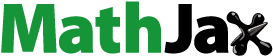
Abstract
Background
The value of integrated care through comprehensive, coordinated, and family-centered services has been increasingly recognized for improving health outcomes of children with special health care needs (CSHCN). In a randomized controlled trial (RCT), the integrated care provided through the Children’s Treatment Network (CTN) was compared with usual care in improving the psychosocial health of target CSHCN. In this paper, we aimed to estimate the effect of CTN care by conducting multiple analyses to handle noncompliance in the trial.
Methods
The trial recruited target children in Simcoe County and York Region, ON, Canada. Children were randomized to receive CTN or usual care and were followed for 2 years. The CTN group received integrated services through multiple providers to address their specific needs while the usual care group continued to receive care directed by their parents. The outcome was change in psychosocial quality of life at 2 years. We conducted intention-to-treat, as-treated, per-protocol, and instrumental variable analyses to analyze the outcome.
Results
The trial randomized 445 children, with 229 in the intervention group and 216 in the control group. During follow-up, 52% of children in the intervention group did not receive complete CTN care for various reasons. At 2 years, we did not find a significant improvement in psychosocial quality of life among the children receiving CTN care compared with usual care (intention-to-treat mean difference 1.50, 95% confidence interval −1.49 to 4.50; P = 0.32). Other methods of analysis yielded similar results.
Conclusion
Although the effect of CTN care was not significant, there was evidence showing benefits of integrated care for CSHCN. More RCTs are needed to demonstrate the magnitude of such an effect. The CTN study highlights the key challenges in RCTs when assessing interventions involving integrated care, and informs further RCTs including similar evaluations.
Introduction
Children with special health care needs (CSHCN) are those “who have or are at increased risk for a chronic physical, developmental, behavioral, or emotional condition, and who also require health and related services of a type or amount beyond that required by children generally”.Citation1 In Canada, about 13%–18% of children are considered to have special needs.Citation2 These children usually have poor quality of lifeCitation3 associated with emotional symptoms,Citation4 psychologic problems,Citation5 and health risk behaviors.Citation6 Two thirds of parents with a disabled child do not get the necessary treatments for their child and more than one third do not know where to look for help.Citation7 These parents endure enormous financial and mental burdens due to their child’s illnesses.Citation8 Since most CSHCN live at home, parents have to quit their jobs, work fewer hours, turn down promotion, or compromise job performanceCitation9 to take care of their child with special needs. “Finding services, battling waitlists, travelling long distances, briefing numerous professionals from different sectors on their child’s history, and coordinating services from multiple agencies” are just part of their daily life.Citation10 These parents commonly describe continuing strain and chronic fatigue concerning their child’s well-being,Citation11 and over 35% of them report high levels of emotional distress.Citation12 Their families are also affected by high divorce rates.Citation13
CSHCN are usually diverse in nature and need an individualized approach to address their specialized and multiple needs.Citation14 The value of continuous, comprehensive, coordinated, and family-centered careCitation15 has been widely recognized. Delivery of this new type of care requires a more integrative approach than the traditional one through: collaboration with parents to decide on case-specific goals; coordination of an individual team of service providers; and provision of a single point of access to address families’ multiple needs. As an example, the medical homeCitation16 in the US is a new development in health care delivery for families with CSHCN. It has led to reductions in wait-time and unmet health care needs for target children,Citation17 increased use of preventive care,Citation18 decreased risk of comorbidities,Citation19 and alleviation of family burden.Citation20 Adoption of these strategies in the Canadian system has been limited. There are examples of innovative interventions (for example, the chronic care model in British Columbia and the Family Health Network in Ontario), but these interventions target adult patients.Citation14 Compared with adults, CSHCN are affected by a larger number of uncommon conditions and demand more tailored services and a more complex model of care.
