Abstract
Medicare is the federal health insurance program for individuals in the US who are aged ≥65 years, select individuals with disabilities aged <65 years, and individuals with end-stage renal disease. The Centers for Medicare and Medicaid Services grants researchers access to Medicare administrative claims databases for epidemiologic and health outcomes research. The data cover beneficiaries’ encounters with the health care system and receipt of therapeutic interventions, including medications, procedures, and services. Medicare data have been used to describe patterns of morbidity and mortality, describe burden of disease, compare effectiveness of pharmacologic therapies, examine cost of care, evaluate the effects of provider practices on the delivery of care and patient outcomes, and explore the health impacts of important Medicare policy changes. Considering that the vast majority of US citizens ≥65 years of age have Medicare insurance, analyses of Medicare data are now essential for understanding the provision of health care among older individuals in the US and are critical for providing real-world evidence to guide decision makers. This review is designed to provide researchers with a summary of Medicare data, including the types of data that are captured, and how they may be used in epidemiologic and health outcomes research. We highlight strengths, limitations, and key considerations when designing a study using Medicare data. Additionally, we illustrate the potential impact that Centers for Medicare and Medicaid Services policy changes may have on data collection, coding, and ultimately on findings derived from the data.
Introduction
Medicare is the federal health insurance program for individuals in the US who are aged ≥65 years, select individuals with disabilities aged <65 years who have been receiving Social Security or Railroad Retirement Board benefits, and individuals with end-stage renal disease (ESRD). Medicare was officially established in 1965 when US President Lyndon B Johnson signed into law a congressional bill authorizing the program for the elderly population. By 1966, ~19.1 million Americans were covered by Medicare. In 1972, the program was expanded to include the disabled, those with ESRD, and recipients of certain organ transplants. By 2015 – the program’s 50th anniversary–more than 55 million beneficiaries were covered.Citation1 While claims data on Medicare beneficiaries have been primarily collected for payment purposes over the last half-century, it has been leveraged to conduct a wide variety of health care research and surveillance projects informing major health care and public policy decisions.
The Centers for Medicare and Medicaid Services (CMS) grants researchers access to the Medicare administrative claims databases for epidemiologic and health outcomes research. The data cover beneficiaries’ encounters with the health care system and receipt of therapeutic interventions, including medications, procedures, and services. Requests for data access are submitted to CMS via the Research Data Assistance Center (ResDAC), which reviews the request for scientific merit and technical feasibility. If sound, the request is then sent to CMS for approval. If approved, CMS enters into a Data Use Agreement (DUA) with the researcher. These data have been used to describe patterns of morbidityCitation2 and mortalityCitation3 and burden of disease,Citation4,Citation5 compare the effectiveness of pharmacologic therapies,Citation6–Citation9 examine the cost of care,Citation10–Citation13 evaluate the effects of provider practices on the delivery of care,Citation14–Citation17 and explore the effects of important policy changes on physician practices and patient outcomes.Citation18–Citation23 The use of the Medicare databases as sources for epidemiologic and health outcomes research has increased substantially over time (). Considering that the vast majority of US citizens ≥65 years of age have Medicare insurance, analyses of Medicare data are now essential for understanding the provision of health care among older individuals in the US ().
Figure 1 Number of publications using Medicare administrative claims by year, 1979–2016.
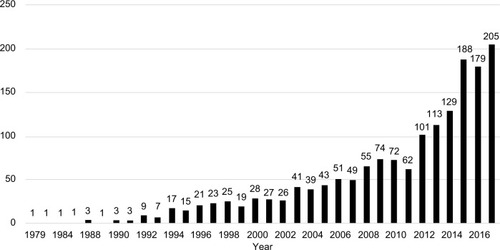
This review is designed to provide researchers with a summary of Medicare data, including the types of data that are captured, and how they may be used in epidemiologic and health outcomes research. We highlight strengths, limitations, and key considerations when designing a study using Medicare data. Additionally, we illustrate the potential impact that CMS policy changes may have on data collection, coding, and ultimately on findings derived from the data. For more information and training on Medicare data, readers should consult the ResDAC website.Citation24
Medicare population
Medicare beneficiaries are those US individuals who are ≥65 years of age, select individuals aged <65 years with a recognized disability who receive Social Security or Railroad Retirement Board benefits, and individuals with ESRD. The 65+ population accounts for 84% of covered individuals. In 2014, 16% of Medicare beneficiaries were <65 years old, 46% 65–74 years, 25% 75–84 years, and 12% ≥85 years.Citation25 Fifty-five percent of beneficiaries were female, 76% were white, 10% black, 9% Hispanic, and 5% Asian or other/unknown race.Citation25 These gender and race distributions are generally consistent with the 2010 US Census, which reports that 56% of the population aged ≥65 are females,Citation26 79% are white, 9% black, 7% Hispanic, and 5% Asian or other/unknown race.Citation27
Medicare coverage
Medicare health insurance is made up of several different types of coverage. Part A (“hospital insurance”) typically covers care provided in the inpatient setting. It may also cover care given in a skilled nursing facility (SNF), hospice, or home health care setting. Part B (“medical insurance”) covers physician services (e.g., procedures, injections, and diagnostic tests) whether rendered in the inpatient or outpatient setting, other outpatient care, medical supplies or durable medical equipment (e.g., oxygen tanks and wheelchairs), preventive services, and some home health care. Nearly all beneficiaries are enrolled in Part A and/or B coverage.Citation25 Since 2006, Medicare beneficiaries have had the option to purchase prescription drug coverage under the Part D plan. Approximately 70% of Medicare beneficiaries were enrolled in the Part D plan as of 2014.Citation25 Parts A, B, and D are available through traditional Medicare fee for service (FFS) plans.
