Abstract
Background
As HIV infection turned into a chronic treatable disease, now ranking as one of the most costly in medicine, long-term sustainability of highly active antiretroviral treatment (HAART) expenses became a major issue, especially in countries with universal access to care. Identification of determinants of higher HAART costs may therefore help in controlling costs of care, while keeping high levels of retention in care and viral suppression.
Methods
With this aim, we enrolled a large multicentric sample of consecutive unselected human immunodeficiency virus (HIV) patients followed at five sites of care in Italy, and evaluated annual individual HAART costs in relation to a number of sociodemographic, clinical, and laboratory variables.
Results
We enrolled 2,044 patients, including 1,902 on HAART. Mean HAART costs were €9,377±€3,501 (range 782–29,852) per year, with remarkable site-based differences, possibly related to the different composition of local assisted populations. Percentages of patients on viral suppression were homogeneously high across all study sites. The factors identified by cross-validation were line of HAART, diagnosis of acquired immune deficiency syndrome, current CD4 T-cell count, and detectable HIV viremia >50 copies/mL. In the final multivariable model, HAART costs were independently directly associated with more advanced HAART line (P<0.001) and inversely correlated with current CD4 T-cell count (P=0.024). Site of care held independent prediction of higher costs, with marked control of expenses at sites 2 (P=0.001) and 5 (P<0.001).
Conclusion
Higher costs of HAART were strongly associated with previous treatment failures, detectable HIV viremia, and lower CD4 T-cell count at the time of evaluation, with no correlation at all with sex, age, hepatitis C virus coinfection, and nadir CD4 T-cell counts. Newer drugs, which are typically those associated with high prices, at the time of the analysis were still prevalently prescribed to rescue and maintain viral suppression in patients with more complex treatment history. Further analyses of the contribution of the single drug/regimen to the estimated cost are warranted.
Supplementary material
The average squared error (ASE) evolved at each step until final step 4 was reached and inclusion of factors terminated because the ten error estimates computed by cross-validation (CV PRESS) stopped showing a decrease using the “one-standard-error” rule. The values for the adjusted R-square at the various steps were the following: 0.146, 0.152, 0.154 and 0.155 at step 4 which was the optimal value criterion, indicated with a star symbol in the plot. The values for the standard R-square were similar (not shown). Step 4 was the chosen closing step for the chosen fit criteria of CV PRESS and for most of other fit criteria considered (eg, the adjusted R-square) or the previous step, removing current CD4 count (Akaike’s Information Criterion [AIC], Bayesian Information Criterion [BIC], see ). The ASE was greatly reduced when the line of highly active antiretroviral therapy (HAART) first entered the model. The plot of the improvements in ASE after the introduction of the various factors is key as it shows that the model including the line of therapy alone is a substantial improvement compared to the null model (with intercept only) but that adding any of the other factors determines only further small improvements to the predictive performance. These minor changes of the ASE were seen on the training, validation, and test datasets (data not shown). Results were similar when we used other methods of selection, such as least absolute shrinkage and selection operator (LASSO) or least angle regression (LAR).
Figure S1 Evolution of the ASE at each step of the GLMSELECT procedure. Factors were included in the analysis as follows: HAART line (HAARTl), AIDS event (AIDS), detectable HIV viral load (vl_20), and current CD4 count (cd4_100).
Abbreviations: Adj R-Sq, adjusted R-squared; ASE, average squared error; AIC, Akaike’s Infor ma tion Criterion; BIC, Bayesian Information Criterion; HAART, highly active antiretroviral therapy; LASSO, least absolute shrinkage and selection operator; LAR, least angle regression; AICC, corrected Akaike’s information criterion; C(p), Mallows statistic; SBC, Schwarz Bayesian information criterion.
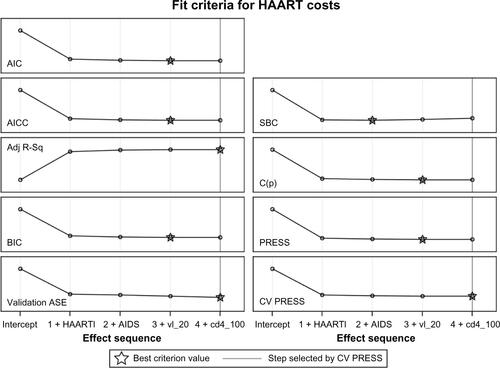
Acknowledgments
We are very grateful to the Fondazione Onlus Camillo de Lellis per l’Innovazione e la Ricerca in Medicina, Pescara, Italy, which supported us throughout this study.
Disclosure
The authors report no conflicts of interest in this work.