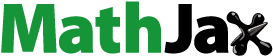
Abstract
Objective:
To assess the cost-effectiveness of two treatments (medical treatment and amputation) in patients with diabetic foot syndrome, one of the most disabling diabetic complications. Diabetes mellitus is a massive health care problem worldwide with a current prevalence of 150 millions diabetic cases, estimated to increase to 300 million cases in 2025.
Methods:
Integrating medical knowledge and advances into the clinical setting is often difficult due to the complexity of the algorithms and protocols. Clinical decision support systems assist the clinician in applying new information to patient care through the analysis of patient-specific clinical variables. We require strategic decision support to analyze the cost-effectiveness of these programs compared to the status quo. We provide a simple partially observable Markov model to investigate that issue, and we propose an heuristic algorithm to find the best policy of intervention.
Results:
This study assesses the potential cost-effectiveness of two alternative treatment interventions in patients with diabetic foot syndrome. The implementation of the heuristic algorithm solution will assist doctors in clinical decision making, and health care organizations in evaluating medication choices for effective treatment. Finally, our study reveals that treatment programs are highly cost-effective for patients at high risk of diabetic foot ulcers and lower extremity amputations.
Introduction
An estimated 24 million Americans suffer from diabetes.Citation1 One of the major risk factors for lower extremity amputation is an antecedent foot ulcer. The symptoms of diabetic foot syndrome comprise a loss of protective sensation and, as a consequence, a lack of awareness, innervation of small muscles, and diminished fine control of pedal circulation. Polyneuropathy peripheral arterial occlusive disease, and infections, lead to diabetic foot syndrome. In most cases, the precipitant of ulceration is an accidental trauma which induces breaks of the skin and further complications such as ulcers, infections, or gangrene. Small bones may break due to loss of structural bone integrity.Citation2 About 15% of all diabetics require foot amputations, and 20%–25% of those even require re-amputations. The amputation aims at leaving a wound that will heal.Citation3 Prevention programs and therapy at an early stage could diminish the number of amputations by as much as 80%–90%.Citation4,Citation5
Researchers have applied several models to investigate the complications of the diabetic foot syndrome. Management of diabetic foot infections involves evaluating and determining the severity of infection, as the basis for selecting the appropriate approach to treatment.Citation6,Citation7 Unfortunately, the lack of consensus on wound definitions and infection classification systems hampers the comparison of published studies. Consensus is developing that the key issues in classifying a diabetic foot wound are its depth (in particular, which tissues are involved) and whether the wound is complicated by either ischemia or infection.Citation8,Citation9
Some researchers have analyzed the cost-effectiveness of treating foot infections in a diabetic cohortCitation10,Citation11 by constructing a Markov model with Monte Carlo techniques. These studies combined multiple diabetic complications and analyzed prevention strategies for diabetes. The model predicted the rates of microvascular complications and mortality,Citation12 describing seven threats to validity for economic evaluations that are based on randomized controlled trials. Habacher et alCitation13 provide a strong overview of the problems that may be encountered with modeling in economic evaluation.
In this paper, we construct and propose a partially observable Markov decision model and assess its implementation in the evaluation of diabetes mellitus prevention strategies for diabetic foot complications in Greece. Our paper is organized in the following sections:
First we present the proposed partially observable Markov decision process (POMDP) model, and we describe and discuss its underlying data. In the following section, we construct a suitable heuristic solution algorithm. The simplicity of the proposed algorithm gives the advantage of a supporting tool required for the prevention of diabetic foot syndrome (alongside clinical examination). In the subsequent section, we present the results of the model’s practical implementation in a case study. Finally, we discuss our findings and we make some suggestions for the practical utilization of the proposed model.
The POMDP model for the treatment of diabetic foot prevention
In this section we formulate a POMDP modelCitation14 in order to assess a cost-effectiveness analysis of prevention strategies for diabetic foot syndrome. Generally, POMDP models have been successfully reviewed for theory and for state-of-the-art solution algorithms.Citation15–Citation18 To construct a Markov model for a particular application, one must first specify the Markov states. These health states should not only reflect all the relevant states of health associated with diabetes and treatment over time, but should also include and take into consideration all relevant clinical history. According to the international literature on the course of the disease (etiopathology), patients with diabetic foot syndrome pass through eight different stages: (Si)Citation19,Citation20
no foot ulcer (S1)
uncomplicated ulcer (S2)
deep foot infection (S3)
foot ulcer and critical ischemia (S4)
primary healing (S5)
minor amputation (S6)
major amputation (S7)
The second step in building a Markov model is to choose a cycle length, which must be a constant increment of time. So, we used the cycle length of half a year, because studies reported wound healing times of about six months for diabetic foot ulcers and amputations.Citation2,Citation3,Citation5 A POMDP model is typically defined as a six-parameter tuple. The six parameters together capture all the required aspects of medical decision making. A partially observable decision process is:
S is the set of physical states of a patient with diabetes mellitus. As mentioned above, we have seven states.
