Abstract
Purpose
To investigate the predictive value of various inflammatory biomarkers in patients with acute ischemic stroke (AIS) and evaluate the relationship between stroke-associated pneumonia (SAP) and the best predictive index.
Patients and Methods
We calculated the neutrophil-to-lymphocyte ratio (NLR), platelet-to-lymphocyte ratio (PLR), monocyte-to-lymphocyte ratio (MLR), prognostic nutritional index (PNI), systemic inflammation response index (SIRI), systemic immune inflammation index (SII), Glasgow prognostic score (GPS), modified Glasgow prognostic score (mGPS), and prognostic index (PI). Variables were selectively included in the logistic regression analysis to explore the associations of NLR, PLR, MLR, PNI, SIRI, SII, GPS, mGPS, and PI with SAP. We assessed the predictive performance of biomarkers by analyzing receiver operating characteristic (ROC) curves. We further used restricted cubic splines (RCS) to investigate the association. Next, we conducted subgroup analyses to investigate whether specific populations were more susceptible to NLR.
Results
NLR, PLR, MLR, SIRI, SII, GPS, mGPS, and PI increased significantly in SAP patients, and PNI was significantly decreased. After adjustment for potential confounders, the association of inflammatory biomarkers with SAP persisted. NLR showed the most favorable discriminative performance and was an independent risk factor predicting SAP. The RCS showed an increasing nonlinear trend of SAP risk with increasing NLR. The AUC of the combined indicator of NLR and C-reactive protein (CRP) was significantly higher than those of NLR and CRP alone (DeLong test, P<0.001). Subgroup analyses suggested good generalizability of the predictive effect.
Conclusion
NLR, PLR, MLR, PNI, SIRI, SII, GPS, mGPS, and PI can predict the occurrence of SAP. Among the indices, the NLR was the best predictor of SAP occurrence. It can therefore be used for the early identification of SAP.
Introduction
Stroke is a leading cause of death and disability worldwide.Citation1 The Global Burden of Diseases, Injuries, and Risk Factors Study (GBD) showed that the annual number of strokes and deaths due to stroke increased substantially from 1990 to 2019.Citation2 The overall age-standardized prevalence, incidence, and mortality rates for stroke of the Chinese population aged 40 years or older in 2020 were 2.6%, 505.2 per 100,000 person-years, and 343.4 per 100,000 person-years, respectively.Citation3,Citation4 Stroke-associated pneumonia (SAP) is a prevalent medical complication in patients with acute ischemic stroke (AIS), having an estimated incidence ranging from 5% to 26%.Citation5 SAP can substantially reduce the quality of life and increase treatment costs, hospital stays, and mortality risk in AIS patients.Citation6–10 Infectious complications remain a major challenge in the treatment of stroke patients.Citation11 Therefore, there is a crucial need to identify high-risk patients to improve their clinical outcomes.
Several common inflammatory biomarkers, including the neutrophil-to-lymphocyte ratio (NLR), platelet-to-lymphocyte ratio (PLR), monocyte-to-lymphocyte ratio (MLR), prognostic nutritional index (PNI), systemic inflammation response index (SIRI), systemic immune-inflammation index (SII), Glasgow Prognostic Score (GPS), modified Glasgow Prognostic Score (mGPS) and prognostic index (PI), have been reported to have predictive value in different fields. Some of these inflammatory biomarkers have demonstrated they are associated with SAP and superior predictive value compared to conventional inflammatory biomarkers.Citation12–15 Studies have identified some of the emerging immune markers for predicting other diseases,Citation16–18 but they have not been used in predicting SAP.
These inflammatory biomarkers can be easily quantified by collecting peripheral blood samples, and they offer several advantages, including simplicity, minimal invasiveness, and affordability. However, it remains uncertain which inflammatory biomarker could be the most reliable predictor of SAP. Furthermore, optimal cutoff values have not yet been established for these inflammatory biomarkers, and the most reliable inflammatory biomarker and cutoff values for predicting SAP may vary.