Some beneficiaries elect to enroll in Medicare Part C plans, a program similar to a health maintenance organization, also referred to as Medicare Advantage (MA). Part C is a type of Medicare health plan offered by private companies that contract with Medicare to provide Parts A and B benefits (and often Part D). As of 2016, 31% of Medicare beneficiaries were enrolled in Part C.Citation28 Part C claims are separate from CMS Medicare data files. These claims are part of the private companies’ data that may be available to researchers via employer group health plans such as United Healthcare.
Medicare standard analytic files (SAFs)
The CMS produces annual SAF for researchers containing final (adjudicated) action claims for Parts A and B services and Part D prescription drugs, which reflect care received in the hospital, physician offices, SNFs, and hospice settings through December 31 of the latest available calendar year. These claims capture inpatient and outpatient diagnoses, procedures, devices, and medication information in the form of alphanumeric codes, dates of service, charged amounts and paid claims, and a limited number of provider characteristics, such as provider number and geographic information (state, county, and zip code) for facilities as well as national physician number and clinical specialty for physicians. SAFs also contain information on beneficiary enrollment and demographic data, including race, ethnicity, and date of death. To use Medicare data, qualified entities must enter into a legally binding DUA, which covers terms of use of the data, particularly in terms of privacy and patient protection. CMS provides these data to researchers organized into specific SAFs that are outlined in . Typically, there is a 2-year lag between the receipt of care and its appearance in a SAF. For example, 2015 is the most recent calendar year of data available to researchers in 2017.
Table 1 Medicare coverage and the corresponding research files available to researchers
Researchers can request data for a 5% or 20% sample of Medicare beneficiaries, or in some situations, 100% of Medicare beneficiaries (generally limited to specific disease cohorts). For the 5% and 20% “cuts”, beneficiaries are selected for inclusion in the database based on the last 2 digits of their health insurance claim number (which, in the vast majority of cases, is their social security number). Information on all beneficiaries included in the 5% or 20% SAF are provided for all years for which they have received Medicare benefits (until death or disenrollment) within the time period included as part of the DUA. Given continuous enrollment in the Medicare FFS, this type of data organization enables longitudinal follow-up of Medicare beneficiaries over multiple years. As mentioned earlier, 100% SAFs containing claims for a complete group of patients with a specific disease of interest, typically rare diseases, are available upon request, although prescription drug claims are only available among the subset with Part D coverage ().
Disease-specific Medicare datasets and linkages
In addition to the SAFs, CMS and other government agencies provide additional survey data, disease-specific databases, and linked databases with access to more detailed information regarding health care utilization and clinical characteristics of Medicare beneficiaries. While it is recognized that these linked datasets provide richer data, it should be noted that these files may be limited by the timing of the linkage (date/year), and the extent to which the linkage covers the entire population or a subset of a population (i.e., Surveillance, Epidemiology, and End Results [SEER]-Medicare-linked file includes only patients in the 18 SEER sites). Here, we highlight 3 of the available disease-specific Medicare datasets and linkages.
Medicare Current Beneficiary Survey (MCBS)
The MCBS is an annual survey of a representative sample of all Medicare beneficiaries. Information on health care coverage (including commercial insurance), expenditures and sources of payment for Medicare services, and socioeconomic and demographic characteristics are collected. The MCBS is linkable to each respondent’s Medicare claims. The objectives of the MCBS are to describe other forms of medical coverage for Medicare beneficiaries (e.g., Medicare supplemental insurance), assess non-covered expenditures for medical services (e.g., deductibles and copayments), and track sources of care and patient satisfaction over time. These data have been used, for example, to track out-of-pocket health care spending among low-income Medicare beneficiaries.Citation29
United States Renal Data System (USRDS)
The USRDS was established in 1989 and is funded by the National Institute of Diabetes and Digestive and Kidney Diseases, one of the US National Institutes of Health.Citation30 It is a national registry of ESRD patients receiving maintenance dialysis that, as part of their mission, offers researchers access to the data. In addition to the CMS Parts A, B, and D claims, the USRDS provides select clinical information for patients with ESRD receiving outpatient dialysis both at initiation of dialysis and upon patient death. While the claims file structure is slightly different than CMS SAFs, information contained in the files is largely the same, allowing researchers to conduct similar types of studies in ESRD patients.
