Abstract
Interest is rising in measuring subjective health outcomes, such as treatment outcomes that are not directly quantifiable (functional disability, symptoms, complaints, side effects and health-related quality of life). Health economists in particular have applied probabilistic choice models in the area of health evaluation. They increasingly use discrete choice models based on random utility theory to derive values for healthcare goods or services. Recent attempts have been made to use discrete choice models as an alternative method to derive values for health states. In this article, various probabilistic choice models are described according to their underlying theory. A historical overview traces their development and applications in diverse fields. The discussion highlights some theoretical and technical aspects of the choice models and their similarity and dissimilarity. The objective of the article is to elucidate the position of each model and their applications for health-state valuation.
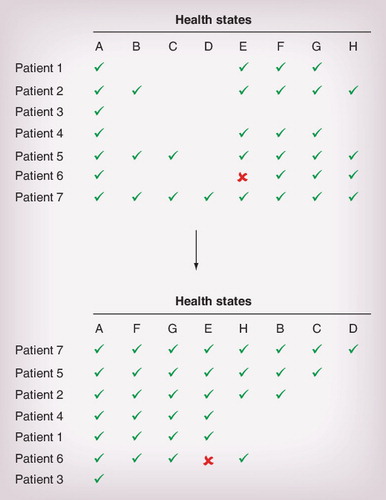
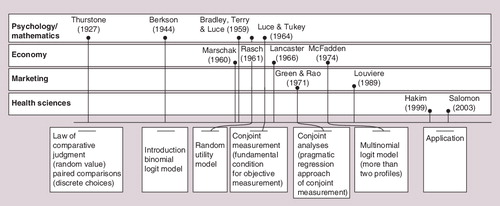
Reproduced with permission from Citation[47].
![Figure 3. Deriving paired comparison data based on rank data.Reproduced with permission from Citation[47].](/cms/asset/0bf8ae91-9119-4cab-942b-788fb6d23542/ierp_a_11215612_f0003_b.jpg)
Health outcome measures can be divided into objective and subjective measures. For many years, the outcomes were articulated primarily in terms of death, disability or cure. Nowadays, the assessment of medical interventions and healthcare services also takes health-related quality of life, treatment process characteristics, side effects and patient satisfaction into account by means of patient-reported outcomes, clinical-reported outcomes and observer-reported outcomes. Subjective measures such as patient-reported outcomes are therefore becoming core outcome measures for clinical trials, interventions in cure organization and other types of health studies. Many of these studies are carried out to demonstrate the efficiency or effectiveness of new interventions or protocols.
Objective measurement is the estimation or determination of extent, dimension or capacity in relation to some standard or unit of measurement. To solve the problem of measuring in the absence of such a standard or unit, methodologies have been developed to measure phenomena that are unobservable, hence subjective. Such methodologies, sometimes known as scaling models, can establish the relative merit (value) of a subjective phenomenon. The values (variously called utilities, strengths of preferences, indices or weights) that scaling methods that will be discussed in this article generate are assumed to have a specific measurement property, namely that the differences between values possess cardinal qualities. This means that the differences between values reflect true differences and lie on a continuous scale (e.g., if a patient’s score changes from 20 to 40, this increase is the same as from 70 to 90).
Over the past decade, the use of discrete choice (DC) models has proliferated in the area of health evaluation, especially in health economics. The vast majority of published studies using this methodology in health evaluation tend to focus on the possibility that individuals derive benefit from nonhealth outcomes and process attributes (e.g., therapy convenience or waiting time) in addition to health outcomes (safety or effectiveness). Applying DC models in health has been described as deriving values beyond health or clinical outcomes Citation[1]. In addition, DC models have been introduced as an alternative method to standard gamble (SG), time trade-off (TTO) and visual analogue scales (VAS) to derive health-state values Citation[2–7]. Access to valid and accurate values for a wide range of health conditions is also advantageous as these can be used in health outcomes research, disease modeling studies and economic evaluations (cost–utility analysis), and to monitor the health-related quality of life of individuals in the general community (as well as in clinical studies).
DC models belong to the class denoted in the statistical literature as probabilistic choice (PC) models. All PC models have in common that they are able to establish the relative merit of a phenomenon. In technical terms, these models take data obtained at one measurement level and transform it to an aggregated higher level. The PC models that will be discussed in this article, which are used in health-state valuation, are supposed to generate an interval scale (cardinal data) from ordinal data. If the phenomenon is described according to characteristics (or attributes) with certain levels, extended PC models make it possible to estimate the relative importance assigned to these attributes and their associated levels, and even to estimate overall value for different combinations of attribute levels. Models falling into the latter subset of PC models are applied in conjoint analysis, a term that is often used interchangeably (and sometimes incorrectly) with DC modeling. Extended PC models have been used widely to elicit values in a number of other research areas, notably in marketing, transportation and environmental economics Citation[8].
PC models are powerful but can be complex. The art of finding the appropriate model for a particular application requires researchers to be familiar with the subject of interest so that the relevant attributes and levels can be applied in the choice task. Additionally, researchers need to understand a model’s methodological and theoretical background in order to be able to arrive at valid conclusions. Furthermore, several different PC models exist. While they are all related, their theoretical assumptions, purposes and practical applicability differ. Another complicating factor is that the subset of DC models within PC models has been described in the literature variously as discrete choice experiments Citation[9], conditional logit (and later on also probit) analysis Citation[10], discrete choice analysis Citation[11], conjoint analysis Citation[12], discrete choice conjoint analysis Citation[13], stated preference discrete choice modeling Citation[14] and random utility choice models Citation[15].
The aim of this paper is to present a historical, theoretical and methodological overview of different PC models used to quantify subjective health outcomes. Particular attention is devoted to the introduction, development and peculiarities of PC models and of the subset of DC models. The similarities and differences between the underlying models are explained. Then the discussion turns to some issues related to the widespread use of DC models to evaluate individuals’ preferences in health-state valuation.
Historical development of modeling preferences
Thurstone’s law of comparative judgment
The long tradition of PC models started in 1927 with Louis Thurstone, who began his career as an electrical engineer and worked for many years as a psychometrician at the University of Chicago (IL, USA). He formulated a mathematical model, which he called the Law of Comparative Judgment (LCJ), that could be used to estimate scale values (a latent trait) based on binary choices between stimuli Citation[16]. A ‘discriminal process’ mediates each psychological stimulus magnitude. Thurstone proposed that perceived physical phenomena (e.g., brightness and weight) or subjective concepts (e.g., seriousness of crimes and taste) could be expressed as a true weight and a random component. In psychology, subjective phenomena are regarded as attitudes: psychological tendencies that are expressed by evaluating a particular entity with some degree of favor and/or disfavor. Attitudes can be regarded as mental constructs of phenomena that people want to acquire or reject, like or dislike, or wish to protect or harm.
Thurstone built upon work by his predecessors, in particular Gustav Fechner (1801–1887), a German experimental psychologist. Fechner was a pioneer in experimental psychology and is credited to be the founder of psychophysics. He inspired many 20th century scientists and philosophers, including Thurstone. Early psychophysical work built upon precise but simple experiments. A typical example is: consider the following two objects with weights, w1 and w2; which one is heavier? Such experiments would demonstrate that the greater the difference in object weight, the greater the probability of choosing correctly (however, note Citation[17]). This measurement approach is based on making comparative judgments. In everyday life, people rarely make absolute judgments (i.e., attach a numeric value). Most choices are based on judgments and are inherently comparative. In psychology, discrimination is therefore regarded as a basic operation of judgment and of generating knowledge.
Thurstone postulated that each stimulus (i.e., object, item, state and scenario) in a set of stimuli would possess some attributes in varying but unknown degrees. For each stimulus and among all subjects, it is assumed that a preference will exist. Furthermore he postulated that for each stimulus the overall preference will be distributed normally around the most frequent (modal) response. To measure such overall preferences, each person’s preference for each stimulus versus every other stimulus has to be obtained. The more people who select one stimulus of a pair over the other stimulus, the greater the preference for that stimulus, and thus the greater its scale weight. Therefore, the basic element of subjective measurement in the framework of comparative measurement (as opposed to monadic measurement, in which stimuli are valued separately) is a simple and straightforward response task based on a comparison between two stimuli, done in such a way that it yields data that contain compelling information.
