Abstract
This paper summarizes the characteristics of regional-scale nitrogen (N) flow models. The regional scale is generally considered to be an area that ranges from more than 10 km2 to the size of a continent. Parameterization is the key process in creating a regional-scale model. During parameterization, transfer functions that reflect the controlling factors must be created at the target scale because the influence of different factors will change with the size of the scale. Watersheds are the most useful unit for evaluating overall N discharge; however, regional activity data is most often available for municipal units. Thus, municipal units must be reaggregated into watershed units. A longer time period is desirable to normalize seasonal and annual variations at regional scales. Parameters that influence N flow must match the investigated spatial and temporal scales. Given the need to use a range of parameters that vary in terms of the quality of the data, models exhibit inevitable uncertainties. Quantification of the uncertainties and verification of the estimated results are required. Error propagation, the Monte Carlo simulation method and maximum and minimum values have been used to obtain different threshold values of uncertainty. To verify regional-scale N flow models, the following five approaches have been used or proposed: (1) calibration of the model by detailed monitoring at multiple sites, (2) verification of the most important process of the extrapolation mechanisms, (3) verification of the N budget, paying particular attention to water quality, (4) comparison with the results quantified by different models, (5) comparison with aerial or satellite image analysis. As regional-scale modeling of N flow will become more important in the future, it is important to develop models than can accurately estimate N dynamics at this scale.
INTRODUCTION
Environmental problems, such as acid rain, global warming and river water pollution, are found not only at the local source area where the pollutant is emitted, but regionally and even globally. The substrates causing such environmental damage travel from the point of emission, and their form and amount can change during this movement. Therefore, understanding the flow of pollutants at a regional to global scale is important.
Although most environmental pollutants stem largely from industrial activities, nitrogen (N) is primarily influenced by agricultural activities. Nitrogen is an essential nutrient for plants, and its application to crops has significantly increased yields in the past century (CitationSmil 2000). Radiatively, chemically or biologically active N is referred to as reactive N (CitationInternational Nitrogen Initiative 2004). In the absence of human activities, biological N fixation is the primary source of reactive N, providing approximately 90–130 Tg N year−1 on land (CitationGalloway et al. 1995). Human activities result in the fixation of an additional 150 Tg N year−1, as a result of energy production (21 Tg N year−1), fertilizer production (78 Tg N year−1), the cultivation of legumes and rice (43 Tg N year−1), and other activities (CitationGalloway 1998).
There is a growing need to estimate regional-scale N movements. Therefore, it is necessary to understand the characteristics that are unique to this scale. The objective of this paper is to analyze the characteristics of regional-scale N movements to improve the monitoring and modeling of these movements. Several types of regional-scale N movement models are discussed and necessary future improvements in the estimation of regional-scale N movements are summarized.
WHAT IS A REGIONAL SCALE?
Although it is difficult to define a regional scale precisely, it is generally considered to be an area that ranges from more than 10 km2 to the size of a continent. CitationHoosbeck and Bouma (1998) presented a schematic diagram in which the scale progresses from the polypedon/farm level to the catena/farm, watershed/country and global levels (). Any study of N in the agroecosystem can be placed within this scheme. Given the difficulties in distinguishing quantitative and qualitative descriptions, CitationSeyfried (2003) used grid size as the x-axis in this scheme. Although the order from farm to country does not apply everywhere, a regional scale can be considered to be larger than a watershed or country scale. There is also the possibility that the catena/farm level might be included as a regional scale depending on its size.
As the targeted area increases, different processes are included. shows the N flow in a larger system in which the plant system has been added to the soil system. Immobilization and mineralization are usually not taken into account in regional-scale N movement models. At the regional scale, there are various types of vegetation and soils, which can be considered as modules of the whole system. The aggregation of these modules builds the whole system. It is also possible to assume that the system shown in represents a farmland subsystem of a larger system that includes livestock, human beings and forest subsystems (). Each subsystem can be divided into greater levels of detail. Although a variety of flows exist in such a complex system, it is possible to divide these flows into four types: N input flow into the system (e.g. chemical fertilizer, atmospheric deposition and biological N fixation), N cycling flow inside the system (e.g. self-supplied feed and food, manure and crop residue), N loss flow from the system (e.g. leaching, surface runoff, ammonia volatilization, denitrification, nitrification products and N disposed of), and N export flow from the system (e.g. crop and livestock products and wood) (CitationKimura and Hatano 2007).
To estimate the nutrient cycling that occurs at a regional scale, grids or raster files containing information on weather, land use, soil and administrative units are required. Information related to human activity is referred to as activity data. In a regional-scale model, all these data are converted into nutrient units. Any study in which N cycling is estimated using coefficients can be referred to as a model, even if it is not defined as such in the study. Mathematical expressions relating various soil characteristics to one another or to N flows are referred to as transfer functions (CitationBouma and van Lanen 1987). Transfer functions might, for example, relate nitrous oxide (N2O) emissions to soil characteristics such as total N or water content. In this case, soil total N and water content are the controlling factors or parameters for the N2O emission rate. As these factors gradually change, both spatially and temporally, they are referred to as continuous transfer functions. Another type of transfer function is represented by the class function, in which a fixed value is defined for a given land use or soil type. Inventory data obtained from the literature are a type of class function and are useful for getting a quick overview of a system. Inventory data have the disadvantage of not usually being obtained from the targeted area; thus, these data do not reflect local environmental conditions. Creating a transfer function that is specific to the targeted mechanism or nutrient flow is referred to as parameterization, and all models are dependent to some extent on this parameterization procedure.