SEER-Medicare
Medicare data can be linked to the SEER cancer registries, which collect clinical, demographic, and cause of death information for individuals with a validated diagnosis of cancer. These linked data create an enriched, population-based database that can be used to examine treatment patterns and clinical outcomes among patients with cancer.Citation31 These data include severity of disease and prognostic factors not available in standard Medicare claims files. A recent study identified ~1,360 publications between January 1993 and January 2016 that used the SEER-Medicare database to address important clinical or policy questions.Citation32
Strengths and limitations of the Medicare data
Medicare data have several strengths, making them valuable for research purposes. First, they are a large set of databases containing longitudinal information on health care services for more than 55 million US individuals as of 2015. As the population ages and individuals live longer,Citation33 this number will continue to grow. This means that these data are useful not only in the study of cardinal public health threats such as cardiovascular disease, diabetes, and cancer but also rare diseases. Second, nearly 98% of US individuals aged ≥65 years receive either Medicare FFS or MA insurance.Citation34 Conducting studies in one or the other of these databases should provide generalizable estimates to the broader FFS or MA populations, respectively. Third, withdrawal from Medicare is extremely rare, so once individuals are enrolled in Medicare, they are typically followed until death.
Fourth, because Medicare data reflect near-complete capture of health care services across all settings of care, the data can be used to answer a wide range of health care-related questions including: 1) understanding the epidemiology of a disease, 2) quantifying the costs related to health care interventions, 3) describing treatment utilization patterns and the delivery of health care services, 4) comparing the effectiveness, safety, and costs of interventions, and 5) studying the effects of policy changes on prescribing patterns and clinical outcomes. In doing so, broad changes in clinical practice patterns can be traced, evidence useful for developing and evaluating adherence to clinical practice guidelines can be generated, and the effects of CMS payment policy decisions can be evaluated.
There are, however, important limitations to the data available to researchers that require careful consideration. Although Medicare data capture longitudinal information on a substantial proportion of the population aged ≥65 years in the US, gaps do exist. First, information on behavioral characteristics such as diet and exercise, the use of alcohol and tobacco; laboratory test results for biochemical parameters such as albumin, serum creatinine, low-density lipoprotein, and HbA1c levels; and results of diagnostic tests such as biopsies, MRI/CT scans, and bone-density measurements (most of which guide treatment decisions); and other disease severity indicators such as cancer staging and evidence of cachexia are not available. As with most other claims databases, diseases are typically defined in Medicare data by the presence of a diagnostic code. This approach may be prone to misclassification depending on the type of disease under study, and the ability to distinguish mild from severe disease may be limited, unless other claims for services such as procedure (e.g., coronary artery bypass graft [CABG] surgery for atherosclerosis) or treatment (e.g., injectable antibiotics for more severe antibiotics) are included in the definition. Because many of these factors are prognostic indicators that can affect treatment decisions (i.e., potential confounders), comparative effectiveness and/or safety analyses performed using these data may be subject to residual error. There are, however, specific data files and linkages that can capture and introduce additional data on patient characteristics, including some of those mentioned above that can help improve the validity of studies conducted in the Medicare data. These include the Medical evidence form, which collects more detailed prognostic information on patients when they initiate dialysis, and the linkage of SEER, which provides rich cancer-specific data obtained at the time of diagnosis.
Second, Medicare data, like other forms of health care claims, are not collected for the purposes of research, but to support reimbursement for health care services. Unlike data captured in an electronic health record or as part of a prospective study, information collected as claims for reimbursement for services provided may be influenced by financial incentives and may be more susceptible to misclassification. This can affect estimates of incidence and prevalence, and can lead to biased estimates of association, and thus may require adjustment.Citation35 There have been validation studiesCitation36–Citation38 conducted with the Medicare data to evaluate algorithms for identifying clinical conditions with strong receiver operating characteristics (i.e., high positive predictive value and high specificity), and although many such algorithms have been identified, concerns about misclassification when identifying a disease cohort or condition of interest remain.
Third, ~30% of Medicare beneficiaries are enrolled in a Medicare Part C MA plan through a private insurer, and this percentage has increased steadily since 1999.Citation28 Data on beneficiaries covered by MA plans are not included in the SAFs provided to researchers by CMS. MA claims are available in some US commercial claims databases, but combining or linking these claims with CMS FFS files may not be feasible. It is, therefore, difficult to conduct studies on the entire Medicare population. This limitation should be given careful consideration when designing studies intended to evaluate trends over time in disease incidence, prevalence, and burden in the ≥65 years population. Fourth, Medicare does not cover all possible services (e.g., items and services furnished outside the US, examinations for hearing aids, and prescription of eye lenses). As such, research on those services would not be possible. When in doubt, researchers should consult CMS’ official list of non-covered items and services.Citation39 Finally, Medicare data for beneficiaries aged <65 years are not representative of the age-matched national population as the latter are typically enrolled in other insurance programs or are uninsured. Younger Medicare beneficiaries tend to have higher levels of disability and a greater burden of chronic illness than their non-Medicare age-matched peers.