In 2006, a new model of care for CSHCN and their families, known as the Children’s Treatment Network (CTN), was launched in Simcoe County and York Region in Ontario, Canada. The CTN model of care is funded by the government and is based on collaboration of local service providers from different agencies and organizations. It provides a single point of access and service navigation for children. The CTN model is unique, in that it assigns a service coordinator and an individual team of health service providers for each target family, develops a single plan of care, and uses an electronic record system to share clinical information and assessment of the child. Some services available to families through the CTN include developmentally appropriate therapy, speech therapy, augmentative communication, in-home social support, behavior therapy, and early childhood education (www.ctn-simcoeyork.ca). Before the launch of the CTN, parents in both communities had limited access to the specialized treatments needed for their children and had to travel outside of the region to access those services. Funded by the Ministry of Children and Youth Services and the Ministry of Health and Long-term Care In Ontario, Canada, Thurston et alCitation21 conducted a randomized controlled trial to compare the integrated interventional program of care organized through the CTN versus usual care for managing children with multiple and complex health needs in Simcoe County and York Region (trial registration in clinicaltrials.gov NCT01379443). This CTN trial was subject to substantial noncompliance, where about 52% of children in the intervention group only received parts of CTN integrated care. Some analyses of the CTN were published previously.Citation22,Citation23
The primary goal of the CTN trial was to assess the effect of CTN integrated care on improving the psychosocial function of target children in Simcoe County and York Region. In this study, we compared the intention-to-treat (ITT) method with alternative methods of analyzing the data. We also aimed to assess the sensitivity of ITT analysis for participants’ noncompliance with the CTN intervention.
Materials and methods
We describe the CTN trial here by adopting the Consolidated Standards of Reporting Trials guideline.Citation24 Using a parallel design, each eligible child was randomized to an intervention group or a control group with a 1:1 allocation ratio.
Participants
Participants were recruited from the Simcoe County and York Region in Ontario, Canada. The Simcoe York District Health CouncilCitation25 projected the total number of children in both regions to be 390,498 in 2007. According to a national survey, 1% of children were estimated to have severe disabilities and receive rehabilitation services.Citation26
Children aged 0–19 years were recruited if they had any of the following conditions: cerebral palsy, brain injury, developmental difficulties, Down syndrome, spina bifida, autism, physical disability, developmental disability, pervasive developmental disorder, or a chronic medical condition. Children who were receiving palliative care, requiring emergency services, or living outside of the region at the time were excluded. Non-English speaking families without an English translator were also excluded.
Potential children were identified through the Community Care Access Centres, school boards, and Early Intervention Services agencies. Eligible families were contacted by mail with an invitation package, which contained a study information letter, and a parental consent and participant contact information form. A second mail-out was done to families who did not respond to the initial mail-out. Only children whose parents had provided written informed consent could participate. Children were enrolled from May to December 2007. A unique patient ID was created for each enrolled child.
Ethics
There were no known harms or safety risks to the children and their families involved in the trial. The research ethics board at Hamilton Health Sciences/McMaster Health Sciences approved the study.
Intervention
Each child in the CTN group was assigned a service navigator who conducted a comprehensive assessment to identify the child’s health conditions. A trained service coordinator then followed up with the family. In working with the parents, an individual team of service providers was formed according to the child’s health and social needs. For example, a team comprising an augmentative communication services consultant, early interventionist, occupational therapist, physiotherapist, speech and language therapist, and service coordinator was assembled for a child with cerebral palsy. This integrative team together with family members developed a single plan of care for the child. The service coordinator organized the delivery of services according to the plan. The team met with the family for ongoing assessment and revision of the plan on a regular basis. All assessments and clinical notes concerning the child were documented in an electronic record system shared by all team members. shows the detailed components of CTN integrated care. Families in the control group continued to manage services for their child in a self-directed manner. This group did not have access to the CTN integrated care; however, they had access to all other service providers (including CTN partners) as requested.
Figure 1 Components of the Children’s Treatment Network versus usual care.