Thurstone’s approach is indirect; it is based on an underlying theory allowing raw individual data to be transformed into aggregate data. Therefore, psychometricians regard it as scaling. In terms of modern psychometric theory, however, it is more aptly regarded as a measurement model [Appendix: A]. Because Thurstone’s model derives group scale values from imprecise individual data, it is also regarded as a probabilistic choice model. Thurstone’s LCJ can only be used to model paired comparisons. The model that will be discussed in the next section allows for modeling comparisons with more than two alternatives.
Bradley–Terry–Luce model
Another approach to comparative data is the Bradley–Terry–Luce (BTL) model, as statistically formulated by Bradley and Terry in 1955 Citation[18] and extended by Luce in 1959 Citation[19]. It extends the Thurstone model by enabling a person to choose from more than two alternatives. The BTL model postulates that measurement on a ratio level can be established if the data satisfy certain structural assumptions Citation[20]. For mathematical reasons, the BTL model is based on the simple logistic function instead of the normal distribution of the Thurstone model [Appendix: B]. If only pairs of alternatives are judged, the BTL model is identical to Thurstone’s (except for the error terms). However, when more than two alternatives are judged, an important assumption must be made, namely the independence of irrelevant alternatives (IIA). The mathematical implication is that the rate of substitution between two or more alternatives remains unchanged by adding an alternative. As discussed later, the IIA assumption is a key property of almost all basic logit DC models Citation[21].
Conjoint measurement
Another advance in mathematical psychology was fundamental measurement representation, developed by Luce and Tukey in 1964 Citation[19,22]. Fundamental measurement theory is a mathematical framework based on logical (not normative) axioms. It concerns exclusively the qualitative conditions under which a particular representation (measurement and scaling) holds. One of the earliest representational measurement theories is conjoint measurement Citation[22].
Scientists realized that the social sciences could not live up to the standards of objective measurement that was being applied in the physical sciences. Conjoint measurement was developed to be able to perform fundamental measurement with subjective entities or concepts. It is used to measure the joint effects of two or more independent variables on the ordering of a dependent variable (the property to be quantified). As Perline, Wright and Wainer Citation[23] put it: “The question is whether or not there exists a monotonic transformation of an ordinal measure of the dependent variable from which an additive representation can be constructed.” The axiomatization of conjoint measurement is complicated. Its full version includes technical axioms (e.g., consistency, transitivity and double cancellation), which can often plausibly be assumed to hold approximately Citation[24,25]. When the axioms hold, the result is that the observed but transformed dependent variable and the concomitantly constructed independent variables are simultaneously (hence the term ‘conjoint’) represented on an interval scale with a common unit Citation[23]. Conjoint measurement, as a member of the class of fundamental measurement theories, is algebraic (designating an expression in which only numbers, letters and arithmetic operations are contained or used) and therefore deterministic (as opposed to most models described in this article that are probabilistic).
Rasch model
Although conjoint measurement is generally acknowledged as an important theoretical contribution, its practicality is in doubt because of its strict axiomatic assumptions. The Rasch Citation[26] model – independently developed from conjoint measurement – can be seen as a practical rendition of conjoint measurement with an underlying stochastic structure Citation[23]. Georg Rasch (1901–1980) was a Danish mathematician, statistician and psychometrician. He applied his model for dichotomous data to data derived from responses to attainment and intelligence tests Citation[26]. These tests do not confront the respondents with a comparative task. The Rasch model is the only one in this overview that uses responses (e.g., agree/disagree, correct/incorrect or able/unable) collected separately from a set of questions (monadic measurement). For this reason, the Rasch model is not a choice model in a strict sense. However, when comparisons are made between one’s own health status versus a hypothetical state, it can be considered a choice model. The Rasch model is particularly useful in psychometrics, the field concerned with the theory and technique of psychological and educational measurement.
Later extensions of the Rasch model are known as item response theory (IRT) models. These are increasingly used in other areas, including the health profession Citation[27–31]. IRT models are mathematical functions that specify the probability of a discrete outcome, such as a correct response to an item, in terms of both item and person parameters [Appendix: C]. Item parameters include the difficulty of an item (for health states: severity) and the discrimination of an item (for health states: the agreement among respondents on the severity). Person parameters may represent the ability of a student or the strength of a person’s attitude, for example (for health states, the person parameter represents a person’s own health state). The items may be questions that have incorrect and correct responses, or they may be statements on questionnaires that allow the respondents to indicate their level of agreement. So far, IRT models have been used for the quantification of single domains and for the selection of relevant domains of classification systems; however, they have not been used to model the quality of health states. However, such an application seems feasible based on responses from patients instead of the general population Citation[32].
It turns out that the Rasch model is very closely related to the BTL model with regard to measurement, while the structure of the items is closely related to Guttman scaling Citation[33]. The key difference between the Rasch model and all other logit models is that the former has been extended with a separate parameter to estimate each respondent’s position on the scale Citation[34]. By an interactive conditional maximum likelihood estimation approach, a scale estimation is obtained without involvement of the person parameter, which is specific to the Rasch model. Therefore, Rasch models have a specific measurement property, namely invariance, which is a critical criterion of fundamental measurement. For health-state valuation, the property of invariance means that the outcome of choices between two (or more) health states should not be dependent on which group of respondents performed the assessments. Additionally, the resulting choices among health states should also be independent of the set of health states that were assessed. Obviously, this demands a strong specification of the structure (Guttman structure, ) of the response data Citation[35], a requirement that is not often satisfied. However, when all assumptions of the Rasch model hold, the model is used to construct the variable of interest. This represents a different philosophical perspective. In the Rasch model, the data are fitted to the model instead of vice versa. Therefore, it can be stated that the Rasch model allows for truly objective (fundamental) measurement. This is similar to Guttman scaling, Coombs Unfolding Citation[36] and measurement in the physical sciences. Extensions of the Rasch model (i.e., IRT models) relax to some extent the strong requirements posed on the response data, but these models do not possess fundamental measurement properties. To estimate both the person and item location parameters, the Rasch model is formulated as a conditional logit model Citation[26].
The standard Rasch model, the BTL model, and Thurstone’s LCJ model can only be used to derive scale values for the judged alternatives. The methods that will be successively discussed below are extended models that also facilitate estimating the contribution of the characteristics of health outcomes, if identifiable and structured.
Conjoint analysis
A professor in marketing, Green Citation[37], recognized that Luce and Tukey’s conjoint measurement article Citation[22] provided a new system to quantify rank order data. This type of data could be applied to marketing research (e.g., to forecast market response for new products). His more pragmatic approach (no formal checks and based on regression models) is what is now called conjoint analysis. Today this technique is used in many of the social and applied sciences. The objective of conjoint analysis is to determine the separate contribution of a limited number of attributes of an object on its overall value.
With conjoint analysis, respondents are generally shown a set of products, goods, services, scenarios or pictures. Each example is similar enough to the others that respondents will see them as close substitutes but dissimilar enough that they can clearly determine a preference. Each example is composed of a unique combination of features. The response task may consist of individual ratings, rank orders or choices among alternative combinations.
In addition to different possible response modes, there are further differences within the conjoint analysis approach. There are different models (i.e., full profile, partial/incomplete profile, hierarchical, Bayesian, and so on) and different designs (i.e., full factorial, fractional factorial, resolution III, and so on). In that regard, conjoint analysis can be taken as an umbrella term describing various methods to derive quantitative measures for subjective phenomena based on a combination of stimulus configuration, experimental design, response modes and statistical analyses. The reader is referred to Louviere et al. Citation[38] for an excellent discussion of the differences between conjoint analysis described above, and discrete choice models, which will be discussed in the next section.
