Abstract
Immunotherapies are coming to the forefront as a treatment paradigm in cancer with multiple US FDA approvals in recent years and a better understanding of their therapeutic mode of action. The control of tumor growth by the immune system is orchestrated by a complex array of cellular interactions and molecular pathways, both in the immune cells as well as the tumor. Although research over the past three decades has elucidated many aspects of tumor immunosurveillance, given the inherent complexity of the immune cell phenotypes and function, high-throughput molecular profiling (‘omics’) approaches have now become essential to support the discovery and development of new therapies. Technologies, such as DNA and protein microarrays, deep sequencing, mass spectrometry, as well as the computational methods for their analyses, are advancing the contributions of systems biology towards the development and mechanistic understanding of cancer immunotherapies. In this review, the authors illustrate this through some recently reported studies.
Each platform can have a multitude of applications, some of which are described in the text.
Adapted with modifications from Citation[39].
![Figure 1. Biospecimens, molecules and omics platforms that can be used in the context of cancer immunotherapy studies.Each platform can have a multitude of applications, some of which are described in the text.Adapted with modifications from Citation[39].](/cms/asset/441ba3e0-d508-4d49-804b-3f2036aacf62/ierj_a_811814_f0001_b.jpg)
A number of platforms can be applied to study both tumor and peripheral samples. Since the omics platforms can have a high false discovery rate, integration of data across orthogonal platforms can enable rapid discovery of antigens that have a higher probability of validation in subsequent preclinical testing.
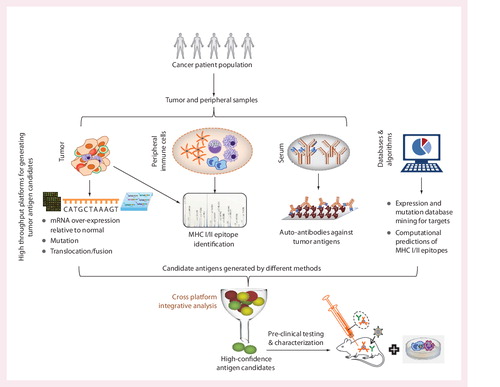
mRNA profiling may be used to assess the extent of tumor infiltration of lymphocytes. Protein or peptide microarrays can be used to assess the diversity of antibody (B cell) responses and next-generation sequencing (T-cell receptor sequencing) can be used to assess the diversity of T cell clones. Specific examples are provided in the text.
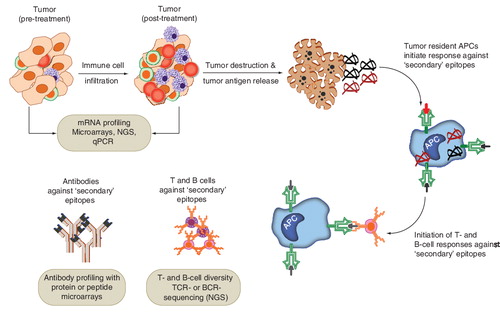
Systems biology is viewed as an approach that integrates and analyzes data from high-content omics platforms, using computational and statistical methods, to better characterize biological phenomena Citation[1–4]. Rather than probe a single element in depth, sometimes referred to as a ‘reductionist approach’, technologies such as microarrays, deep sequencing, high-throughput proteomics or metabolomics, aim to broadly study the state of the biological systems, from which hypotheses can be generated for further exploration. In cancer immunotherapy, the applications of systems biology have ranged from new target discovery, to the mechanistic understanding of therapies and the identification of biomarkers for patient stratification according to therapeutic response. Through known examples we will broadly cover these applications, after providing a brief overview of systems biology in drug development and recent advances in cancer immunotherapy. There are numerous examples of systems biology applications in cancer biology, basic immunology and infectious disease research. Therefore, where suitable, we will draw examples from these related areas as well.
Systems biology applications in drug discovery & development
Since DNA microarrays were developed about 20 years ago, omics profiling has been extensively used for addressing essential issues in drug development, such as, the elucidation of the biological pathways associated with disease development, demonstration of therapeutic mechanism of action (MoA), development of molecular classifiers for identifying subpopulations in disease and drug response and prediction of drug toxicity Citation[1–3,5–8]. Many other platforms and methods have been developed over the years that can be included in the broad spectrum of approaches for systems biology, such as mass spectrometry for proteomics and metabolomics, protein and antibody microarrays for monitoring antibody or protein levels, whole-genome single nucleotide polymorphisms (SNP) genotyping, and next-generation sequencing (NGS) for DNA and RNA analytics.
One potential benefit of the systems biology approaches is the ability to generate a more comprehensive understanding of biological pathways and their relationships with disease or drug response. This can enable data-driven decision making in many phases of drug discovery and development Citation[1,2,5–8]. Results from these approaches can facilitate the de-risking or re-prioritizing of therapeutic candidates in development in order to improve their probability of success in clinical testing. Regulatory agencies, such as the US FDA, also encourage the application of such approaches, as these may improve and streamline the assessments of safety and effectiveness of the therapies Citation[9].
To date, the applications of omics and systems biology have been most widespread in oncology, where characterizing the molecular complexity and heterogeneity of the tumor is critical not only for the development of targeted therapies, but also for the identification of responder patient populations for such treatments. Work in oncology provided the first successful examples of the utility of gene-expression profiling for disease subtyping Citation[10–12], disease prognosis Citation[13,14] and multi-gene diagnostic tests Citation[14–16]. Significant progress is also being made in the areas of autoimmune and infectious diseases, where systems biology is being used in characterizing disease pathogenesis, early disease detection, and understanding the MoA of vaccines and adjuvants Citation[17–21]. The use of systems biology approaches in the above areas has improved the trajectory of therapeutic development, and it has the potential to be useful in similar ways in the domain of cancer immunotherapy.
Below we discuss known applications of systems biology in cancer immunotherapy. For the purpose of this review, we segment the discussions into sections such as new antigen discovery, understanding therapeutic mechanisms of action, and clinical biomarker discovery. In reality however, these topics are intimately related. For example, better characterization of a therapeutic target and its mechanism can significantly inform the development of biomarkers of in vivo efficacy.
Recent advances in cancer immunotherapy
The engagement of the immune system to control tumor growth and the evasion of tumor destruction from the immune system has come to be recognized as a hallmark of cancer pathogenesis Citation[22–24]. Over the past 30 years, research and clinical development of cancer immunotherapies have resulted in a deeper understanding of the interplay between the immune system and tumor development Citation[23–28]. The importance of the immune system in controlling tumor growth is supported by several key clinical observations: (i) cancer incidence is increased in immunocompromised individuals Citation[29–31], (ii) increased infiltration of T lymphocytes into the tumor is predictive of survival Citation[32,33], and (iii) therapies that engage or activate the immune system, including cytokines, therapeutic vaccines, immune checkpoint inhibitors and monoclonal antibodies, have proven to be effective in treating cancers Citation[25,34,35].
Recent advances in the field have been rapid, and have led to the regulatory approval of two therapies by the FDA: (i) sipuleucel-T or Provenge® (Dendreon Corporation, WA, USA), an autologous cellular therapy, activated using an antigen cassette consisting of prostatic acid phosphatase (PAP, a prostate antigen) and granulocyte–macrophage colony-stimulating factor (GM-CSF), used for the treatment of asymptomatic or minimally symptomatic, hormone-refractory, metastatic prostate cancer Citation[35,36]; and (ii) ipilimumab or Yervoy® (Bristol–Myers Squibb, NY, USA), an immune modulating antibody directed against CTLA-4, a negative regulator of T-cell activation, for the treatment of metastatic melanoma Citation[34,37]. Immunotherapies currently undergoing clinical testing represent a complex array of approaches, but may be broadly classified into: (i) cellular therapies (such as autologous dendritic cells [DCs], antigen-presenting cells [APCs], adoptive T-cell therapies, or, genetically engineered T cells containing chimeric antigen receptors or CARs), (ii) cytokine and adjuvant therapies, (iii) immune-modulating antibodies such as those targeting immune checkpoints CTLA-4 or PD-1, and (iv) cancer vaccines containing tumor antigens (e.g., MAGE-A3, NY-ESO-1 and MUC-1) Citation[23,28,38]. Although tumor targeting monoclonal antibodies (e.g., rituximab or trastuzumab) constitute an important mode of cancer immunotherapy Citation[28], for the purpose of this review, we only cover those modalities (mentioned above) that directly target the immune system for its activity against the tumor, or involve tumor targeting by engineered immune cells. Given the promising results from ongoing clinical trials, it appears likely that several immunotherapies will gain regulatory approval for treating various cancers in the coming years.
Applications of systems biology in cancer immunotherapy
Various omics platforms can be used to interrogate biospecimens from the subjects under study (see and Citation[39]). Of these, mRNA expression profiling (transcriptomics) has been the most widely used and the resources for the interpretation of such data (statistical and bioinformatics methods, as well as databases) are well developed. However, other high-content platforms, including proteomics (mass spectrometry, protein and antibody microarrays Citation[40–42]) and NGS (nucleic acid sequencing and quantification) Citation[43,44] are also routinely used to investigate distinct aspects of discovery and development in cancer immunotherapy.
