Abstract
Background
Ferroptosis is a type of cell death characterized by the accumulation of iron-dependent lethal lipid peroxides, which is associated with various pathophysiological processes. Psoriasis is a chronic autoimmune skin disease accompanied by abnormal immune cell infiltration and excessive production of lipid reactive oxygen species (ROS). Currently, its pathogenesis remains elusive, especially the potential role of ferroptosis in its pathophysiological process.
Methods
The microarrays GSE13355 (58 psoriatic skin specimens versus 122 healthy skin specimens) and the ferroptosis database were employed to identify the common differentially expressed genes (DEGs) associated with psoriasis and ferroptosis. The functions of common DEGs were investigated through functional enrichment analysis and protein-protein interaction analysis. The potential diagnostic markers for psoriasis among the common DEGs were identified using four machine-learning algorithms. DGIdb was utilized to explore potential therapeutic agents for psoriasis. Additionally, CIBERSORT was employed to investigate immune infiltration in psoriasis.
Results
A total of 8 common DEGs associated with psoriasis and ferroptosis were identified, which are involved in intercellular signaling and affect pathways of cell response to stress and stimulation. Four machine-learning algorithms were employed to identify poly (ADP-ribose) polymerase 12 (PARP12), frizzled homolog 7 (FZD7), and arachidonate 15-lipoxygenase (ALOX15B) among the eight common DEGs as potential diagnostic markers for psoriasis. A total of 18 drugs targeting the five common DEGs were identified as potential candidates for treating psoriasis. Additionally, significant changes were observed in the immune microenvironment of patients with psoriasis.
Conclusion
This study has contributed to our enhanced comprehension of ferroptosis-related genes as potential biomarkers for psoriasis diagnosis, as well as the alterations in the immune microenvironment associated with psoriasis. Our findings offer valuable insights into the diagnosis and treatment of psoriasis.
Keywords:
Introduction
Psoriasis is a prevalent chronic disease affecting approximately 2% of the general population, characterized by its incurable nature. It can induce pain, disrupt sleep patterns, and impair concentration abilities. The presence of psoriatic disease has been significantly associated with elevated levels of circulating inflammatory molecules.Citation1 Furthermore, the inflammation associated with psoriasis not only causes damage to other physiological systems but also contributes to increased mortality rates linked to cardiovascular diseases, infections, and cancers. In individuals with psoriasis, various immune cells (such as granulocytes, dendritic cells, and lymphocytes) exhibit excessive production of lipid reactive oxygen species (ROS) and lipid mediators.Citation2
Ferroptosis, a regulated iron-dependent type of cell death characterized by intracellular accumulation of lipid peroxides, has been extensively implicated in various diseases. A significant association between ferroptosis and chronic conditions, including cancer, neurological disorders, metabolic disorders, and inflammatory bowel diseases, has been well-documented.Citation3 Ferroptosis exerts dual effects on immune cells, influencing both their quantity and functionality. Moreover, immune cells possess the ability to recognize ferroptosis cells, subsequently eliciting a spectrum of inflammatory or specific responses. The activation of various signaling pathways associated with inflammation, such as Janus kinase-signaling transducer and activator of transcription (JAK-STAT), nuclear factor-κB (NF-κB), inflammasome, cyclic guanosine monophosphate-adenosine monophosphate synthase-stimulator of interferon genes (cGAS-STING), and mitogen-activated protein kinase (MAPK) signaling pathways, is closely linked to the occurrence of ferroptosis.Citation4
Bioinformatics approaches have been utilized to discern the differentially regulated genes and affected pathways in psoriasis; however, limited knowledge exists regarding the multitude of genes and signaling pathways implicated in psoriatic inflammation. Exploring the mechanisms of ferroptosis in psoriasis and elucidating the role of inflammation and immune regulation is crucial, given that specific molecules associated with ferroptosis have been discovered to enhance the inflammatory response in psoriasis.Citation5–7 However, there is still a lack of research on the key regulatory genes associated with ferroptosis in psoriasis. In this study, we explored the main biomarkers associated with ferroptosis and their correlation with immune cell infiltration in psoriasis, thus providing valuable insights for the diagnosis and drug development of psoriasis.
Materials and Methods
Study Design and Data Collection
Three datasets were retrieved from the Gene Expression Omnibus (GEO) database by conducting a search using ‘psoriasis’ as the keyword to identify relevant gene expression datasets. The selection criteria for dataset inclusion were as follows: 1) Availability of arrays containing both psoriasis and normal samples; 2) Inclusion of test specimens obtained from adult humans. Based on these selection criteria, three datasets, namely GSE13355 (58 psoriatic skin specimens versus 122 healthy skin specimens),Citation8 GSE30999 (85 psoriatic skin specimens versus 85 healthy skin specimens),Citation9 and GSE50790 (4 psoriatic skin specimens versus 4 healthy skin specimen),Citation10 were ultimately included in this study.
Identification of Differentially Expressed Genes with R Software
We obtained the series matrix files of datasets from GEO for subsequent analysis. To identify differentially expressed genes (DEGs) between healthy and patient samples in GSE13355, we employed R 3.4.1’s (https://www.r-project.org/) Affy and Limma package. The fold changes (FCs) were calculated to determine alterations in gene expression. The corresponding plots were made by R and the genes that followed the following criteria were regarded as DEGs: p < 0.05 and |logFC| > 0.3. An online Venn diagram tool available at http://bioinformatics.psb.ugent.be/webtools/Venn/ was utilized to identify DEGs.