Factors to consider when using Medicare data for research
In addition to the limitations of the Medicare data described above, it is important for researchers to be aware of several aspects before designing and implementing studies and interpreting results. Below, we describe some specific characteristics of the Medicare data and discuss the potential influence that CMS policy decisions can have on data collection. It should be noted that this is not meant to be an exhaustive list, but a commentary on the types of issues that the authors have experienced in their work.
Position and number of diagnosis code fields
When designing research studies and defining a disease cohort of interest in any claims-based database, high specificity is attained by limiting entry criteria based on International Classification of Diseases (ICD)-9/10 diagnosis code(s) to the primary diagnosis field. However, the diagnosis code in the primary position does not invariably represent the main reason that a patient was admitted from a clinical perspective; it may instead be a condition present with a relatively high reimbursement rate. As such, a research design might have to be altered by defining the condition of interest as being present when the diagnosis is present in, for example, any of the first five positions on the claim. This is especially pertinent when defining an outcome event of interest from which a rate will be calculated. This issue is inherent in the use of all claims-based research, such as that utilizing employer group health plans, and so is not unique to use of Medicare data.
In Medicare specifically, the issue is further complicated by the expansion of the number of ICD-9/10 diagnosis and procedure code fields on a claim from 9 and 6 to 25 and 25, respectively. This expansion took place in 2010, but did not noticeably affect the resulting data in the SAFs until January 2011. This expansion in the number of available fields could conceivably result in an artificial increase in estimates of disease burden, particularly if more sensitive definitions of disease are used (e.g., the presence of a single ICD-9 code in any position).
As an example of this phenomenon, displays the average number of diagnosis codes per inpatient claim per calendar year from 1995 to 2013. In 2011, the number of codes increased substantially in response to the change in number of available code positions on hospital claims. displays the percent of inpatient claims with an diagnosis code for coronary atherosclerosis from 2005 to 2012, using all available diagnosis fields compared with limiting to the first 9 fields. Because of the increase in diagnosis fields in 2011, the percentage appears to increase in 2011 and 2012, while limiting to the first 9 fields show a continuation of the decreasing trend observed prior to 2011. Researchers should consider how to address these sources of error in the design (e.g., limit to primary position) or analysis stage (e.g., consider performing sensitivity analyses where the robustness of the results is assessed when varying the number of fields or position within the fields used to define the case definition or comorbidity) ().
Figure 2 Impact of the expansion of Medicare diagnosis fields in 2010.
Abbreviation: IP, inpatient.
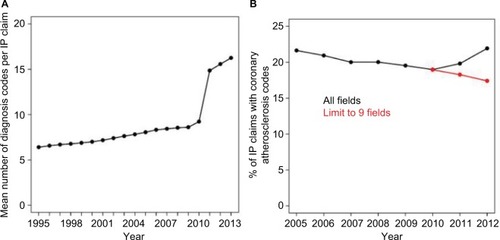
With the introduction of the ICD-10 coding system in October 2015, researchers also face the challenge of an expanded number of ICD diagnosis codes (from 17,000 in ICD-9 to 77,000 in ICD-10). While the CMS offers a crosswalk between the two systems, efforts to validate this complex mapping in both Medicare and commercial claims databases will be necessary.
Completeness of inpatient claims at the end of each calendar year
Next, a small but non-trivial percentage of claims for inpatient services (~2%) at the end of each calendar year are not included in the SAF provided to researchers. This occurs because for each calendar year SAF, CMS extracts inpatient claims based on the discharge date (“through date”), and a small percentage of patients are admitted into the hospital in one calendar year but not discharged until the following. For example, if a patient is admitted into the hospital on December 15, 2010 and discharged on January 20, 2011, their inpatient claim will not appear in the 2010 SAF, resulting in an underestimation of event rates if the study period extends only to the end of 2010. This can be particularly problematic when studying major clinical events that result in long hospitalizations (e.g., stroke or serious infections). These claims can be properly allocated to the appropriate calendar year only when the subsequent year SAF becomes available and the inpatient claims bridging two calendar years are identified. Based on the authors’ experience, the claims for services crossing calendar years together with those that are reprocessed or processed late (which contribute only a small percent) can affect ~20% of December hospital admissions, resulting in spuriously low event rates at the end of a calendar year.
The influence of CMS policy on data collection and coding
Reimbursement policies implemented by CMS may affect the way in which the provision of health care is coded by hospitals and health care providers. It is important for researchers working with Medicare data to stay abreast of CMS policy decisions and consider how these decisions may impact trends in disease and treatment utilization patterns observed in the data. We provide several examples of changes in coding practices and health care resource utilization that followed relevant policy changes.