Discovery of targets for cancer immunotherapy
Therapeutic targets for cancer immunotherapy can be broadly classified into two categories. One class of targets is typically expressed on the cells of the immune system, and depending on the therapeutic objective, can be activated (as by cytokine or agonistic antibody) or repressed (as by a checkpoint inhibitor) to modulate the immune function for activity against the tumor. Antigen targets, by contrast, are expressed by the tumor cells, and can be used as immunogens to specifically direct the immune effector activity against a malignancy. While the requirements related to immunogenicity confer certain restrictions on antigen selection, the range of the tumor antigens for an immunotherapy may also be wider than those that can be targeted by small molecule or monoclonal antibody therapies. For example, targets such as transcription factors and intracellular proteins, may be targeted in an immunotherapy (e.g., Citation[45]), since the antigens targeted by the immune system do not need to be ‘druggable’ (as is usually required by the small molecule inhibitors) Citation[46] or expressed on the cell surface (as required by monoclonal antibody therapies). With the emphasis on the discovery of tumor antigens, we discuss below some of the primary considerations for selecting targets for immunotherapies, and how systems biology approaches are being used to discover such targets.
The selection of the suitable tumor antigen(s) is critical for antigen-targeting cancer immunotherapies Citation[47,48]. Ideally, these targets should possess a number of attributes, including tumor or tissue specific alterations (aberrant expression, mutation, splicing or post-translational modifications) and immunogenicity Citation[47]. Since off-target, immune responses may be potentially severe, long-term and difficult to control Citation[49], tumor or tissue specificity is a particularly important consideration in the selection of an antigen. Some antigens used in immunotherapies are mutated or over-expressed specifically in the tumor (such as immunotherapies targeting KRas Citation[50], p53 Citation[50] and Her2/neu Citation[51]). There are also immunotherapies that have targeted antigens which show restricted normal expression in a tissue that is considered non-essential (such as the testicular germ cells), but aberrant expression in certain tumors (viz. the cancer testis antigens) Citation[52]. Antigens that are tissue specific (such as, gp100 for melanocytes Citation[53], PAP Citation[35,36] or PSA Citation[54] for the prostate and CD19 for B cells Citation[55]) have also been used effectively as targets for treating malignancies originating from those tissues. As antigen targets should ideally have tumor or tissue specific alterations, the mining of the gene expression or mutation databases Citation[56–58] have contributed to the discovery of many tumor antigens over the years Citation[59–62]. The application of deep sequencing now has the potential to unravel tumor gene alterations at a resolution previously not possible Citation[43,62].
The efficacy of an immunotherapy relies upon enhancing the immune system’s ability to suppress tumor growth by the tumor-targeting lymphocytes. Therefore, immunogenicity is a critical consideration for selecting a tumor antigen. Immunity against a tumor antigen depends on the recognition of the peptides from the antigen presented to the lymphocytes by the MHC molecules on the target (tumor) cells, as well as the professional APCs. The repertoire and activity of lymphocytes targeting auto-antigens is generally limited by the immune system’s natural tendency to delete or suppress auto-reactive effector lymphocytes. Tumor-specific alterations may increase the immunogenicity of the antigens by expressing new epitopes that the immune system may not be tolerized to.
Immunogenicity can be assessed by the frequency with which the antigen peptides are presented by the MHCs and the frequency with which naturally occurring cellular or humoral responses to the antigen are observed. There are experimental as well as computational methods for assessing immunogenicity. These include high-throughput humoral profiling techniques for detecting antibodies, and the identification of MHC-restricted epitopes using computational predictions or mass spectrometry. Serological analysis of recombinant cDNA expression libraries (SEREX) Citation[63], has been used for over a decade to identify a host of well-known cancer testis antigens Citation[52], such as NY-ESO-1, and the MAGE, LAGE, GAGE families Citation[64,65]. SEREX involves the construction of cDNA expression libraries and protein expression followed by autologous serological screening for detecting reactivity of those proteins with antibodies present in the sera of the cancer subjects. While SEREX has been a productive approach, it is a relatively laborious technique and may not readily yield quantitative data. High-content protein microarrays provide an alternative approach for the identification of antibodies against tumor antigens Citation[61,66]. Antibodies against hundreds or thousands of self-antigens can be quantified rapidly using these microarrays. For identifying MHC-restricted epitopes, both computational Citation[67,68] and experimental Citation[69–72] methods are available. Computational prediction of MHC-restricted epitopes can be challenging because of their high degree of heterogeneity in length and sites of natural truncations. Therefore, mass spectrometry has often been used as an experimental alternative to identify and quantitate the peptide epitopes from MHC-peptide complexes Citation[69–73].
The application of high-throughput approaches, and in particular, the integrative analysis of data across platforms, can significantly facilitate the discovery and characterization of tumor antigens . For example, it is now possible to rapidly screen the sera from cancer patients using protein microarrays to identify tumor antigens with detectable serum antibodies Citation[61,66]. This information can be integrated with cancer gene expression or alteration data Citation[56–58,74] to identify tumor antigens with data supporting both their tumor alterations as well as immunogenicity. Since the omics platforms may produce hits with high false discovery rates, integration of information from orthogonal platforms can enable the identification of antigen candidates with a higher probability of success in follow-on validation studies. Walter et al. have pursued such an integrative approach for the discovery of tumor antigens in renal cell carcinoma Citation[75]. By combining tumor gene expression profiling with mass spectrometric identification of MHC-restricted peptides from tumor specimens, they identified a set of immunogenic antigen peptides. These peptides were then used to develop a vaccine, which is under clinical investigation. In a Phase II study of this vaccine, immune responses against these peptide epitopes were associated with improved overall survival Citation[75].
Tumor progression in patients can be frequently driven by multiple genetic events, evolving over the course of the disease. It may therefore be ideal to use multiple tumor antigens in an immunotherapy, and if possible, tailor the therapy for a patient with the specific tumor antigens that are altered in that patient Citation[50]. Recently, Castle et al. have explored how deep sequencing technologies can be used to survey the mutational profile of tumor antigens in individuals and how that profile may be used, in principle, to enable the formulation of individualized immunotherapies Citation[62,76].
In this section, we have discussed several important considerations for immunotherapy target selection and the omics methods for the identification of tumor antigens. The application of various omics platforms, followed by judicious integration of data, has the potential to significantly enhance the rate of discovery and prioritization of tumor antigens for future therapeutic design.
Understanding therapeutic MoA
The molecular pathways that are involved in the immune surveillance of a tumor and the tumor’s escape of the immune system are complex. Hypothesis driven investigation is often insufficient to obtain the necessary mechanistic understanding of the responses associated with an immunotherapy. Omics platforms can be particularly useful in such situations. In this section, we discuss applications of transcriptomics, proteomics and high-throughput sequencing for the characterization of MoA. We describe the applications of transcriptomics in detail and provide examples of a few unique applications of proteomics and high-throughput sequencing in the context of cancer immunotherapies.
Transcriptional profiling for understanding MoA
Since the DNA microarray has been an established platform for interrogating cellular pathways, it has been used in numerous studies for investigating MoA. Here we discuss some methods for interpreting transcriptomic data for elucidating MoA and provide examples of their applications in cancer immunotherapy. We also discuss related research in basic immunology for characterizing gene-sets(also referred to as signatures or modules) associated with immune cell lineages, signaling pathways, activation and differentiation (e.g., Citation[77–83]). These gene-sets are key to the interpretation of new transcriptomic data, especially from complex biological samples such as peripheral blood mononuclear cells (PBMCs) or whole blood.
One way to interpret a novel whole-genome gene-expression data-set is to analyze that data using information from known biological pathways or gene-sets derived from previous studies. This approach is often referred to as gene-set enrichment analysis Citation[84–86]. There are well-curated databases for gene-sets or signatures (e.g., MsigDB Citation[84], PAGED Citation[87] and GeneSigDB Citation[88]) and biological pathways (e.g., Gene Ontology Citation[89], KEGG Citation[90] and Reactome Citation[91]). In addition, there are repositories of raw transcriptomic data from which gene-sets can be derived (gene expression omnibus or GEO) Citation[92]. There are several statistical methods for determining enrichment Citation[85,86], which assess whether genes from a previously known gene-set or pathway are significantly over-represented amongst a list of genes identified from a new experiment that is being analyzed (e.g., genes that are perturbed in response to a given therapy). Despite its usefulness and widespread use, there are some limitations of gene-set based analyses for understanding MoA. For example, gene-sets are frequently incomplete and context (e.g., tissue or cell-type) dependent. Since the quality of the gene sets determine, in large part, the success of these approaches, incomplete or context-specific gene-sets can result in misleading inference about the mechanism. Also, the computational methods for performing enrichment analysis are still under active investigation Citation[85,86,93]. As progress is made towards better definition of the biological pathways and gene-sets, and improved computational methods for enrichment analyses, such methods can only become more useful for the interpretation of transcriptomic data from new studies.