Functional Annotation and Pathway Enrichment Analysis
To further reveal the potential functions of DEGs and their relationships with psoriasis, gene ontology (GO) enrichment analysis and Kyoto encyclopedia of genes and genomes (KEGG) functional network analysis were performed using the “clusterProfiler” package in R software. The GO terms encompass three components: biological process (BP), cellular component (CC), and molecular function (MF). To perform GO functional enrichment analysis and KEGG pathway enrichment, we utilized the Database for Annotation, Visualization, and Integrated Discovery (DAVID) online tool available at http://david.abcc.ncifcrf.gov/.Citation11 A significance threshold of p < 0.05 was applied as the cutoff criterion.
PPI Network Construction and Identification of Hub Genes
Based on the identified DEGs, protein-protein interaction (PPI) networks were constructed using the online Search Tool for the Retrieval of Interacting Genes (STRING) database (https://cn.string-db.org/) and visualized through Cytoscape 3.9.0 software. Reliable interactions were determined by setting intermediate confidence scores (>0.4). To identify hub genes, CytoHubba, a Cytoscape plug-in that evaluates nodes based on their network characteristics, was employed for exploring crucial nodes in biological networks.Citation12
Validation of Hub Gene Expression
The expression levels of the identified hub genes were validated in two independent datasets, namely GSE30999 and GSE50790, followed by a comparative analysis employing the Wilcoxon test. A significance level of p < 0.05 was considered statistically significant.
Identification of Potential Ferroptosis Biomarkers with Machine Learning
After conducting different expression analyses, four machine learning algorithms, namely random forest, support vector machines, LightGBM, and XGBoost, were employed for model construction. To mitigate overfitting risks, a ten-fold cross-validation was employed on both the training and validation sets to develop predictive models. The entire dataset was randomly divided into ten folds; nine of them were utilized as the training set for model development while one fold was reserved as the validation set for model validation. Given that each of the 10-folds served as the validation set, this process was iterated 10 times. Ultimately, the performance of each model was validated and compared using the validation set. The optimal model for each algorithm was selected based on the highest area under the curve (AUC) of the receiver operating characteristic (ROC) curve.
ROC Curves
The predictive accuracy of potential biomarker genes was evaluated by generating ROC curves using the pROC package in the R language.Citation13
Immune Infiltration Analysis
The CIBERSORT method is utilized to characterize the cellular composition of complex tissues based on their gene expression profiles. CIBERSORT has demonstrated superior performance compared to other techniques in quantifying hematopoietic subpopulations within RNA mixtures derived from fresh, frozen, and fixed tissues, including solid tumors. It offers several advantages such as noise reduction, handling of unknown mixture content, and distinguishing closely related cell types. Consequently, CIBERSORT facilitates large-scale analysis of cellular biomarkers and therapeutic targets within RNA mixtures. To further investigate alterations in immune cells within samples, the risk score and correlation between tumor metastasis and tumor cell invasion were analyzed using the CIBERSORT algorithm along with the “vioplot” package.
Statistical Analysis
All statistical analyses in this study were performed using R software (version 4.1.2; https://www.r-project.org/). A significance level of p < 0.05 was considered to indicate statistical significance.
Results
Identification of Common DEGs
Differential gene expression analysis was performed on both healthy and psoriasis patient samples obtained from GSE13355, resulting in the identification of 159 DEGs using the limma package (Supplementary Table 1). DEGs were selected based on a significance threshold of p < 0.05 and |logFC| > 0.1 criteria (). A dataset comprising 484 ferroptosis-related genes was obtained from the Ferroptosis database (FerrDb), which was then intersected with psoriasis-related DEGs to identify ferroptosis-related DEGs (called common DEGs). By taking the intersection of Venn diagrams, a total of 8 common DEGs including poly ADP-ribose polymerase 12 (PARP12), frizzled homolog 7 (FZD7), arachidonate 15-lipoxygenase type B (ALOX15B), DnaJ homolog subfamily B member 6 (DNAJB6), enhancer of zeste homolog 2 (EZH2), GTP cyclohydrolase 1 (GCH1), pannexin 1 (PANX1), and androgen receptor (AR) were identified (). Heat maps depicting these intersecting DEGs are shown in and Supplementary Table 1.
GO and KEGG Pathway Analyses
To further investigate the underlying biological information of these psoriasis-related DEGs as well as ferroptosis-related DEGs, the “cluster profiler” package in R software was employed to perform GO analysis and KEGG pathway enrichment. The results revealed that, in terms of molecular function, the genes were predominantly enriched in protein binding, cytokine activity, peptidase inhibitor activity, and purine nucleobase transmembrane transporter activity; in terms of cellular components, the genes exhibited significant enrichment in the extracellular region, extracellular exosome, endoplasmic reticulum, basolateral plasma membrane, and actin cytoskeleton; in terms of biological processes, the genes main enrichment in innate immune response, immune response, positive regulation of cell proliferation, cell adhesion, and positive regulation of cell migration regulation (). The pro-inflammatory cytokines possess the potential to facilitate the progression of psoriasis through immune pathways. This GO analysis has revealed significant alterations in the inflammatory response within psoriasis-associated skin tissue.
Figure 3 Pathway enrichment of differentially expressed genes. (A) The enrichment GO pathways of psoriasis-related DEGs. (B) The enrichment GO pathways of ferroptosis-related DEGs. (C) The enrichment reaction pathways of ferroptosis-related DEGs.