Discrete choice models
In Thurstone’s LCJ, the perceived level of a stimulus equals a systematic component plus a random error. In the LCJ choices are modeled as the probability that one object is rated higher than a second because this alternative has the higher perceived stimulus. When the perceived stimuli are defined in terms of utility, this law can be turned into a model for economic choice in which utility is modeled as a random variable. This implication was drawn by the economist Marschak in 1960, who thereby introduced Thurstone’s work into economics. Marschak called this the random utility maximization hypothesis or random utility model (RUM) Citation[39]. The RUM assumes, in line with neoclassical economic theory, that the decision-makers are rational in the sense that they make choices which maximize their perceived utility (subject to economic and cognitive constraints). However, to accommodate for the demonstrated inability of individuals to discriminate perfectly and of the analyst to exactly measure the subject of interest a random utility function is assumed Citation[16,40].
Modern DC models came from econometrics and built upon the work of McFadden, who was awarded the Nobel Prize in economics in 2000 Citation[10]. DC models encompass a variety of experimental design techniques, data collection protocols and statistical procedures that can be used to predict the choices that subjects will make between alternatives. These techniques can be applied when subjects have the ability to choose between two or more distinct (‘discrete’) alternatives. In the mid-1960s, McFadden was working with a graduate student who had obtained data on freeway routing decisions from the California Department of Transportation. His graduate student was looking for a way to analyze her data to study economic decision-making behavior. McFadden developed the first version of what he called ‘conditional logit analysis’ Citation[10] (often referred to as the multinomial logistic model, this term is used in other contexts to refer to a partially different model [Appendix: D]). He proposed an econometric model in which the values of alternatives depended on values assigned to their attributes, such as construction cost, route length, and areas of parkland and open space taken up Citation[41]. He developed a computer program that allowed him to estimate this model, based on an axiomatic theory of choice behavior developed by the mathematical psychologist Luce Citation[19].
As the foundation for his probabilistic choice model, Luce’s choice axiom states that the probability of choosing one stimulus over another from a set of many stimuli is not affected by the presence or absence of other stimuli in the set (IIA assumption [Appendix: E]), and that these stimuli have independent and identically distributed measurement errors Citation[19]. The IIA axiom simplifies experimental collection of choice data by allowing multinomial choice probabilities to be inferred from binomial choice experiments Citation[42].
Drawing upon the work of Thurstone, Marschak and Lancaster Citation[43], McFadden was able to show how his model was linked to the economic theory of choice behavior. McFadden then investigated further the RUM foundations of the conditional multinomial logistic model. He showed that the Luce model was consistent with the RUM model with independent and identically distributed random variables (IID) if and only if the error term had a distribution called extreme value type I (also called Gumbel distribution).
Before the contribution of Louviere et al. Citation[8,44], DC models had been used to analyze behavior that could be observed in real market contexts. Louviere and other researchers applied DC models to choices collected from respondents who were presented profiles of features of hypothetical products; this is what they called ‘simulated choice situations’. So, instead of modeling the actual choices made by people, as McFadden did with the revealed preferences approach, Louviere modeled the choices made by subjects in carefully constructed experimental studies (discrete choice experiments), using the stated preferences approach. This new approach made it possible to predict values for alternatives that could not be judged in the real world (see for an overview).
Examples of probabilistic choice models applied in health-state valuation
In the following section, some examples will be provided of PC model analyses that have been performed in the field of health-state valuation. This section does not claim to be exhaustive and the studies that will be highlighted are for illustrative purposes only. The reader is encouraged to consult the original articles for more details.
As early as 1970 the field of health-state valuation recognized that Thurstone’s model might be useful for valuing health states Citation[45], which was later on applied by Hadorn et al. Citation[46] and Krabbe Citation[47]. Stolk et al. Citation[48] compared several health-state methodologies to each other, one of which was a DC experiment (DCE) modeled with a conditional multinomial logit analysis to estimate the main effects (no second order or higher interactions). The classification system that was valued was the EuroQol-5D (EQ-5D) Citation[49]. The other valuation techniques that they investigated were TTO, VAS, rank-ordered logit and Thurstone’s LCJ. Other well-known studies that used the conditional multinomial logit model to estimate EQ-5D values are Salomon Citation[42], and Hakim and Pathak Citation[2]. McCabe et al. Citation[3] used the conditional multinomial logit to model HUI-2 and SF-6D values. Coast et al. Citation[50] applied the conditional multinomial logit to model best–worst scaling preferences for the ICECAP-O instrument.
Ratcliffe et al. Citation[5] valued a disease-specific classification system (SQOL-3D), comparing TTO with a DC experiment. They analyzed the DCE data with a random effects probit model, in which they took into account the fact that multiple responses were obtained from the same individual. Additionally, they also investigated a rank-ordered logit model. They found that the probit model resulted in higher values than the rank-ordered logit model, and both these methods produced values dissimilar to TTO models. Another example of the conditional multinomial probit is Brazier et al. who used it to model values for the Asthma Quality of Life Classification and the Overactive Bladder-Specific Measure Citation[51]. A third example using probit models is Craig et al., who used a homoskedastic probit model based on rank responses (exploded probit) Citation[52]. presents an overview of techniques and probabilistic choice models that can be used for the measurement of health-state values.
Discussion
General considerations
Discrimination is a basic operation of judgment and of generating knowledge. Most judgments in daily life consist of making choices between some competitive alternatives and are thus inherently comparative. Therefore, the core activity of measurement with PC models is to compare two or more stimuli so that the data provide relevant information on individuals’ choice behavior. PC models present relatively simple and straightforward response tasks. These are easy to perform from simulated (i.e., hypothetical but realistic) scenarios, yet they provide information to arrive at quantitative measures.
All probabilistic choice models that allow the estimation of values for alternatives are basically versions of the Thurstonian LCJ model. Analytical complexity is, unfortunately, typical of most probabilistic choice models, in particular the logit models. A few software packages (e.g., Stata) have addressed the issue by building in analytical procedures that, to varying degrees, simplify discrete choice analysis.
Some salient differences between the initial (Thurstone) and the subsequent DC models warrant elaboration. First, DC models were extended to analyze choices between more than two scenarios in a choice set. This refinement is straightforward and allows for more realistic scenarios. For instance, these models facilitate analyses of choices from sets including an opt-out option, such as not being treated at all rather than being treated with two different drug regimens, or preferring to be dead rather than to live in the two presented health states. Second, whereas the classic Thurstone model is only applicable to derive values for empirically judged scenarios, the DC models are extended to parameterize by regression models the contribution of the levels of attributes based on the assessment of a subset of the complete set of scenarios Citation[8]. This makes it possible to capture the relationships between rankings and levels of the attributes in a value-based health status classification system. Some research has already been conducted in this area Citation[2,5,50,53]. However, the process of going from choice data between states to values for these states is (almost) identical Citation[47]. The classic Thurstonian models are particularly suitable when the differences between objects are small, as the underlying response task (paired comparisons) is very well suited to detecting differences where direct or monadic measurement methods (e.g., visual analogue scale, TTO) will fail. It has been shown that the LCJ and extensions of it can even be embedded in the contemporary and very general framework of structural equation modeling Citation[54]. Moreover, modern computational estimation techniques have overcome many of the earlier restrictions, so Thurstone’s model can be estimated in its full generality Citation[55]. Third, choice sets with more than two health states are in principle more informative (fewer choices are needed to yield the same amount of information). However, additional assumptions are required to apply such wider choice sets ([relaxed] IIA assumption [Appendix: E]). Some of these assumptions may be violated in assessing health states. Furthermore, recent studies from the EuroQol group provide evidence that using paired comparisons with the five-level classification system imposes significant cognitive burden on respondents, which might indicate that a choice task with three or more health states would become too difficult Citation[56]. Therefore, in the context of health-state valuations, it seems more convenient to restrict the applications to the standard paired comparison approach or ranking.
The Rasch model occupies a special position in the field of subjective measurement. Its underlying mathematical theory is a special form of the one-parameter IRT model. The Rasch model has a specific measurement property – quantifying independently the weight of the items (e.g., health domains) and the position of the respondents (e.g., a patient’s own health state) – that provides a criterion for fundamental measurement. This formal property distinguishes the Rasch model from other IRT models used to quantify peoples’ responses to items or questions Citation[57]. In contrast to IRT and other PC models, the Rasch model is the only model that allows for fundamental measurement, thus transforming subjective measurements into objective measurements. As such, the authors encourage the implementation of the Rasch model in the field of health-state valuation and other areas of health evaluation research.