An example of one of the easiest calculations of regional-scale N flow is the soil surface N balance, which is an environmental impact index used by the Organisation for Economic Co-operation and Development (CitationOrganisation for Economic Co-Operation and Development 2001). In this N balance method, the input data are the quantities of chemical fertilizer, compost manure, biological N fixation, atmospheric deposition, organic fertilizer and seeds. Product exports are subtracted from the sum of these inputs to calculate the soil surface N balance. Other flows, as shown in , are not considered in this method. Data concerning the area of land use, production, amount of applied fertilizer, livestock numbers, population and other characteristics are collected from agricultural census data from the Food and Agriculture Organization of the United Nations (FAOSTAT), statistical records of each country and agricultural census data for each country. The flow of nutrients is calculated by multiplying this statistical data by inventory data of N concentration, discharge coefficients and the amount of excreta per person and per head of livestock or poultry. A comparison of the 1985–1987 and 1995–1997 data clearly showed differences in soil surface N balance for each country (CitationOrganisation for Economic Co-Operation and Development 2001). For example, the Netherlands had the highest value with 262 kg N ha−1 in 1995–1997, although this amount was 52 kg N ha−1 lower than its 1985–1987 value (). Although this approach might appear to be too simple, other estimations incorporating more detailed flows have also shown that the Netherlands has a higher N load than other countries (CitationDe Vries et al. 2003).
CitationGreen et al. (2004) rearranged statistical data for each country into a grid of 30′ cells and compared, for the pre-industrial period and the mid-1990s, the amount of N that was at risk of entering into aquifers. The technique was similar to the method used by the CitationOrganisation for Economic Co-Operation and Development (2001). Averaging the amount of chemical fertilizer and the population and livestock numbers inside a country reduced the accuracy of the regional distribution, resulting in an overestimation in densely populated countries and an underestimation in sparsely populated countries. Despite this problem, characteristics can be compared among regions using such estimations. There was no region where the N content entering into the aquifers exceeded 2 Mg N km−2 before the industrial revolution. In contrast, several regions exceeded 50 Mg N km−2, particularly in North America, Europe and South Asia, by the mid-1990s.
Figure 4 Surface N balance in Organisation for Economic Co-Operation and Development countries for 1995/1997 and 1985/1987 (Organisation for Economic Co-Operation and Development 2001).
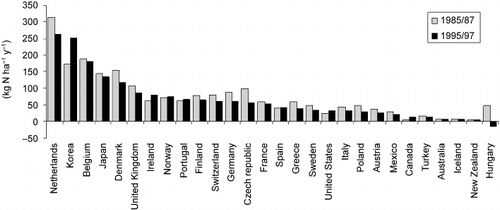
Models using inventory data and statistical records have several disadvantages, including the following: change within a year cannot be reflected because the estimated result is a mean annual value; the estimated values for locations for which no inventory data exist are quite uncertain; and the range of fluctuation is not properly estimated because the inventory data are only used as average values without information on standard deviations. However, using inventory data and statistical records in modeling does enable an easy comparison of differences in N management methods, which vary greatly according to country, region and era.
CHANGE IN THE INFLUENCING FACTORS ACCORDING TO SCALE
To examine a system more thoroughly, factors controlling the targeted flow must be analyzed and transfer functions must be created. However, the factors that influence nutrient flows will differ if the targeted scale is changed. For example, CitationLark et al. (2004) determined the spatial dependency of the factors influencing N2O emissions using wavelet analysis, and found that N2O emissions depended on the dissolved organic carbon content and moisture in the soil at a small scale (4–8 m), whereas they depended on pH, soil moisture and nitrate-N content at larger scales (64–256 m) (; CitationLark et al. 2004).
In the case of surface runoff, the controlling factor at the micro scale is the change in soil structure as a result of rainfall. At the next larger scale, the controlling factor is the formation of rills, and at the scale above that, it is the intensity of rainfall and the absence or presence of vegetation (CitationKirkby et al. 1996). CitationKirkby et al. (1996) proposed connecting models designed for different temporal and spatial scales to reflect the influencing factor at the different scales. Although there are several examples of such models for surface runoff from river banks (CitationCouper 2004), there are fewer models that connect different scales for N movement. Many studies have been carried out on a comparatively small scale, such as soil column experiments, to precisely understand the N cycle. Although this type of experiment is indispensable for understanding the overall N flow, calibrated models at small scales cannot necessarily be used to provide predictions at regional scales. If the influencing factors of the model can easily be obtained at the regional scale and the impact of the composition factor changes linearly when the model is scaled up, the scale for which the model was designed will not be a problem (CitationSeyfried 2003). However, the relative influence of different factors often changes non-linearly with scale, and spatial variations in the influencing factor reduce the quality of the results. Therefore, if the data used for developing a model do not accurately reflect the spatial variation on a regional scale, the model cannot accurately represent the actual process, no matter how precisely the model reflects the process for the column.
Figure 5 Wavelet correlation analysis of nitrous oxide emission and soil properties (Lark et al. 2004). Error bars indicate 95% confidence intervals. SOC, extractable soil organic carbon; WFPS, water-filled pore spaces.
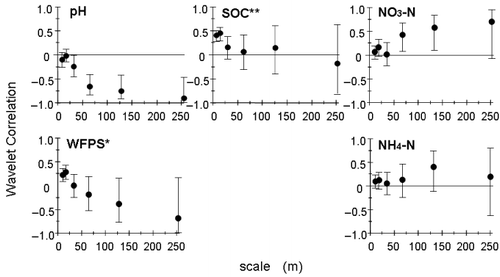
CitationMulla et al. (2003) presented a case study in which the result, estimated using a simple empirical formula, was closer to the actual N discharge from rivers than that of an estimate derived from aggregating minute process models. Conversely, CitationMeissner et al. (1999) conducted a study at a location with very uniform soil and land use, and reported that the N leaching process model, for which calibration was carried out using a lysimeter, was able to estimate similar amounts of N leaching into groundwater for the field and watershed levels. To create a regional-scale model, a transfer function that reflects the controlling factors at the targeted scale is necessary. The key to successfully applying a dynamic model to a regional scale is a good understanding of the available factors at a regional scale and an accurate understanding of their spatial distribution.