We highlight a few examples of the application of gene expression profiling and data interpretation for the mechanistic characterization of cancer immunotherapies. The applications can be in multiple areas, including the characterization of the cell therapy products, understanding in vivo response, and identification of immune characteristics of subjects associated with clinical response to immunotherapy.
DC or APC therapies constitute an important modality for cellular immunotherapy Citation[94–96]. Detailed characterization of these cellular products presents a particular challenge in their development. Cellular products have an inherent complexity, and achieving consistency and uniformity in the preparation of such products require thorough quality control and understanding of a range of product parameters. Gene-expression profiling can be helpful in characterizing the activity and potency of such products. DCs for therapeutic applications are generated through culture with a wide range of activating factors (such as cytokines, adjuvants and so on). Depending on the precise nature of the cytokines or adjuvants used in their production, DCs may have different functional properties. Moschella et al. cultured DCs with IFN-α-2b and GM-CSF (to produce mature dendritic cells called ‘DCAs’), and GM-CSF and IL-4 (to produce ‘DC4’ cells), and compared the changes in the post-culture DCs using DNA microarrays Citation[97]. Comparison of mRNA profiles between DCA and DC4 revealed that the expression of genes involved in antigen presentation was increased in DCA relative to DC4 (such as TAP-2, HLA-DQ and HLA-DM). DCA also showed upregulation of TLRs, several TNF family ligands (e.g., TRAIL, TNFα and Fas), and inflammatory chemokine receptors (e.g., CCR2) for the migration of DCs to inflamed tissues. Consistent with expression profiling, functional assays showed that DCAs were more potent mediators of naive T-cell responses and tumor-cell cytotoxicity than DC4 Citation[97]. Similar work was done by Han and colleagues, where they compared the global gene expression profiles of immature DCs matured with lipopolysaccharide (LPS; a TLR4 agonist), IFNγ, IL-1β and TNF-α, to evaluate different clinical DC maturation protocols Citation[98]. Understanding the association of particular genes and pathways in the product with its clinical efficacy will help facilitate the development of reliable and effective production methods for cellular therapeutics as well as establish robust methods of quality control for such products.
Transcriptional profiling from complex and heterogeneous biological specimens such as whole blood and PBMCs, along with the related bioinformatics methods for data interpretation, can significantly enable the elucidation of the MoA associated with immunotherapeutic responses. Often in clinical trials, the availability of biological materials is limited. Therefore, a detailed phenotypic or functional characterization of the immune cells by conventional assays (such as multi-parameter flow-cytometry, cell proliferation or ELISpot) may not be feasible. In such cases, whole blood or PBMCs can be analyzed using transcriptional profiling (which requires a relatively small amount of the specimen) and the data interpreted with gene-sets Citation[19,20,99,100]. Significant progress has been made in recent years in characterizing the gene-sets that are associated with different immune-cell lineages Citation[83,101–103], cell signaling pathways Citation[82], cellular differentiation and activation (e.g., naive, memory and effector T cells Citation[80,83,104] or monocyte differentiation to macrophages and DCs Citation[105]). The computational methods for the analysis of complex samples, such as whole blood, are also under development Citation[99,106]. Taken together, these methods can significantly benefit the studies investigating in vivo MoA and clinical biomarkers from easily accessible peripheral samples such as whole blood and PBMCs.
Transcriptomic profiling of tumors is also providing insights into the in vivo MoA of cancer immunotherapies. Multiple cancer immunotherapy clinical studies are now showing evidence that increased lymphocyte infiltration within the tumor, reflected by a higher expression of lymphocyte markers, cytokines and chemokines, might be associated with better clinical outcome from the therapies Citation[107–109]. Pre-treatment gene-expression signatures (consisting of T-cell markers and cytokines) from melanoma and non-small-cell lung tumor were found to be associated with overall survival upon treatment with a MAGE-A3 vaccine in patients with tumors expressing the MAGE-A3 antigen Citation[107,110]. More recently, Ji et al. have arrived at similar a conclusion for ipilimumab (anti-CTLA-4 antibody) using gene-expression profiling of tumor biopsies collected from melanoma patients before and three weeks after the start of treatment in a Phase II clinical trial Citation[108]. Transcriptional profiling of pre-treatment tumors indicated that patients with high expression levels of immune related genes (T-cell activation, antigen presentation) were more likely to show clinical activity upon treatment with ipilimumab. In patients with clinical activity, ipilimumab treatment increased the expression of genes involved in immune responses (IFN-γ-inducible genes and Th1-associated markers) and decreased the expression of melanoma antigens and cell-proliferation genes. These post-treatment gene expression changes were associated with the lymphocyte infiltrate into the tumors. Thus, gene expression profiling is providing valuable mechanistic insights into the in vivo activity of the immunotherapies against the tumor.
Applications of proteomics in assessment of antigen spread or epitope spread
It is proposed that an effective cancer immunotherapy, which leads to the destruction of tumor tissue, may subsequently lead to priming of T or B lymphocytes against tumor antigens that are not contained in the therapy (secondary antigens). This phenomenon is often referred to as antigen spread, epitope spread or determinant spread Citation[111–113]. A broadened immune response against tumor antigens may promote more efficient killing of the tumor cells Citation[111]. Epitope spread has been observed in response to PSA-targeting immunotherapy Citation[114], CTLA-4 blockade in melanoma and prostate cancer Citation[113,115], Her2/neu vaccination for breast cancer Citation[116,117], and MUC1 DC vaccination in renal cancer patients Citation[118]. Data from early studies suggest that a broadened immune response may occur with a higher frequency in clinical responders compared with non-responders Citation[111,113,119].
Evaluation of humoral response against a diverse set of self-antigens can be explored rapidly using the high-content protein or peptide microarrays Citation[61,66,115,120]. A few studies have now reported the detection and validation of post-therapy antibody responses to tumor antigens using this technology Citation[115,120]. For example, Kwek et al. reported the discovery of PAK6 as a tumor antigen against which both humoral as well as CD4+ T-cell responses were observed post-CTLA-4 blockade in prostate cancer patients Citation[115]. The authors also found that immunization with PAK6 could be protective in mouse tumor models. It is expected that future clinical studies will apply such high-throughput technologies to study the breadth of the immune response to assess the therapeutic activity against the tumor in vivo.
Application of NGS in lymphocyte receptor sequencing & monitoring of T-cell clones
High-throughput NGS technologies Citation[43] have numerous applications in the domain of DNA and RNA analyses Citation[44,121–123]. Among these, one that is uniquely applicable to the study of immunotherapy is the sequencing and quantification of antigen receptors on T and B lymphocytes Citation[124–128]. Since the antigen receptor molecules from certain B cells are present in secreted form as antibodies, and there are high-throughput techniques available for identifying the specificities of antibodies (e.g., protein or peptide microarrays), the application of NGS has been particularly useful in the high-throughput assessment of the diversity of receptors on the T cells (which are present only on the cell surface). The T-cell receptor (TCR) genomic loci undergo somatic recombination, as well as addition or subtraction of nucleotides at the recombination junctions, to generate a wide array of T cells with specificities against a wide array of antigens Citation[125,126,128,129]. The potential diversity of T-cell repertoire has been estimated to be greater than 1014 Citation[129]. High-throughput DNA and RNA sequencing technologies now allow for a much deeper assessment of this diversity than previously possible Citation[124–128]. Although the techniques and analytical approaches are still emerging and being refined Citation[130,131], the applications are already addressing important issues in cancer diagnosis and immunotherapy development Citation[132–135]. We provide some examples of such applications in both preclinical as well as clinical studies.
The population of individual clonotypes is dynamic and capable of rapid expansion or contraction in response to immune stimulation or treatment with an immunotherapy. In cancer immunotherapies, sequencing of TCR (TCR-sequencing) can help to describe the dynamics of the T-cell repertoire before and after therapy and may aid in characterizing the efficacy of specific T-cell clones in vivo. Using such an approach, Chervin et al. identified the T-cell clones present in the tumors following adoptive T-cell therapy in mice Citation[135]. A library of TCRs with a range of binding properties to a model antigen, SIY, was introduced into T cells and adoptively transferred into a B16 melanoma mouse model containing SIY-positive tumors. High-throughput sequencing of the TCRs from tumor-infiltrating lymphocytes and lymphoid organs allowed them to determine the TCRs that were selected for in vivo. They observed that CD8+ T cells expressing the highest-affinity TCR variants were deleted in both the tumor-infiltrating lymphocyte population and in the peripheral lymphoid tissues. The same high-affinity TCR variants were however preferentially expressed by the intra-tumoral CD4+ T cells, suggesting that the CD4+ T cells played a role in antigen-specific tumor response. The results enabled the authors to characterize the clonotypes of the transduced TCRs that controlled the survival and tumor infiltration of the transferred T cells. Such applications can aid in the in vivo characterization of T cells and the development of adoptive T-cell therapies. Other applications have included the detection of minimal residual disease in leukemia/lymphoma patients Citation[132,133], and assessment of the T-cell repertoire after adoptive transfer of activated autologous T cells to enhance immunological function in neuroblastoma patients treated with high-dose cytotoxic therapy Citation[134].