Another topic that has gained attention in recent years is variance-scale heterogeneity Citation[58,59]. Random utility theory states that utilities can be decomposed into a systematic (predictable) component and a random (unpredictable) component. The most commonly applied DC models are limited dependent variable models, which confound estimates of the mean (the systematic component) and variance (scale factor) of the random component Citation[11]. One intuitive explanation of variance-scale heterogeneity is the confidence with which respondents answer in a DCE, although other explanations are also possible. Flynn et al. demonstrated that using latent-class analyses to identify variance-scale heterogeneity is a feasible method, and that using such results can lead to different (interpretation of) algorithms for certain subgroups. In the field of health-state valuation, the matter of variance-scale heterogeneity seems less relevant, as here the focus is on measurement and not on prediction of choice behavior, otherwise it is a matter of policy. In countries such as the UK a societal perspective is used, which means that the utility scale should represent the preferences of health states for the general population. In these instances, differences in subgroups seem irrelevant. However, a policy maker can only decide on the relevance of subgroups if he knows them to exist. Nevertheless, correctly identifying relevant subgroups is a challenging task for any researcher and no DC model is suited to give a definitive explanation of heterogeneity.
Limitations of DC models
As mentioned previously, DC models estimate the relative contribution of attributes and attribute levels. However, in many situations, the health-state values need to be anchored on the quality-adjusted life years (QALY) scale, where 0 is death and 1 is full health. There are difficulties with estimating the health-state ‘death’ in DC experiments. McCabe et al. Citation[3] and Salomon Citation[42] both proposed solutions where the state ‘dead’ is mixed in the choice set. This way a parameter for the state ‘dead’ is estimated as part of the model. However, Flynn et al. Citation[60] notes that estimated values are likely to be incorrectly anchored when assumptions about the decisions between living states and death are not satisfied. When there is a significant proportion of the sample that regards all life worth living (e.g., because of religious beliefs) this is likely to be true. Additionally, not only is the sample of influence, but so is the classification system that is being valued. For example, when a disease-specific classification system is being valued, one expects the range of total health states to be limited. Ratcliffe et al. performed a valuation study on the sexual quality of life questionnaire (SQOL-3D) Citation[5]. They found that all respondents found the worst health state to be better than death (using the TTO). They did not include death in their DCE, but had they done so, it would have been likely that all respondents would prefer the SQOL-3D health states to death. Such assumptions of the random utility model might be violated and thus decrease the validity of the results.
Response tasks
Some differences emerge in the simulated behavioral process and in the amount and type of information provided by each response task. In that light, experts have sought to clearly distinguish between rating, ranking and discrete choice tasks. Choice-based and non-choice-based techniques differ in that the former attempt to simulate human behavior in real-world situations. Only choice-based tasks have close links with economic theory Citation[8]. This argument is commonly used in marketing, as consumers in the real world are making actual choices between products instead of ordering or rating them.
With rating tasks, the respondents assign a value for each profile presented. Rating tasks do not inherently imply comparisons between alternatives and have therefore been criticized as being unrealistic. In contrast, the ranking approach does inherently imply making comparisons between a number of alternative options by ordering them according to the respondents’ own preferences. Nonetheless, a discrete choice task is considered to be much easier than a rating or ranking task (in general, presenting more alternatives to be ranked would correspond to greater difficulty in ordering them). However, a discrete choice task would require more respondents (or more choice tasks per respondent), since choosing one alternative over two or more others is less informative than providing ratings (or rankings) for each one.
A response task that combines both a discrete choice and an indication on the strength of the preference could provide more information per choice task. Such responses can be elicited by presenting respondents with two health states. The response options could vary from ‘definitely prefer A’ to ‘definitely prefer B’. These responses could be modeled with conditional ordinal logit or probit models. However, at least to our knowledge such models have thus far not been applied in the valuation of health states (but note Citation[46]). Nonetheless, these models appear very promising as they might require fewer respondents, or provide more precise estimates with an identical number of respondents. Careful consideration should be paid to the feasibility if researchers attempt to use such a methodology. Since the response task is extended, cognitive burden is likely to increase, which might also lead to more noise in the data.
Another response task that might be useful in the valuation of health states is best–worst scaling (BWS), also known as maximum difference scaling Citation[61]. There are three types of BWS, namely attribute BWS, profile BWS and attribute-level BWS. In attribute BWS, respondents choose the best and worst attributes from those available. An example of the attribute BWS is the study by Finn and Louviere Citation[62]. In such a response task, respondents select the best and worst attributes of those given. In the field of health-state valuation, such a task might provide relevant information for the selection of attributes for a classification system. In profile BWS, respondents are shown several profiles (health states of varying attribute levels) and have to indicate which profile they consider best and which one they consider worst. This task is very similar to a paired comparison with more than two profiles, although respondents provide more information since they also indicate which profile they consider worst. In the field of health-state valuation such a response task might be useful; however, if the classification system that is being valued consists of many attributes and levels, respondents might find this task too difficult to complete Citation[56]. The final type is the attribute-level BWS. In this response task, respondents are presented with a single profile (health state). They have to indicate which attribute level they consider to be the best, and which the worst attribute level. This type of BWS might prove to be very useful in the context of health-state valuation, since the amount of information respondents need to process seems lower than in a pair-wise comparison. Attribute-level BWS has been applied successfully in the field of health-state valuation Citation[50,63]; however, the reader is referred to Flynn et al. for some methodological considerations Citation[59,60,64].
To implement fundamental measurement of health states, the Rasch model needs to be applied. A response task that would allow this and make use of the advantages that DC experiments offer would appear as follows. Respondents first indicate their own health status on the instrument to be valued. Subsequently, they are confronted with a choice task that incorporates their own health state, and one or more health states that are expected to have a somewhat similar value to the respondent’s health state. Such a task would require computer adaptive testing, and it would only be feasible when patients are used instead of the general population Citation[32]. This is because the general population on average suffers from very few health limitations, and thus a respondent’s own health state would be dominant to almost all other health states. In addition, the patient sample needs to be heterogeneous; ideally, it should cover the entire health-state continuum.
Probabilistic choice models versus other methodologies
Assigning values to health states with probabilistic choice models that are based on response tasks, such as ranking of states or choices between states (e.g., paired comparisons), may be preferred to trade-off techniques, such as the SG and the TTO, which have been frequently applied to assign values to health states Citation[65–67]. For years, SG was considered the gold standard because it was developed under the expected utility theory by von Neumann and Morgenstern (vNM). However, as empirical research has shown, people’s behavior systematically violates the underlying assumptions of the vNM utilities. People have difficulty operating with probabilities, and they may be averse to taking risks Citation[68].
TTO was developed by Torrance et al. as an alternative to SG that would be easier to administer. The main characteristic of TTO is that it collapses into one single measure of the relationship between a health state, its duration and its value. However, there is some doubt about the validity of the assumption in the TTO method, which states that people can trade off a constant proportion of their remaining duration of life irrespective of the number of years that remain. In fact, this technique assumes that the amount of time an individual is willing to give up to be in a given health state is independent of the time horizon of that state. Research has shown that the value of health depends on the time spent in a certain health state. For states better than death, a longer duration may be preferred over a shorter duration, while the opposite may hold for states worse than death Citation[69]. In addition, certain states better than death may be considered worse than death as the amount of time in such states increases. This implies more complex relationships than the standard linear relationship of duration that is assumed with TTO.