The pathways influenced by ferroptosis-related DEGs were also depicted in and . GO analysis revealed that these genes primarily function within the nucleus and macromolecular complexes, playing a crucial role in cell-cell signaling (). KEGG analysis revealed that ferroptosis-related DEGs were predominantly enriched in cellular responses to stimuli and cellular responses to stress (). These findings provided support for the concept that ferroptosis, a non-apoptotic and iron-dependent type of cell death, can be induced in diseased cells by both natural stimuli and synthetic agents. Oxidative damage of nuclear DNA serves as a biochemical indicator of ferroptosis. For instance, the translocation of lysosomal carpal tunnel syndrome (CTS) or mitochondrial apoptosis-inducing factor mitochondria-associated 1 (AIFM1) to the nucleus can induce local damage and trigger ferroptosis.Citation14,Citation15 Thus, protein translocation between nuclear and extranuclear compartments can influence cellular susceptibility to ferroptosis. Moreover, recent experimental observations have demonstrated that cell-cell contacts play a significant regulatory role in controlling ferroptosis.Citation16
PPI Network Construction of Common DEGs
To further reveal the potential protein-protein relationships encoded by common DEGs and identify hub genes, the PPI network of the DEGs was screened using STRING. The resulting network comprised 78 nodes and 254 edges, exhibiting a highly significant PPI enrichment (p < 1.0e-16, ). The top 10 genes involved in cellular interaction include cyclin D1 (CCND1), EZH2, Cell-division cycle protein 20 homolog (CDC20), epithelial cell adhesion molecule (EPCAM), ZW10 interactor (ZWINT), CD52, cyclin E2 (CCNE2), age and apolipoprotein E (APOE), and C-C motif ligand 2 (CCL2). Among these genes, CCND1, CDC20, and CCNE2 encode proteins belonging to the highly conserved cyclin family. These genes function sequentially to regulate the cell cycle and exhibit expression during G1/S/M phases. The mitotic cycle in psoriasis is dysregulated, resulting in the hyperproliferation of keratinocyte cells and acanthosis hypertrophy. As a result, the renewal time of epidermal cells is shortened from 30 days in normal cells to 3–5 days.Citation17,Citation18 Previous studies have shown the upregulation of CDC20 and downregulation of CCND1 in psoriatic patients,Citation19 which could be targeted for drug optimization in the management of psoriasis as well as its comorbidities.Citation20
Figure 4 Protein regulatory network of differentially expressed genes. (A) PPI network of differentially expressed genes. (B) The degree of top genes in the PPI network.
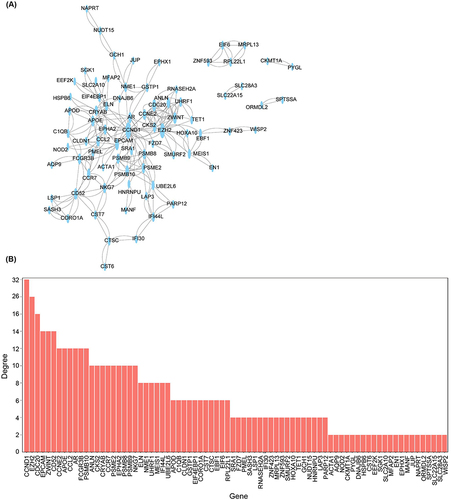
To investigate genes potentially implicated in psoriasis, hub genes for DEGs were identified using the CytoHubba algorithm. Given the heterogeneous nature of biological networks, multiple topological analysis algorithms were concurrently employed to identify these hub genes. Specifically, four algorithms including 80% principal components (EPC), Degree, Closeness, and BottleNeck were utilized to predict and explore the top 10 significant hub genes within PPI networks. In the results obtained, the algorithm based on EPC successfully identified anillin (ANLN), septin 7 (CDC10), ZWINT, cyclin-dependent kinase subunit 2 (CKS2), CCNE, CCND, EZH, proteasome subunit beta type 10 (PSMB10), AR, and EPCAM as highly influential factors in this context (). The Degree algorithm revealed APOE, chronic critical limb ischemia (CCLI), EPCAM, CCND, AR, EZH2, ZWINT, CDC20, and CD52 as significant variables in the analysis (). Similarly, the Closeness algorithm identified sombrero 9 (SMB9), ephrin type-a receptor 2 (EPHA2), PSMB10, CCND1, CCNE2, EPCAM, EZH2, AR, CDC20 and CCNE2 as relevant factors (). Furthermore, the BottleNeck algorithm identified proteasome subunit beta type 10 (PSMB10), nucleoside diphosphate kinases 1 (NME1), AR, CCND1, EIH2, EPCAM, CCL2, CDC20, Fc gamma receptor 3B (FCGR3B) and CD52 as key determinants (). Among these genes, androgen and its receptor (AR) were DEGs associated with ferroptosis, playing pivotal roles in various skin-related disorders. Given the involvement of aberrant or excessive inflammatory responses in psoriasis, it has been established that activation of AR in macrophages upregulated the expression of tumor necrosis factor α (TNF-α), thereby suppressing wound healing.Citation21,Citation22
Figure 5 Hub genes of the PPI network identified by Cytoscape. (A) Top 10 hub genes identified by EPC algorithm in Cytoscape. (B) Top 10 hub genes identified by degree algorithm in Cytoscape. (C) Top 10 hub genes identified by Closeness algorithm in Cytoscape. (D) Top 10 hub genes identified by BottleNeck algorithm in Cytoscape.