Instead of focusing on a single inflammatory biomarker, the aim of this study was to assess the ability of various inflammatory biomarkers, including NLR, PLR, MLR, PNI, SIRI, SII, GPS, mGPS, and PI, to predict the occurrence of SAP in patients with AIS upon admission and to investigate their potential utility in identifying SAP at an early stage, thus providing a comprehensive picture of the predictive values of these biomarkers in patients with AIS. Additionally, we aimed to identify the best predictive index and explore its correlation with SAP.
Materials and Methods
Study Population
In this retrospective cohort study, AIS patients from the Second Affiliated Hospital of Nanchang University between January 2018 and December 2021 were selected. The study was approved by the Medical Ethics Committee of the Second Affiliated Hospital of Nanchang University.
Patients were included if they met the following requirements: they had to be 18 years of age or older, be admitted to the hospital within 48 hours of the onset of AIS symptoms, and have a confirmed diagnosis of AIS on DW-MRI according to the World Health Organization guidelines.Citation19,Citation20 Patients were excluded if any of the following criteria were met: 1) they had pulmonary edema, pulmonary embolism, pulmonary atelectasis, tuberculosis, pulmonary tumor, or noninfective interstitial lung disease, which have clinical symptoms similar to pneumonia; 2) they had pneumonia prior to admission; or 3) they had incomplete clinical data. Finally, a total of 1543 eligible patients were included ().
Diagnostic Criteria for SAP
SAP diagnosis was made within the first 7 days of admission based on the Centers for Disease Control and Prevention criteria (CDC).Citation21 According to the diagnostic standards indicated above, patients were classified into the SAP and non-SAP groups.
Data Collection
The following data were collected from the patients: demographics (age, sex), past history (drinking, smoking), comorbidities (atrial fibrillation, hypertension), treatments and the National Institute of Health Stroke Scale (NIHSS, reflects the severity of the stroke) score. Laboratory tests (leukocytes, neutrophils, lymphocytes, monocytes, erythrocytes, hemoglobin, platelet, albumin, C-reactive protein (CRP)) were performed within 24 hours of admission. The following inflammatory biomarkers were calculated: NLR, PLR, MLR, PNI, SIRI, SII, GPS, mGPS, and PI. The constituents and calculation of these nine inflammatory biomarkers are listed in .
Table 1 Inflammatory Biomarkers
Statistical Analysis
We conducted statistical analyses using R software (version 4.2.1) and IBM SPSS software (version 26.0). Normally distributed variables are reported as the mean ± standard deviation (SD), while nonnormally distributed variables are reported as the median (first quartile, third quartile). We employed Student’s t-test to compare normally distributed variables between the SAP and non-SAP groups and the Mann‒Whitney U-test for nonnormally distributed variables. Categorical variables are reported as numbers (percentage), and we used the chi-squared (χ2) test for comparison. To compare the predictive performance of all nine inflammatory biomarkers, we calculated the area under the receiver operating characteristic (ROC) curve (AUC) and determined which cutoff point of each one maximized the Youden index. We employed multivariable logistic regression analysis to identify independent determinants for SAP and presented the findings in a forest plot. We also employed restricted cubic splines (RCS) with four knots at the 5th, 35th, 65th, and 95th centiles to flexibly model the association of the NLR with SAP. Finally, we conducted subgroup analyses in groups stratified by age (<60 or ≥60 years), sex, risk factors (drinking, smoking, atrial fibrillation, and hypertension), stroke severity (NIHSS score on admission ≤4 or >4), and treatments (intravenous thrombolysis, endovascular thrombectomy, conservative treatment). ROCs were compared with the DeLong test.
Results
Comparison of Clinical Characteristics
In this study, a total of 1543 patients (mean/sd age 65.19/11.97 years) were included, comprising 558 (36.16%) females and 985 (63.84%) males. Out of all patients, 184 (11.92%) developed SAP. Patients in the SAP group had higher age, NIHSS scores, more atrial fibrillation, more intravenous thrombolysis and endovascular thrombectomy, and significantly elevated levels of leukocytes, neutrophils, monocytes, and CRP, while the levels of lymphocytes, erythrocytes, hemoglobin, and albumin were significantly lower in the SAP group than in the non-SAP group (P < 0.05) ().