One example concerns Diagnosis-Related Groups (DRGs), which were implemented by CMS in 1985 as part of the prospective payment system (PPS) for hospitalizations. Conceived as a mechanism to identify the “product” produced by a hospital in a quantifiable way (thereby exerting constraints on how care was provided and reimbursed), DRG-specific diagnoses and procedures into homogeneous units of hospital activity to which binding prices can be attached. The DRG determines how much the hospital is reimbursed by CMS for the inpatient stay. Patients classified according to a specific DRG are expected to use the same amount of hospital resources regardless of the intensity of the actual service provided.
The DRG system helps manage the costs of hospitalizations but can also influence the coding of disease within hospitalizations. Because the DRG is assigned based on the principal diagnosis and procedure codes, hospitals and/or providers may select principal diagnoses that correspond to DRGs with larger reimbursement. Consequently, diagnostic codes captured in the primary discharge position within a hospital record may, for example, not accurately reflect the reason the patient was admitted. For example, acute myocardial infarction (AMI) may be selected as the principal diagnosis as opposed to another disease condition because the DRG for AMI would likely have a substantially higher reimbursement payment. Researchers may want to consider if a disease or comorbidity of interest is a part of an existing DRG and the impact this may have on the coding of that condition when designing a study, especially those focused on the inpatient setting. As in other databases, using disease definitions that have been validated (i.e., high receiver operating characteristics) in that specific database may provide the most accurate results.
The DRG system was further revised on October 1, 2007, when CMS implemented Medicare Severity-Diagnosis Related Groups (MS-DRG) as a revision to the already existing DRGs. Previously, DRGs had a two-tiered structure; with and without complication/comorbidity (CC). DRGs with a CC had a higher weight, and therefore higher reimbursement, than the same DRG without a CC. With MS-DRGs, a third tier of “major” CC (MCC) was added. This additional tier resulted in increased reimbursement claims associated with conditions categorized as MCCs. For example, Gohil et al found significant increases in hospital discharges with sepsis, leading to an artificial increase in sepsis-related hospitalization rates from 2000 to 2010 following the policy change.Citation40 This example illustrates the importance of considering the introduction of MS-DRGs when evaluating trends in disease rates over this time period.
Another important policy change that occurred was the 2003 Medicare Modernization Act (MMA).Citation41 The MMA reduced reimbursement for outpatient drugs administered in physicians’ offices covered under Medicare Part B. These changes were prompted by the increasing expenditures for these drugs and reports that Medicare payments for the drugs were much higher than physicians’ costs for purchasing the drugs. Using the SEER-Medicare linked database, several studies have examined the impact of the MMA on the use of androgen deprivation therapy (ADT) (the standard of care for locally advanced or metastatic prostate cancer), including gonadotropin-releasing hormone (GnRH) agonists, on males with prostate cancer. Shahinian et al concluded that changes in Medicare reimbursement policy implemented through the MMA were associated with significant reductions in the use of ADT, particularly among males for whom the benefits of such therapy were unclear.Citation21 Elliott et al found that for the appropriate group of patients with metastatic disease, use of GnRH agonists did not change after MMA implementation but for males with very low-risk cancers, among whom there was a large decrease.Citation42 Additional studies were also able to evaluate cost implications, showing that payments to physicians for GnRH agonists decreased substantially between 2003 and 2005.Citation43,Citation44 The timing and influence of these types of policy changes on physician practices are important to consider when evaluating medication use patterns and conducting comparative effectiveness/safety research.
The introduction of the Affordable Care Act (ACA) in April 2010 provides another example of how policy changes affect the conductance of research. As part of the ACA, the Hospital Readmissions Reduction Program was implemented by CMS. The program aims at reducing costs associated with hospital readmissions within 30 days after discharge by penalizing hospitals with higher than expected 30-day readmission rates for a number of selected conditions. As of fiscal year 2015, the Hospital Readmissions Reduction Program targets AMI, heart failure, pneumonia, acute exacerbation of COPD, total hip arthroplasty, total knee arthroplasty, and CABG. The maximum penalty as of fiscal year 2015 is 3% of a hospital’s Medicare DRG payments.
Although hospital readmission rates have decreased since the implementation of the program, there are concerns that hospitals may be achieving this via the increased use of observation unit stays.Citation45 In their 2016 publication, Zuckerman et al used Medicare Parts A and B claims data to evaluate trends in readmission rates from October 2007 through May 2015.Citation19 They found the risk-adjusted rates of readmissions for the initially targeted conditions (AMI, heart failure, and pneumonia) decreased between 2007 and 2015 while stays in observation units increased. It is important for researchers to be aware of this significant policy change when exploring disease trends and rates of hospital readmission. It may be helpful to also consider the condition as seen in the observation unit, typically coded as outpatient, when evaluating disease trends for the targeted conditions of the Hospital Readmissions Reduction Program.