Biomarkers of clinical outcome
Numerous profiling studies over the years have shown that cancer is highly complex at a molecular level, with significant variability within a given indication. Tumor gene-expression profiling has led to the identification of diagnostic markers, some of which are widely used in clinical practice Citation[14,15]. Increasingly, diagnostic markers are allowing more efficient and successful development of new therapeutics by enabling the identification of patients most likely to respond to a given therapy.
It has been noted that ideal biomarkers of clinical outcome should have some of the following properties: i) readily detectable in bodily fluids, ii) suitable for quantitative analysis, iii) associated with relevant clinical outcomes (e.g., overall survival), and iv) indicative of the in vivo mode of action Citation[136]. The development of biomarkers predictive of clinical outcome to cancer immunotherapies is still relatively new. However, omics technologies are contributing to the discovery and testing of such markers from preclinical and clinical studies. Recent studies across various immunotherapeutic modalities are starting to reveal some consistent themes. There is promise that such markers can be identified and translated into clinical practice. Below we discuss some early examples, with the focus on predictive (pre-treatment) and pharmacodynamic (post-treatment) markers of clinical outcome.
Predictive (pre-treatment) markers of clinical outcome
As mentioned earlier, pre-treatment tumor gene-expression profiles, indicative of host immune response to the tumor, were observed to be associated with clinical outcomes upon MAGE-A3 vaccine Citation[107,110] and ipilimumab Citation[108] treatments. Therapeutic outcome in subjects with specific gene-expression signatures are being evaluated as secondary endpoints in the current Phase III MAGE-A3 vaccine trials Citation[110]. Beyond tumor gene expression profiles, peripheral whole blood or PBMC profiles hold promise as potential sources of genomic signatures predictive of response to immunotherapies. Early efforts to develop gene expression signatures from PBMCs that are predictive of clinical outcome to a peptide vaccine for the treatment of advanced prostate cancer have been reported Citation[137]. Recently, multiple groups have also reported the discovery and validation of gene expression signatures from whole blood that are prognostic of overall survival in castrate-resistant prostate cancer Citation[138,139]. T-helper cell activation and signaling pathways (iCOS-iCOSL and CD28 signaling) were found to be associated with prognosis Citation[139]. Given such results, and their suggestion that immune status can predict disease course, it is conceivable that in the future studies, predictive expression signatures associated with clinical outcome to immunotherapy may be detected from whole blood or PBMCs. This would provide the tools for identifying interpretable RNA-based predictive biomarkers from easily accessible biological materials (peripheral rather than tumor samples).
SNPs and other DNA variations can be very useful as predictive markers. Relative to RNA, DNA variations are easier to assess since DNA is more stable as biological material and the number of variable states can be limited (as in the case of SNPs, somatic mutations or certain rearrangements). There are early examples where SNPs in candidate genes have been shown to be associated with response to immunotherapies Citation[140,141]. Genome-wide studies, associating SNPs to clinical efficacy of immunotherapies, are currently lacking. SNP platforms that are focused on genes with known immune functions may provide a cost-effective way to perform large-scale genotyping studies in immunotherapy trials Citation[142,143]. In addition, where available, integration of SNP data with other molecular data, such as gene expression, can significantly enhance the identification and annotation of functional SNPs associated with clinical outcomes Citation[144–146]. As larger clinical studies are completed and evaluated, we may see additional results of SNP associations with clinical efficacy as well as validation of the early results.
Pharmacodynamic (post-treatment) markers of clinical outcome
Robust and reliable pharmacodynamic markers of clinical efficacy is a critical need in cancer immunotherapy development Citation[136,147,148]. Clinical outcome to immunotherapy treatment is often not reflected accurately by the conventional imaging measures for solid tumors, such as the RECIST (Response Evaluation Criteria in Solid Tumors) or the WHO criteria Citation[149,150]. Tumor infiltration by active immune cells following therapy may be misleadingly diagnosed as tumor growth and disease progression by these above measures. In cases where immunotherapeutic intervention leads to prolonged survival without showing reduced tumor progression by the above measures, reliable early read-outs would provide useful guidance, both to the developers of novel therapies, as well as to the clinicans managing their use in patients.
Immune response monitoring in clinical studies has traditionally been performed using techniques such as ELISA for measuring antibody levels, multi-parameter flow cytometry for characterization of immune cell phenotypes, lymphocyte proliferation assays, ELISpot for quantitating antigen-specific cells and multiplexed cytokine/chemokine measurements Citation[148,151]. Where the therapy contains tumor antigen(s), a significant effort is usually focused on characterizing the B- or T-cell responses against the immunizing antigen(s) Citation[75,152,153]. In some cases, immune responses against the immunizing antigen(s) have been shown to be associated with clinical outcome Citation[75,152]. In addition, in cell therapies, specific molecular markers of cellular activation in the product may be associated with clinical efficacy Citation[152].
Systems biology is gradually becoming an important component in immune response monitoring and pharmacodynamic biomarker discovery. Applications include the monitoring of: i) immune cell characteristics, ii) the diversity of antibody responses (potentially related to epitope spread), iii) dynamics of T-cell clones and iv) immune characteristics of the tumor microenvironment. We discuss two examples below.
Earlier, we noted that Ji et al. have found gene expression patterns associated with lymphocyte infiltration and tumor destruction following treatment with ipilimumab (CTLA-4 blockade) Citation[108]. Further clinical studies are needed to establish whether these post-treatment expression signatures can be used as biomarkers of overall survival benefit upon ipilimumab therapy.
Early studies have demonstrated the utility of protein microarrays for the high-throughput measurement of antibody responses post-therapy Citation[115,120]. In clinical studies of GVAX® (Cell Genesys, CA, USA; GM-CSF-secreting whole cell immunotherapy) in prostate cancer, Nguyen et al. have reported the use of protein microarrays to identify specific antibody responses induced post-therapy Citation[120]. An antibody response against TARP (T cell alternative reading frame protein, a protein linked to prostate carcinogenesis) was significantly associated with the survival duration Citation[120]. Thus, protein microarrays appear to hold promise for the discovery of post-treatment serum antibody biomarkers of clinical outcome that can be translated for routine immune monitoring in patients. Such antibody responses have many of the characteristics of an ideal biomarker: readily accessible for quantitative analysis, and intimately tied to the in vivo therapeutic mechanism.
Expert commentary & five-year view
Omics technologies and systems biology are having an impact in many aspects of cancer immunotherapy discovery and development. There are elegant and practical examples of target discovery, mechanistic characterization and predictive or pharmacodynamic biomarker identification. We have been able to cover some of these in the current review. We believe we will see more successful applications in the near future, as new therapies are tested in clinical studies and new technologies for addressing the unique needs in immunotherapy become more mature and widely used. Application of the omics platforms has served as a complementary approach to the conventional immunological assays (such as multi-parameter flow-cytometry, ELISpot, ELISA and so on) for the generation of hypotheses and candidate biomarkers. Data from larger immunotherapy clinical trials will help to confirm the mechanistic themes and biomarkers that are emerging from the preclinical and early clinical studies. There are lessons to be learned from the omics applications in oncology, basic immunology and infectious diseases; advances in those areas should be leveraged for interpretation of the data that are being generated from the cancer immunotherapy studies.
New technological developments in omics platforms (e.g., NGS) are likely to affect the discovery efforts. In certain areas, multiple platforms have now become available, so researchers have the flexibility to use the one best suited to address the purpose of the study. For example, there are now a multitude of platforms for measurement of gene expression, including microarrays, NGS (RNA-sequencing) and multiplexed-qPCR. Therefore, a judicious selection of the appropriate, fit-for-purpose, platform is important. For addressing new target discovery or understanding MoA, RNA-sequencing or DNA microarrays may provide more value since they survey expression at the genome-wide level. For candidate biomarker discovery and translation to a limited quantitative panel, or for studying formalin-fixed, paraffin-embedded tumor tissues (where RNA is degraded), multiplexed qPCR or digital mRNA counting with color-coded probes can be a better choice Citation[154].
Genomic analyses of whole blood and PBMCs have a tremendous potential for enabling the discovery of mechanisms and biomarkers associated with clinical outcome to immunotherapeutics. Methods are being developed for such analyses Citation[19–21,39,99,106]. Data interpretation in this domain will benefit from the work being done to characterize the gene expression signatures associated with purified immune cell-types, or their differentiation, activation and signaling states. However, such approaches can have some issues. Methods for cell purification can alter their activation or signaling states, and certain expression patterns associated with purified cell-types or cellular activation may not be discernible from whole blood or PBMCs. Continued development and improvement of the methods for the analysis of complex immune cells will be important.