Table 2 Comparisons of Clinical Characteristics
Inflammatory Biomarkers of the Study Population
The median (IQR) values of NLR, PLR, MLR, PNI, SIRI, and SII in the SAP group and the non-SAP group are presented in . Patients in the SAP group showed a significantly higher NLR (P<0.001), higher PLR (P<0.001), higher MLR (P<0.001), higher SIRI (P<0.001), higher SII (P<0.001) and lower PNI (P<0.001) (). ROC curve analysis was conducted to determine the optimal cutoff values of these inflammatory biomarkers in relation to the occurrence of SAP in patients with AIS. The ROC curve analyses yielded the following optimal cutoff values for the continuous inflammatory biomarkers: 3.287 for NLR, 160.484 for PLR, 0.471 for MLR, 40.600 for PNI, 2.357 for SIRI, and 809.678 for SII. Based on these cutoff values, the patients were classified into two groups; the details of the inflammatory biomarkers, along with the number of patients in each group, are summarized in .
Figure 2 Violin plots of NLR (A), PLR (B), MLR (C), SIRI (D), SII (E) and PNI (F) in the non-SAP and SAP groups.
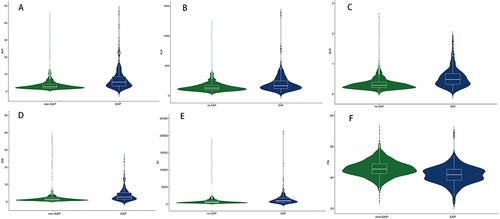
Variables were selectively included in the logistic regression analysis to explore the associations of NLR, PLR, MLR, PNI, SIRI, SII, GPS, mGPS, and PI with SAP. Model 1 was unadjusted; Model 2 was adjusted for demographic factors, including age and sex; and Model 3 was adjusted for all factors in model 2 and traditional or clinical risk factors including atrial fibrillation, hypertension, NIHSS, smoking, and drinking. Model 4 was adjusted for all factors in model 3 and treatments including endovascular thrombectomy, intravenous thrombolysis and conservative treatment. After adjustment for potential confounders, the association of inflammatory biomarkers with SAP persisted (as shown in ).
Table 3 Associations of Inflammatory Biomarkers with SAP
Comparison of the Predictive Value of Inflammatory Biomarkers
Inflammatory biomarkers, including NLR, PLR, MLR, PNI, SIRI, SII, GPS, mGPS, and PI, were computed using laboratory parameters obtained from peripheral blood samples. The ability of these inflammatory biomarkers to predict the incidence of SAP was evaluated as the AUC values. NLR had the highest AUC among all the inflammatory biomarkers, with a value of 0.737 (95% CI 0.697–0.776, P<0.001) (, ).
Table 4 ROC Curve Analysis of Inflammatory Biomarkers
Multivariate Logistic Regression Analysis of NLR for SAP
The patients were categorized into high-NLR (NLR≥3.287) and low-NLR groups (NLR<3.287) using the optimal cutoff value for NLR determined by the ROC curve analysis.
In comparison to the low-NLR group, patients in the high-NLR group had a significantly greater risk of SAP (OR: 4.733, 95% CI: 3.352, 6.683, P<0.001). and show that this link remained significant even after adjusting for potential confounding factors that were significantly different in the univariate analysis (OR: 2.453, 95% CI: 1.618, 3.718, P<0.001). In addition, age, atrial fibrillation, NIHSS score, and CRP were significant factors increasing the likelihood of SAP, according to the multivariate regression analysis (; ).
Table 5 Comparisons of Characteristics Between the SAP and Non-SAP Groups by Multivariate Logistic Analysis
The Nonlinear Relationship Between the NLR and SAP Risk
The plot demonstrated a nonlinear relationship between NLR and the risk of SAP (P for nonlinearity < 0.001, ). At an NLR value of 2.881, the odds ratio (OR) was 1. The RCS model demonstrated that the risk of SAP increased significantly with increasing NLR when the NLR was above 2.325, and the risk remained stable at NLR levels above 11.139.