A(t) ∈ A, decision taken at time t, t = 0, 1, 2, …
In our paper, we suggest that optimal prevention for high-risk patients is cost-saving, under the assumption of a 25% lower incidence of foot ulcers and extremity amputation compared to baseline prevention scenarios. (Also, we do not implement age- and risk group-specific transitions in our POMDP model). For simplicity, we consider two alternative treatments: medical treatments, and amputation, which are coded as 1 and 2 respectively; A = {1, 2}.
Given transition probability matrices:
(2.1)
In preparation for building the POMDP model, we are going to specify the transition probabilities with cohort studies in a central hospital in Athens.
An observation on the system Y (t), and invariant conditional probabilities:
(2.2) Θ/j may also be assumed to be a continuous random variable, with a known conditional density f (θ/j). In this paper, we supposed that in a given time interval (check-up period), the physician counts the number of failed tests Y (t) = θ.
(2.3) where λi is the rate of failures tests in state i multiplied by the check-up time.Citation17
Family physicians infrequently perform foot examinations in diabetic patients during routine office visits. Careful inspection of the diabetic foot on a regular basis is one of the easiest, least expensive, and most effective measures for preventing foot complications.Citation7 Appropriate care of the diabetic foot requires recognition of the most common risk factors for limb loss.Citation21 Many of these risk factors can be identified based on specific aspects of the clinical history, and a brief and systematic examination of the foot.
Common risk factors for amputation of the diabetic foot include peripheral neuropathy, structural foot deformity, ulceration, infection, and peripheral vascular disease. It is important to recognize that foot ulcers can have a multi-factorial etiology.Citation22
Peripheral arterial occlusive disease typically involves the tibial and peroneal arteries, but spares the dorsalis pedis artery.Citation23
The presence of lower extremity ischemia is suggested by a combination of clinical signs and symptoms, plus abnormal results on noninvasive vascular tests. Signs and symptoms may include claudication, and pain occurring in the arch or forefoot at rest or during the night.Citation21
Noninvasive vascular tests include transcutaneous oxygen measurement, absolute toe systolic pressure, and the ankle-brachial index (ABI). The ABI is a noninvasive test that can be performed easily in the office using a handheld Doppler device. The sensitivity and specificity of noninvasive vascular tests are a matter of some controversy.Citation24
Digital symmetric polyneuropathy is perhaps the most common complication affecting the lower extremities of patients with diabetes mellitus.Citation4
The nylon monofilament test is a simply-performed office test to diagnose patients at risk for ulcer formation due to peripheral sensory neuropathy. The test is abnormal if the patient cannot sense the touch of the monofilament when it is pressed against the foot with just enough pressure to bend the filament.
Foot deformities, which are common in diabetic patients, lead to focal areas of high pressure. When an abnormal focus of pressure is coupled with lack of sensation, a foot ulcer can develop.Citation23
Also, a diabetic patient with a history of previous ulceration is at increased risk for further ulceration, infection, and subsequent amputation.
The probabilistic relation between observation process Y(t) and core process X(t) is given by the 7 × 7 time-invariant observation matrix R. We apply the expectation-maximization (EM) algorithmCitation18 to estimate the above observation probabilities. Alternatively, we can apply cohort studies.Citation22
Given real values q(i, k), which signify the expected incurred immediate costs/rewards in taking action k, while being in patient state i.
We have a discount factor β, 0 < β < 1.
In a POMDP model, the state of the patient is not known with certainty. The vector of state probabilities π(t) = (π1(t), (π2(t), …, (π7(t)) is called the information vector:
(2.4)
It is well known that π(t) is a sufficient statistic.Citation25 More precisely, π(t) summarizes all of the necessary information on the history of the process for choosing an action at time t. The probabilities πi(t) in the type (2.4) are updated and reassigned to the system states by the decision maker at every step t, given the history of the process. However, the update of πi(t) is Bayesian, such that the detail of the history is not used directly. Specifically,
(2.5a)
(2.5b) where, Pl is the first column of P, and matrix multiplication is implied where appropriate. To clarify, the order of things in the decision process is the following:
At time t, the state of a patient with diabetes mellitus is an unknown state X(t) = i. Based on π(t – 1), the matrices P, and the vectors q(·, A(t – 1)) a decision is taken: A(t) = k, k = 1, 2. Instantaneously, the patient switches to unknown state j. According to
, an immediate average reward or cost q(i, k) is gained, and the observation process provides a signal Y(t) = θ. The decision maker then updates the state probabilities by (2.5a) or (2.5b). The process repeats itself indefinitely. Focusing on the total discounted reward criterion, we wish to optimize the decisions so as to maximize:
(2.6) for all initial probability vectors π (0). It is assumed that the process is initiated with a known probability distribution over the state of the patient. The dependence on π(0) has been dropped from the notation in (2.6), and 0 < β < 1 is a discount factor.