PARAMETERIZATION FOR A SIMPLE MODEL
Ideally, parameters used for a regional-scale model should be extracted by means of a sensitivity analysis of a detailed process model run at a regional scale to determine which factor has the most influence on the targeted flow (CitationDe Vries et al. 1998). The parameters required to describe the process should then be reduced on the basis of the sensitivity analysis. Then aggregation of the processes and input data based on the desired temporal resolution should follow. Simplification based on a sensitivity analysis is often impossible because regional soil and environmental data are incomplete, or they are only available at a resolution that is unacceptable for the targeted scale. To date, there have been no examples showing systematic parameterization for N flow at a regional scale, but parameterization is usually conducted with all available data for the targeted area. For example, CitationDel Grosso et al. (2005) estimated greenhouse gas emissions from farmlands for the entire US. Based on monitoring data obtained in the US, these researchers created transfer functions that considered climatic conditions, soils and fertilizer management and estimated a value for N2O emissions that was 41% lower than the value obtained using the emission factor of the Intergovernmental Panel on Climate Change (CitationIntergovernmental Panel on Climate Change 1996), and the amount of indirect emissions was 80% higher. The Intergovernmental Panel on Climate Change emission factor represents the ratio of N2O emissions to the amount of fertilizer applied; thus, the influence of climate and soil are ignored. CitationDel Grosso et al. (2005) showed that differences in global warming potential among the regions were mainly influenced by differences in crop variety. Their results indicated that the use of crop-specific emission factors (tier 2; CitationIntergovernmental Panel on Climate Change 2006) yields more precise estimates of N2O emissions compared with using the CitationIntergovernmental Panel on Climate Change (1996) uniform emission factor for all crops.
Figure 6 Total amount of N discharged to river water as a percentage of surplus N in the watershed (Jansons et al. 2003; Jordan et al. 1997). Different symbols represent different sub-watersheds. %, proportion of total N discharge to surplus N.
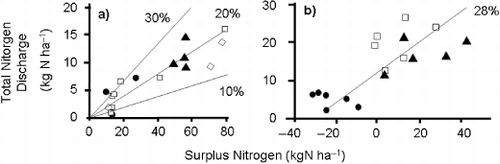
Attention should also be paid to the uncertainty of the estimated results. CitationFreibauer (2003) estimated the emission of N2O, carbon dioxide and methane from farmland in Europe for the year 2001 using data obtained in long-term monitoring experiments. These researchers expressed uncertainty as the coefficient of variation, which is the ratio of the standard deviation to the appropriate average (CitationIntergovernmental Panel on Climate Change 2006). As CitationFreibauer's (2003) model reflected soil and climate conditions and used a database that had a smaller uncertainty than that of the CitationIntergovernmental Panel on Climate Change (1996), the uncertainty of the estimates was reduced by half. CitationKimura et al. (2007) also estimated N2O emissions from farmlands in a watershed based on monitoring data collected in the same watershed. Spatial and temporal variations were averaged by parameterization of the monitored emissions at the targeted scale. The estimated uncertainty was very large owing to the use of monitoring data from different spatial and temporal scales. In addition, the estimated uncertainty did not distinguish between variations depending on input data (e.g. the amount of applied fertilizer) and on errors (e.g. spatial variation in measurement and error in parameterization). It has been noted that uncertainty in a regional-scale model mainly results from uncertainty concerning soil information (CitationLi et al. 2004). A distinction between the uncertainty resulting from actual variability in management methods and that resulting from spatial variability is required to reduce the uncertainty of the estimation.
Parameterization is highly empirical because it is markedly dependent on measured data and is bound to the time and area for which it was composed or parameterized. Elaborating a model for a specific region increases the accuracy of the estimated N movement in the targeted region, but it also makes the model more site specific and often more complicated. There has been a plethora of research in recent years concerning the application of existing models to different regions, and it is very likely that highly versatile models on a regional scale will be generated in the future.
STRENGTH OF THE WATERSHED SCALE
Water movement boundaries provide unique opportunities in regional-scale N movement studies. The quality of river water is influenced by non-uniform conditions inside the watershed, and there is a correlation between river-water quality and land use in a watershed (CitationArbuckle and Downing 2001; CitationJordan et al. 1997; CitationTabuchi 1995; CitationWoli et al. 2002). A transfer function can be created using a linear regression between the N concentration in river water and the proportion of land use. Thus, the proportion is an indicator for the N management method within the system. The slope of the regression line varies according to the difference in the amount of N flow variables inside a system (CitationWoli et al. 2004). The amount of N discharged and the net N load resulting from anthropological N input is also positively correlated (CitationHowarth et al. 1996). However, only a small proportion of N is discharged into surface water. Various studies have reported that the ratio of the total amount of N discharged into the river water to surplus N in the watershed ranges from 10 to 30% (; CitationJansons et al. 2003; CitationJordan et al. 1997) and that denitrification, plant uptake and retention in the system account for the remaining 70–90% of the total N input. The river N concentration level and the amount of N discharged are the result of all the N flows in the watershed; thus, they are good indicators of N movement throughout the whole watershed system.