Subgroup Analysis
Next, we conducted subgroup analyses to investigate whether specific populations had SAP that were more susceptible to NLR. We assessed the effect modification of the association between NLR and SAP from drinking (yes or no), smoking (yes or no), NIHSS (NIHSS ≤ 4 and NIHSS > 4), hypertension (yes or no), atrial fibrillation (yes or no), age (age < 60 and age ≥ 60), sex (male and female) and treatment (intravenous thrombolysis, endovascular thrombectomy, and conservative treatment). The results indicated that the association between the NLR and SAP was not significantly influenced by any of these factors (all P for interaction>0.05), suggesting a good generalizability of the predictive effect ().
The Combined Use of NLR and CRP for Predicting the Occurrence of SAP
Due to the convenience of obtaining NLR and CRP through routine laboratory tests, as well as they are independent factors associated with SAP, coupled with the absence of a consensus regarding the optimal cutoff value for NLR and CRP in predicting SAP, we calculated the optimal cutoff value using ROC analysis. NLR values were categorized into high-NLR (NLR≥3.287) and low-NLR (NLR<3.287) using the optimal cutoff value for NLR determined by ROC curve analysis. The values of CRP were categorized into high CRP (CRP≥7.11) and low CRP (CRP<7.11) using the optimal cutoff value for CRP determined by the ROC curve analysis. For ease of use in practice, based on the values of NLR and CRP, patients were categorized into mild (low NLR and low CRP), moderate (high NLR or high CRP alone), and severe (high NLR and high CRP) groups.
We used NLR and CRP and the combination of NLR and CRP to predict SAP. Among these three indicators, the combined indicator had the highest AUC of 0.739 (compared with NLR alone and CRP alone, both P<0.001, DeLong test) (). Therefore, the combined indicator of NLR and CRP was found to be a new predictive indicator for SAP in AIS patients.
When this new predictive indicator was added to multivariate logistic regression analysis, compared to the mild group, the moderate group (unadjusted OR: 2.669, 95% CI: 1.725, 4.130; adjusted OR: 1.839, 95% CI: 1.146, 2.953, P=0.012) and the severe group (unadjusted OR: 11.297, 95% CI: 7.358, 17.346; adjusted OR: 5.166, 95% CI: 3.091, 8.635, P<0.001) were found to be associated with SAP significantly in both unadjusted and adjusted models.
Discussion
SAP is a serious complication that can result in physical harm to AIS patients. Therefore, timely identification of SAP is crucial in preventing adverse outcomes.Citation22,Citation23 In this retrospective study, our aim was to examine the predictive abilities of easily accessible inflammatory biomarkers for the occurrence of SAP and compare their predictive capacities. Therefore, our study included multiple inflammatory biomarkers, and we found that the NLR, PLR, MLR, SIRI, SII, GPS, mGPS and PI were significantly elevated and PNI was significantly decreased in SAP patients. The results of this study have important implications for clinical practice, by calculating these biomarkers in AIS patients, we can identify those at risk of SAP, provide a basis for early anti-infection intervention, and achieve the purpose of improving prognosis.
Moreover, previous studies did not explore the potential nonlinear relationship between the NLR and SAP. Therefore, we examined the relationship between NLR and SAP and specifically investigated the possibility of a nonlinear relationship between the two. NLR, as a marker of inflammation, has been the focus of increasing attention due to its ability to reflect the balance between the innate and adaptive immune responses.Citation24 Our study identified that NLR showed the most promising discriminative performance in predicting the occurrence of SAP compared to the other biomarkers. In addition, the relationship between NLR and SAP risk may be complex and variable. Specifically, we observed that the risk of SAP increased steeply with increasing NLR when NLR was greater than 2.325 and then increased with relative stability when NLR was greater than 11.139. Furthermore, we identified the optimal cutoff value for the NLR to be 3.287.
