archive Center (LP DAAC), South Dakota, USA). The MOD11A2V4 data during the plant growth season (1 April to 31 October) from 2001 to 2005 were downloaded from the MODIS website http://edcimswww.cr.usgs.gov/pub/imswelcome. The MOD11A2V4 contains the daytime and night-time surface temperature (CitationWan 2006), and the mean surface temperature data was calculated using both. To create the relative surface temperature map, each mean value of MOD11A2V was divided by the pixel value of the MOD11A2V located at the Barayev Institute. Air temperature and soil temperature at a depth of 5 cm were monitored at the Barayev Institute using a micro-logger (from 21 April to 21 September 2004; 30-min interval), and the converted equation between the air and soil temperatures was calculated:
It was assumed that the amount of precipitation was the same throughout the study area. D9 was calculated using the daily soil temperature map and the daily precipitation data monitored by the Barayev Institute. Then, daily CO2 emission (resolution was 250 m) was calculated for each land-use type, using the land-use map, PMC distribution map, daily soil temperature map, D9 distribution map and P15 data. The relationship between the measured CO2 emission and the predicted CO2 emission is shown in . The RMSE was 3.81, and appeared to be underestimated because the actual CO2 emission was higher than 15 kg C ha−1 day−1. However, such a high rate of CO2 emission was observed only in June and July 2002 (CitationTakata et al. 2007a). As reported by CitationTakata et al. (2007a), there was a new monthly precipitation record in June 2002 at the site. Hence, the rate of CO2 emission in June and July 2002 might be not be representative of the study area. Finally, annual CO2 emission was calculated as the sum of daily CO2 emission from 1 April to 31 October.
Satellite image processing for estimating biomass production
The MODIS surface reflectance daily L2G global 250 m products (MOD09GQK and MDY09GQK (The Land Processes Distributed Active Archive Center (LP DAAC), South Dakota, USA)) were used for estimating crop yield and carbon input as plant residues. In the present study, we assumed that all plant residues were incorporated into the soil. The MOD09GQK and MDY09GQK data during the crop growth stage (12 July to 23 August) from 2001 to 2005 (MDY09GQK; from 2002 to 2005) were downloaded. The MOD09GQK and MDY09GQK products contain red and near-infrared channels, which have been used for computing the vegetation index. In this study, the Modified Soil Adjusted Vegetation Index (MSAVI) was used to estimate plant biomass and was computed as follows (CitationQi et al. 1994):
The maximum value compositing (MVC) technique was used to create a cloud-free MSAVI map with minimal atmospheric effects by selecting, on a pixel-by-pixel basis, the highest MSAVI value as output to the composited product. Maximum MSAVI values select the least cloud and atmospheric contaminated pixels (CitationHuete et al. 1999). Variable composite periods have been tested to obtain cloud-free vegetation index data. The minimum compositing period is limited by cloud cover frequency. According to the recommendation of CitationHuete et al. (1999) the compositing period should be set to at least 5 days in higher latitude zones and because the study area is located at a high latitude (N51°) and in a semi-arid region, we set the compositing period at 6 days.
Predicted total plant biomass was obtained after fitting the data of total plant biomass to a hyperbola equation using a non-linear regression model (SPSS, Chicago, USA, 1998):
The harvest indices, the ratio of grain yield to total plant biomass, of wheat and barley were 0.326 (N = 209, R2 = 0.67) and 0.429 (N = 24, R2 = 0.77), respectively. CitationSinclair (1998) reviewed this index and recorded wheat and barley indices of 0.37 and 0.48, respectively. Our result was lower than his reviewed result because our total plant biomass included both crop and weed biomass. The carbon contents of wheat and barley residues were 0.396 (N = 209, R2 = 0.95) and 0.412 (N = 24, R2 = 0.98), respectively. And, these coefficients were used to calculate the carbon input as plant residues.
Table 2 Parameters of the predicted equations for total plant biomass
Table 3 Climatic data of the agricultural term from 2001 to 2005
Climatic conditions from 2001 to 2005
Climatic conditions of the agricultural term from 2001 to 2005 are shown in . Air temperature over the active growing season (May to July) in 2002 was clearly below the average. On the contrary, air temperature of the growing season in 2004 was above the average. In the active growing season, precipitation in 2002 and 2005 was obviously above the average. Precipitation in the previous winter to spring (October to April) in 2002 was also above the average. On the contrary, precipitation in the active growing season in 2004 was well below the average. Hence, 2002 and 2004 were defined as wet and drought years, respectively.