To explain the process inside a system, CitationSmith et al. (1997) used water-quality variables as indicators and developed a discharge estimation model called SPARROW (spatially referenced regressions on watershed attributes), which relates the increased amount of N discharge among the monitoring points of the Mississippi River to land use and features of the river channels. This model uses the slope of the watershed, the water density in the watershed and the flow velocity of the river channel for the regression analysis. According to the model, more than 90% of the total N input into the Mississippi River from the nearest point of a deep river channel with fast river velocity was discharged to the sea, regardless of the distance from the river outlet (CitationAlexander et al. 2000). Other studies have been conducted using parameters based on the assumption that the watershed can be considered to be one uniform system. The ReNuMa (regional nutrient management) model (CitationHong and Swaney 2007) was developed using the “generalized watershed loading functions” established by CitationHaith and Shoemaker (1987). This model has one coefficient for each flow, such as N flux in surface runoff, dry and wet deposition, N flux in groundwater, and denitrification for the whole watershed. Transfer functions are created specifically for the watershed using monitoring data. Testing of these watershed models under different soil, climate and land use conditions is required to improve the models.
A watershed is not only an area where water gathers, but it can also be considered to be a natural geological unit dividing the land into sub-watersheds. Surface runoff and leaching are the major flows in N losses from agroecosystems. Those flows are strongly related to water movement, which can be described and measured in watershed units. The different approaches described above show the strength of using the watershed unit as a boundary for N movement studies. However, a drawback of using watershed units is that statistical data are given in municipal units, which are sometimes not equal to watershed units. A reaggregation of municipal units into watershed units is necessary, as conducted by CitationKohyama et al. (2003).
CONSIDERATION OF TIME
When the targeted area is on a regional scale, a longer observation period is required. CitationGroffman and Tiedje (1989) showed that there was a stronger correlation of the soil environmental factor with cumulative annual N2O flux than with the individual measured values. CitationMu et al. (2005) reported a similar result for the soil environmental factor and the amount of N2O emission from seven fields. The annual N2O emission was influenced by the amount of fertilizer applied and the estimated amount of mineralized N, whereas hourly N2O flux was influenced by various soil parameters in each field. In a subsequent study, CitationMu et al. (2008) found similar results for CO2 flux. The duration and frequency of the measurements also changes the amount of N2O accumulated. A longer monitoring time has been shown to increase the accumulated amount of N2O (CitationBouwman et al. 2002). A period of more than 120 days was required for a database study on annual N2O emissions from farmlands in Japan (CitationAkiyama et al. 2006). A higher measurement frequency reduces the risk of over-estimation resulting from extremely high values and under-estimation resulting from missing peak events (CitationKronvang and Bruhn 1996). To evaluate N dynamics at a regional scale, the timespan must be long. At the same time, attention must be paid to the sampling methods used to obtain the original data when comparing data with different sampling durations and frequencies.
Seasonal and annual differences can both be normalized as the observation period increases. The monitoring results of one season or one year are strongly influenced by the conditions specific to that season or year, whereas a longer timespan integrates seasonal and annual deviations. This is illustrated by the relationship between surplus N and the amount of leached nitrate-N, shown in , where an individual year might show a weak or year-specific relationship, but the cumulative amount can show a clear tendency. In a study by CitationShepherd and Bhogal (1998; ), the first year of the experiment showed only a small amount of leached N, whereas the third year showed a higher amount of leached N compared with the surplus N. Annual differences were also found by CitationRasse et al. (1999; ) and CitationLiu et al. (2003; ). However, in all three cases, the cumulative values over the whole study period showed a consistent relationship between surplus N and leached nitrate-N. On a regional scale, the spatial extension leads to time lags among different places within the target area. Thus, comparing cumulative amounts provides more useful information to explain the region than comparing site-specific and temporal-specific information. Thus, investigations and evaluations using a timespan that matches the targeted spatial and temporal scale are required (CitationCouper 2004).
FUTURE TASKS FOR REGIONAL-SCALE NITROGEN FLOWS MODELS
Quantification of uncertainty is an important task in regional-scale N flow models. Uncertainties can be introduced at any stage of the model preparation, and the level of uncertainty can be influenced by many factors, including data collection and analytical techniques, the accuracy and validity of the data that is used, the selected explanatory factors and transfer formulas, and computational complexity. The experiments required for preparing a model can include incubation of soils in the laboratory and collection of samples in the field. The results are restricted by the investigated system or applied treatment. Sampling frequency, collection methods, sample preparation methods for analysis and analytical methods all influence the results used to create the model. Although it is known that spatial variation in a field is large and that the representativeness of the collected samples is problematic (CitationBrown 1993; CitationYanai et al. 2000), it is less well known that the information obtained also varies greatly as a result of the methods of sample preparation and analysis. In particular, the influence of soil structure is often not considered. Although the method of sampling does not contribute to the uncertainty of the modeling process itself, it has a great influence on creating and verifying a model. The uncertainty of a regional-scale model increases because it must often use information obtained through different sampling and preparation methods for the parameterization.
Figure 7 Relationship between surplus N and leached NO3-N for annual and total amounts of N. (a) Shepherd and Bhogal (1998), (b) Rasse et al. (1999) and (c) Liu et al. (2003).