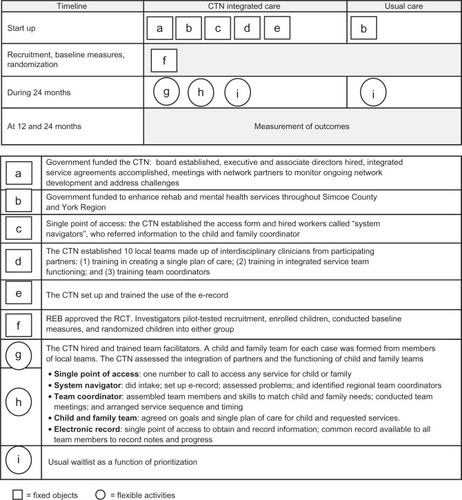
Fidelity to integrated child and family team care was evaluated by: content in the child and family team of providers (extent, scope, and agreement in collaboration); quality of team functioning, measured by the Partnership Functioning Scale;Citation27 and the frequency and duration coverage of the prescribed services as outlined in the single plan of care compared with providers’ entries on electronic record.
Outcome and variables
The outcome was the change in the child’s psychosocial quality of life (QoL) in 24 months. This was reported by parents using the short form Pediatric Quality of Life (SF PedsQL).Citation28 Psychosocial QoL was the sum of the emotional, social, and school functions and was extrapolated to have a score from 0 (the worst QoL) to 100 (the best QoL). Information on each child’s age, sex, and admission diagnosis was collected. The age, marital status, educational level, and family income of the parent who was the most knowledgeable (PMK) about the child were recorded.
Parental distress score
The Kessler scale (K10)Citation29 was used to measure PMK symptoms of depression and anxiety. Ten questions measured these feelings: sad, nervous, restless, hopeless, worthless, everything was an effort, tired for no good reason, so nervous that nothing could calm down, so restless and could not sit still, and depression during the past month. The PMK rated each item on a five-point scale (1, “all of the time” to 5, “none of the time”). Scores range from 10 (no symptom of distress) to 50 (severe distress).
Positive parenting score
The National Longitudinal Survey of Children and Youth parenting scale was used, and five questions adapted from the Parent Practices ScaleCitation30 were used to measure positive parenting behaviors (eg, praise, play). The PMK rated each item (eg, “Do something special with your child that he/she enjoys”) on a five-point scale (0, “never” to 4, “many times each day”). Higher scores indicate more positive parenting behaviors.
Social support score
An eight-item shortened version of the Social Provisions ScaleCitation31 was used to measure the level of social support that the PMK received in guidance, reliable alliance, and attachment. PMK rated each item on a four-point scale (0, “strongly disagree” to 3, “strongly agree”). Higher scores represent a greater level of social support.
Family function score
Thirteen items taken from the National Longitudinal Survey of Children and Youth population survey, based on a subscale of the McMaster Assessment Device,Citation32 were used to measure size was calculated to be 240 for a two-sided various aspects of family functioning in problem-solving, communication, affective responsiveness, affective involvement, and behavior control. The PMK rated each item on a four-point scale (0, “strongly agree” to 3, “strongly disagree”). Scores of negatively oriented items (eg, “We avoid discussing our fears or concerns”) were reversed so that higher scores represent greater family dysfunction.
Sample size
Using a minimum clinically important difference of ten on the target children’s psychosocial QoLCitation33 and an estimated standard deviation of 28 estimated from our pilot sample, the sample size was calculated to be 240 for a two-sided test with 5% alpha and 80% statistical power. To allow for ten independent variables and an attrition rate of 20%, the required sample size was 425.
Randomization
Using a parallel design, eligible children with parental consent were randomized to the intervention and control groups with a 1:1 allocation ratio. Children were stratified by region (Simcoe/York), Community Care Access Centre, and age (pre-school/school). They were randomized within stratum by using a block size of six. The randomization list was generated by the Health and Social Service Utilization Research Unit (HSSURU) at McMaster University (fhs.mcmaster.ca/slru). The allocation codes were then sequentially linked to the patient IDs for enrolled children.