Discoveries using systems biology rely heavily upon the integration of heterogeneous data. Integration of omics data with clinical parameters or immune cell phenotypes (assessed from multi-parameter flow-cytometry) is often critical for understanding and interpretation of MoA and biomarkers Citation[155,156]. Large amounts of data can be generated even from small-scale omics experiments, which require appropriate database management systems and software for storage, integration and mining. Data from the very same biological specimen or patient are frequently stored in heterogeneous representations, making their integration difficult. Biologists often lack basic understanding of data management issues and the appreciation of how their data can be integrated across platforms; and professionals in database management or software development may lack a basic understanding of the nature of the biological questions being addressed. This can create a technical or communication gap between the professionals who are involved in the integration and mining of the data.
It will therefore be most fruitful to approach the systems biology challenges in a multidisciplinary manner that brings together expertise from immunology, cancer biology, computational biology and statistics, along with the appropriate infrastructure and tools needed to integrate and analyze the experimental data generated from a multitude of platforms Citation[156–158]. Appropriate statistical and network reconstruction methodologies are also needed for integrative mining of the data and generating comprehensive biological models from the omics data (e.g., Citation[159,160]). Such environments and methodologies are essential to obtain the best mechanistic insights possible into the therapies and to identify the robust and interpretable biomarkers that can be translated into practical clinical use for the benefit of patients receiving cancer immunotherapies.
Key issues
• The development of cancer immunotherapies is complex and challenging as it involves many poorly understood mechanisms of tumor targeting by the immune system and tumor’s immune evasion.
• Unbiased systems biology approaches can be important for many aspects of cancer immunotherapy discovery and development, starting from new tumor antigen discovery to the discovery of biomarkers reflective of the activity and efficacy of immunotherapies.
• There are many omics approaches and related analytical methods that are mature and well suited for the study of cancer immunotherapies, such as transcriptomic analyses of complex immune cells, mass spectrometry, T-cell receptor sequencing and protein microarrays.
• Although omics data from large Phase III clinical trials is limited in cancer immunotherapy, the current data from preclinical and early stage clinical studies are showing several consistent mechanistic themes across different modalities.
• Data from larger clinical studies are required to confirm the emerging mechanistic themes and for the validation of pre-treatment and pharmacodynamic biomarkers of clinical outcome. This should enable the identification of patients most likely to benefit from the immunotherapies, as well as faster development times and improved probability of success in future clinical testing.
• Experimental and computational methods for omics analysis of complex immune cells (e.g., whole blood, PBMCs) should significantly benefit the discovery of biomarkers of clinical efficacy. More of such methods need to be developed and made widely available.
• Integrative mining of data emerging from different platforms is often the key for obtaining deeper insights into MoA, new targets and biomarkers. To facilitate this, a diverse set of expertise is needed to support discovery and development efforts. It will be critical to use an interdisciplinary approach and integrative analysis in order to make rapid progress through the discovery and development cycles of cancer immunotherapies.
Acknowledgements
The authors thank Mark Frohlich for a critical review of the manuscript and many helpful comments. The authors also thank Kenneth Brasel and Harini Kandadi for useful discussions.
Financial & competing interests disclosure
The authors are all employees of Dendron Corporation. The authors have no other relevant affiliations or financial involvement with any organization or entity with a financial interest in or financial conflict with the subject matter or materials discussed in the manuscript apart from those disclosed.
No writing assistance was utilized in the production of this manuscript.
References
- Hood L, Heath JR, Phelps ME, Lin B. Systems biology and new technologies enable predictive and preventative medicine. Science 306(5696), 640–643 (2004).
- Zhu J, Zhang B, Schadt EE. A systems biology approach to drug discovery. Adv. Genet. 60, 603–635 (2008).
- Chuang HY, Hofree M, Ideker T. A decade of systems biology. Annu. Rev. Cell Dev. Biol. 26, 721–744 (2010).
- Germain RN, Meier-Schellersheim M, Nita-Lazar A, Fraser ID. Systems biology in immunology: a computational modeling perspective. Annu. Rev. Immunol. 29, 527–585 (2011).
- Stoughton RB, Friend SH. How molecular profiling could revolutionize drug discovery. Nat. Rev. Drug Discov. 4(4), 345–350 (2005).
- Pujol A, Mosca R, Farrés J, Aloy P. Unveiling the role of network and systems biology in drug discovery. Trends Pharmacol. Sci. 31(3), 115–123 (2010).
- Wang IM, Stone DJ, Nickle D, Loboda A, Puig O, Roberts C. Systems biology approach for new target and biomarker identification. Curr. Top. Microbiol. Immunol. 363, 169–199 (2013).
- Naylor S, Chen JY. Unraveling human complexity and disease with systems biology and personalized medicine. Per. Med. 7(3), 275–289 (2010).
- Tezak Z, Ranamukhaarachchi D, Russek-Cohen E, Gutman SI. FDA perspectives on potential microarray-based clinical diagnostics. Hum. Genomics 2(4), 236–243 (2006).
- Golub TR, Slonim DK, Tamayo P et al. Molecular classification of cancer: class discovery and class prediction by gene expression monitoring. Science 286(5439), 531–537 (1999).
- Perou CM, Sørlie T, Eisen MB et al. Molecular portraits of human breast tumours. Nature 406(6797), 747–752 (2000).
- Alizadeh AA, Eisen MB, Davis RE et al. Distinct types of diffuse large B-cell lymphoma identified by gene expression profiling. Nature 403(6769), 503–511 (2000).
- Van De Vijver MJ, He YD, Van’t Veer LJ et al. A gene-expression signature as a predictor of survival in breast cancer. N. Engl. J. Med. 347(25), 1999–2009 (2002).
- Paik S, Shak S, Tang G et al. A multigene assay to predict recurrence of tamoxifen-treated, node-negative breast cancer. N. Engl. J. Med. 351(27), 2817–2826 (2004).
- Slodkowska EA, Ross JS. MammaPrint 70-gene signature: another milestone in personalized medical care for breast cancer patients. Expert Rev. Mol. Diagn. 9(5), 417–422 (2009).
- Lyman GH, Cosler LE, Kuderer NM, Hornberger J. Impact of a 21-gene RT-PCR assay on treatment decisions in early-stage breast cancer: an economic analysis based on prognostic and predictive validation studies. Cancer 109(6), 1011–1018 (2007).
- Nakaya HI, Li S, Pulendran B. Systems vaccinology: learning to compute the behavior of vaccine induced immunity. Wiley Interdiscip. Rev. Syst. Biol. Med. 4(2), 193–205 (2012).
- Pulendran B, Li S, Nakaya HI. Systems vaccinology. Immunity 33(4), 516–529 (2010).
- Querec TD, Akondy RS, Lee EK et al. Systems biology approach predicts immunogenicity of the yellow fever vaccine in humans. Nat. Immunol. 10(1), 116–125 (2009).
- Berry MP, Graham CM, McNab FW et al. An interferon-inducible neutrophil-driven blood transcriptional signature in human tuberculosis. Nature 466(7309), 973–977 (2010).
- Pascual V, Chaussabel D, Banchereau J. A genomic approach to human autoimmune diseases. Annu. Rev. Immunol. 28, 535–571 (2010).
- Hanahan D, Weinberg RA. Hallmarks of cancer: the next generation. Cell 144(5), 646–674 (2011).
- Finn OJ. Cancer immunology. N. Engl. J. Med. 358(25), 2704–2715 (2008).
- Finn OJ. Immuno-oncology: understanding the function and dysfunction of the immune system in cancer. Ann. Oncol. 23(Suppl. 8), viii6–viii9 (2012).
- Kirkwood JM, Butterfield LH, Tarhini AA, Zarour H, Kalinski P, Ferrone S. Immunotherapy of cancer in 2012. CA. Cancer J. Clin. 62(5), 309–335 (2012).
- Chow MT, Möller A, Smyth MJ. Inflammation and immune surveillance in cancer. Semin. Cancer Biol. 22(1), 23–32 (2012).
- Mellman I, Coukos G, Dranoff G. Cancer immunotherapy comes of age. Nature 480(7378), 480–489 (2011).
- Topalian SL, Weiner GJ, Pardoll DM. Cancer immunotherapy comes of age. J. Clin. Oncol. 29(36), 4828–4836 (2011).
- Kasiske BL, Snyder JJ, Gilbertson DT, Wang C. Cancer after kidney transplantation in the United States. Am. J. Transplant. 4(6), 905–913 (2004).
- Grulich AE, van Leeuwen MT, Falster MO, Vajdic CM. Incidence of cancers in people with HIV/AIDS compared with immunosuppressed transplant recipients: a meta-analysis. Lancet 370(9581), 59–67 (2007).
- Clifford GM, Polesel J, Rickenbach M et al.; Swiss HIV Cohort. Cancer risk in the Swiss HIV Cohort Study: associations with immunodeficiency, smoking, and highly active antiretroviral therapy. J. Natl. Cancer Inst. 97(6), 425–432 (2005).