Several studies have explored the “normal” values of NLR in a healthy adult population, but normal values differ in pathological states or processes such as cancer, atherosclerosis, infection, and inflammation. A normal range of NLR is between 1.0 and 2.0, while values higher than 3.0 and below 0.7 in adults are considered pathological.Citation25 Therefore, in this study, we concluded that NLR levels between 2.325 and 2.881 may serve as an early warning sign of SAP. AIS patients with elevated NLR levels (NLR≥3.287) should be made aware of their increased risk for SAP. There are several possible explanations for the aforementioned findings.
Initially, neutrophils release neutrophil extracellular traps (NETs), which can form clots in the lungs and activate an inflammatory response, leading to lung tissue damage.Citation26,Citation27 The change in lymphocyte count may be related to the patient’s immune status.Citation28 A moderate increase in lymphocytes can help prevent the occurrence and worsening of infections in the body.Citation29–31 An increase in lymphocyte count may be associated with a better prognosis and a lower incidence of complications.Citation32 Increased lymphocytes regulate the activity of neutrophils and prevent inflammatory overreaction, thereby reducing the risk of lung infection.
However, once the NLR reaches a certain threshold, it may lead to immunosuppression. The notion that poststroke infection is largely caused by stroke-induced immunodepression syndrome is supported by both experimental and clinical evidence.Citation33–37 Neutrophils are highly enriched in tissue-destructive proteases. In inflammatory and airway diseases such as asthma and chronic obstructive pulmonary disease, the activation of neutrophils is an important process. Meanwhile, it can lead to the release of several enzymes, including myeloperoxidase and neutrophil elastase.Citation38 Myeloperoxidase release can induce damage to resident lung cells by increasing oxidative stress and causing DNA damage, thereby disrupting cellular homeostasis and promoting cell damage, ultimately eliciting proinflammatory responses in human lung epithelial cells.Citation39,Citation40 The respiratory epithelial cell structure may change as a result of neutrophil elastase, and increased local mucin secretion and other factors may also contribute to airway obstruction.Citation41,Citation42 Decreased neutrophil counts can result in reduced immunity.Citation28 A rapid decrease in the number of lymphocytes can lead to the downregulation of the immune response, thereby increasing the risk of SAP.Citation33,Citation35–37
Another explanation is that these markers may indirectly affect the risk of developing SAP by affecting the brain damage after a stroke. Higher levels of NETs and neutrophils have been associated with worse clinical outcomes in AIS, which could exacerbate ischemic brain damage.Citation43 Other studies have demonstrated a close relationship between the NLR and severe stroke.Citation44,Citation45 Stroke severity and large infarct volumes are widely recognized as significant risk factors for the development of SAP,Citation46 and exacerbating injury to a certain extent increases the risk of SAP.
CRP is a protein synthesized by the liver. CRP increased the diagnostic accuracy of SAP in afebrile patients.Citation47 Therefore, on the premise that both indicators are independent predictors of SAP, we proposed a new predictive indicator that combined NLR and CRP for predicting the occurrence of SAP. We found that combined indicator was more predictive than either sole indicator. It can be used as a new indicator to help predict the incidence of SAP.
There are several limitations that should be acknowledged in our study. First, our study’s cross-sectional design prevented us from defining a causal connection between the NLR and SAP. Second, we were limited in our ability to track changes in the NLR and other inflammatory biomarkers throughout hospitalization as we only measured these markers once upon admission. Finally, this was a single-centre study and may suffer from selection bias. Therefore, further studies are needed to confirm our findings.
Conclusion
The results of this study suggest that the NLR, PLR, MLR, PNI, SIRI, SII, GPS, mGPS, and PI are potential predictors of SAP occurrence in AIS patients. Among these biomarkers, the NLR exhibited the best predictive performance. Furthermore, the relationship between the NLR and SAP was nonlinear. As NLR is a readily available and cost-effective indicator, it can be used for the early identification of SAP. The combination of NLR and CRP can be a new indicator to help predict the incidence of SAP.
Ethics Statement
This study received ethical approval from the Medical Ethics Committee of the Second Affiliated Hospital of Nanchang University and complied with the Declaration of Helsinki. Due to the retroactive nature of the investigation, the review committee relaxed the necessity for written informed consent. We eliminated all private patient data before examining the data.