Identified and Efficacy Evaluation of Potential Marker Genes via Machine Learning
We have identified eight ferroptosis-related genes, namely PARP12, FZD7, ALOX15B, DNAJB6, EZH2, GCH1, PANX1, and AR (Supplementary Table 2). PARP12 primarily regulates disease by exerting control over protein transcription and immune response pathways during the process of transcription.Citation23 FZD7 functions as a Wnt receptor that modulates intracellular reduction-oxidation status, glutathione peroxidase 4 (Gpx4) expression, and glutathione metabolism by activating the canonical β-catenin/p63 pathway.Citation24 The protein encoded belongs to the 2,5-dihydroxypyridine dioxygenase (NicX) family, which is implicated in the biosynthesis of fatty acid hydroperoxides. During ischemia, ALOX15 can initiate phospholipid oxidation leading to ferroptosis signaling.Citation25 Overexpression of DNAJB6 leads to a significant reduction in the protein levels of Gpx4 and phosphorylated AKT (p-AKT).Citation26 AKT regulates various downstream pathways by modulating the activity of target protein through phosphorylation. The downstream pathways, including mammalian target of rapamycin (mTOR), vascular endothelial growth factor (VEGF), MAPK, glycolysis/gluconeogenesis, forkhead box O (FoxO), cell cycle, apoptosis, NF-κB predominate, P53, and other well-known factors in the field demonstrate the paramount importance of the PI3K-AKT pathway.Citation27 EZH2 and its highly related homologous gene EZH1 are recognized as epigenetic silencing factors that play a pivotal role in cellular growth and differentiation, serving as essential components of the polycomb inhibitory complex 2 (PRC2). Clinical trials have confirmed their tumor-restraining effects.Citation28 The proteins encoded by the PANX1 gene belong to the innexin family, which function as integral components of gap junctions. These proteins, along with pannexin 2, exhibit high expression in the central nervous system and co-localize within various neuronal cells that are closely associated with autoimmune diseases.Citation29,Citation30 The AR gene, belongs to the steroid receptor within the nuclear receptor superfamily, functions as a transcription factor that regulates eukaryotic gene expression and plays a pivotal role in the development and homeostasis of reproductive, skeletal muscle, cardiovascular, nervous, immune, and hematopoietic systems.Citation31,Citation32 Therefore, it is postulated that ferroptosis-related DEGs might have pivotal effects on the pathogenesis of psoriasis by modulating kinases, cytokines, and immune cells.
Subsequently, a diagnostic model for psoriasis was developed by employing random forest, support vector machine, LightGBM, and XGBoost algorithms. The pROC package in the R language was utilized to generate ROC curves based on the expression of eight genes in both training and test datasets, thereby evaluating the accuracy of the diagnostic features. According to the results, the LightGBM model demonstrated superior performance with the highest AUC values in both the training datasets (0.9999) and test datasets (test 1 = 1, test 2 = 0.9783) (). The random forest model exhibited relatively lower AUC values of 0.6681 (training datasets), 0.9375 (test 1), and 0.5095 (test 2). The support vector machine model yielded moderate AUC values of 0.7996 (training datasets), perfect prediction with an AUC value of 1 for test dataset 1, and a respectable value of 0.7337 for test dataset 2. The XGBoost model achieved AUC values of 1 (train datasets), 0.75 (test 1), and 0.7391 (test 2) (Supplementary Figure 1). Notably, it is worth mentioning that the LightGBM model outperformed others by surpassing an impressive threshold of exceeded 0.9 in both test datasets, indicating its exceptional predictive capabilities at their maximum plausible level. These findings suggested that PARP12, FZD7, ALOX15B, DNAJB6, EZH2, GCH1, PANX1, and AR exhibit potential as diagnostic markers for psoriasis, and the LightGBM model built based on these eight genes well distinguish psoriasis from healthy samples.
Figure 6 Model performance of candidate marker genes. (A) The ROC curve of the LightGBM model in 10-fold cross-validation. (B) The ROC curve of the LightGBM model in test datasets.
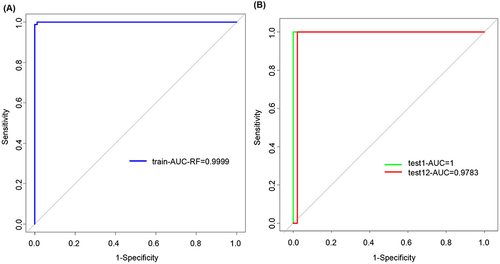
Furthermore, we conducted an analysis to determine the diagnostic potential of individual genes. The PARRP12 gene exhibited a ROC value of 0.9451 in the training datasets, 0.75 in test 1, and 0.9946 in test 2. The FZD7 gene demonstrated a ROC value of 0.8529 in the training datasets, perfect discrimination (ROC = 1) in test 1, and 0.9783 in test 2. The ALOX15B gene displayed an ROC value of 0.981 in the training datasets, 0.6875 in test 1, and 0.9851 in test 2. These findings suggested that PARRP12, FZD7, and ALOX15B hold promise as potential markers for diagnosing psoriasis (Supplementary Figure 2). Compared with these single genes, our LightGBM model was more specific and accurate in distinguishing psoriasis from healthy samples.
The Potential Drugs of Ferroptosis-Related Genes
To investigate potential drugs for psoriasis, DGIdb was utilized in this study. The findings revealed that FZD7 was associated with the drug VANTICTUMAB; GCH1 was linked to the drug GUANINE; ALOX15B exhibited associations with four drugs: RHODOMYRTOXIN B, DIPYRONE SODIUM, CHEMBL1448292, and CHEMBL601616; EZH2 demonstrated associations with six drugs: SEUMETINIB, VORINOSTAT, TAZEMETOSTAT, MK-2206, CPI-1205, and DABRAFENIB; AR displayed associations with six drugs: ENZALUTAMIDE, OXANDROLONE, APALUTAMIDE, ENOBOSARM, NANDROLONE ONEPHENPROPIONATE, and DAROLUTAMIDE ().