- Galon J, Costes A, Sanchez-Cabo F et al. Type, density, and location of immune cells within human colorectal tumors predict clinical outcome. Science 313(5795), 1960–1964 (2006).
- Jochems C, Schlom J. Tumor-infiltrating immune cells and prognosis: the potential link between conventional cancer therapy and immunity. Exp. Biol. Med. (Maywood) 236(5), 567–579 (2011).
- Hodi FS, O’Day SJ, McDermott DF et al. Improved survival with ipilimumab in patients with metastatic melanoma. N. Engl. J. Med. 363(8), 711–723 (2010).
- Kantoff PW, Higano CS, Shore ND et al.; IMPACT Study Investigators. Sipuleucel-T immunotherapy for castration-resistant prostate cancer. N. Engl. J. Med. 363(5), 411–422 (2010).
- Cheever MA, Higano CS. PROVENGE (Sipuleucel-T) in prostate cancer: the first FDA-approved therapeutic cancer vaccine. Clin. Cancer Res. 17(11), 3520–3526 (2011).
- Lipson EJ, Drake CG. Ipilimumab: an anti-CTLA-4 antibody for metastatic melanoma. Clin. Cancer Res. 17(22), 6958–6962 (2011).
- Grupp SA, June CH. Adoptive cellular therapy. Curr. Top. Microbiol. Immunol. 344, 149–172 (2011).
- Chaussabel D, Pascual V, Banchereau J. Assessing the human immune system through blood transcriptomics. BMC Biol. 8, 84 (2010).
- Yates JR, Ruse CI, Nakorchevsky A. Proteomics by mass spectrometry: approaches, advances, and applications. Annu. Rev. Biomed. Eng. 11, 49–79 (2009).
- Stoevesandt O, Taussig MJ, He M. Protein microarrays: high-throughput tools for proteomics. Expert Rev. Proteomics 6(2), 145–157 (2009).
- Holm A, Wu W, Lund-Johansen F. Antibody array analysis of labelled proteomes: how should we control specificity? N. Biotechnol. 29(5), 578–585 (2012).
- Metzker ML. Sequencing technologies – the next generation. Nat. Rev. Genet. 11(1), 31–46 (2010).
- Ozsolak F, Milos PM. RNA sequencing: advances, challenges and opportunities. Nat. Rev. Genet. 12(2), 87–98 (2011).
- Roselli M, Fernando RI, Guadagni F et al. Brachyury, a driver of the epithelial-mesenchymal transition, is overexpressed in human lung tumors: an opportunity for novel interventions against lung cancer. Clin. Cancer Res. 18(14), 3868–3879 (2012).
- Hopkins AL, Groom CR. The druggable genome. Nat. Rev. Drug Discov. 1(9), 727–730 (2002).
- Cheever MA, Allison JP, Ferris AS et al. The prioritization of cancer antigens: a national cancer institute pilot project for the acceleration of translational research. Clin. Cancer Res. 15(17), 5323–5337 (2009).
- Miles AK, Matharoo-Ball B, Li G, Ahmad M, Rees RC. The identification of human tumour antigens: current status and future developments. Cancer Immunol. Immunother. 55(8), 996–1003 (2006).
- Morgan RA, Chinnasamy N, Abate-Daga D et al. Cancer regression and neurological toxicity following anti-MAGE-A3 TCR gene therapy. J. Immunother. 36(2), 133–151 (2013).
- Carbone DP, Ciernik IF, Kelley MJ et al. Immunization with mutant p53- and K-ras-derived peptides in cancer patients: immune response and clinical outcome. J. Clin. Oncol. 23(22), 5099–5107 (2005).
- Peoples GE, Holmes JP, Hueman MT et al. Combined clinical trial results of a HER2/neu (E75) vaccine for the prevention of recurrence in high-risk breast cancer patients: US Military Cancer Institute Clinical Trials Group Study I-01 and I-02. Clin. Cancer Res. 14(3), 797–803 (2008).
- Caballero OL, Chen YT. Cancer/testis (CT) antigens: potential targets for immunotherapy. Cancer Sci. 100(11), 2014–2021 (2009).
- Bakker AB, Schreurs MW, de Boer AJ et al. Melanocyte lineage-specific antigen gp100 is recognized by melanoma-derived tumor-infiltrating lymphocytes. J. Exp. Med. 179(3), 1005–1009 (1994).
- Kantoff PW, Schuetz TJ, Blumenstein BA et al. Overall survival analysis of a Phase II randomized controlled trial of a Poxviral-based PSA-targeted immunotherapy in metastatic castration-resistant prostate cancer. J. Clin. Oncol. 28(7), 1099–1105 (2010).
- Brentjens RJ, Curran KJ. Novel cellular therapies for leukemia: CAR-modified T cells targeted to the CD19 antigen. Hematology Am. Soc. Hematol. Educ. Program 2012, 143–151 (2012).
- Loging WT, Lal A, Siu IM et al. Identifying potential tumor markers and antigens by database mining and rapid expression screening. Genome Res. 10(9), 1393–1402 (2000).
- Scanlan MJ, Gordon CM, Williamson B et al. Identification of cancer/testis genes by database mining and mRNA expression analysis. Int. J. Cancer 98(4), 485–492 (2002).
- Forbes SA, Bindal N, Bamford S et al. COSMIC: mining complete cancer genomes in the Catalogue of Somatic Mutations in Cancer. Nucleic Acids Res. 39(Database issue), D945–D950 (2011).
- Condomines M, Hose D, Rème T et al. Gene expression profiling and real-time PCR analyses identify novel potential cancer-testis antigens in multiple myeloma. J. Immunol. 183(2), 832–840 (2009).
- Scanlan MJ, Gure AO, Jungbluth AA, Old LJ, Chen YT. Cancer/testis antigens: an expanding family of targets for cancer immunotherapy. Immunol. Rev. 188, 22–32 (2002).
- Gnjatic S, Ritter E, Büchler MW et al. Seromic profiling of ovarian and pancreatic cancer. Proc. Natl Acad. Sci. USA 107(11), 5088–5093 (2010).
- Castle JC, Kreiter S, Diekmann J et al. Exploiting the mutanome for tumor vaccination. Cancer Res. 72(5), 1081–1091 (2012).
- Li G, Miles A, Line A, Rees RC. Identification of tumour antigens by serological analysis of cDNA expression cloning. Cancer Immunol. Immunother. 53(3), 139–143 (2004).
- Chen YT, Güre AO, Tsang S et al. Identification of multiple cancer/testis antigens by allogeneic antibody screening of a melanoma cell line library. Proc. Natl Acad. Sci. USA 95(12), 6919–6923 (1998).
- Chen YT, Scanlan MJ, Sahin U et al. A testicular antigen aberrantly expressed in human cancers detected by autologous antibody screening. Proc. Natl Acad. Sci. USA 94(5), 1914–1918 (1997).
- Hudson ME, Pozdnyakova I, Haines K, Mor G, Snyder M. Identification of differentially expressed proteins in ovarian cancer using high-density protein microarrays. Proc. Natl Acad. Sci. USA 104(44), 17494–17499 (2007).
- Liao WW, Arthur JW. Predicting peptide binding to Major Histocompatibility Complex molecules. Autoimmun. Rev. 10(8), 469–473 (2011).
- Lundegaard C, Lund O, Nielsen M. Predictions versus high-throughput experiments in T-cell epitope discovery: competition or synergy? Expert Rev. Vaccines 11(1), 43–54 (2012).
- Bozzacco L, Yu H, Zebroski HA et al. Mass spectrometry analysis and quantitation of peptides presented on the MHC II molecules of mouse spleen dendritic cells. J. Proteome Res. 10(11), 5016–5030 (2011).
- Tan CT, Croft NP, Dudek NL, Williamson NA, Purcell AW. Direct quantitation of MHC-bound peptide epitopes by selected reaction monitoring. Proteomics 11(11), 2336–2340 (2011).
- Seliger B, Dressler SP, Massa C et al. Identification and characterization of human leukocyte antigen class I ligands in renal cell carcinoma cells. Proteomics 11(12), 2528–2541 (2011).
- Weinschenk T, Gouttefangeas C, Schirle M et al. Integrated functional genomics approach for the design of patient-individual antitumor vaccines. Cancer Res. 62(20), 5818–5827 (2002).
- Hawkins OE, Vangundy RS, Eckerd AM et al. Identification of breast cancer peptide epitopes presented by HLA-A*0201. J. Proteome Res. 7(4), 1445–1457 (2008).
- Xu QW, Zhao W, Wang Y et al. An integrated genome-wide approach to discover tumor-specific antigens as potential immunologic and clinical targets in cancer. Cancer Res. 72(24), 6351–6361 (2012).