A solution method
The generality of the standard POMDP modelCitation14 limits practical application of the framework, due to the computational complexity of associated solution methods. However, for many specialized problems, the full-blown generality of the POMDP approach and its associated solution methods is superfluous. In this section, we provide a heuristic rule that it is stationary and extremely simple. It runs as follows:
Step 1
We solve the fully observable problem by Howard’s policy iteration,Citation25 and then we value the determination routine. We find d*(i) for all 1 ≤ i ≤ 7, where d*(i) stands for the Howard’s optimal decision, assuming state i is fully observed, d(i) ∈ D. As an output of the algorithm, we get the Vi values; the expected total discounted reward starting in state i.
Notably:
(3.1)
Step 2
For the current state vector π(t), we calculate an approximate reward functional Ṽ(π(t))
(3.2) and set A(t) = α̃ = arg max Ṽ(π(t)). The advantage of the above algorithm is the fact that it requires only a single run of Howard’s routine.
Implementation of the proposed model – a case study
For comprehension of the above algorithm, we present the implementation results from the treatment of patients with diabetic foot syndrome in a middle-sized public hospital in Greece, during the year 2008. We have used seven health states S = {1, 2, 3, …, 7}, and two treatments A = {1, 2}.
The rewards/costs are derived from the National Statistical Service of Greece. The direct costs include drug costs, hospitalization costs, monitoring costs, and physician visit costs.
The costs for the diabetic foot syndrome, and the model’s effectiveness parameters were taken from various studies.Citation20,Citation26–Citation28 We considered a discount factor β=0.90. When estimating transition probabilities, it is important to be mindful of the cohorts or target populations that are to be analyzed, as one can have a number of different transition probability matrices that are specific for different cohorts, defined by demographic or clinical variables. In order to find the final information vector, we can use regression models.
For example, if the initial information vector for a patient with diabetic foot syndrome is π = (0.10, 0.20, 0.10, 0.10, 0.30, 0.20, 0.00), using Howard’s algorithm, we have:
Ṽ (π) = max {3723, 3970} = 3970, and the optimal decision is amputation: Ṽ* (5) = 2. To clarify, the order of things in the decision process according above POMDP model is the following. At time t = 0, a patient with diabetic foot syndrome has an information vector π(0) = (0.10, 0.20, 0.20, 0.20, 0.30, 0.00, 0.00). This information vector is a sufficient statistic for taking an action.Citation25 In terms of the above model, we take amputation after the state “primary healing”. So, the doctor has a supporting tool in their decision making.
Discussion
The risk of injury to the diabetic foot is high, and the consequences of injury can be severe. Therefore, prevention based upon clinical trials and doctors is undoubtedly the best strategy. Decision analytic modeling, undertaken for the purpose of economic evaluation of health technologies, involves the application of mathematical techniques to synthesize available information about health care processes and their implications.Citation10,Citation15,Citation20
In our paper, we provide a simple POMDP model for taking a cost-effective decision for the treatment of foot ulcer syndrome. Many researchers have proposed similar models for the management of diabetic foot ulcer.Citation13,Citation19,Citation29 However, in these models, the integration of medical knowledge and advances into the clinical setting is often difficult, due to the complexity of the algorithms and protocols. So, in our model, we propose the incorporation of the required information into a Clinical Decision Support System (CDSS), in order to analyze the patient-specific clinical variables. In this way, we can essentially assist the clinician toward their best medical decision making. In our proposed model, we also used cost-related information, incorporating utility functions, to support the cost-effectiveness evaluation of these programs, and the strategic management of health care organizations.
So, the main advantage of the proposed model lies in the clinician’s capability for full adaptation control, meaning ease of modification of any of the model’s parameters incorporated into the CDSS.
Conclusion
This model is significant because it provides an explicit two-way bridge between primary clinical data and early and efficient medical decision making. Typically, the objective of this decision model is to obtain a clearer understanding of the relationship between incremental costs and their consequences.