Proportion of Immune Cells in Normal Samples and Psoriasis Samples
According to the immune infiltration analysis, these were significant differences in the distribution of B cell subsets (naive and memory), plasma cells, T cells CD4+ memory activated, T cells gamma delta, natural killer (NK) cells resting, NK cells activated, monocytes, macrophages M0, macrophages M1, macrophages M2, dendritic cells resting, dendritic cells activated, mast cells resting, and mast cells activated between the healthy group and psoriasis groups (). In psoriasis samples, the downregulation of B cells naive, CD4 T cells memory activated, T cells gamma delta, NK cells activated, Macrophages M0, and Mast cells activated was observed. B cells naive are recruited to participate in immune responses through B cell receptor (BCR) stimulation initiated by antigen binding along with co-stimulatory signaling or in combination with T cell help to induce a T cell-dependent immune response.Citation33,Citation34 Macrophages M0 derived from the bone marrow are typically considered precursors of polarized macrophages. The prevailing perspective suggests that both M1 and M2 macrophages originate from the M0 state, where M0 represents a quiescent stage of macrophage activation. Notably, M1 macrophages play pivotal roles in promoting inflammation, sterilization, and phagocytosis.Citation35–37 Compared to normal samples, psoriasis samples exhibited higher infiltration levels of B cell memory, M1 and M2 macrophages, and activated dendritic cells, indicating an immune-active status.
Discussion
In this study, the differentially expressed genes in psoriasis samples were analyzed by bioinformatic analysis of public databases to explore whether ferroptosis is significant in psoriasis. Psoriasis is a chronic inflammatory disease propelled by proinflammatory cytokines through an underlying immune-mediated mechanism. The activation of ferroptosis-related cell death in psoriatic lesions subsequently contributed to the inflammatory reactions. Our findings revealed that psoriatic-related DEGs were predominant involvement in immune response, innate immune response, positive regulation of cell proliferation, cell adhesion, and positive regulation of cell migration. The most notable DEGs include interleukin 36 alpha (IL36α), interferon-induced protein 44-like (IFI44L), CKS2, CDC20, secretoglobin family 2A member 1 (SCGB2A1), chemokine receptor 7 (CCR7), ALOX15B, gremlin 1 (GREM1), FCGR3B, and ubiquitin-like with plant homeodomain and ring finger domains (UHRF). IL36α is a cytokine that triggers the activation of NF-κB and MAPK signaling pathways in target cells associated with an inflammatory response by activating the IL1RL2/IL-36R receptor.Citation38 IFI44L is classified as an interferon-stimulating gene, indicating its up-regulation in response to interferon stimulation.Citation39 CKS2 is a 9 kDa protein composed of 79 amino acids and encoded by a gene located on human chromosome 9q22. It exhibits aberrant expression in various malignant tumors, thereby contributing to tumorigenesis.Citation40 CDC20 binds and activates the anaphase-promoting complex (APC) during mitosis and G1 phase of the cell cycle.Citation41 CDC20 plays an essential role in the activity of APC/ cyclosome (APC/C) ubiquitin ligases and has been found to be associated with immune infiltration by CD8+ T cells, general T cells, monocytes, and exhausted T cells.Citation42 CCR7 plays a pivotal role as a transmembrane protein in coordinating the migration of white blood cells through the lymphatic system to orchestrate immune defense against pathogens. It is strategically positioned within the cellular membrane, enabling it to receive extracellular signals and transmit them intracellularly.Citation43 ALOX15 plays a crucial role in the biosynthesis of essential lipid mediators, such as lipoxins and resolvins, which are pivotal for resolving inflammation. This gene exerts inhibitory effects on the TNF-α, IL-1β/NF-κB, and IL-6/STAT3 signaling pathways that significantly contribute to colorectal cancer promotion through chronic inflammation.Citation44 The protein encoded by the FCGR3B gene is low-affinity γ receptors located in the Fc region of immunoglobulin G (IgG). The monomeric protein has a high binding affinity towards both monomeric and aggregated forms of IgG, thereby playing an important role in sequestering immune complexes within the peripheral circulation.Citation45 UHRF1 may function as an oncogene by inducing dysregulated immune infiltration in hepatocellular carcinoma (HCC) and has been identified as a novel prognostic biomarker and potential therapeutic target for HCC.Citation46 In the PPI analysis, CDC20, CCR7, and FCGR3B were found to regulate a larger number of downstream genes. Specifically, CDC20 was observed to regulate ribonuclease H2 subunit A (RNASEH2A), CCND1, ANLN, EZH2, CCNE2, ZWINT, UHRF1, and CKS2. CCR7 was found to regulate natural killer cell group 7 (NKG7), CCL2, premelanosome protein (PMEL), CD52, and FCGR3B. Additionally, FCGR3B was identified as a regulator of aquaporin 9 (AQP9), CCL2, CCR7, EPCAM, C1q subcomponent subunit B (C1QB), and CD52. These results suggested that the inflammatory reactions within psoriatic tissues were significantly altered.