Some researchers argue that both SG and TTO response tasks might be regarded as a series of DC experiments since respondents make a choice between two health states Citation[52] (however, note that it is long and well known that iterative tasks of this type are subject to starting point bias – e.g., Citation[70] – and that most DCE researchers would generally not agree with such a characterization). For example, consider a TTO in which a respondent is asked to choose between 10 years in health state A, or 8 years in full health. This single question might be regarded as a paired comparison. Suppose the respondent chooses 8 years in full health, so the TTO continues and produces another scenario, 10 years in health state A, or 4 years in full health. Again, this question can be regarded as a paired comparison. Suppose now that the respondent indicates to be indifferent between the two health states. Since both SG and TTO are iterative tasks until a point of indifference is found, each health state utility is measured by a series of paired comparisons (in the example above only two). The authors would like to stress, however, that these tasks contain factors extraneous to health (such as risk aversion in SG and time preference in TTO). Therefore, the scales that such techniques produce might include dimensions other than health and might thus not be unidimensional (as is required in the QALY framework). Nonetheless, modeling TTO and SG tasks as PC models might improve the health-state values Citation[52]. In both SG and TTO only a single (impaired) health state is valued. As such, these response tasks could be regarded as monadic response tasks. The DC experiments using pairwise or multiple comparisons have simultaneous assessment of multiple health states. This puts constraints on the possible biases that are associated with monadic measurement approaches. One recent study attempted to compare the TTO technique with a DCE that includes a time attribute Citation[71]. The authors showed that adding a time attribute to a health state is feasible for eliciting health-state values; however, more research is needed to verify that the constant proportional TTO in such a DCE is not violated.
Whereas visual aids and face-to-face interviews may be necessary in the application of SG and TTO Citation[72], the VAS technique can easily be self-administered. Furthermore, there are some similarities between the VAS and the ranking or the discrete choice tasks, but only when respondents position a number of health states simultaneously on a single scale (multi-item VAS). Then they are implicitly comparing health states and making decisions about which ones are preferable Citation[73,74]. For this reason, the multi-item VAS may be regarded as a compound task of multiple paired comparisons for (discrete) choices supplemented with a level of rating. However, the above is not applicable to the single-item VAS, which has some methodological flaws; in particular, it is prone to end-aversion and context bias. Furthermore, it is not embedded in a clear underlying theoretical measurement framework Citation[75–78]. In addition, the anchors in these scales are potentially ambiguous or not noticed at all by respondents Citation[79,80].
It seems likely that the multi-item VAS, Thurstone scaling, and the DC models will produce almost identical results. The DC experiments, however, prove to have an advantage over VAS: the former may eliminate any context bias that might occur in the VAS Citation[73,78]. Nevertheless, systematic comparisons between health state values derived with DC models and other elicitation techniques are rarely made Citation[81]. To explore the possible benefits of ‘modern’ methods such as discrete choice modeling in valuing health states, initial attempts have been made to compare these various methodologies Citation[48,51].
Expert commentary
PC models have become a focal point of attention and work in the area of health evaluation, especially in health economics. In particular, there has been rapid growth in the use of DC models to derive values by trading off between attributes of different natures (e.g., health outcomes vs process attributes) with respect to potential competing goods or services. Modeling individuals’ preferences with the current repertoire of techniques and instruments has often been found to be difficult. This has encouraged researchers to develop and make available more sophisticated statistical models and programs. Recent work considers PC models as potentially straightforward means to assess health-state values. PC models are now the object of investigations comparing their properties with those of more widely applied techniques for health-state valuation.
Five-year view
Current research on health-state valuation focuses on the comparison between PC models and widely applied techniques such as SG and TTO. The latter techniques are associated with biases that are caused by the elicitation technique. Choice experiments offer a great alternative as elicitation techniques since these are less associated with known biases. The largest body of research is predominantly being published by health economists and econometricians. To a smaller extent, researchers with a background in psychology or psychometrics are involved in this field. The authors argue that both of these disciplines should strive to keep up to date with one another. There have been a great many advances in choice modeling by econometricians that might be unknown to many psychologists/psychometricians. Similarly, many econometricians might fail to appreciate the value of psychometric innovations. There are very interesting ongoing attempts to arrive at a generalized measurement framework that incorporates many distinct analytical techniques to quantify subjective phenomena, such as factor analysis Citation[82], multidimensional scaling or unfolding techniques Citation[36,83,84], Rasch analysis and item response theory Citation[26,85,86], and structural equation modeling Citation[54,87]. All of these techniques have in common that they try to scale a (latent) trait or construct. This implies that all of these techniques could in principle be used to measure the values of health states. Although it is currently unknown whether these techniques can be embedded in the random utility framework, future studies might discover the assumptions under which these techniques can be incorporated in random utility theory. The authors hope that these methodologies will be identified by more researchers as valid alternatives for the current health-state valuation techniques. The combination of these methodologies may eventually lead to a dynamic concept of health status, where respondents themselves decide the most relevant attributes. Such methodologies would make the most use of individual variability in preferences and aggregate them to values usable for the estimation of QALYs.
Table 1. Overview of techniques and probabilistic choice models for the measurements of health-state values.
Key issues
• Probabilistic choice models have been identified as an alternative method for the estimation of health-state values.
• Many different models are available; researchers should carefully consider the assumptions, advantages and disadvantages of each particular model.
• Different models require different response tasks. Researchers should carefully consider the effect of the response tasks on respondents’ cognitive burden.
• Contemporary research focuses on the comparison between probabilistic choice models and techniques such as standard gamble and time trade-off.
• Future research should focus on extending the scope of health-state valuation by investigating methodologies such as item response theory and multidimensional scaling.
Appendix
A. Law of comparative judgment
Thurstone Citation[1] proposed that perceived physical phenomena or subjective concepts (e.g., health states, treatment outcomes and process characteristics) can be expressed as follows:
where θi is the true weight of an object (e.g., item, stimulus, health state) i, α is the measurable component of that weight for the object i, and ϵ is a random error term. The assumption in the model proposed by Thurstone is that ϵ is normally distributed. This assumption yields the binomial probit model.
In Thurstone’s terminology, choices are mediated by a ‘discriminal process’. He defined this as the process by which an organism identifies, distinguishes or reacts to stimuli. Consider the theoretical distributions of the discriminal process for any two objects, like two different health states i and j. In the LCJ model, the standard deviation of the distribution associated with a given health state is called the discriminal dispersion of that health state. Discriminal dispersions may be different for different health states.
Let θi and θj correspond to the scale values of the two health states. The difference θi – θj is measured in units of discriminal differences. This difference process, θi – θj = (αi − αj) + (ϵi − ϵj), is normally distributed with mean θi – θj and variance corresponding to
Thurstone stated that the relation between the difference in the means of what he called the discriminable process, θi – θj, the z score of the probability of selecting the one object as larger (better) than the other, and the variance and correlations of the random variables θi and θj can be modeled. This is known as the law of comparative judgment:
where θi, θj denotes the standard deviations of the two stimuli (health states) i and j, ρij denotes the correlation between the pairs of discriminal processes i and j, and zij is the unit normal deviate corresponding to the theoretical proportion of times health state j is judged greater than health state i. This basic form of the model can be represented as, θi – θj = zij, for which the probability that object j is judged to have more of an attribute than object i is
where ϕ is the cumulative normal distribution with mean zero and variance unity.
B. Bradley–Terry–Luce model
While the probit model (by Thurstone) has normally distributed error terms, a logit model is simply a log ratio of the probability of choosing a stimulus to the probability of not choosing a stimulus. If P is a probability, then P/(1 − P) is the corresponding odds, and the logit of the probability is the logarithm of the odds. The logit function is defined as the inverse of the logistic function. The logistic model is not linear, nor additive. Rather, it assumes an S-shaped response curve. One of the reasons the logit model was formulated was its’ ease of use. In comparison, probit models require the computation of integrals, which is why these models were less often used in the past. Modern computing however has made this computation fairly simple. The main difference between the logit and probit models lies on the distributional assumption of the error term. Consequently, the weighting of the cumulative probabilistic curve is different, as the logistic distribution tends to be a little flat tailed. The coefficients obtained with these two models are actually fairly close in most cases.
In the Bradley–Terry–Luce model Citation[2,3], the probability that object i is judged to have more of an attribute than object j is:
where θi and θj are respectively the scale values or weights of the two objects.
C. Rasch model
In the Rasch model for dichotomous data Citation[4], the probability that the outcome is correct (or better than another) is given by:
where ηn identifies a characteristic of the person n, as for instance his or her ability or the quality of his or her health status, and θi refers to the item i, as for instance the difficulty of an item (or seriousness of a health state). By an interactive conditional maximum likelihood estimation approach, an estimate θi − θj is obtained without involvement of η, which is specific to the Rasch model. This estimation approach leads to invariance: a fundamental aspect of measurement Citation[5].