On January 1, 2011, CMS implemented the Medicare Improvement for Patients and Providers Act, an expanded capitation payment policy for separately billable dialysis services to the ESRD PPS, often referred to as “the bundle”.Citation46 Separately billable dialysis services within Part B were included in the capitated payment rate, changing the incentives for use of injectable medicines such that providers receive greater remuneration when less medicine is administered. Subsequent to PPS implementation, use patterns for the medicines covered in the capitated rate changed significantly.Citation47–Citation50 Awareness of these reimbursement changes and their possible influence on provider practices are critical when conducting studies to address a specific clinical question. In fact, designing and conducting studies that take advantage of such policy changes can often provide compelling information regarding the effectiveness and/or safety of therapeutic interventions.Citation22,Citation23
Finally, the recent increase in the rates of fluid overload provides an example of the impact multiple CMS policy changes can have on disease trends. Fluid overload (including pleural effusion) is an adverse event often associated with ESRD patients that may be indistinguishable from heart failure. The hospitalization rate for fluid overload in 2011 was 2.5 times higher than the rate in 2004. This increase may be confounded by two main factors.Citation51 First, fluid overload was included in the MS-DRG “miscellaneous disorders of nutrition, metabolism, fluid, and electrolytes” with a higher relative weight than other MS-DRGs; and second, practices may have been undercoded for heart failure in order to avoid penalties under the Hospital Readmissions Reduction Program. The increase in the rates of fluid overload and accompanying decrease in the rates of hospitalization for heart failure emphasize the impact of multiple policy decisions on the apparent rates of disease in the Medicare population, as well as the interdependencies between different policy changes.
The above examples illustrate the need for researchers to be aware of the impacts of CMS reimbursement policy on changes in medical coding, especially when evaluating trends in disease occurrence and hospitalizations. Furthermore, they illustrate not only the impact of policy on the Medicare data itself, but the utility of Medicare research files to empirically assess both the expected and unexpected impacts of CMS reimbursement policy. In addition to reimbursement policy, other ecologic factors such as updated clinical practice guidelines may also be considered when assessing trends in disease, medication use, and medical procedures.
Conclusion and future directions
Medicare claims data represent population-based data for the Medicare-covered US population that provide researchers with a unique and far-reaching ability to conduct epidemiologic and health outcomes research. These data have been shown to improve the understanding of disease burden and trends, as well as the uptake of therapeutics, real-world implications of policy changes, and the overall health of the US population covered by the Medicare program. It is important for those using the data for research to consider its limitations as well as the potential impact CMS policy changes may have on data collection and coding, and ultimately on findings.
In this review, we provided a resource guide for researchers on using Medicare reimbursement claims data for epidemiologic and health services research. We presented pragmatic considerations and highlighted select studies to showcase how the data were particularly informative for CMS reimbursement policy evaluations in the US. The ResDACCitation24 provides additional support services to educate investigators on the proper application of Medicare data for research purposes through a series of seminars and in-person conferences.
Looking to the near future, Medicare data will be useful for the surveillance and effectiveness studies of new therapeutics, such as biosimilars, as they enter the US market. With CMS’ focus on higher quality and coordination of care, the Medicare data will also allow researchers to evaluate the impact of capitated payment systems such as the recently introduced Oncology Care Model.Citation52 Medicare data are particularly relevant in characterizing the treatments and patterns of care, dosing, adverse events, and other patient outcomes when clinical trials do not adequately capture the older population.53 Furthermore, with a growing focus on improved patient experience through medical devices and new technologies, Medicare data will be a valuable resource for the surveillance of these new tools. The linking of Medicare data to ongoing cohort studies such as the Nurses’ Health Study, population-based registry data from SEER, and detailed clinical databases such as DaVita Inc. (a large dialysis organization) further build on its value and potential. Finally, improvements in timing of data availability through the availability of quarterly SAF and the development of CMS’ Qualified Entity Program, allowing public, academic, and private entities access to the Medicare claims data, also highlight the increasing value of the Medicare claims data for epidemiologic and health services research.
As the population ages and average life expectancy increases,Citation33 the projected growth of the older population across the world will present challenges to policy makers and public programs. Considering that the major chronic conditions including cardiovascular disease, heart failure, diabetes, cancer, and Alzheimer’s disproportionately affect the Medicare population, identifying the most effective and safe interventions that provide the most value to Medicare beneficiaries and society will continue to be a first-order priority. Medicare data have been and will continue to be critical for enabling such investigations and providing much-needed real-world evidence to guide decision-makers.
Disclosure
KEM, AL, RZ, and BDB are Amgen Inc. employees, and are stock owners in Amgen Inc. The authors report no other conflicts of interest in this work.