The proposed simple model (incorporated into a CDSS) results in health care quality improvement, cost-effective clinical decision making, and adaptability and transferability through different health care environments (either organizations or countries). So, the exchange of reliable and timely information through various levels of the health care system in Greece will facilitate the efficiency and effectiveness of the proposed model.
Acknowledgements
The authors gratefully acknowledge the reviewers of this manuscript for their invaluable comments and suggestions.
Disclosure
The authors report no conflict of interest in this work.
References
- HuangEBasuAO’GradyMCaprettaJProjecting the future diabetes population size and related costs for the U.SDiabetes Care200932122225222919940225
- JeffcoateWJMacfarlaneRMThe Diabetic Foot: An Illustrated Guide to ManagementLondonChapman and Hall Medical1995
- JeffcoateWJHardingKGDiabetic foot ulcersLancet200336193681545155112737879
- WildSRoglicGGreenASicreeRKingHGlobal prevalence of diabetes: estimates for the year 2000 and projections for the year 2030Diabetes Care20042751047105315111519
- SinghNArmstrongDLipskyBPreventing foot ulcers in patients with diabetesJAMA2005293221722815644549
- PathareNBalATalvalkarGAntaniDDiabetic foot infections: a study of microorganism associated with the different Wagner gradesIndian J Pathol Microbiol19984144374419866905
- FrykbergRDiabetic foot ulcers: pathogenesis and managementAm Fam Physician20026691655166212449264
- WagnerFWJrThe diabetic footOrthopedics19871011631723809012
- CalhounJHCantrellJCobosJTreatment of diabetic foot infections: Wagner classification, therapy, and outcomeFoot Ankle1988931011063229695
- EckmanMHGreenfieldSMackeyWCFoot infections in diabetic patients. Decision and cost-effectiveness analysesJAMA1995127397127207853629
- OyiboSOJudeEBNguyenHCHarklessLBBoultonAJA comparison of two diabetic foot ulcer classification systems: the Wagner and the University of Texas wound classification systemsDiabetes Care2001241848811194247
- O’BrienBEconomic evaluation of pharmaceuticals. Frankenstein’s monster or vampire of trials?Med Care199634Suppl 12DS99DS1088969318
- HabacherWRakovacIGorzerEA model to analyse costs and benefit of intensified diabetic foot care in AustriaJ Eval Clin Pract20071390691218070261
- SondikEThe optimal control of partially observable Markov decision processes over the infinite horizon: discounted costsOper Res197826282304
- GoulionisJVozikisAMedical decision making for patients with Parkinson disease under Average Cost CriterionAust New Zealand Health Policy20096151819159485
- GoulionisJMedical treatments using partially observable Markov decision processesJP J Biostat2009327797
- GoulionisJKoutsioumarisBStengosDDynamic decision making for clinical patient management with Parkinson’s diseaseJP J Biostat200823217235
- LovejoyWA survey of algorithmic methods for partially observed Markov decision processesAnn Oper Res19912814765
- Ragnason TennvallGApelqvistJPrevention of diabetes-related foot ulcers and amputations: a cost-utility analysis based on Markov model simulationsDiabetologia200144112077208711719840
- FosterAEdmondsMSimple staging system: a tool for diagnosis and managementDiabetic Foot200035662
- ManesCPapazoglouNSossidouEPrevalence of diabetic neuropathy and foot ulceration: identification of potential risk factors – a population based studyWounds2002141115
- DallaPFagliaECaminitiMClericiGNinkovicSDeanesiVUlcer recurrence following first ray amputation in diabetic patients: a cohort prospective studyDiabetic Care200326618741878
- BelhadjMLa place du pied diabetiqueDiabetes Metab199824Suppl 1LXVII
- RamseySDNewtonKBloughDIncidence, outcomes, and cost of ulcers in patients with diabetesDiabetes Care199922338238710097914
- BertsekasDDynamic Programming and Optimal ControlI and IIBelmont, MAAthena Scientific1995
- BoultonAVileikyteLRagnason TennvallGApelqvistJThe global burden of diabetic foot diseaseLancet20053661719172416291066
- ApelqvistJWhat is the most effective way to reduce incidence of amputation in the diabetic foot?Diabetes Metab Res Rev200016Suppl 1S75S8311054894
- OrtegonMRedekopWNiessenLCost-effectiveness of prevention and treatment of the diabetic foot: a Markov analysisDiabetes Care20042790190715047646
- RaunerMHeidenbergerKPesendorferEModel-based evaluation of diabetic foot prevention strategies in AustriaHealth Care Manag Sci2005825326516379409