D. Multinomial logit models versus conditional multinomial logit models
There is much confusion in the literature about the differences and similarities between multinomial and conditional logit models. The authors have contacted several experts in this field of research and received almost as many different explanations as experts approached. Multinomial logistic and conditional (multinomial) logistic regression models are different but often the terminology to describe the model is used differently or incorrectly. In fact, the term multinomial logit is quite confusing because different fields and people use it to refer to different things. The term conditional logit unfortunately includes a wide array of sub-models that depend on whether certain effects of interest are generic or differ for at least one of the choice alternatives.
The term multinomial logit (MNL) model refers to a regression logit model that generalizes logistic regression by allowing more than two discrete outcomes. This model assumes that data are case specific; that is, each independent variable has a single value for each case. Consider an individual choosing among K alternatives (e.g., health states) in a choice set. Let Xj represent the characteristics of individual j and βk the regression parameters (each of which is different, even though Xj is constant across alternatives):
Let Pjk denote the probability that individual j chooses alternative k. The probability that individual j chooses alternative k is
It is important to clarify some terminology. The model mentioned and used for behavioral modeling of polytomous choice situations, developed by McFadden Citation[6], is generally called MNL. Yet some important distinctions have to be made between the (conventional) MNL model and the conditional MNL model. Although the McFadden model is often simply referred to as the MNL model, this refers to the conditional model. In conditional logistic regression, none, some or all of the observations in a choice set may be labeled. Thus, McFadden’s choice model (discrete choice) is a special case of conditional logistic regression (conditional logistic analysis is also applied in epidemiology when analyzing matched case control data). In the conditional logit model, θ is a single vector of regression coefficients; the explanatory variables Z assume different values for each alternative; and the impact of a unit of Z is assumed to be constant across alternatives:
The probability that the individual j chooses alternative k is
Both models can be used to analyze the choice of an individual among a set of K alternatives. The central difference between the two is that the conventional MNL model focuses on the individual as the unit of analysis and uses the individual’s characteristics (e.g., gender, age, religion) as explanatory variables. In contrast, the conditional MNL model focuses on the set of alternatives for each individual and the explanatory variable comprises characteristics of those alternatives. This is the typical mechanism (see ) that seems required in the case of measurement (health-state valuation), whereas the conventional MNL model is used for the prediction of choice behavior. It is possible to combine the two models to simultaneously take into account both the alternatives’ and the individual’s characteristics as explanatory variables. This is called a mixed-logit model:
Where Ujk is the utility of the alternative k assigned by the individual j. Ujk depends on both the alternatives’ characteristics X and on the individuals’ characteristics Z, plus a nonestimable part represented by ϵ.
In addition to the mixed-logit model (where ‘mixed’ refers to characteristics), where both respondents’ and stimuli characteristics are being taken into account, an even more general model is the logit-mixture model (where ‘mixture’ refers to the distributions of error terms). This model also takes individual taste variation into account, by partitioning the error term in a random part (or any other type of distribution) and an extreme value part. The model has the following form:
where βxjk is the systematic component of the utility (which can include both respondent and attribute characteristics) and µjzjk and ϵjk are error terms; µ is a vector of random terms with a mean of zero (or of any other distribution than the normal distribution) and ϵjk is IID and has an extreme value type 1 distribution. The component µjzjk allows for the induction of heteroscedasticity and correlation across the random part of the utility of the different alternatives in the choice set. It is this model that in the literature is most often referred to as the mixed-logit model. As stated before, these types of models, with a component directed on the prediction of respondent characteristics, are less valuable in the case of the measurement (valuation) of health states, but of course, may be very relevant for evaluation research in general.
Interestingly, the conditional multinomial logistic model could be extended to analyze ordinal preferences. Accordingly, it is conceivable that rank orderings can be generated by a process in which an individual first chooses his most preferred alternative from all available alternatives. From the remaining alternatives he again chooses his most preferred one – thereby stating his second preference – and so on, until there is only one remaining alternative, which is, of course, his last preference. Thus, an observed preference order can be understood as being generated by a repeated selection process in which the best alternative is always chosen and subsequently deleted from the choice set. The later decisions are assumed to be independent of the previous ones, which is to say that IIA holds. This model is also called ‘conditional logit’, ‘exploded logit’ or ‘rank-ordered logit’, as the ranking of K states is exploded into K − 1 decision stages (see ). The contribution of using a rank-ordered logit model is that more information is incorporated in the estimation of the representative function compared with the standard logit models.
E. Independence of irrelevant alternatives
The IIA property, which arises from the assumption of independent random errors and equal variances for the choice alternatives (IID assumption), implies that the odds of choosing one alternative over another must be constant regardless of whatever other alternatives are present Citation[3]. To give an example put forward by Debreu Citation[7] where IIA does not hold: suppose an individual wants to buy a CD, and she is equally likely to choose a Beethoven or a Debussy recording (Pr{B|B, D} = Pr{D|B, D} = 0.5). Now suppose that she encounters a second Beethoven recording that she likes just as much as the first (Pr{B1|B1, B2} = Pr{B2 |B1, B2} = 0.5). If she were rational, how would she choose among all three recordings {B1, B2, D}? We would expect Pr{B1|B1, B2, D} = 0.25, Pr{B2|B1, B2, D} = 0.25 and Pr{D|B1, B2, D} = 0.5. However, IIA implies that Pr{B1|B1, B2, D} = 1/3, Pr{B2|B1, B2, D} = 1/3, and Pr{D|B1, B2, D} = 1/3 (in this context this makes perfect sense, as a second Beethoven recording is unlikely to be irrelevant from the first). This IIA assumption may be too restrictive in practical situations can be unrealistic in many settings. The outcomes that could theoretically violate IIA (such as the outcome of multicandidate elections, or according to Arrow Citation[8] any choice made by humans) may make conditional MNL an invalid estimator. Nonetheless, when IIA reflects reality, it offers many advantages, but whether IIA holds in a particular setting is an empirical question amenable to statistical investigation. There seems to be ample scope for research aimed at developing models that allow for managing contexts where IIA may not hold. Some models such as the logit-mixture relaxed the assumption of IIA. This means that these models can allow for random taste variation, correlations in unobserved factors over time and unrestricted substitution patterns. McFadden and Train showed that given an appropriate specification of variables and distribution of coefficients, a logit-mixture can approximate to any degree of accuracy any true random utility model of discrete choice Citation[9].
References
- Thurstone LL. A law of comparative judgment (Reprinted from Psychological Review, Vol 34, Pg 273, 1927). Psychol. Rev. 101(2), 266–270 (1994).
- Bradley RA, Terry ME. Rank analysis of incomplete block designs.1. The method of paired comparisons. Biometrika 39(3–4), 324–345 (1952).
- Luce RD. Individual Choice Behavior: a Theoretical Analysis. Wiley, New York, NY, USA (1959).
- Rasch G. An item analysis which takes individual differences into account. Br. J. Math. Stat. Psychol. 19(1), 49–57 (1966).
- Engelhard G. Historical views of invariance – evidence from the measurement theories of Thorndike, Thurstone, and Rasch. Educ. Psychol. Meas. 52(2), 275–291 (1992).
- McFadden D. Conditional logit analysis of qualitative choice behavior. In: Frontiers in Econometrics. Zarembka P. Academic Press, San Diego, CA, USA, 105–142 (1974).
- Debreu G. Review of R.D. Luce’s individual choice behavior: a theoretical analysis. Am. Econ. Rev. 50, 186–188 (1960).
- Arrow K. Social Choice and Individual Values, Second edition (Cowles Foundation Monographs Series). Yale University Press, New Haven, CT, USA (1970).
- McFadden D, Train K. Mixed MNL models for discrete response. J. Appl. Econom. 15(5), 447–470 (2000).
Acknowledgement
We would like to thank four anonymous reviewers for their insightful and excellent suggestions on an earlier draft of this manuscript.