Using the FerrDb database, we identified eight differentially expressed ferroptosis-related genes (PARP12, FZD7, ALOX15B, DNAJB6, EZH2, GCH1, PANX1, and AR) between normal and psoriasis samples. The Random Forest model effectively validated these genes, exhibiting high associated ROC values, thereby indicating their potential as accurate biomarkers for psoriasis. Furthermore, these genes play crucial roles in disease pathways; for example, PARP12 is implicated in immune response pathways during transcription.Citation23 FZD7 acts as a Wnt receptor, regulating Gpx4 and intracellular reduction-oxidation status to activate the canonical β-catenin/p63 pathway.Citation24 ALOX15 induces phospholipid oxidation, generating ferroptosis signals during ischemia.Citation25 Overexpression of DNAJB6 modulates AKT protein levels.Citation26 EZH2 plays a pivotal role in cellular growth and differentiation.Citation28 AR governs the regulation of eukaryotic gene expression and plays a pivotal role in the development and maintenance of reproductive, skeletal muscle, cardiovascular, nervous, immune, and hematopoietic systems.Citation31
The drugs of these eight genes were simultaneously analyzed in the DGIdb database. A total of 18 compounds with potential as psoriasis drugs were identified, which can be further investigated for their clinical treatment efficacy. Additionally, the interplay between immune cells and cytokines emerges as a critical factor in the pathogenesis of psoriasis. Immune infiltration analysis revealed significant differences in immune profiles between patients with psoriasis and healthy controls, including alterations in B cells, T cells, NK cells, macrophages, dendritic cells, and mast cells. Despite the availability of various therapeutic interventions, psoriasis remains an incurable condition. These findings may provide additional insights into the pathogenesis and management of psoriasis.
The study had several limitations. Firstly, the key pathways and hub genes identified in the present study have not been validated through laboratory experiments. However, it is worth noting that extensive elucidation of the roles of ferroptosis-related genes in psoriasis has been achieved through multiple preclinical and clinical studies, thereby enhancing the reliability of this investigation. Secondly, a more comprehensive collection of omics data about psoriasis is warranted for further analysis and validation of marker genes.
Conclusion
This bioinformatic study revealed that PARP12, FZD7, ALOX15B, DNAJB6, EZH2, GCH1, PANX1, and AR as potential biomarkers of ferroptosis in psoriasis. The immune gene expression and immune cell infiltration observed in the skin lesions play a central role in the pathogenesis of psoriasis. These findings have significant implications for understanding the underlying mechanisms and developing novel therapeutic approaches for psoriasis.
Abbreviations
AIFM1, apoptosis-inducing factor mitochondria-associated 1; ALOX15, arachidonate 15-lipoxygenase; ALOX15B, arachidonate 15-lipoxygenase type B; ANLN, anillin; APC/C, APC/cyclosome; APC, anaphase-promoting complex; APOE, age and apolipoprotein E; AQP9, aquaporin 9; AR, androgen receptor; AUC, area under the curve; BP, biological process; C1QB, C1q subcomponent subunit B; CC, cellular component; CCL2, C-C motif ligand 2; CCLI, chronic critical limb ischemia; CCND1, cellular interaction are cyclin D1; CCNE2, cyclin E2; CCR7, chemokine receptor 7; CDC10, septin 7; CDC20, Cell-division cycle protein 20 homolog; cGAS-STING, cyclic guanosine monophosphate-adenosine monophosphate synthase -stimulator of interferon genes; CKS2, cyclin-dependent kinase subunit 2; CTS, carpal tunnel syndrome; DAVID, database for annotation, visualization, and integrated discovery; DEG, differentially expressed gene; DNAJB6, DnaJ homolog subfamily B member 6; EPC, 80% principal components; EPCAM, epithelial cell adhesion molecule; EPHA2, ephrin type-a receptor 2; EZH2, enhancer of zeste homolog 2; FC, fold change; FCGR3B, Fc gamma receptor 3B; FoxO, forkhead box O; FZD7, frizzled homolog 7; GCH1, GTP cyclohydrolase 1; GEO, gene expression omnibus; GO, gene ontology; Gpx4, glutathione peroxidase 4; GREM1, gremlin 1; HCC, hepatocellular carcinoma; IFI44L, interferon-induced protein 44-like; IgG, immunoglobulin G; IL36A, interleukin 36A; JAK-STAT, janus kinase-signaling transducer and activator of transcription; KEGG, Kyoto encyclopedia of genes and genomes; MAPK, mitogen-activated protein kinase; MF, molecular function; mTOR, mammalian target of rapamycin; NF-κB, nuclear factor-κB; NicX, 2.5-dihydroxypyridine dioxygenase; NK, natural killer; NME1, nucleoside diphosphate kinases 1; p-AKT, phosphorylated AKT; PANX1, pannexin 1; PARP12, poly ADP-ribose polymerase 12; PPI, protein–protein interaction; PRC2, polycomb inhibitory complex 2; PSMB10, proteasome subunit beta type 10; PSMB10, proteasome subunit beta type 10; RNASEH2A, ribonuclease H2 subunit A; ROC, receiver operating characteristic; ROS, reactive oxygen species; SCGB2A1, secretoglobin family 2A member 1; SMB9, sombrero 9; STRING, search tool for the retrieval of interacting genes database; UHRF1, ubiquitin-like with plant homeodomain and ring finger domains 1; VEGF, vascular endothelial growth factor; ZWINT, ZW10 interactor.
Data Sharing Statement
The original contributions presented in the study are included in the article/Supplementary Material. The datasets used in this research (GSE13355 GSE30999, and GSE50790) were directly obtained from the GEO public data platform (https://www.ncbi.nlm.nih.gov/geo/). Further inquiries can be directed to the corresponding authors.
Ethics Approval and Informed Consent
The datasets used in this research (GSE13355 GSE30999, and GSE50790) were directly obtained from the GEO public data platform (https://www.ncbi.nlm.nih.gov/geo/). GEO is a public database that encompasses patients who have obtained ethical approval. Researchers are granted unrestricted access to pertinent data for conducting studies and publishing relevant articles. This research was approved by the Ethics Review Committee of Shenzhen Second People’s Hospital (No. 2024-149-01PJ).
Disclosure
The authors declare that they have no competing interests in this work.