- Walter S, Weinschenk T, Stenzl A et al. Multipeptide immune response to cancer vaccine IMA901 after single-dose cyclophosphamide associates with longer patient survival. Nat. Med. doi:10.1038/nm.2883 (2012) (Epub ahead of print).
- Kreiter S, Castle JC, Türeci O, Sahin U. Targeting the tumor mutanome for personalized vaccination therapy. Oncoimmunology 1(5), 768–769 (2012).
- Medzhitov R, Horng T. Transcriptional control of the inflammatory response. Nat. Rev. Immunol. 9(10), 692–703 (2009).
- Haining WN, Wherry EJ. Integrating genomic signatures for immunologic discovery. Immunity 32(2), 152–161 (2010).
- Willinger T, Freeman T, Hasegawa H, McMichael AJ, Callan MF. Molecular signatures distinguish human central memory from effector memory CD8 T cell subsets. J. Immunol. 175(9), 5895–5903 (2005).
- Holmes S, He M, Xu T, Lee PP. Memory T cells have gene expression patterns intermediate between naive and effector. Proc. Natl Acad. Sci. USA 102(15), 5519–5523 (2005).
- Best JA, Blair DA, Knell J et al.; Immunological Genome Project Consortium. Transcriptional insights into the CD8(+) T cell response to infection and memory T cell formation. Nat. Immunol. 14(4), 404–412 (2013).
- Gilchrist M, Thorsson V, Li B et al. Systems biology approaches identify ATF3 as a negative regulator of Toll-like receptor 4. Nature 441(7090), 173–178 (2006).
- Abbas AR, Baldwin D, Ma Y et al. Immune response in silico (IRIS): immune-specific genes identified from a compendium of microarray expression data. Genes Immun. 6(4), 319–331 (2005).
- Subramanian A, Tamayo P, Mootha VK et al. Gene set enrichment analysis: a knowledge-based approach for interpreting genome-wide expression profiles. Proc. Natl Acad. Sci. USA 102(43), 15545–15550 (2005).
- Hung JH, Yang TH, Hu Z, Weng Z, DeLisi C. Gene set enrichment analysis: performance evaluation and usage guidelines. Brief. Bioinformatics 13(3), 281–291 (2012).
- Huang DAW, Sherman BT, Lempicki RA. Bioinformatics enrichment tools: paths toward the comprehensive functional analysis of large gene lists. Nucleic Acids Res. 37(1), 1–13 (2009).
- Huang H, Wu X, Sonachalam M et al. PAGED: a pathway and gene-set enrichment database to enable molecular phenotype discoveries. BMC Bioinformatics 13(Suppl. 15), S2 (2012).
- Culhane AC, Schröder MS, Sultana R et al. GeneSigDB: a manually curated database and resource for analysis of gene expression signatures. Nucleic Acids Res. 40(Database issue), D1060–D1066 (2012).
- Ashburner M, Ball CA, Blake JA et al. Gene ontology: tool for the unification of biology. The Gene Ontology Consortium. Nat. Genet. 25(1), 25–29 (2000).
- Kanehisa M, Goto S, Sato Y, Furumichi M, Tanabe M. KEGG for integration and interpretation of large-scale molecular data sets. Nucleic Acids Res. 40(Database issue), D109–D114 (2012).
- Croft D, O’Kelly G, Wu G et al. Reactome: a database of reactions, pathways and biological processes. Nucleic Acids Res. 39(Database issue), D691–D697 (2011).
- Barrett T, Troup DB, Wilhite SE et al. NCBI GEO: archive for functional genomics data sets – 10 years on. Nucleic Acids Res. 39(Database issue), D1005–D1010 (2011).
- Irizarry RA, Wang C, Zhou Y, Speed TP. Gene set enrichment analysis made simple. Stat. Methods Med. Res. 18(6), 565–575 (2009).
- Banchereau J, Palucka AK. Dendritic cells as therapeutic vaccines against cancer. Nat. Rev. Immunol. 5(4), 296–306 (2005).
- Tacken PJ, de Vries IJ, Torensma R, Figdor CG. Dendritic-cell immunotherapy: from ex vivo loading to in vivo targeting. Nat. Rev. Immunol. 7(10), 790–802 (2007).
- Palucka K, Banchereau J. Cancer immunotherapy via dendritic cells. Nat. Rev. Cancer 12(4), 265–277 (2012).
- Moschella F, Bisikirska B, Maffei A et al. Gene expression profiling and functional activity of human dendritic cells induced with IFN-α-2b: implications for cancer immunotherapy. Clin. Cancer Res. 9(6), 2022–2031 (2003).
- Han TH, Jin P, Ren J, Slezak S, Marincola FM, Stroncek DF. Evaluation of 3 clinical dendritic cell maturation protocols containing lipopolysaccharide and interferon-γ. J. Immunother. 32(4), 399–407 (2009).
- Chaussabel D, Quinn C, Shen J et al. A modular analysis framework for blood genomics studies: application to systemic lupus erythematosus. Immunity 29(1), 150–164 (2008).
- Wang IM, Bett AJ, Cristescu R, Loboda A, ter Meulen J. Transcriptional profiling of vaccine-induced immune responses in humans and non-human primates. Microb. Biotechnol. 5(2), 177–187 (2012).
- Painter MW, Davis S, Hardy RR, Mathis D, Benoist C; Immunological Genome Project Consortium. Transcriptomes of the B and T lineages compared by multiplatform microarray profiling. J. Immunol. 186(5), 3047–3057 (2011).
- Robbins SH, Walzer T, Dembélé D et al. Novel insights into the relationships between dendritic cell subsets in human and mouse revealed by genome-wide expression profiling. Genome Biol. 9(1), R17 (2008).
- Chan CW, Crafton E, Fan HN et al. Interferon-producing killer dendritic cells provide a link between innate and adaptive immunity. Nat. Med. 12(2), 207–213 (2006).
- Haining WN, Angelosanto J, Brosnahan K et al. High-throughput gene expression profiling of memory differentiation in primary human T cells. BMC Immunol. 9, 44 (2008).
- Lehtonen A, Ahlfors H, Veckman V, Miettinen M, Lahesmaa R, Julkunen I. Gene expression profiling during differentiation of human monocytes to macrophages or dendritic cells. J. Leukoc. Biol. 82(3), 710–720 (2007).
- Shen-Orr SS, Tibshirani R, Khatri P et al. Cell type-specific gene expression differences in complex tissues. Nat. Methods 7(4), 287–289 (2010).
- Gajewski TF, Louahed J, Brichard VG. Gene signature in melanoma associated with clinical activity: a potential clue to unlock cancer immunotherapy. Cancer J. 16(4), 399–403 (2010).
- Ji RR, Chasalow SD, Wang L et al. An immune-active tumor microenvironment favors clinical response to ipilimumab. Cancer Immunol. Immunother. 61(7), 1019–1031 (2012).
- Prins RM, Soto H, Konkankit V et al. Gene expression profile correlates with T-cell infiltration and relative survival in glioblastoma patients vaccinated with dendritic cell immunotherapy. Clin. Cancer Res. 17(6), 1603–1615 (2011).
- Tyagi P, Mirakhur B. MAGRIT: the largest-ever Phase III lung cancer trial aims to establish a novel tumor-specific approach to therapy. Clin. Lung Cancer 10(5), 371–374 (2009).
- Ribas A, Timmerman JM, Butterfield LH, Economou JS. Determinant spreading and tumor responses after peptide-based cancer immunotherapy. Trends Immunol. 24(2), 58–61 (2003).
- Vanderlugt CL, Miller SD. Epitope spreading in immune-mediated diseases: implications for immunotherapy. Nat. Rev. Immunol. 2(2), 85–95 (2002).
- Yuan J, Adamow M, Ginsberg BA et al. Integrated NY-ESO-1 antibody and CD8+ T-cell responses correlate with clinical benefit in advanced melanoma patients treated with ipilimumab. Proc. Natl Acad. Sci. USA 108(40), 16723–16728 (2011).
- Nesslinger NJ, Ng A, Tsang KY et al. A viral vaccine encoding prostate-specific antigen induces antigen spreading to a common set of self-proteins in prostate cancer patients. Clin. Cancer Res. 16(15), 4046–4056 (2010).
- Kwek SS, Dao V, Roy R et al. Diversity of antigen-specific responses induced in vivo with CTLA-4 blockade in prostate cancer patients. J. Immunol. 189(7), 3759–3766 (2012).
- Disis ML, Goodell V, Schiffman K, Knutson KL. Humoral epitope-spreading following immunization with a HER-2/neu peptide based vaccine in cancer patients. J. Clin. Immunol. 24(5), 571–578 (2004).
- Mittendorf EA, Gurney JM, Storrer CE, Shriver CD, Ponniah S, Peoples GE. Vaccination with a HER2/neu peptide induces intra- and inter-antigenic epitope spreading in patients with early stage breast cancer. Surgery 139(3), 407–418 (2006).
- Wierecky J, Müller MR, Wirths S et al. Immunologic and clinical responses after vaccinations with peptide-pulsed dendritic cells in metastatic renal cancer patients. Cancer Res. 66(11), 5910–5918 (2006).