Financial and competing interests disclosure
The authors have no relevant affiliations or financial involvement with any organization or entity with a financial interest in or financial conflict with the subject matter or materials discussed in the manuscript apart from those disclosed.
No writing assistance was utilized in the production of this manuscript.
References
- Ryan M, Bate A, Eastmond CJ, Ludbrook A. Use of discrete choice experiments to elicit preferences. Qual. Health Care 10(Suppl. 1), i55–i60 (2001).
- Hakim Z, Pathak DS. Modelling the EuroQol data: a comparison of discrete choice conjoint and conditional preference modelling. Health Econ. 8(2), 103–116 (1999).
- McCabe C, Brazier J, Gilks P et al. Using rank data to estimate health state utility models. J. Health Econ. 25(3), 418–431 (2006).
- McKenzie L, Cairns J, Osman L. Symptom-based outcome measures for asthma: the use of discrete choice methods to assess patient preferences. Health Policy 57(3), 193–204 (2001).
- Ratcliffe J, Brazier J, Tsuchiya A, Symonds T, Brown M. Using DCE and ranking data to estimate cardinal values for health states for deriving a preference-based single index from the sexual quality of life questionnaire. Health Econ. 18(11), 1261–1276 (2009).
- Ryan M, Netten A, Skåtun D, Smith P. Using discrete choice experiments to estimate a preference-based measure of outcome – an application to social care for older people. J. Health Econ. 25(5), 927–944 (2006).
- Szeinbach SL, Barnes JH, McGhan WF, Murawski MM, Corey R. Using conjoint analysis to evaluate health state preferences. Drug Inf. J. 33(3), 849–858 (1999).
- Louviere JJ, Hensher DA, Swait JD. Stated Choice Methods. Cambridge University press, Cambridge, UK (2000).
- Ryan M, Gerard K, Amaya-Amaya M. Using Discrete Choice Experiments to Value Health and Health Care. Springer Academic Publishers, Berlin, Germany (2008).
- McFadden D. Conditional logit analysis of qualitative choice behavior. In: Frontiers in Econometrics. Zarembka P. Academic Press, San Diego, CA, USA, 105–142 (1974).
- Ben-Akiva ME, Lerman SR. Discrete Choice Analysis: Theory and Application to Travel Demand. MIT Press, Cambridge, UK (1985).
- Bridges JF, Hauber AB, Marshall D et al. Conjoint analysis applications in health – a checklist: a report of the ISPOR Good Research Practices for Conjoint Analysis Task Force. Value Health 14(4), 403–413 (2011).
- Cunningham CE, Deal K, Rimas H, Chen Y, Buchanan DH, Sdao-Jarvie K. Providing information to parents of children with mental health problems: a discrete choice conjoint analysis of professional preferences. J. Abnorm. Child Psychol. 37(8), 1089–1102 (2009).
- Hall J, Viney R, Haas M, Louviere J. Using stated preference discrete choice modeling to evaluate health care programs. J. Bus. Res. 57(9), 1026–1032 (2004).
- Swait JD. Choice models based on mixed discrete/continuous PDFs. Transport. Res. Part B Meth. 43(7), 766–783 (2009).
- Thurstone LL. A law of comparative judgment (Reprinted from Psychological Review, Vol 34, Pg 273, 1927). Psychol. Rev. 101(2), 266–270 (1994).
- Stevens SS. On the psychophysical law. Psychol. Rev. 64(3), 153–181 (1957).
- Bradley RA, Terry ME. Rank analysis of incomplete block designs.1. The method of paired comparisons. Biometrika 39(3–4), 324–345 (1952).
- Luce RD. Individual Choice Behavior: a Theoretical Analysis. Wiley, New York, NY, USA (1959).
- Kind P. A comparison of two models for scaling health indicators. Int. J. Epidemiol. 11(3), 271–275 (1982).
- Luce RD, Suppes P. Preference, utility, and subjective probability. In: Handbook of mathematical psychology. Luce RD, Bush RR, Galanter E (Eds). John Wiley & Sons, New York, NY, USA, 235–406 (1965).
- Luce RD, Tukey JW. Simultaneous conjoint-measurement – a new type of fundamental measurement. J. Math. Psychol. 1(1), 1–27 (1964).
- Perline R, Wright BD, Wainer H. The Rasch model as additive conjoint measurement. Appl. Psychol. Meas. 3(2), 237–255 (1979).
- Maas A, Stalpers L. Assessing utilities by means of conjoint measurement: an application in medical decision analysis. Med. Decis. Making 12(4), 288–297 (1992).
- Stalmeier PF, Bezembinder TG, Unic IJ. Proportional heuristics in time tradeoff and conjoint measurement. Med. Decis. Making 16(1), 36–44 (1996).
- Rasch G. An item analysis which takes individual differences into account. Br. J. Math. Stat. Psychol. 19(1), 49–57 (1966).
- Holman R, Weisscher N, Glas CA et al. The Academic Medical Center Linear Disability Score (ALDS) item bank: item response theory analysis in a mixed patient population. Health Qual. Life Outcomes 3, 83 (2005).
- Schultz-Larsen K, Kreiner S, Lomholt RK. Mini-Mental Status Examination: mixed Rasch model item analysis derived two different cognitive dimensions of the MMSE. J. Clin. Epidemiol. 60(3), 268–279 (2007).
- Brazier J, Czoski-Murray C, Roberts J, Brown M, Symonds T, Kelleher C. Estimation of a preference-based index from a condition-specific measure: the King’s Health Questionnaire. Med. Decis. Making 28(1), 113–126 (2008).
- Young T, Yang Y, Brazier JE, Tsuchiya A, Coyne K. The first stage of developing preference-based measures: constructing a health-state classification using Rasch analysis. Qual. Life Res. 18(2), 253–265 (2009).
- Young TA, Yang Y, Brazier JE, Tsuchiya A. The use of rasch analysis in reducing a large condition-specific instrument for preference valuation: the case of moving from AQLQ to AQL-5D. Med. Decis. Making 31(1), 195–210 (2011).
- Krabbe PFM. A generalized measurement model to quantify health: the multi-attribute preference response model. Proceedings of the 29th Plenary Meeting of the EuroQol Group, Conference Paper. Rotterdam, The Netherlands, 13–14 September 2012.
- Brogden HE. Rasch model, law of comparative judgment and additive conjoint measurement. Psychometrika 42(4), 631–634 (1977).
- Andrich D. Relationships between the Thurstone and Rasch approaches to item scaling. Appl. Psychol. Meas. 2(3), 451–462 (1978).
- Guttman L. The basis for Scalogram analysis. In: Measurement & Prediction, The American Soldier. Stouffer SA, Suchman A, Leland C et al. (Eds). Wiley, NY, USA (1950).
- Coombs CH. A Theory of Data. John Wiley & Sons, NY, USA (1964).
- Green PE, Rao VR. Conjoint measurement for quantifying judgmental data. J. Market. Res. 8(3), 355–363 (1971).
- Louviere JJ, Flynn TN, Carson RT. Discrete choice experiments are not conjoint analysis. J. Choice Model. 3(3), 57–72 (2010).
- Marschak J. Binary-choice constraints and random utility indicators. In: Mathematical Methods in the Social Sciences. Arrow K, Karlin S, Suppes P (Eds). Stanford University Press, Stanford, Germany, 312–329 (1960).
- McFadden D. Econometric models for probabilistic choice among products. J. Bus. 53(3), S13–S29 (1980).
- McFadden D. Economic choices. Am .Econ. Rev. 91(3), 351–378 (2001).
- Salomon JA. Reconsidering the use of rankings in the valuation of health states: a model for estimating cardinal values from ordinal data. Popul. Health Metr. 1(1), 12 (2003).
- Lancaster KJ. A new approach to consumer theory. J. Politic. Econ. 74, 132–157 (1966).
- Louviere JJ, Woodworth G. Design and analysis of simulated consumer choice or allocation experiments – an approach based on aggregate data. J. Market. Res. 20(4), 350–367 (1983).
- Fanshel S, Bush JW. A health-status index and its application to health-services outcomes. Oper. Res. 18(6), 1021–1066 (1970).