References
- Centers for Medicare & Medicaid Services on its 50th anniversary, more than 55 million Americans covered by Medicare2015 Available from: https://www.cms.gov/Newsroom/MediaReleaseDatabase/Press-releases/2015-Press-releases-items/2015-07-28.htmlAccessed October 15, 2016
- JencksSFWilliamsMVColemanEARehospitalizations among patients in the Medicare fee-for-service programN Engl J Med2009360141418142819339721
- GornickMEEggersPWReillyTWEffects of race and income on mortality and use of services among Medicare beneficiariesN Engl J Med1996335117917998703185
- SchermerhornMLBuckDBO’MalleyAJLong-term outcomes of abdominal aortic aneurysm in the medicarepopulationN Engl J Med2015373432833826200979
- WetmoreJBPengYJacksonSMatlonTJCollinsAJGilbertsonDTPatient characteristics, disease burden, and medication use in stage 4–5 chronic kidney disease patientsClin Nephrol201685210111126636331
- SchneeweissSSolomonDHWangPSRassenJBrookhartMASimultaneous assessment of short-term gastrointestinal benefits and cardiovascular risks of selective cyclooxygenase 2 inhibitors and non-selective nonsteroidal antiinflammatory drugs: an instrumental variable analysisArthritis Rheum200654113390339817075817
- WangPSSchneeweissSAvornJRisk of death in elderly users of conventional vs. atypical antipsychotic medicationsN Engl J Med2005353222335234116319382
- IzurietaHSThadaniNShayDKComparative effectiveness of high-dose versus standard-dose influenza vaccines in US residents aged 65 years and older from 2012 to 2013 using Medicare data: a retrospective cohort analysisLancet Infect Dis201515329330025672568
- YunHDelzellESaagKGFractures and mortality in relation to different osteoporosis treatmentsClin Exp Rheumatol201533330230925068266
- GildenDMKubisiakJMPohlGMTreatment patterns and cost-effectiveness of first line treatment of advanced non-squamous non-small cell lung cancer in Medicare patientsJ Med Econ201720215116127574722
- GozaloPPlotzkeMMorVMillerSCTenoJMChanges in Medicare costs with the growth of hospice care in nursing homesN Engl J Med2015372191823183125946281
- PadegimasEMVermaKZmistowskiBRothmanRHPurtillJJHowleyMMedicare reimbursement for total joint arthroplasty: the driving forcesJ Bone Joint Surg Am201698121007101327307361
- LairsonDRParikhRCCormierJNChanWDuXLCost-utility analysis of chemotherapy regimens in elderly patients with stage III colon cancerPharmacoeconomics201432101005101324920195
- BoeroIJGillespieEFHouJParavatiAJKimEEinckJPThe impact of radiation oncologists on the early adoption of hypofractionated radiation therapy for early-stage breast cancerInt J Radiat Oncol Biol Phys201797357158028126306
- O’MalleyASReschovskyJDSaiontz-MartinezCInterspecialty communication supported by health information technology associated with lower hospitalization rates for ambulatory care-sensitive conditionsJ Am Board Fam Med201528340441725957373
- KoCWDominitzJAGreenPKreuterWBaldwinLMSpecialty differences in polyp detection, removal, and biopsy during colonoscopyAm J Med2010123652853520569759
- HirthRATurenneMNWheelerJRPanQMaYMessanaJMProvider monitoring and pay-for-performance when multiple providers affect outcomes: an application to renal dialysisHealth Serv Res2009445 Pt 11585160219555398
- ArnesonTJLiSGilbertsonDTBridgesKRAcquavellaJFCollinsAJImpact of centers for medicare and medicaid services national coverage determination on erythropoiesis-stimulating agent and transfusion use in chemotherapy-treated cancer patientsPharmacoepidemiol Drug Saf201221885786422450901
- ZuckermanRBSheingoldSHOravEJRuhterJEpsteinAMReadmissions, observation, and the hospital readmissions reduction programN Engl J Med2016374161543155126910198
- BarrCDDiezDMWangYDominiciFSametJMComprehensive smoking bans and acute myocardial infarction among Medicare enrollees in 387 US counties: 1999–2008Am J Epidemiol2012176764264822986145
- ShahinianVBKuoYFGilbertSMReimbursement policy and androgen-deprivation therapy for prostate cancerN Engl J Med2010363191822183221047226
- ChertowGMLiuJMondaKLEpoetinalfa and outcomes in dialysis amid regulatory and payment reformJ Am Soc Nephrol201627103129313826917691
- WangCKaneRLevensonMAssociation between changes in CMS reimbursement policy and drug labels for erythrocyte-stimulating agents with outcomes for older patients undergoing hemodialysis covered by Fee-for-service medicareJAMA Intern Med2016176121818182527775769
- ResDACResearch Data Assistance Center Available from: http://www.resdac.org/Accessed December 15, 2016
- 2015 CMS StatisticsServices US Department of Health and Human Services2015
- U.S. Census BureauCurrent Population Survey, 2012 Annual Social and Economic (ASEC) Supplement 2012 Available from: https://www.census.gov/prod/techdoc/cps/cpsmar12.pdfAccessed July 26, 2016