RESULTS AND DISCUSSION
Soil water content on seeding time
shows the correlation coefficient between total plant biomass and soil water content in relation to seeding time. There was a significant positive relationship between soil water content and plant biomass in 2004. Soil water content also showed a slightly positive relationship with plant biomass for 2001 and 2002. Our results, therefore, probably illustrate a positive correlation between soil water content and plant biomass. In addition, CitationFunakawa et al. (2004b) reported that soil water content at seeding time was strongly correlated with plant biomass (N = 9, r = 0.83) in the region. Thus, to evaluate spatial and temporal patterns in the SOC budget, it is important to understand the spatial and temporal variation of soil water content.
Stepwise multiple linear regression (P < 0.15) was used to estimate soil water content up to 90 cm depth at each seeding time using the following parameters: CTI, SLOPE, PROFC, PLANC, ELEV, slope aspect (converted to sine and cosine forms), total amount of precipitation (November to March) and land use of the previous year. The Barayev Institute recorded precipitation. The land use of the previous year was expressed as 1 or 0; plots were set to 1 if summer fallow had taken place the previous year, otherwise 0.
Residuals of the multiple linear regression were calculated using actual data and estimated data by each plot and by each year. Simple kriging was applied to the residuals. Then, the spatially distributed regression result and the kriged residuals were summed to estimate the target variable (SW). This method is known as simple kriging with varying local means (SKlm) (CitationGoovaerts 1997), and good performance of this method was reported by CitationGoovaerts (2000), CitationLopez-Granados et al. (2002) and CitationTakata et al. (2007b).
Table 4 Correlation coefficients between plant biomass and soil water content at seeding time
Figure 4 The distribution map of soil water content (0-90 cm) made by simple kriging with varying local mean method, the parenthetic values indicate the total precipitation (mm) during November to March.
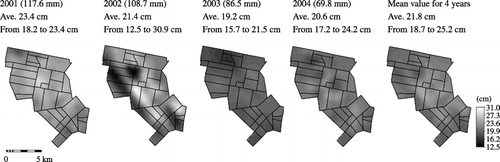
The distribution map of soil water content delineated by SKlm is shown in . There was higher than average soil water content in 2001 and 2002 than in 2003 and 2004 because there was more precipitation during the winter season of 2001 and 2002 than 2003 and 2004 (). Soil water content was highest on the plateau, followed by the north-facing then south-facing slopes, except for post-fallow fields in 2002. This might result from drifting snow being caught on the plateau (CitationYanai et al. 2005) and more sunshine and, hence, higher evaporation on the south-facing slope.
Potentially mineralizable carbon map
First, to estimate the spatial patterns of CO2 emission, spatial prediction of PMC was accomplished by the application of ordinary kriging (CitationYanai et al. 2005). The distribution map of PMC predicted in 2001 and 2003 is shown in . The PMC in 2001 and 2003 had average values of 2.6 and 2.5 Mg C ha−1, and ranged from 0.7 to 5.9 Mg C ha−1 and from 1.4 to 5.1 Mg C ha−1, respectively, indicating that the spatial patterns of PMC were similar, and the potential to act as a source of CO2 was highest in the plateau, followed by the north-facing and then the south-facing slopes in both years. CitationYanai et al. (2005) reported that geographical characteristics were correlated with snow depth, moisture content and plant productivity in the area. A high plant productivity area could be a high carbon input area, hence, the high elevation area might be a high PMC area.
Figure 6 Carbon input as plant residues and carbon output as CO2 emission from 2001 to 2005 and 5 years results. The upper figures show distribution patterns of annual carbon input as plant residues from 2001 to 2005, and right figure shows 5 years carbon input. The lower figures show distribution patterns of annual carbon output as CO2 emission from 2000 to 2005, and right figure shows 5 years carbon output.