Author Contributions
All authors made a significant contribution to the work reported, whether that is in the conception, study design, execution, acquisition of data, analysis and interpretation, or in all these areas; took part in drafting, revising or critically reviewing the article; gave final approval of the version to be published; have agreed on the journal to which the article has been submitted; and agree to be accountable for all aspects of the work.
Disclosure
The authors report no conflicts of interest in this work.
Additional information
Funding
References
- Campbell BCV, Khatri P. Stroke. Lancet. 2020;396(10244):129–142. doi:10.1016/S0140-6736(20)31179-X
- Feigin VL, Stark BA, Johnson CO; Collaborators GBDS. Global, regional, and national burden of stroke and its risk factors, 1990–2019: a systematic analysis for the Global Burden of Disease Study 2019. Lancet Neurol. 2021;20(10):795–820. doi:10.1016/S1474-4422(21)00252-0
- Tu WJ, Zhao Z, Yin P, et al. Estimated Burden of Stroke in China in 2020. JAMA Netw Open. 2023;6(3):e231455. doi:10.1001/jamanetworkopen.2023.1455
- Tu WJ, Wang LD; Special Writing Group of China Stroke Surveillance R. China stroke surveillance report 2021. Mil Med Res. 2023;10(1):33. doi:10.1186/s40779-023-00463-x
- Finlayson O, Kapral M, Hall R, et al. Risk factors, inpatient care, and outcomes of pneumonia after ischemic stroke. Neurology. 2011;77(14):1338–1345. doi:10.1212/WNL.0b013e31823152b1
- Koennecke HC, Belz W, Berfelde D, et al. Factors influencing in-hospital mortality and morbidity in patients treated on a stroke unit. Neurology. 2011;77(10):965–972. doi:10.1212/WNL.0b013e31822dc795
- Katzan IL, Cebul RD, Husak SH, et al. The effect of pneumonia on mortality among patients hospitalized for acute stroke. Neurology. 2003;60(4):620–625. doi:10.1212/01.WNL.0000046586.38284.60
- Feigin VL, Nguyen G. Collaborators GBDLRoS. Global, regional, and country-specific lifetime risks of stroke, 1990 and 2016. N Engl J Med. 2018;379(25):2429–2437.
- Vermeij FH, Scholte op Reimer WJ, de Man P, et al. Stroke-associated infection is an independent risk factor for poor outcome after acute ischemic stroke: data from the Netherlands Stroke Survey. Cerebrovasc Dis. 2009;27(5):465–471. doi:10.1159/000210093
- Forti P, Maioli F, Procaccianti G, et al. Independent predictors of ischemic stroke in the elderly: prospective data from a stroke unit. Neurology. 2013;80(1):29–38. doi:10.1212/WNL.0b013e31827b1a41
- Langhorne P, Stott DJ, Robertson L, et al. Medical complications after stroke: a multicenter study. Stroke. 2000;31(6):1223–1229. doi:10.1161/01.STR.31.6.1223
- Nam KW, Kim TJ, Lee JS, et al. High neutrophil-to-lymphocyte ratio predicts stroke-associated pneumonia. Stroke. 2018;49(8):1886–1892. doi:10.1161/STROKEAHA.118.021228
- Yan D, Dai C, Xu R, Huang Q, Ren W. Predictive ability of systemic inflammation response index for the risk of pneumonia in patients with acute ischemic stroke. Gerontology. 2023;69(2):181–188. doi:10.1159/000524759
- Zawiah M, Hayat Khan A, Abu Farha R, Usman A, Bitar AN. Neutrophil-lymphocyte ratio, monocyte-lymphocyte ratio, and platelet-lymphocyte ratio in stroke-associated pneumonia: a systematic review and meta-analysis. Curr Med Res Opin. 2023;39(3):475–482. doi:10.1080/03007995.2023.2174327