- Butterfield LH, Ribas A, Dissette VB et al. Determinant spreading associated with clinical response in dendritic cell-based immunotherapy for malignant melanoma. Clin. Cancer Res. 9(3), 998–1008 (2003).
- Nguyen MC, Tu GH, Koprivnikar KE, Gonzalez-Edick M, Jooss KU, Harding TC. Antibody responses to galectin-8, TARP and TRAP1 in prostate cancer patients treated with a GM-CSF-secreting cellular immunotherapy. Cancer Immunol. Immunother. 59(9), 1313–1323 (2010).
- Bamshad MJ, Ng SB, Bigham AW et al. Exome sequencing as a tool for Mendelian disease gene discovery. Nat. Rev. Genet. 12(11), 745–755 (2011).
- Alkan C, Coe BP, Eichler EE. Genome structural variation discovery and genotyping. Nat. Rev. Genet. 12(5), 363–376 (2011).
- Meyerson M, Gabriel S, Getz G. Advances in understanding cancer genomes through second-generation sequencing. Nat. Rev. Genet. 11(10), 685–696 (2010).
- Wang C, Sanders CM, Yang Q et al. High throughput sequencing reveals a complex pattern of dynamic interrelationships among human T cell subsets. Proc. Natl Acad. Sci. USA 107(4), 1518–1523 (2010).
- Warren RL, Freeman JD, Zeng T et al. Exhaustive T-cell repertoire sequencing of human peripheral blood samples reveals signatures of antigen selection and a directly measured repertoire size of at least 1 million clonotypes. Genome Res. 21(5), 790–797 (2011).
- Robins HS, Campregher PV, Srivastava SK et al. Comprehensive assessment of T-cell receptor β-chain diversity in αβ T cells. Blood 114(19), 4099–4107 (2009).
- Boyd SD, Marshall EL, Merker JD et al. Measurement and clinical monitoring of human lymphocyte clonality by massively parallel VDJ pyrosequencing. Sci. Transl. Med. 1(12), 12ra23 (2009).
- Freeman JD, Warren RL, Webb JR, Nelson BH, Holt RA. Profiling the T-cell receptor β-chain repertoire by massively parallel sequencing. Genome Res. 19(10), 1817–1824 (2009).
- Murugan A, Mora T, Walczak AM, Callan CG Jr. Statistical inference of the generation probability of T-cell receptors from sequence repertoires. Proc. Natl Acad. Sci. USA 109(40), 16161–16166 (2012).
- Baum PD, Venturi V, Price DA. Wrestling with the repertoire: the promise and perils of next generation sequencing for antigen receptors. Eur. J. Immunol. 42(11), 2834–2839 (2012).
- Bolotin DA, Mamedov IZ, Britanova OV et al. Next generation sequencing for TCR repertoire profiling: platform-specific features and correction algorithms. Eur. J. Immunol. 42(11), 3073–3083 (2012).
- Wu D, Sherwood A, Fromm JR et al. High-throughput sequencing detects minimal residual disease in acute T lymphoblastic leukemia. Sci. Transl. Med. 4(134), 134ra63 (2012).
- Logan AC, Gao H, Wang C et al. High-throughput VDJ sequencing for quantification of minimal residual disease in chronic lymphocytic leukemia and immune reconstitution assessment. Proc. Natl Acad. Sci. USA 108(52), 21194–21199 (2011).
- Grupp SA, Prak EL, Boyer J et al. Adoptive transfer of autologous T cells improves T-cell repertoire diversity and long-term B-cell function in pediatric patients with neuroblastoma. Clin. Cancer Res. 18(24), 6732–6741 (2012).
- Chervin AS, Stone JD, Soto CM et al. Design of T-cell receptor libraries with diverse binding properties to examine adoptive T-cell responses. Gene Ther. doi:10.1038/gt.2012.80 (2012) (Epub ahead of print).
- Disis ML. Immunologic biomarkers as correlates of clinical response to cancer immunotherapy. Cancer Immunol. Immunother. 60(3), 433–442 (2011).
- Komatsu N, Matsueda S, Tashiro K et al. Gene expression profiles in peripheral blood as a biomarker in cancer patients receiving peptide vaccination. Cancer 118(12), 3208–3221 (2012).
- Ross RW, Galsky MD, Scher HI et al. A whole-blood RNA transcript-based prognostic model in men with castration–resistant prostate cancer: a prospective study. Lancet Oncol. 13(11), 1105–1113 (2012).
- Olmos D, Brewer D, Clark J et al. Prognostic value of blood mRNA expression signatures in castration-resistant prostate cancer: a prospective, two-stage study. Lancet Oncol. 13(11), 1114–1124 (2012).
- Breunis WB, Tarazona-Santos E, Chen R, Kiley M, Rosenberg SA, Chanock SJ. Influence of cytotoxic T lymphocyte-associated antigen 4 (CTLA4) common polymorphisms on outcome in treatment of melanoma patients with CTLA-4 blockade. J. Immunother. 31(6), 586–590 (2008).
- Liu D, O’Day SJ, Yang D et al. Impact of gene polymorphisms on clinical outcome for stage IV melanoma patients treated with biochemotherapy: an exploratory study. Clin. Cancer Res. 11(3), 1237–1246 (2005).
- Cortes A, Brown MA. Promise and pitfalls of the Immunochip. Arthritis Res. Ther. 13(1), 101 (2011).
- Cerhan JR, Ansell SM, Fredericksen ZS et al. Genetic variation in 1253 immune and inflammation genes and risk of non-Hodgkin lymphoma. Blood 110(13), 4455–4463 (2007).
- Cheung VG, Spielman RS. Genetics of human gene expression: mapping DNA variants that influence gene expression. Nat. Rev. Genet. 10(9), 595–604 (2009).
- Schadt EE, Molony C, Chudin E et al. Mapping the genetic architecture of gene expression in human liver. PLoS Biol. 6(5), e107 (2008).
- Nicolae DL, Gamazon E, Zhang W, Duan S, Dolan ME, Cox NJ. Trait-associated SNPs are more likely to be eQTLs: annotation to enhance discovery from GWAS. PLoS Genet. 6(4), e1000888 (2010).
- Fox BA, Schendel DJ, Butterfield LH et al. Defining the critical hurdles in cancer immunotherapy. J. Transl. Med. 9(1), 214 (2011).
- Butterfield LH, Disis ML, Khleif SN, Balwit JM, Marincola FM. Immuno-oncology biomarkers 2010 and beyond: perspectives from the iSBTc/SITC biomarker task force. J. Transl. Med. 8, 130 (2010).
- Hoos A, Eggermont AM, Janetzki S et al. Improved endpoints for cancer immunotherapy trials. J. Natl. Cancer Inst. 102(18), 1388–1397 (2010).
- Madan RA, Gulley JL, Fojo T, Dahut WL. Therapeutic cancer vaccines in prostate cancer: the paradox of improved survival without changes in time to progression. Oncologist 15(9), 969–975 (2010).
- Butterfield LH, Palucka AK, Britten CM et al. Recommendations from the iSBTc-SITC/FDA/NCI Workshop on Immunotherapy Biomarkers. Clin. Cancer Res. 17(10), 3064–3076 (2011).
- Sheikh NA, Petrylak D, Kantoff PW et al. Sipuleucel-T immune parameters correlate with survival: an analysis of the randomized Phase 3 clinical trials in men with castration-resistant prostate cancer. Cancer Immunol. Immunother. 62(1), 137–147 (2013).
- Lonchay C, van der Bruggen P, Connerotte T et al. Correlation between tumor regression and T cell responses in melanoma patients vaccinated with a MAGE antigen. Proc. Natl Acad. Sci. USA 101(Suppl. 2), 14631–14638 (2004).
- Geiss GK, Bumgarner RE, Birditt B et al. Direct multiplexed measurement of gene expression with color-coded probe pairs. Nat. Biotechnol. 26(3), 317–325 (2008).
- Philippi S, Köhler J. Addressing the problems with life-science databases for traditional uses and systems biology. Nat. Rev. Genet. 7(6), 482–488 (2006).
- Chaussabel D, Ueno H, Banchereau J, Quinn C. Data management: it starts at the bench. Nat. Immunol. 10(12), 1225–1227 (2009).
- Disis ML, Slattery JT. The road we must take: multidisciplinary team science. Sci. Transl. Med. 2(22), 22cm9 (2010).
- Hawkins RD, Hon GC, Ren B. Next-generation genomics: an integrative approach. Nat. Rev. Genet. 11(7), 476–486 (2010).
- Hwang D, Rust AG, Ramsey S et al. A data integration methodology for systems biology. Proc. Natl Acad. Sci. USA 102(48), 17296–17301 (2005).
- Schadt EE, Björkegren JL. NEW: network-enabled wisdom in biology, medicine, and health care. Sci. Transl. Med. 4(115), 115rv1 (2012).