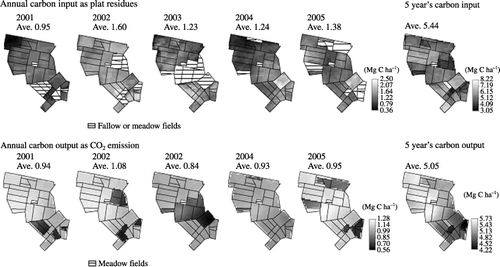
Spatial and temporal variation of carbon input as plant residues
As shown in , annual carbon input as plant residues for cereal and abandoned fields ranged from 0.95 (2001) to 1.60 (2002) Mg C ha−1 year−1, and carbon input in the wet year (2002) exceeded that of the drought year (2004). Carbon input as plant residues was highest in 2002 because of favorable soil water conditions at seeding time () and heavy precipitation during the winter and growing seasons in 2002 (). High carbon input was found in post-fallow fields characterized by high soil water content at seeding time (). The distribution patterns of carbon input as plant residues were similar to those of soil water content except for 2003. In addition, barley fields seemed to have lower carbon input than wheat fields. As the variety of the barley seeded in the study area has a short stem length as well as drought tolerance, carbon input as barley residues could be lower than that of wheat residues.
Spatial and temporal variation in annual CO2 emission
Annual carbon output as CO2 emission is shown in . The average annual CO2 emission of cereal fields (wheat and barley) and of abandoned fields ranged from 1.00 (2004) to 1.17 (2005) Mg C ha−1 year−1, and CO2 emission in the wet year (2002) was greater than that in the drought year (2004). It is well known that CO2 emission under dry conditions is lower than under wet conditions (CitationCarlyle and Bathan 1988; CitationHoward and Howard 1993; CitationJia et al., 2007; CitationMariko et al. 2007; CitationOrchard and Cook 1983). In general, annual CO2 emission of cereal fields showed a similar pattern to PMC and was higher on north-facing than south-facing slopes and highest on the central plateau. Average annual CO2 emission of fallow fields ranged from 0.76 (2002) to 0.89 (2004) Mg C ha−1 year−1, showing a different trend from the cereal fields. It is noteworthy that fallow management was applied on high PMC fields in 2004, and average annual CO2 emission from fallow fields was highest even under dry conditions. Compared to fallow fields, cereal fields emitted more CO2. CitationRochette et al. (1992) found higher CO2 emission for fallow fields than for barley fields, but this was because of the lower soil moisture of barley fields during summer. Fields under fallow were mechanically harrowed several times to keep the land bare and to minimize evapotranspiration during the cropping season to reserve soil water content in deeper horizons. On the contrary, there was lower surface soil water content for fallow fields than for cereal fields at the study site (CitationTakata et al. 2007a). CitationJia et al. (2007) reported that soil respiration in the semi-arid region is mainly affected by surface soil water content, followed by subsurface soil water content and soil temperature. Furthermore, lower CO2 emission for fallow fields could result primarily from the lower surface soil water content for fallow fields. In addition, in fallow fields CO2 emission was highest on the plateau and higher on the north-facing than south-facing slopes. This spatial pattern was similar to that of the PMC content. The pattern of CO2 emission depended on land use and topography, and basal CO2 emission might be determined by precipitation in summer time.
Spatial and temporal variation in the carbon budget
To calculate the annual carbon budget of fallow fields, annual carbon input as weed biomass of fallow fields was set to 0.02 Mg C ha−1 year−1 (CitationTakata et al. 2007a). The annual carbon budget of all fields was then calculated by subtracting the annual CO2 emission from the annual carbon input. The average annual carbon budget in this study's fields ranged from –0.06 (2001) to 0.28 (2002) Mg C ha−1 year−1, and the 5-year carbon budget was 0.27 Mg C ha−1 year−1 (). It is noteworthy that this study site contributed to carbon sequestration, which conflicts with data from the CitationWorld Bank (2003) that reported 14% humus loss in the surface soil of arable land during 1960–1995 in this region, that is, approximately 0.4 Mg ha−1 year−1 of carbon lost over 35 years. It seems that our carbon budget estimation failed to consider the occasional practice of plant residue burning in the study area. A preliminary study showed 77% of carbon was lost by residue burning. And, interviews with farm managers clarified that crop residues were burnt in 20% of the studied fields. Plant residue burning has agronomic advantages linked to soil management and disease control. The advantages of not burning, especially the long-term increase in soil organic matter, coverage of surface soil and its promotion of soil aggregation, have been reported in detail elsewhere (CitationCrovetto 1996). The advantage of plant residues, such as carbon sequestration and surface soil protection, should therefore receive more attention. The map of the annual carbon budget shows the existence of negative budget zones; all fallow fields and some cereal fields showing low carbon input or high CO2 emission were included in these zones. Except for 2002, most barley fields had a negative budget, whereas conversely, almost all post-fallow fields had a positive carbon budget. The highest carbon budget was found in the abandoned fields in 2004. The abandoned field was covered with weed and spontaneous wheat, oat and millet. Plant biomass of the abandoned field was the same as the adjacent cereal field. However, non-harvested in the abandoned field caused higher carbon input than in the cereal field. Therefore, the carbon budget of the abandoned field was the highest in the study area. A similarity between the carbon budget map and the carbon input map was clear. The correlation coefficients between carbon budget and carbon input as plant residues from 2001 to 2005 ranged from 0.95 to 0.99, and between carbon budget and annual CO2 emission from –0.19 to 0.70. This result indicates that the annual carbon budget strongly depended on carbon input as plant residues at this site.