- U.S. Census Bureau, Population DivisionAnnual Estimates of the Resident Population by Sex, Age, Race, and Hispanic Origin for the United States and States: April 1, 2010 to July 1, 2015 Available from: https://factfinder.census.gov/faces/tableservices/jsf/pages/productview.xhtml?src=bkmkAccessed November 21, 2016
- The HenryJKaiser Family FoundationMedicare Advantage Available from: http://kff.org/medicare/fact-sheet/medicare-advantage/Accessed March 22, 2017
- GrossDJAlecxihLGibsonMJCoreaJCaplanCBranganNOut-of-pocket health spending by poor and near-poor elderly Medicare beneficiariesHealth Serv Res1999341 Pt 22415410199672
- United States Renal Data System2016 USRDS Annual Data Report: Epidemiology of Kidney Disease in the United StatesBethesda, MDNational Institutes of Health, National Institute of Diabetes and Digestive and Kidney Diseases2016
- WarrenJLKlabundeCNSchragDBachPBRileyGFOverview of the SEER-Medicare data: content, research applications, and generalizability to the United States elderly populationMed Care2002408 SupplIV-318
- DaneseMDCangialoseCOverview of All SEER-Medicare Publications: Outcomes Insights, Inc2016 Available from: http://www.outins.com/wp-content/uploads/2016/03/seer_medicare_report_2016-01-18.pdfAccessed October 25, 2016
- WanHEGoodkindDKowalPAn Aging World: 2015International Population ReportsBureau USCWashington D.CU.S. Government Publishing Office2016
- McBeanMIntroduction to the Use of Medicare Data for Research: Research Data Assistance Center (ResDAC)2012 Available from: http://www.resdac.org/sites/resdac.umn.edu/files/Overview%20of%20the%20Medicare%20Program%20%28Slides%29.pdfAccessed July 20, 2016
- StrolloSEAdjemianJAdjemianMKPrevotsDRThe burden of pulmonary nontuberculous mycobacterial disease in the United StatesAnn Am Thorac Soc201512101458146426214350
- HennessySLeonardCEFreemanCPValidation of diagnostic codes for outpatient-originating sudden cardiac death and ventricular arrhythmia in Medicaid and Medicare claims dataPharmacoepidemiol Drug Saf201019655556219844945
- ThackerELMuntnerPZhaoHClaims-based algorithms for identifying Medicare beneficiaries at high estimated risk for coronary heart disease events: a cross-sectional studyBMC Health Serv Res20141419524779477
- KentSTSaffordMMZhaoHOptimal use of available claims to identify a Medicare population free of coronary heart diseaseAm J Epidemiol2015182980881926443420
- Centers for Medicare & Medicaid ServicesItems and Services That Are Not Covered Under the Medicare Program2015 Available from: https://www.cms.gov/Outreach-and-Education/Medicare-Learning-Network-MLN/MLNProducts/Downloads/Items-and-Services-Not-Covered-Under-Medicare-Booklet-ICN906765.pdfAccessed October 27, 2016
- GohilSKCaoCPhelanMImpact of policies on the rise in sepsis incidence, 2000–2010Clin Infect Dis201662669570326787173
- Medicare Prescription Drug Improvement and Modernization Act of 2003 Available from: https://www.congress.gov/bill/108th-congress/house-bill/1
- ElliottSPJarosekSLWiltTJVirnigBAReduction in physician reimbursement and use of hormone therapy in prostate cancerJ Natl Cancer Inst2010102241826183421131577
- WeightCJKleinEAJonesJSAndrogen deprivation falls as orchiectomy rates rise after changes in reimbursement in the U.S. Medicare populationCancer2008112102195220118393326
- WeaverCMathewsAWMcgintyTMedicare Rules Reshape Hospital Admissions: Return-visit rate drops, but change in billing tactics skews numbers: The Wall Street Journal2015 Available from: http://www.wsj.com/articles/medicare-rules-reshape-hospital-admissions-1449024342Accessed October 26, 2016
- Centers for Medicare & Medicaid ServicesMedicare program: end-stage renal disease prospective payment system, final rule, 42 CFR Parts 410, 413, 414Services DoHaHSCfMM Federal Register2010
- MondaKLJosephPNNeumannPJBradburyBDRubinRJComparative changes in treatment practices and clinical outcomes following implementation of a prospective payment system: the STEPPS studyBMC Nephrol2015166725928734
- BrunelliSMMondaKLBurkartJMEarly trends from the study to evaluate the prospective payment system impact on small dialysis organizations (STEPPS)Am J Kidney Dis201361694795623332991
- U.S. Renal Data SystemUSRDS 2012 Annual Data Report: Atlas of Chronic Kidney Disease and End-Stage Renal Disease in the United StatesBethesda, MDNational Institutes of Health, National Institute of Diabetes and Digestive and Kidney Diseases2012
- DOPPS Dialysis Practice Monitor Dialysis Outcomes and Practice Patterns Study Program Available from: http://www.dopps.org/dpm/default.aspxAccessed December 16, 2016
- Peer Kidney Care InitiativePeer Report: Dialysis Care and Outcomes in the United StatesMinneapolis, MNChronic Disease Research Group2014
- Oncology Care Model 2017 Available from: https://innovation.cms.gov/initiatives/oncology-care/Accessed January 10, 2017
- MurthyVHKrumholzHMGrossCPParticipation in cancer clinical trials: race-, sex-, and age-based disparitiesJAMA2004291222720272615187053