- Hadorn DC, Hays RD, Uebersax J, Hauber T. Improving task comprehension in the measurement of health state preferences. A trial of informational cartoon figures and a paired-comparison task. J. Clin. Epidemiol. 45(3), 233–243 (1992).
- Krabbe PF. Thurstone scaling as a measurement method to quantify subjective health outcomes. Med. Care 46(4), 357–365 (2008).
- Stolk EA, Oppe M, Scalone L, Krabbe PF. Discrete choice modeling for the quantification of health states: the case of the EQ-5D. Value Health 13(8), 1005–1013 (2010).
- Rabin R, de Charro F. EQ-5D: a measure of health status from the EuroQol Group. Ann. Med. 33(5), 337–343 (2001).
- Coast J, Flynn TN, Natarajan L et al. Valuing the ICECAP capability index for older people. Soc. Sci. Med. 67(5), 874–882 (2008).
- Brazier J, Rowen D, Yang Y, Tsuchiya A. Comparison of health state utility values derived using time trade-off, rank and discrete choice data anchored on the full health–dead scale. Eur. J. Health Econ. 13(5), 575–587 (2012).
- Craig BM, Busschbach JJ. The episodic random utility model unifies time trade-off and discrete choice approaches in health state valuation. Popul. Health Metr. 7, 3 (2009).
- Craig BM, Busschbach J, Salomon JA. Ordinal valuation of health states: a seven country comparison. Proceedings of the EuroQol plenary meeting. Barcelona, Spain September 14–16 2006
- Maydeu-Olivares A, Böckenholt U. Structural equation modeling of paired-comparison and ranking data. Psychol. Methods 10(3), 285–304 (2005).
- Maydeu-Olivares A, Böckenholt U. Modeling subjective health outcomes: top 10 reasons to use Thurstone’s method. Med. Care 46(4), 346–348 (2008).
- Xie F, Pullenayegum E, Gaebel K, Oppe M, Krabbe PFM. Eliciting preferences to the EQ-5D-5L health states: discrete choice experiment or multiprofile case of best–worst scaling. Value Health 15(4), A198–A199 (2012).
- Andrich D. Controversy and the Rasch model: a characteristic of incompatible paradigms? Med. Care 42(1 Suppl.), I7–16 (2004).
- Flynn TN, Louviere JJ, Peters TJ, Coast J. Using discrete choice experiments to understand preferences for quality of life. Variance-scale heterogeneity matters. Soc. Sci. Med. 70(12), 1957–1965 (2010).
- Flynn TN, Louviere JJ, Peters TJ, Coast J. Best–worst scaling: what it can do for health care research and how to do it. J. Health Econ. 26(1), 171–189 (2007).
- Flynn TN, Louviere JJ, Marley AA, Coast J, Peters TJ. Rescaling quality of life values from discrete choice experiments for use as QALYs: a cautionary tale. Popul. Health Metr. 6, 6 (2008).
- Marley AAJ, Louviere JJ. Some probabilistic models of best, worst, and best–worst choices. J. Math. Psychol. 49(6), 464–480 (2005).
- Finn A, Louviere JJ. Determining the appropriate response to evidence of public concern: the case of food safety. J. Public Policy Market 11, 12–25 (1992).
- Ratcliffe J, Couzner L, Flynn T et al. Valuing Child Health Utility 9D health states with a young adolescent sample: a feasibility study to compare best–worst scaling discrete-choice experiment, standard gamble and time trade-off methods. Appl. Health Econ. Health Policy 9(1), 15–27 (2011).
- Flynn TN. Valuing citizen and patient preferences in health: recent developments in three types of best-worst scaling. Expert Rev. Pharmacoecon. Outcomes Res. 10(3), 259–267 (2010).
- Bleichrodt H, Johannesson M. The validity of QALYs: an experimental test of constant proportional tradeoff and utility independence. Med. Decis. Making 17(1), 21–32 (1997).
- Nord E. Methods for quality adjustment of life years. Soc. Sci. Med. 34(5), 559–569 (1992).
- Froberg DG, Kane RL. Methodology for measuring health-state preferences – II: Scaling methods. J. Clin. Epidemiol. 42(5), 459–471 (1989).
- Bleichrodt H. A new explanation for the difference between time trade-off utilities and standard gamble utilities. Health Econ. 11(5), 447–456 (2002).
- Stalmeier PF, Lamers LM, Busschbach JJ, Krabbe PF. On the assessment of preferences for health and duration: maximal endurable time and better than dead preferences. Med. Care 45(9), 835–841 (2007).
- Boyle KJ, Bishop RC, Welsh MP. Starting point bias in contingent valuation bidding games. Land Econ. 61(2), 188–194 (1985).
- Bansback N, Brazier J, Tsuchiya A, Anis A. Using a discrete choice experiment to estimate health state utility values. J. Health Econ. 31(1), 306–318 (2012).
- Oppe M, Devlin N, Van Hout B, Krabbe PFM, De Charro FTH. EuroQol Group’s international protocol for the valuation of the EQ-5D-5L. Presented at: 29th Plenary Meeting of the EuroQol Group. Rotterdam, The Netherlands, September 13–14 2012.
- Krabbe PF, Stalmeier PF, Lamers LM, Busschbach JJ. Testing the interval-level measurement property of multi-item visual analogue scales. Qual. Life Res. 15(10), 1651–1661 (2006).
- Parkin D, Devlin N. Is there a case for using visual analogue scale valuations in cost–utility analysis? Health Econ. 15(7), 653–664 (2006).
- Robinson A, Loomes G, Jones-Lee M. Visual analog scales, standard gambles, and relative risk aversion. Med. Decis. Making 21(1), 17–27 (2001).
- Schwartz A. Rating scales in context. Med. Decis. Making 18(2), 236 (1998).
- Torrance GW, Feeny D, Furlong W. Visual analog scales: do they have a role in the measurement of preferences for health states? Med. Decis. Making 21(4), 329–334 (2001).
- Bleichrodt H, Johannesson M. An experimental test of a theoretical foundation for rating-scale valuations. Med. Decis. Making 17(2), 208–216 (1997).
- McPhail S, Beller E, Haines T. Reference bias: presentation of extreme health states prior to EQ-VAS improves health-related quality of life scores. A randomised cross-over trial. Health Qual. Life Outcomes 8, 146 (2010).
- Ubel PA, Jankovic A, Smith D, Langa KM, Fagerlin A. What is perfect health to an 85-year-old? Evidence for scale recalibration in subjective health ratings. Med. Care 43(10), 1054–1057 (2005).
- Bryan S, Dolan P. Discrete choice experiments in health economics. For better or for worse? Eur. J. Health Econ. 5(3), 199–202 (2004).
- Nunnally JC, Bernstein IH. Psychometric Theory. McGraw-Hill, New York, NY, USA (1994).
- Krabbe PF, Salomon JA, Murray CJ. Quantification of health states with rank-based nonmetric multidimensional scaling. Med. Decis. Making 27(4), 395–405 (2007).
- Torgerson WS. Theory and Methods of Scaling. Oxford, England (1958).
- De Ayala RJ. The Theory and Practice of Item Response Theory. Guilford Publications, New York, NY, USA (2008).
- Lord FM. Applications of Item Response Theory to Practical Testing Problems. Erlbaum Associates, Abingdon, UK (1980).
- Maydeu-Olivares A. Thurstonian modeling of ranking data via mean and covariance structure analysis. Psychometrika 64(3), 325–340 (1999).
- Engelhard G. Historical views of invariance – evidence from the measurement theories of Thorndike, Thurstone, and Rasch. Educ. Psychol. Meas. 52(2), 275–291 (1992).
- Debreu G. Review of R.D. Luce’s individual choice behavior: a theoretical analysis. Am. Econ. Rev. 50, 186–188 (1960).
- Arrow K. Social Choice and Individual Values, Second edition (Cowles Foundation Monographs Series). Yale University Press, New Haven, CT, USA (1970).
- McFadden D, Train K. Mixed MNL models for discrete response. J. Appl. Econom. 15(5), 447–470 (2000).