Influence of the crop rotation system on the carbon budget
The 5-year carbon budgets for the six crop rotation systems listed in ranged from –0.49 (F) to 1.61 (O) Mg C ha−1, and the fallow-based and barley-based systems were depletive. The W-5-yr system sequestrated considerable amounts of carbon into the soil. The B-5-yr system, in contrast, even when excluding fallow, had a negative carbon budget. Carbon input as plant residues tended to be lower in barley fields than in wheat fields; thus, the B-5-yr and B-4-yr systems had negative carbon budgets. Most of the F fields and 43% of the W-4-yr fields had negative carbon budgets. In addition, the negative carbon budget sites of W-4-yr were mainly located on the high PMC zones. In contrast, only 3% of the W-5-yr fields had a negative carbon budget.
Table 5 Five-year carbon budget of the crop rotation systems
The average 5-year carbon budget for the entire study area was 0.27 Mg C ha−1 year−1, and 2104 Mg of carbon was sequestrated. The conversion of a wheat-fallow system to continuous wheat without burning of plant residues sequestrated between 0.22 (W-4-yr to W-5-yr) and 0.32 (F to W-5-yr) Mg ha−1 year−1 of carbon into the soil. This estimated value is the same as that recorded in the Canadian prairie (CitationDumanski et al. 1998) and the world database of 67 long-term agricultural experiments (CitationWest and Post 2002).
Optimization of the crop rotation system from an environmental viewpoint
This study discussed the SOC budget with some uncertainty because of the low predictive ability of the regression equations; however, summer fallow management without concern for the soil environment resulted in a remarkable decrease in SOC at the study site. Therefore, to optimize the regional crop rotation system to mitigate the depletion of soil organic matter, summer fallow management should be reconsidered. Fallow-based crop rotation systems were mainly distributed on the south-facing slope. In addition, most of the negative carbon budget zones of W-4-yr were distributed on the central plateau, which had the highest PMC zones (). The frequency of fallow management should be decreased or alternative management in these zones should replace fallow management. CitationFunakawa et al. (2004b) suggested that snow management is a promising approach for capturing water in the study area rather than summer fallow practice. Snow management applies drifting-snow trap technology by making parallel snow rows at certain intervals. However, in poorly productive zones, where snow management would not be profitable for the carbon budget, meadow management should be introduced (CitationTakata et al. 2007a) into a new crop rotation system. The barley-based crop rotation system is mainly distributed on the north-facing slope, which has relatively high PMC zones (). The negative carbon budget in this area resulted from low carbon input and relatively high carbon output as CO2 emission. Thus, we recommend the frequency of barley planting be reduced in the high PMC zones.
Total plant biomass showed a slightly positive correlation with soil water content at seeding time (), and soil water content was higher in post-fallow fields than in other fields. Moreover, the 5-year average cereal yield of W-4-yr was higher than that for W-5-yr, and was also higher in B-4-yr than in B-5-yr (). Given these results, alternative management, including re-allocation of the crop rotation system and/or the introduction of new technology to maintain cereal productivity, should also take soil water conservation into consideration. As CitationYanai et al. (2005) reported, the distribution pattern of snow depth depends on the topographical characteristics, and the benefit of snow management might, therefore, largely depend on the landscape. Furthermore, snow management requires some investment, including fuel. It is very important to determine which strategy, changing the crop rotation system or utilizing new technology, is more effective to avoid SOC depletion while maintaining cereal productivity. Therefore, future studies should investigate the optimization of the crop rotation system and the use of new technology for this agro-landscape using SOC budget and economic data. In conclusion, optimization of the regional crop rotation system based on the spatial and temporal variation of the SOC budget appears to be a suitable option to limit organic matter depletion and promote sustainable agriculture.