Additional information
Funding
References
- Visser MJE, Venter C, Roberts TJ, Tarr G, Pretorius E. Psoriatic disease is associated with systemic inflammation, endothelial activation, and altered haemostatic function. Sci Rep. 2021;11(1):13043. doi:10.1038/s41598-021-90684-8
- Egeberg A, Mallbris L, Warren RB, et al. Association between psoriasis and inflammatory bowel disease: a Danish nationwide cohort study. Br J Dermatol. 2016;175(3):487–492. doi:10.1111/bjd.14528
- Hu W, Liang K, Zhu H, Zhao C, Hu H, Yin S. Ferroptosis and its role in chronic diseases. Cells. 2022;11(13):2040. doi:10.3390/cells11132040
- Chen Y, Fang Z, Yi X, Wei X, Jiang D. The interaction between ferroptosis and inflammatory signaling pathways. Cell Death Dis. 2023;14(3):205. doi:10.1038/s41419-023-05716-0
- Shou Y, Yang L, Yang Y, Xu J. Inhibition of keratinocyte ferroptosis suppresses psoriatic inflammation. Cell Death Dis. 2021;12(11):1009. doi:10.1038/s41419-021-04284-5
- Zhang D, Li Y, Du C, et al. Evidence of pyroptosis and ferroptosis extensively involved in autoimmune diseases at the single-cell transcriptome level. J Transl Med. 2022;20(1):363. doi:10.1186/s12967-022-03566-6
- Liu L, Kang X. ACSL4 is overexpressed in psoriasis and enhances inflammatory responses by activating ferroptosis. Biochem Biophys Res Commun. 2022;623:1–8. doi:10.1016/j.bbrc.2022.07.041
- Nair RP, Duffin KC, Helms C, et al. Genome-wide scan reveals association of psoriasis with IL-23 and NF-kappaB pathways. Nat Genet. 2009;41(2):199–204. doi:10.1038/ng.311
- da Rosa JC, Kim J, Tian S, Tomalin LE, Krueger JG, Suárez-Fariñas M. Shrinking the psoriasis assessment gap: early gene-expression profiling accurately predicts response to long-term treatment. J Invest Dermatol. 2017;137(2):305–312. doi:10.1016/j.jid.2016.09.015
- Swindell WR, Xing X, Stuart PE, et al. Heterogeneity of inflammatory and cytokine networks in chronic plaque psoriasis. PLoS One. 2012;7(3):e34594. doi:10.1371/journal.pone.0034594
- Dennis JG, Sherman BT, Hosack DA, et al. DAVID: database for annotation, visualization, and integrated discovery. Genome Biol. 2003;4(5):56.
- Chin C, Chen S, Wu H, Ho C, Ko M, Lin C. cytoHubba: identifying hub objects and sub-networks from complex interactome. BMC Syst Biol. 2014;8 Suppl 4(Suppl 4):S11. doi:10.1186/1752-0509-8-S4-S11
- Robin X, Turck N, Hainard A, et al. pROC: an open-source package for R and S+ to analyze and compare ROC curves. BMC Bioinf. 2011;12:77. doi:10.1186/1471-2105-12-77
- Neitemeier S, Jelinek A, Laino V, et al. Bid links ferroptosis to mitochondrial cell death pathways. Redox Biol. 2017;12:558–570. doi:10.1016/j.redox.2017.03.007
- Seiler A, Schneider M, Förster H, et al. Glutathione peroxidase 4 senses and translates oxidative stress into 12/15-lipoxygenase dependent- and AIF-mediated cell death. Cell Metab. 2008;8(3):237–248. doi:10.1016/j.cmet.2008.07.005
- Dietrich C, Hofmann TG. Ferroptosis meets cell-cell contacts. Cells. 2021;10(9):2462. doi:10.3390/cells10092462
- Huang Y, Zheng Y, Zhou Y, et al. OAS1, OAS2, and OAS3 contribute to epidermal keratinocyte proliferation by regulating cell cycle and augmenting IFN-1‒induced Jak1‒signal transducer and activator of transcription 1 phosphorylation in psoriasis. J Invest Dermatol. 2022;142(10):2635–2645. doi:10.1016/j.jid.2022.02.018
- Gelfant S. The cell cycle in psoriasis: a reappraisal. Br J Dermatol. 1976;95(6):577–590. doi:10.1111/j.1365-2133.1976.tb07028.x
- Gudjonsson JE, Ding J, Johnston A, et al. Assessment of the psoriatic transcriptome in a large sample: additional regulated genes and comparisons with in vitro models. J Invest Dermatol. 2010;130(7):1829–1840. doi:10.1038/jid.2010.36
- Dorai S, Anand DA. Differentially expressed cell cycle genes and STAT1/3-driven multiple cancer entanglement in psoriasis, coupled with other comorbidities. Cells. 2022;11(23):3867. doi:10.3390/cells11233867
- Ashcroft GS, Mills SJ. Androgen receptor-mediated inhibition of cutaneous wound healing. J Clin Invest. 2002;110(5):615–624. doi:10.1172/JCI15704
- Lai J, Lai K, Chuang K, et al. Monocyte/macrophage androgen receptor suppresses cutaneous wound healing in mice by enhancing local TNF-alpha expression. J Clin Invest. 2009;119(12):3739–3751. doi:10.1172/JCI39335
- Welsby I, Hutin D, Gueydan C, Kruys V, Rongvaux A, Leo O. PARP12, an interferon-stimulated gene involved in the control of protein translation and inflammation. J Biol Chem. 2014;289(38):26642–26657. doi:10.1074/jbc.M114.589515