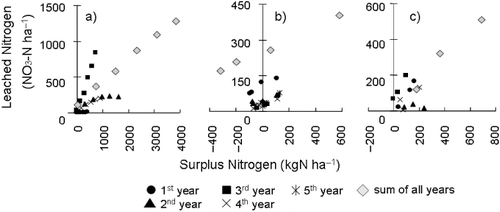
Errors can also be associated with the data used for the model factors, such as information on meteorological conditions, land use, soil type and fertilizer management. For example, when a historical land-use map is used, the accuracy and validity of the map compared with the targeted year's actual land use can be a problem. Mapping errors exist regardless of whether the land-cover analysis was obtained from remote sensing or from ground exploration. The accepted standard for land-use classification accuracy is 80% (CitationSabins 1997). Therefore, it is very important to quantitatively handle the uncertainty of the land-use map used, as well as any uncertainties in the distribution of meteorological conditions (e.g. precipitation and temperature) and soil types. In addition to uncertainties in the information on land use, there can be uncertainty about actual management practices and the range of actual management methods. Although the uncertainty in land-use and soil-type maps can be reduced by more accurate methods of investigation, uncertainties resulting from management practices cannot be reduced because the value reflects all of the actual practices and should, therefore, be considered to be the range rather than the uncertainty. There is a need to distinguish between these two types of uncertainties (CitationKimura et al. 2007).
The results of a model are also determined by elements such as the flow considered, the selected explanatory factors and the formula used to create the transfer function. In a study where six specialists each created a leaching model of agricultural chemicals based on the same measured values, the estimated values of the leaching risk differed tremendously depending on the selected explanatory factors and the type of transfer function used (CitationBeulke et al. 2006). CitationCsendes et al. (2007) also pointed out that the fitted coefficient value of an existing formula might depend on the original coefficient value when the coefficient of an existing model is fitted. Therefore, appropriate methods for the fitting of the coefficient value are required.
In addition, when a model becomes complex, errors in computation can occur. For example, CitationCalvetti (1991) found a rounding error in which very small decimal numbers were not correctly displayed by the binary notation. A program that deals with this error was developed, and its utility has been pointed out (CitationCalvetti 1991). This problem should be kept in mind if the operating model becomes complex. Several models have been used to quantify the uncertainty of regional-scale N models, and the application of error-propagation equations (CitationIntergovernmental Panel on Climate Change 2006), Monte Carlo simulations (CitationDe Vries et al. 2003; CitationKimura et al. 2007) and maximum and minimum values (CitationTonitto et al. 2007) have been implemented to obtain different threshold values of uncertainty. Although a quantitative evaluation of uncertainty is required, there are mathematical problems with each of these methods, and there are ongoing discussions about how to address these issues.
Table 1 Verification methods of the regional models
A fatal defect of the regional-scale models described in the present review is that it is difficult, and sometimes impossible, to verify their results. The following five approaches have been used in previous studies to address this problem (): (1) calibration of the model by detailed monitoring at multiple sites, followed by a comparison with the results of verified models at other sites, (2) verification of the most important process in the extrapolation mechanisms, (3) verification of the N budget with particular attention to water quality, (4) comparison of the results obtained from different models, (5) comparison of the results with aerial photographs or satellite images.
Calibration of the model by detailed monitoring at more than one site (method 1) is generally used to verify a model that has been developed at a plot scale. Estimation for a regional scale is possible by assuming that the model can be successfully generalized for the whole region. This method is useful in areas with detailed information on soil properties and land-use characteristics.
Verification of the most important process of the extrapolation mechanisms (method 2) was suggested by CitationWagenet (1998). In the case of functional models based on statistical relationships, or more mechanistic models that must be simplified, testing basic assumptions of the models or extrapolations can be quite useful. For example, CitationGroffman et al. (1992) studied the annual denitrification flux at a regional scale and used denitrification enzyme activity as a proxy for actual annual denitrification N loss to validate the extrapolation of data from individual experimental sites to the entire region. The estimated denitrification potential by the landscape model showed a strong positive correlation with the potential measured by denitrification enzyme activity. The patterns of denitrification influenced by soil texture and drainage observed at the landscape scale held up at the regional scale and CitationGroffman et al. (1992) concluded that extrapolation of the data from the experimental sites was justified.
Verification of the N budget with particular attention to the water quality (method 3) is the most direct method to verify the estimated results at a regional scale. It is especially suitable for the verification of a model targeted to a regional scale because it includes the non-uniform system conditions of N management within a farm field and watershed. (CitationRandall and Gross 2001). Several studies have examined groundwater, drainage and surface-water quality and compared the monitored N concentrations in groundwater and the calculated farmland surplus N (CitationGreen et al. 2004; CitationHowarth et al. 1996; CitationJansons et al. 2003; CitationJordan et al. 1997; CitationNishio 2003).
Results obtained from different types of models (method 4) have also been compared in an attempt to verify the certainty of the result. This method is referred to as a “multi-model ensemble”, and examples where this technique has been applied include atmospheric deposition (CitationDentener et al. 2006), atmospheric nitrogen dioxide concentration (Citationvan Noije et al. 2006), N2O and nitric oxide emission (CitationReiners et al. 2002), river-water quality (CitationMulla et al. 2003) and soil carbon flux (CitationEaster et al. 2007). One disadvantage of this approach is that it requires a large amount of work for proper parameterization because expert knowledge is required for the structure of the model and the influence of input data on the result (CitationEaster et al. 2007).
Although there are no examples directly relating N cycling to aerial photographs or satellite images (method 5), several methods have been proposed to estimate regional variability by integrating model results and image analysis. Examples include predicting the status of land surface moisture content using microwave images of each watershed and soil physical characteristics (CitationTischler et al. 2007), and a crop growth model that used 10-day averages of the normalized difference vegetation index (CitationMo et al. 2005; CitationMoriondo et al. 2007).
CONCLUDING REMARKS
Although a number of models intended for various scales exist, a perfect model including all N flow does not. Issues that require future elaboration include matching monitoring results with the targeted scale, targeting N flow, and determining whether a region-specific or a highly versatile model is necessary. Different approaches should be connected to each other, as depicted by the research chain diagram shown in .