- Cao F, Wan Y, Lei C, et al. Monocyte-to-lymphocyte ratio as a predictor of stroke-associated pneumonia: a retrospective study-based investigation. Brain Behav. 2021;11(6):e02141. doi:10.1002/brb3.2141
- Forrest LM, McMillan DC, McArdle CS, Angerson WJ, Dunlop DJ. Evaluation of cumulative prognostic scores based on the systemic inflammatory response in patients with inoperable non-small-cell lung cancer. Br J Cancer. 2003;89(6):1028–1030. doi:10.1038/sj.bjc.6601242
- Proctor MJ, Morrison DS, Talwar D, et al. An inflammation-based prognostic score (mGPS) predicts cancer survival independent of tumour site: a Glasgow Inflammation Outcome Study. Br J Cancer. 2011;104(4):726–734. doi:10.1038/sj.bjc.6606087
- Kasymjanova G, MacDonald N, Agulnik JS, et al. The predictive value of pre-treatment inflammatory markers in advanced non-small-cell lung cancer. Curr Oncol. 2010;17(4):52–58. doi:10.3747/co.v17i4.567
- Mendelson SJ, Prabhakaran S. Diagnosis and management of transient ischemic attack and acute ischemic stroke: a review. JAMA. 2021;325(11):1088–1098. doi:10.1001/jama.2020.26867
- Stroke WH. Recommendations on stroke prevention, diagnosis, and therapy. Report of the WHO task force on stroke and other cerebrovascular disorders. Stroke. 1989;20(10):1407–1431. doi:10.1161/01.STR.20.10.1407
- Smith CJ, Kishore AK, Vail A, et al. Diagnosis of Stroke-associated pneumonia: recommendations from the pneumonia in Stroke Consensus Group. Stroke. 2015;46(8):2335–2340. doi:10.1161/STROKEAHA.115.009617
- Teh WH, Smith CJ, Barlas RS, et al. Impact of stroke-associated pneumonia on mortality, length of hospitalization, and functional outcome. Acta Neurol Scand. 2018;138(4):293–300. doi:10.1111/ane.12956
- Walter U, Knoblich R, Steinhagen V, Donat M, Benecke R, Kloth A. Predictors of pneumonia in acute stroke patients admitted to a neurological intensive care unit. J Neurol. 2007;254(10):1323–1329. doi:10.1007/s00415-007-0520-0
- Buonacera A, Stancanelli B, Colaci M, Malatino L. Neutrophil to lymphocyte ratio: an emerging marker of the relationships between the immune system and diseases. Int J Mol Sci. 2022;23(7):3636. doi:10.3390/ijms23073636
- Zahorec R. Neutrophil-to-lymphocyte ratio, past, present and future perspectives. Bratisl Lek Listy. 2021;122(7):474–488. doi:10.4149/BLL_2021_078
- Cavalcante-Silva LHA, Carvalho DCM, Lima EA, et al. Neutrophils and COVID-19: the road so far. Int Immunopharmacol. 2021;90:107233. doi:10.1016/j.intimp.2020.107233
- Brinkmann V, Reichard U, Goosmann C, et al. Neutrophil extracellular traps kill bacteria. Science. 2004;303(5663):1532–1535. doi:10.1126/science.1092385
- Shim R, Wong CHS, Wong CH. Ischemia, immunosuppression and infection--tackling the predicaments of post-stroke complications. Int J Mol Sci. 2016;17(1):64. doi:10.3390/ijms17010064
- Belay T, Woart A. Cold-induced stress increases the intensity of Chlamydia genital infection in mice. J Microbiol Immunol Infect. 2013;46(5):330–337. doi:10.1016/j.jmii.2012.06.002
- Leschiera E, Lorenzi T, Shen S, Almeida L, Audebert C. A mathematical model to study the impact of intra-tumour heterogeneity on anti-tumour CD8(+) T cell immune response. J Theor Biol. 2022;538:111028. doi:10.1016/j.jtbi.2022.111028
- Cronkite DA, Strutt TM. The regulation of inflammation by innate and adaptive lymphocytes. J Immunol Res. 2018;2018:1467538. doi:10.1155/2018/1467538