- Wang Y, Zhao G, Condello S, et al. Frizzled-7 identifies platinum-tolerant ovarian cancer cells susceptible to ferroptosis. Cancer Res. 2021;81(2):384–399. doi:10.1158/0008-5472.CAN-20-1488
- Ma X, Liu J, Liu C, et al. ALOX15-launched PUFA-phospholipids peroxidation increases the susceptibility of ferroptosis in ischemia-induced myocardial damage. Signal Transduct Target Ther. 2022;7(1):288. doi:10.1038/s41392-022-01090-z
- Jiang B, Zhao Y, Shi M, et al. DNAJB6 promotes ferroptosis in esophageal squamous cell carcinoma. Dig Dis Sci. 2020;65(7):1999–2008. doi:10.1007/s10620-019-05929-4
- Manning BD, Toker A. AKT/PKB signaling: navigating the network. Cell. 2017;169(3):381–405. doi:10.1016/j.cell.2017.04.001
- Hanaki S, Shimada M. Targeting EZH2 as cancer therapy. J Biochem. 2021;170(1):1–4. doi:10.1093/jb/mvab007
- Yang K, Xiao Z, He X, Weng R, Zhao X, Sun T. Mechanisms of pannexin 1 (PANX1) channel mechanosensitivity and its pathological roles. Int J Mol Sci. 2022;23(3):1523. doi:10.3390/ijms23031523
- Bisht K, Okojie KA, Sharma K, et al. Capillary-associated microglia regulate vascular structure and function through PANX1-P2RY12 coupling in mice. Nat Commun. 2021;12(1):5289. doi:10.1038/s41467-021-25590-8
- Grad J, Lyons L, Robins D, Burnstein K. The androgen receptor (AR) amino-terminus imposes androgen-specific regulation of AR gene expression via an exonic enhancer. Endocrinology. 2001;142(3):1107–1116. doi:10.1210/endo.142.3.8049
- Kaltz-Wittmer C, Klenk U, Glaessgen A, et al. FISH analysis of gene aberrations (MYC, CCND1, ERBB2, RB, and AR) in advanced prostatic carcinomas before and after androgen deprivation therapy. Lab Invest. 2000;80(9):1455–1464. doi:10.1038/labinvest.3780152
- Jain RW, Yong VW. B cells in central nervous system disease: diversity, locations and pathophysiology. Nat Rev Immunol. 2022;22(8):513–524. doi:10.1038/s41577-021-00652-6
- Akkaya M, Kwak K, Pierce SK. B cell memory: building two walls of protection against pathogens. Nat Rev Immunol. 2020;20(4):229–238. doi:10.1038/s41577-019-0244-2
- Pucci M, Raimondo S, Urzì O, et al. Tumor-derived small extracellular vesicles induce pro-inflammatory cytokine expression and PD-L1 regulation in M0 macrophages via IL-6/STAT3 and TLR4 signaling pathways. Int J Mol Sci. 2021;22(22):12118. doi:10.3390/ijms222212118
- Xie J, Chen L, Cao Y, et al. Single-cell sequencing analysis and weighted co-expression network analysis based on public databases identified that TNC is a novel biomarker for keloid. Front Immunol. 2021;12:783907. doi:10.3389/fimmu.2021.783907
- Genin M, Clement F, Fattaccioli A, Raes M, Michiels C. M1 and M2 macrophages derived from THP-1 cells differentially modulate the response of cancer cells to etoposide. BMC Cancer. 2015;15:577. doi:10.1186/s12885-015-1546-9
- Bassoy EY, Towne JE, Gabay C. Regulation and function of interleukin-36 cytokines. Immunol Rev. 2018;281(1):169–178. doi:10.1111/imr.12610
- Coit P, Jeffries M, Altorok N, et al. Genome-wide DNA methylation study suggests epigenetic accessibility and transcriptional poising of interferon-regulated genes in naïve CD4+ T cells from lupus patients. J Autoimmun. 2013;43:78–84. doi:10.1016/j.jaut.2013.04.003
- Lan Y, Zhang Y, Wang J, Lin C, Ittmann MM, Wang F. Aberrant expression of Cks1 and Cks2 contributes to prostate tumorigenesis by promoting proliferation and inhibiting programmed cell death. Int, J, Cancer. 2008;123(3):543–551. doi:10.1002/ijc.23548
- Fang G, Yu H, Kirschner MW. Direct binding of CDC20 protein family members activates the anaphase-promoting complex in mitosis and G1. Mol Cell. 1998;2(2):163–171. doi:10.1016/s1097-2765(00)80126-4
- Xiong C, Wang Z, Wang G, et al. Identification of CDC20 as an immune infiltration-correlated prognostic biomarker in hepatocellular carcinoma. Invest New Drugs. 2021;39(5):1439–1453. doi:10.1007/s10637-021-01126-1
- Han L, Zhang L. CCL21/CCR7 axis as a therapeutic target for autoimmune diseases. Int Immunopharmacol. 2023;121:110431. doi:10.1016/j.intimp.2023.110431
- Xu X, Li J, Zhang Y, Zhang L. Arachidonic acid 15-lipoxygenase: effects of its expression, metabolites, and genetic and epigenetic variations on airway inflammation. Allergy Asthma Immunol Res. 2021;13(5):684–696. doi:10.4168/aair.2021.13.5.684
- Typiak M, Rękawiecki B, Rębała K, Dubaniewicz A. Comparative analysis of FCGR gene polymorphism in pulmonary sarcoidosis and tuberculosis. Cells. 2023;12(9):1221. doi:10.3390/cells12091221
- Li D, Chen B, Zeng Y, Wang H. UHRF1 could be a prognostic biomarker and correlated with immune cell infiltration in hepatocellular carcinoma. Int J Gen Med. 2021;14:6769–6776. doi:10.2147/IJGM.S335016