Linkage of N models to C models is expected to contribute greatly to the estimation of N cycling in the future. A C cycle model for regional scales has been developed in recent years. Monitoring of CO2 fluxes by flux towers using eddy covariance measurements, referred to as FLUXNET (CitationFiend et al. 2007), has been established for several ecosystems around the world. Models based on FLUXNET are typically at a half-degree grid at the global scale and are based on calculations of the net flux of C and its dependence on environmental conditions, and these models usually do not describe processes occurring on the surface or inside the soil. However, N is also associated with C fixation by plants, and the decomposition of plant materials leads to the release of N. Advances in monitoring methods for estimating C flux will contribute to more precise estimations of N flows. In contrast, linkage to N flows might contribute to the development of C flow models owing to the more detailed descriptions of movements in the soil. Regional-scale modeling of N flow will become more important in the future, and the development of models that match the unique characteristics of a regional scale is highly desirable.
ACKNOWLEDGMENTS
This study was supported in part by the Strategic International Cooperative Program “Comparative Study of Nitrogen Cycling and Its Impact on Water Quality in Agricultural Watersheds in Japan and China” by the Japan Science and Technology Agency.
REFERENCES
- Akiyama , H , Yan , X and Yagi , K . 2006 . Estimations of emission factors for fertilizer-induced direct N2O emissions from agricultural soils in Japan: Summary of available data . Soil Sci. Plant Nutr , 52 : 774 – 787 .
- Alexander , RB , Smith , RA and Schwarz , GE . 2000 . Effects of stream channel size on the delivery of nitrogen to the Gulf of Mexico . Nature , 403 : 758 – 761 .
- Arbuckle , KE and Downing , JA . 2001 . The influence of watershed land use on lake N:P in a predominantly agricultural landscape . Limnol. Oceanograph , 46 : 970 – 975 .
- Beulke , S , Brown , CD Dubus , IG . 2006 . User subjectivity in Monte Carlo modeling of pesticide exposure . Environ. Toxicol. Chem , 25 : 2227 – 2236 .
- Bouma , J and van Lanen , HAJ . Transfer functions and threshold values: from soil characteristics to land qualities . Workshop on quantification land evaluation process, Vol 6 International Institute for Aerosphere Survey and Earth Sciences . Edited by: Beek , KJ . pp. 106 – 110 . Madison, Wisconsin, , The Netherlands : International Union of Soil Sciences/Soil Science Society of America . ISS/SSSA
- Bouwman , AF , Boumans , LJM and Batjes , NH . 2002 . Emissions of N2O and NO from fertilized fields: Summary of available measurement data . Global Biogeochem. Cycles , 16 ( 4 ) : 1058 http://www.agu.org/pubs/crossref/2002/2001GB001811.shtml
- Brown , AJ . 1993 . A review of soil sampling for chemical analysis . Aust. J. Exp. Agr , 33 : 983 – 1006 .
- Calvetti , D . 1991 . Roundoff error for floating point representation of real data communications in statistics . Theory Methods , 20 : 2687 – 2695 .
- Couper , PR . 2004 . Space and time in river bank erosion research: A review . Area , 36 : 387 – 403 .
- Csendes , T , Banhelyi , B and Hatvani , L . 2007 . Towards a computer-assisted proof for chaos in a forced damped pendulum equation . J. Comput. Appl. Math , 199 : 378 – 383 .
- De Vries , W , Kros , J , Oenema , O and de Klein , J . 2003 . Uncertainties in the fate of nitrogen II: A quantitative assessment of the uncertainties in major nitrogen fluxes in the Netherlands . Nutr. Cycl. Agroecosyst , 66 : 71 – 102 .
- De Vries , W , Kros , J , van der Salm , C , Groenenberg , JE and Reinds , GJ . 1998 . The use of upscaling procedures in the application of soil acidification models at different spatial scales . Nutr. Cycl. Agroecosys , 50 : 223 – 236 .
- Del Grosso , SJ , Mosier , AR , Parton , WJ and Ojima , DS . 2005 . DAYCENT model analysis of past and contemporary soil N2O and net greenhouse gas flux for major crops in the USA . Soil Tillage Res , 83 : 9 – 24 .
- Dentener , F , Drevet , J Lamarque , JF . 2006 . Nitrogen and sulfur deposition on regional and global scales: a multimodel evaluation . Global Biogeochem. Cyc , 20 : 4003
- Easter , M , Paustian , K Killian , K . 2007 . The GEFSOC soil carbon modelling system: A tool for conducting regional-scale soil carbon inventories and assessing the impacts of land use change on soil carbon . Agric. Ecosys. Environ , 122 : 13 – 25 .
- Fiend , AD , Arneth , A Kiang , NY . 2007 . FLUXNET and modelling the global carbon cycle . Global Change Biol , 13 : 610 – 633 .
- Freibauer , A . 2003 . Regionalised inventory of biogenic greenhouse gas emissions from European agriculture . Eur. J. Agron , 19 : 135 – 160 .
- Galloway , JN . 1998 . The global nitrogen cycle: changes and consequences . Environ. Pollut , 102 : 15 – 26 .
- Galloway , JN , Schlesinger , WH , Levy , IH , Michaels , A and Schnoor , JL . 1995 . Nitrogen fixation: Anthropogenic enhancement–environmental response . Global Biogeochem. Cycl , 9 : 235 – 252 .
- Green , PA , Vörösmarty , CJ , Meybeck , M , Galloway , JN , Peterson , BJ and Bozer , EW . 2004 . Pre-industrial and contemporary fluxes of nitrogen through rivers: A global assessment based on typology . Biogeochem , 68 : 71 – 105 .
- Groffman , PM and Tiedje , JM . 1989 . Denitrification in north temperate forest soils: Relationships between denitrification and environmental factors at the landscape scale . Soil. Biol. Biochem , 21 : 621 – 626 .