Blinding
Trained interviewers at the HSSURU, who were blinded to group allocation, collected data using a standardized questionnaire booklet containing questions on demographics and outcome measures. Data collection was performed by telephone at baseline, and at 12 and 24 months. After enrollment, children remained anonymous and were identified by their patient IDs. Only the statistician responsible for randomization had access to the allocation codes. The data analyst was blinded to group allocation; however, the participants were aware of their allocation. All data were stored and maintained at the HSSURU.
Statistical methods
We adopted the ITT principle by analyzing all patients according to how they were randomized, regardless of whether they complied with the assigned treatment or not. The ITT estimate was obtained by fitting a linear regression model with adjustment for baseline variables. The multiple imputation technique was used to impute the missing data by assuming that they were missing at random. Five imputation datasets were produced and combined to produce an estimate of 98.5% relative efficiency, given a fraction of about 30% missing data.Citation34
In the sensitivity analysis of the impact of noncompliance, we employed three other analytical approaches, ie, as-treated, per-protocol, and instrumental variable analyses. In the as-treated analysis, we compared the treated children (those who received complete CTN integrated care) with the untreated ones (those who received parts or none of the CTN integrated care) to estimate the effect of the intervention. In the per-protocol analysis, we analyzed the children by their randomization, but only ones who complied with the assigned treatment. Children in the control group did not have access to CTN integrated care and were deemed to comply with usual care. Both as-treated and per-protocol analyses compromised original randomization and were prone to selection bias and confounding. We employed the propensity score technique to adjust for those factors. Application of the propensity score in the as-treated and per-protocol analyses could potentially be a novel approach. Because we could not find any study comparing different uses of the propensity score in this context, we included all four commonly used propensity score methods, ie, matching, stratification, weighting, and covariate adjustment. The details of these propensity score methods are given in the Supplementary materials section.
The instrumental variable analysisCitation35 estimated the effect of CTN integrated care through an instrumental variable. The randomization allocation (Z) was associated with the exposure of CTN integrated care (X) but independent of confounders and any factor that explained the outcome (Y). Thus, Z satisfied the conditions to be an instrumental variable, and the treatment effect can be estimated by
where β(Z-Y) is the association between randomization and the outcome and β(Z-X) is the association between randomization and the receipt of CTN integrated care. We assumed that the Z-Y association was independent of the Z-X association. Under the assumption, we used the delta method to derive the variance of βIV for calculating the 95% confidence interval (CI) and P-value. The details are provided in the Supplementary materials section.
We compared the ITT method with other methods of analysis to assess the sensitivity of the results. summarizes the different approaches to analysis. The results are expressed as the mean difference, 95% CI of the mean difference, and corresponding P-value. The level of significance was set at 5%. All analyses were performed using statistical software R version 2.12.1 and Statistical Package for the Social Sciences version 19 software (IBM Corporation, Armonk, NY, USA).
Results
Recruitment, baseline data, and participant flow
Between May and December 2007, 2,319 eligible children were identified and their families were contacted by mail. Parents of 465 eligible children returned signed consent forms to indicate their willingness to participate. Later, 20 consented parents did not complete the baseline interview and were excluded from the study. The remaining 445 children were randomized to either the intervention group (n = 229) or the control group (n = 216).
The children were followed until December 2009. During the 2-year follow-up, 53 children in the CTN group did not have a team of service providers assembled or did not have the services available for their specific needs. Another 58 children in the CTN group withdrew from CTN integrated care. Common reasons for withdrawal were: death of the child, movement of the family outside of the region, a parent’s decision to manage the child without assistance, and transfer to another health care center. Overall, 64 (28%) children in the intervention group and 57 (26%) children in the control group were lost to follow-up. shows the flow of participants in the study. All 445 children were analyzed using a multiple imputation technique.