REFERENCES
- Bausch , WC . 1993 . Soil background effects on reflectance based crop coefficients for corn . Remote Sens. Environ , 46 : 1 – 10 .
- Carlyle , JC and Bathan , U . 1988 . Abiotic controls of soil respiration beneath an eighteen-year old Pinus radiatastand in southeastern Australia . J. Ecology , 76 : 654 – 662 .
- Colwell , JE . 1974 . Vegetation canopy reflectance . Remote Sens. Environ , 3 : 175 – 183 .
- Crovetto , C . 1996 . Stubble Over the Soil: the Vital Role of Plant Residue and Soil Management to Improve Soil Quality , Madison : American Society of Agronomy .
- Deering , DW . 1978 . Rangeland reflectance characteristics measured by aircraft and spacecraft sensors(PhD Dissertation) , College Station, Texas, , USA : Texas A and M University .
- Dumanski , J , Desjardins , RL Tarnocai , C . 1998 . Possibilities for future carbon sequestration in Canadian agriculture in relation to land use changes . Clim. Change , 40 : 81 – 103 .
- Food Agriculture Organization . 2005 . Food and Agriculture Indicators , Rome : Food and Agriculture Organization .
- Food Agriculture Organization, International Soil Reference and Information Centre (ISRIC), International Union of Soil Sciences (IUSS) . 1998 . World Reference Base for Soil Resources Rome
- Funakawa , S , Nakamura , I , Akshalov , K and Kosaki , T . 2004a . Soil organic matter dynamics under grain farming in Northern Kazakhstan . Soil Sci. Plant Nutr , 50 : 1211 – 1218 .
- Funakawa , S , Nakamura , I , Akshalov , K and Kosaki , T . 2004b . Water dynamics in soil–plant systems under grain farming in Northern Kazakhstan . Soil Sci. Plant Nutr , 50 : 1219 – 1227 .
- Goovaerts , P . 1997 . Geostatistics for Natural Resource Evaluation , New York : Oxford University Press .
- Goovaerts , P . 2000 . Geostatistics in soil science: state-of-the-art and perspectives . Geoderma , 89 : 1 – 45 .
- Goward , SN , Markham , B , Dye , DG , Dulaney , W and Yang , J . 1991 . Normalized difference vegetation index measurements from the Advanced Very High Resolution radiometer . Remote Sens. Environ , 35 : 257 – 277 .
- Howard , DM and Howard , PJA . 1993 . Relationships between CO2evolution, moisture content and temperature for range of soil types . Soil Biol. Biochem , 25 : 1537 – 1546 .
- Huete , AR . 1988 . A soil adjusted vegetation index (SAVI) . Remote Sens. Environ , 25 : 295 – 309 .
- Huete , AR , Hua , G , Qi , J , Chehbouni , A and van Leeuwen , WJDG . 1992 . Normalization of multidirectional red and NIR reflectance with the SAVI . Remote Sens. Environ , 40 : 1 – 20 .
- Huete , AR , Justice , C and van Leewen , W . 1999 . MODIS Vegetation Index (MOD13) Algorithm Theoretical Basis Document version 3 , Tucson : University of Arizona .
- Inoue , Y and Olioso , A . 2006 . Estimating the dynamics of ecosystem CO2flux and biomass production in agricultural fields on the basis of synergy between process models and remotely sensed signatures . J. Geophys. Res-Atoms , 111 : S24 – 91 .
- Jackson , RD and Pinter , PJ Jr . 1986 . Spectral response of architecturally different wheat canopies . Remote Sens. Environ , 20 : 43 – 56 .
- Jia , BR , Zhou , GS and Yuan , WP . 2007 . Modeling and coupling of soil respiration and soil water content in fenced Leymus chinensis steppe, Inner Mongolia . Ecol. Model , 201 : 157 – 162 .
- Justice , CO , Townshend , JRG , Holben , BN and Tucker , CJ . 1985 . Analysis of phenology of global vegetation using meteorological satellite data . Int. J. Remote Sens , 6 : 1271 – 1318 .
- Karbozova-Saljnikov , E , Funakawa , S , Akhmetov , K and Kosaki , T . 2004 . Soil organic matter status of Chernozem soil in North Kazakhstan: effects of summer fallow . Soil Biol. Biochem , 36 : 1373 – 1381 .