- Groffman , PM , Tiedje , JM , Mokma , DL and Simkins , S . 1992 . Regional scale estimates of denitrification in north temperate forest soils . Landscape Ecol , 7 : 45 – 53 .
- Haith , DA and Shoemaker , LL . 1987 . Generalized watershed loading functions for stream flow nutrients . Water Res. Bull , 23 : 471 – 478 .
- HongB SwaneyDP2007Regional Nutrient Management Model Version 1.0User's ManualAvailable from URL: http://www.eeb.cornell.edu/biogeo/nanc/usda/renuma.htm (http://www.eeb.cornell.edu/biogeo/nanc/usda/renuma.htm)
- Hoosbeck , MR and Bouma , J . 1998 . Obtaining soil and land quality indicators using research chains and geostatistical methods . Nutr. Cycl. Agroecosyst , 50 : 35 – 50 .
- Howarth , RW , Billen , G Swaney , DP . 1996 . Riverine inputs of nitrogen to the North Atlantic Ocean: fluxes and human influences . Biogeochem , 35 : 75 – 139 .
- Intergovernmental Panel on Climate Change . Revised 1996 Guidelines for National Greenhouse Gas Inventories: Chapter 4. Agriculture . IPCC Guidelines for National Greenhouse Gas Inventories Workbook . Edited by: IPCC/OECD/IEA . Volume 2 , pp. 1 – 140 . Geneva : Intergovernmental Panel on Climate Change .
- International Panel on Climate Change2006IPCC Guidelines for National Greenhouse Gas Inventories. Volume 4 Agriculture, Forestry and Other Land UseAvailable from URL: http://www.ipcc-nggip.iges.or.jp/public/2006gl/vol4.htm (http://www.ipcc-nggip.iges.or.jp/public/2006gl/vol4.htm)
- Jansons , V , Busmanis , P , Dzalbe , I and Kirsteina , D . 2003 . Catchment and drainage field nitrogen balances and nitrogen loss in three agriculturally influenced Latvian watersheds . Eur. J. Agron , 20 : 73 – 179 .
- Jordan , TE , Corell , DL and Weller , DE . 1997 . Effect of agriculture on discharges of nutrients from coastal plain watersheds of Chesapeake Bay . J. Environ. Qual , 26 : 836 – 848 .
- Kimura , SD and Hatano , R . 2006 . [Estimation and evaluation of nitrogen cycle at regional scale – Present and future] . Jap. J. Soil Sci. Plant Nutr , 77 : 351 – 359 . (in Japanese)
- Kimura , SD and Hatano , R . 2007 . An Eco-Balance approach to evaluate the historical change in N loads caused by agricultural land use change at a regional scale . Agric. Sys , 94 : 165 – 176 .
- Kimura , SD , Mu , Z-J , Toma , Y and Hatano , R . 2007 . An eco-balance analysis of global warming potential, farmland surplus nitrogen and yield of six agricultural land uses in the Ikushunbetsu Watershed . Soil Sci. Plant Nutr , 53 : 373 – 386 .
- Kirkby , MJ , Imeson , AC , Bergkamp , G and Cammeraat , LH . 1996 . Scaling up processes and models from the field plot to watershed and regional areas . J. Soil Water Conserv , 51 : 391 – 396 .
- Kohyama , K , Hojito , M , Sasaki , H and Miyaji , H . 2003 . [Generation of agricultural statistics mesh data using digital national land information.] . Jpn. J. Soil Sci. Plant Nutr , 74 : 415 – 424 . (in Japanese)
- Kronvang , B and Bruhn , AJ . 1996 . Choice of sampling strategy and estimation method for calculating nitrogen and phosphorus transport in small lowland streams . Hydro. Process , 10 : 1483 – 1501 .
- Lark , RM , Milne , AE , Addiscott , TM , Goulding , KWT , Webster , CP and O’Flaherty , S . 2004 . Scale- and location-dependent correlation of nitrous oxide emissions with soil properties: An analysis using wavelets . Eur. J. Soil Sci , 55 : 611 – 627 .
- Li , CS , Mosier , A Wassmann , R . 2004 . Modeling greenhouse gas emissions from rice-based production systems: Sensitivity and upscaling . Global Biochem. Cycl , 18 : 1043
- Liu , X , Ju , X , Zhang , F , Pan , J and Christie , P . 2003 . Nitrogen dynamics and budgets in a winter wheat–maize cropping system in the North China Plain . Field Crops Res , 83 : 111 – 124 .
- Meissner , R , Seeger , J and Rupp , H . 1999 . Estimating the effects of set-aside on water quality: scaling-up of lysimeter studies . Land Deg. Develop , 10 : 13 – 20 .
- Mo , X , Liu , S , Lin , Z , Xu , Y , Xiang , Y and McVicar , TR . 2005 . Prediction of crop yield, water consumption and water use efficiency with a SVAT-crop growth model using remotely sensed data on the North China Plain . Ecol. Model , 183 : 301 – 322 .
- Moriondo , M , Maselli , F and Bindi , M . 2007 . A simple model of regional wheat yield based on NDVI data . Eur. J. Agron , 26 : 266 – 274 .
- Mu , Z-J , Kimura , SD and Hatano , R . Contributions of individual greenhouse gases from upland cropping systems in Central Hokkaido to the net global warming potentials . The 3rd International Nitrogen Conference Nanjing . October 2004 , China. Edited by: Zhu , Z-L , Minami , K and Xing , G-X . pp. 707 – 714 . Beijing : Science Press USA . Proceedings 2005
- Mu , Z-J , Kimura , SD , Toma , Y and Hatano , R . 2008 . Evaluation of soil carbon sequestration under different upland cropping systems in central Hokkaido, Japan . Soil Sci. Plant Nutr , 54 : 650 – 661 .