- Xiao J, Qiu QW, Qin C, et al. Dynamic changes of peripheral blood lymphocyte subsets in acute ischemic stroke and prognostic value. Brain Behav. 2021;11(1):e01919. doi:10.1002/brb3.1919
- Prass K, Meisel C, Hoflich C, et al. Stroke-induced immunodeficiency promotes spontaneous bacterial infections and is mediated by sympathetic activation reversal by poststroke T helper cell type 1-like immunostimulation. J Exp Med. 2003;198(5):725–736. doi:10.1084/jem.20021098
- Offner H, Subramanian S, Parker SM, Afentoulis ME, Vandenbark AA, Hurn PD. Experimental stroke induces massive, rapid activation of the peripheral immune system. J Cereb Blood Flow Metab. 2006;26(5):654–665. doi:10.1038/sj.jcbfm.9600217
- Klehmet J, Harms H, Richter M, et al. Stroke-induced immunodepression and post-stroke infections: lessons from the preventive antibacterial therapy in stroke trial. Neuroscience. 2009;158(3):1184–1193. doi:10.1016/j.neuroscience.2008.07.044
- Dirnagl U, Klehmet J, Braun JS, et al. Stroke-induced immunodepression: experimental evidence and clinical relevance. Stroke. 2007;38(2 Suppl):770–773. doi:10.1161/01.STR.0000251441.89665.bc
- Iadecola C, Anrather J. The immunology of stroke: from mechanisms to translation. Nat Med. 2011;17(7):796–808. doi:10.1038/nm.2399
- Lacy P. Mechanisms of degranulation in neutrophils. Allergy Asthma Clin Immunol. 2006;2(3):98–108. doi:10.1186/1710-1492-2-3-98
- Davies MJ, Hawkins CL. The Role of Myeloperoxidase in Biomolecule Modification, Chronic Inflammation, and Disease. Antioxid Redox Signal. 2020;32(13):957–981. doi:10.1089/ars.2020.8030
- Haegens A, Vernooy JH, Heeringa P, Mossman BT, Wouters EF. Myeloperoxidase modulates lung epithelial responses to pro-inflammatory agents. Eur Respir J. 2008;31(2):252–260. doi:10.1183/09031936.00029307
- Hao W, Li M, Zhang Y, Zhang C, Wang P. Severity of chronic obstructive pulmonary disease with ‘exacerbator with emphysema phenotype’ is associated with potential biomarkers. Postgrad Med J. 2020;96(1131):28–32. doi:10.1136/postgradmedj-2019-136599
- Roghanian A, Sallenave JM. Neutrophil elastase (NE) and NE inhibitors: canonical and noncanonical functions in lung chronic inflammatory diseases (cystic fibrosis and chronic obstructive pulmonary disease). J Aerosol Med Pulm Drug Deliv. 2008;21(1):125–144. doi:10.1089/jamp.2007.0653
- Novotny J, Oberdieck P, Titova A, et al. Thrombus NET content is associated with clinical outcome in stroke and myocardial infarction. Neurology. 2020;94(22):e2346–e2360. doi:10.1212/WNL.0000000000009532
- Tokgoz S, Keskin S, Kayrak M, Seyithanoglu A, Ogmegul A. Is neutrophil/lymphocyte ratio predict to short-term mortality in acute cerebral infarct independently from infarct volume? J Stroke Cerebrovasc Dis. 2014;23(8):2163–2168. doi:10.1016/j.jstrokecerebrovasdis.2014.04.007
- Xue J, Huang W, Chen X, et al. Neutrophil-to-lymphocyte ratio is a prognostic marker in acute ischemic stroke. J Stroke Cerebrovasc Dis. 2017;26(3):650–657. doi:10.1016/j.jstrokecerebrovasdis.2016.11.010
- Hannawi Y, Hannawi B, Rao CP, et al. Stroke-associated pneumonia: major advances and obstacles. Cerebrovasc Dis. 2013;35(5):430–443. doi:10.1159/000350199
- Kalra L, Smith CJ, Hodsoll J, Vail A, Irshad S, Manawadu D. Elevated C-reactive protein increases diagnostic accuracy of algorithm-defined stroke-associated pneumonia in afebrile patients. Int J Stroke. 2019;14(2):167–173. doi:10.1177/1747493018798527