- Labus , MP , Nielsen , GA , Lawrence , RL and Engel , R . 2002 . Wheat yield estimates using multi-temporal NDVI satellite imagery . Int. J. Remote Sens , 23 : 4169 – 4180 .
- Lal , R . 2004 . Carbon sequestration in soils of central Asia . Land Degrad. Develop , 15 : 563 – 572 .
- Lal , R and Bruce , JP . 1999 . The potential of world cropland soils to sequester C and mitigate the greenhouse effect . Environ. Sci. Policy , 2 : 177 – 185 .
- Lopez-Granados , F , Jurado-Exposito , M , Atenciano , S , Garcia-Ferrer , A , Orden , MS and Garcia-Torres , L . 2002 . Spatial variability of agricultural soil parameters in southern Spain . Plant Soil , 246 : 97 – 105 .
- Main management of geology and cartography, USSR (GUGK) . 1982 . Atlas Kazakhskoi SSR Vol. TOM 1 , Russia (in Russian)
- Mariko , S , Urano , T and Asanuma , J . 2007 . Effects of irrigation on CO2and CH4fluxes from Mongolian steppe soil . J. Hydrol , 333 : 118 – 123 .
- Moore , ID , Gessler , PE , Nielsen , GA and Peterson , GA . 1993 . Soil attribute prediction using terrain analysis . Soil Sci. Soc. Am. J , 57 : 443 – 452 .
- Nicholson , SE and Farrar , TJ . 1994 . The influence of soil type on the relationships between NDVI, rainfall, and soil moisture in semiarid Botswana. NDVI response to rainfall . Remote Sens. Environ , 50 : 107 – 120 .
- Orchard , VA and Cook , FJ . 1983 . Relationship between soil respiration and soil moisture . Soil Biol. Biochem , 15 : 447 – 453 .
- Qi , J , Chehbouni , A , Huete , AR and Kerr , YH . 1994 . Modified soil adjusted vegetation index . Remote Sens. Environ , 48 : 119 – 126 .
- Qi , J , Huete , AR , Moran , MS , Chehbouni , A and Jackson , RD . 1993 . Interpretation of vegetation indices derived from multi-temporal SPOT images . Remote Sens. Environ , 43 : 1 – 10 .
- Rochette , P , Desjardins , RL , Gregorich , EG , Pattey , E and Lessard , R . 1992 . Soil respiration in barley (Hordeum vulgare L.) and fallow fields . Can. J. Soil Sci , 72 : 591 – 603 .
- Running , SW , Justice , C and Salomonson , V . 1994 . Terrestrial remote sensing science and algorithms planned for EOS/MODIS . Int. J. Remote Sens , 6 : 1271 – 1318 .
- Sinclair , TR . 1998 . Historical changes in harvest index and crop nitrogen accumulation . Crop Sci , 38 : 638 – 643 .
- Soil Survey Staff . 2003 . Keys to Soil Taxonomy , 9th edn , Washington : USDA Natural Resources Conservation Service .
- Takata , Y , Funakawa , S , Akshalov , K , Ishida , N and Kosaki , T . 2007a . Influence of land use on the dynamics of soil organic carbon in northern Kazakhstan . Soil Sci. Plant Nutr , 53 : 162 – 172 .
- Takata , Y , Funakawa , S , Akshalov , K , Ishida , N and Kosaki , T . 2007b . Spatial prediction of soil organic matter in northern Kazakhstan based on topographic and vegetation information . Soil Sci. Plant Nutr , 53 : 289 – 299 .
- Tucker , CJ and Sellers , PJ . 1986 . Satellite remote sensing of primary productivity . Int. J. Remote Sens , 7 : 1395 – 1416 .
- Wan , Z . 2006 . MODIS Land Surface Temperature Products Users’ Guide , Santa Barbara : Institute for Computational Earth System Science (ICESS), University of California .
- West , TO and Post , WM . 2002 . Soil carbon sequestration by tillage and crop rotation: a global data analysis . Soil Sci. Soc. Am. J , 66 : 1930 – 1946 .
- World Bank . 2003 . Kazakhstan drylands management project Project Proposal Document 25929-KZ
- Yanai , J , Mishima , A , Funakawa , S , Akshalov , K and Kosaki , T . 2005 . Spatial variability of organic matter dynamics in semi-arid croplands in northern Kazakhstan . Soil Sci. Plant Nutr , 51 : 261 – 269 .