- Mulla , DJ , Gowda , PH , Birr , AS and Dalzell , BJ . 2003 . “ Estimating nitrate-N losses at different spatial scales in agricultural watersheds ” . In Scaling Methods in Soil Physics , Edited by: Pachepsky , Y , Radcliff , DE and Selim , HM . 295 – 307 . Boca Raton : CRC Press .
- Nishio , D . 2003 . [Flow sheet of livestock excreta N and estimation of soil load primary unit based upon statistical data.] . Jap. Soil Sci. Plant Nutr , 74 : 721 – 730 . (in Japanese)
- Organisation for Economic Co-Operation and Development . 2001 . “ Environmental indicators for agriculture ” . In Methods and Results , Edited by: OECD . Vol 3 , 409 Paris : Organisation for Economic Co-Operation and Development .
- Randall , GW and Gross , MJ . 2001 . “ Nitrate losses to surface water through subsurface, tile drainage ” . In Nitrogen in the Environment: Source, Problems, and Management , Edited by: Follett , RF and Hatfield , JL . 95 – 122 . Amsterdam : Elsevier .
- Rasse , DP , Ritchie , JT , Peterson , WR , Loudon , TL and Martin , EC . 1999 . Nitrogen management impacts on yield and nitrate leaching in inbred maize systems . J. Environ. Qual , 28 : 1365 – 1371 .
- Reiners , WA , Liu , S , Gerow , KG , Keller , M and Schimel , DS . 2002 . Historical and future land use effects on N2O and NO emissions using an ensemble modeling approach: Costa Rica's Caribbean lowlands as an example . Global Biochem. Cycl , 16 : 1068
- Sabins , FF . 1997 . Remote Sensing: Principles and Interpretation , New York : W.H. Freeman and Company .
- Seyfried , MS . 2003 . “ Incorporation of remote sensing data in an upscaled soil water model ” . In Scaling Methods In Soil Physics , Edited by: Pachepsky , Y , Radcliff , DE and Selim , HM . 309 – 345 . Boca Raton : CRC Press .
- Shepherd , M and Bhogal , A . 1998 . Regular applications of poultry litter to a sandy arable soil: effects on nitrate leaching and nitrogen balance . J. Sci. Food Agric , 78 : 19 – 29 .
- Smil , V . 2000 . Feeding the World – a Challenge for the Twenty-first Century , Cambridge : The MIT Press .
- Smith , RA , Schwarz , GE and Alexander , RB . 1997 . Regional interpretation of water quality monitoring data . Water Resources Res , 33 : 2781 – 2798 .
- Tabuchi , T . 1995 . Nitrogen outflow model with nitrogen removal function by paddy fields - studies on nitrogen outflow analysis in agricultural watersheds (1) . Soil Physics , 78 : 11 – 18 .
- Tischler , M , Garcia , M Peter-Lidard , C . 2007 . A GIS framework for surface-layer soil moisture estimation combining satellite radar measurements and land surface modeling with soil physical property estimation . Environ. Model. Software , 22 : 891 – 898 .
- Tonitto , C , David , MB , Drinkwater , LE and Li , CS . 2007 . Application of the DNDC model to tile-drained Illinois agroecosystems: Model calibration, validation, and uncertainty analysis . Nutr. Cycl. Agroecosys , 78 : 51 – 63 .
- van Noije , TPC , Eskes , HJ Dentener , FJ . 2006 . Multi-model ensemble simulations of tropospheric NO2compared with GOME retrievals for the year 2000 . Atoms. Chem. Physics , 6 : 2943 – 2979 .
- Wagenet , RJ . 1998 . Scale issues in agroecological research chains . Nutr. Cycl. Agroecosys , 50 : 23 – 34 .
- Woli , KP , Nagumo , T and Hatano , R . 2002 . Evaluating impact of land use and N budgets on stream water quality in Hokkaido, Japan . Nutr. Cycl. Agroecosys , 63 : 175 – 184 .
- Woli , KP , Nagumo , T , Kuramochi , K and Hatano , R . 2004 . Evaluating river water quality through land use analysis and N budget approaches in livestock farming areas . Sci. Total Environ , 329 : 61 – 74 .
- Yanai , J , Ritadashi , N and Umeda , M . 2000 . [Analyzing the spatial variation in values of soil chemical properties in paddy fields by using geo-statistics.] . Jap. Soil Sci. Plant Nutr , 71 : 520 – 529 . (in Japanese)
- Butterbach-Bahl , K , Kesik , M , Miehle , P , Papen , H and Li , C . 2004 . Quantifying the regional source strength of N-trace gases across agricultural and forest ecosystems with process based models . Plant Soil , 260 : 311 – 329 .
- International Nitrogen Initiative . 2004 . “ Purpose: The Nitrogen Paradox ” . http://www.initrogen.com/
- Plant , RAJ . 2000 . Regional analysis of soil-atmospere nitrous oxide emissions in the Northern Atlantic Zone of Costa Rica . Global Change Biol , 6 : 629 – 653 .
- Rimski-Korsakov , H , Rubio , G and Lavado , RS . 2004 . Potential nitrate losses under different agricultural practices in the pampas region, Argentina . Agric. Water Management , b : 83 – 94 .
- Wendland , F , Bach , M and Kunkel , R . 1998 . The influence of nitrate reduction strategies on the temporal development of the nitrate pollution of soil and groundwater throughout Germany - a regionally differenced case study . Nutr. Cycl. Agroecosys , 50 : 167 – 179 .