Statistical analysis
The children’s baseline characteristics are summarized in . Categorical and continuous variables are expressed as the frequency (percentage) or mean (standard deviation), respectively. Within the CTN arm, baseline variables were compared between the children who received complete CTN integrated care (treated) and those who received partial or none of the CTN integrated care (untreated). The results of this comparison are reported in . From the comparison, we did not find any significant differences between treated and untreated children except for parenting style (mean difference 1.4; P = 0.02); however, a mean difference of 1.4 on a score ranging from 0 to 20 did not seem to be a clinically relevant association.
Table 1 Baseline characteristics
Table 2 Comparing treated with untreated children in the intervention group
We conducted different analyses to estimate the effect of CTN integrated care. The results did not show a significant improvement in the children’s psychosocial QoL in the CTN group compared with the usual care group (mean difference 1.50, 95% CI–1.49, 4.50; P = 0.32). The results for the estimated treatment effect are reported in . In general, the conclusion from the ITT analysis was consistent with that drawn from the as-treated, per-protocol, and instrumental variable analyses. We also noticed some systematic patterns in the direction, magnitude, and precision of the estimates. All estimates except for the as-treated estimates favored CTN integrated care. The per-protocol estimates were the closest to 0 which represented no difference between CTN integrated care and usual care. Both ITT and instrumental variable estimates showed a larger effect of CTN integrated care over usual care than the per-protocol estimates. The instrumental variable estimate had the largest 95% CI. For the as-treated and per-protocol analyses which compromised the original randomization, adjusting for confounders and imbalance by matching on the propensity score produced estimates with a wider 95% CI than did the other propensity score methods. shows a comparison of the estimates obtained from different analyses.
Figure 4 Comparing the estimates of treatment effect from different analyses.
Abbreviations: ITT, intention-to-treat; AT, as-treated; PP, per-protocol; IV, instrumental variable; PS, propensity score.
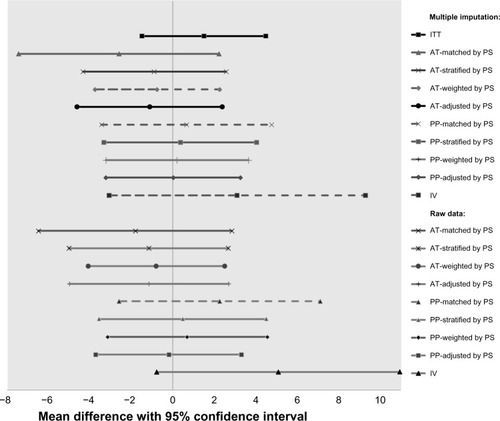
Table 3 Summary of estimates of treatment effect
Discussion
The ITT analysis did not show a significant improvement in psychosocial QoL among children receiving CTN integrated care than those receiving usual care in Simcoe County and York Region over 2 years. We conducted as-treated, per-protocol, and instrumental variable analyses to assess the sensitivity of this conclusion under substantial noncompliance with the CTN intervention. These alternative analyses also showed no significant difference between groups. Previously, two CTN studies have been published,Citation22,Citation23 which explored the interactions between multiple factors and system integration on the child’s psychosocial QoL and examined the associations between multiple factors and level of psychiatric distress experienced by parents.
Our findings were limited by a number of factors. First, the child’s psychosocial QoL was reported by their parents. Parents might have limited knowledge concerning their children’s health-related QoL. The parents’ responses reflected their own perception of children’s disease-related experiences, which might not be the same as how the children felt. For example, it was found that children with a congenital below-the-elbow deficiency reported better QoL than that perceived by their parents.Citation36 Second, the early development of the CTN model of care was associated with suboptimal intervention fidelity. Implementation of the CTN was a major undertaking in changing systems and operations management. It took longer than anticipated for the network hosts to hire and train appropriate staff at the local team level, get local team sites operational, and engage all the needed agency partner staff in the teams. The delay in creating the child and family teams was the key challenge in organizing services for some children. About 52% of the children assigned to the CTN group did not receive CTN integrated care as planned during the follow-up period. The limited success in truly engaging and retaining families in the process of care is often the reason for failure to achieve the desired health outcome.Citation37 Third, there was potential intervention contamination in the usual care group. Given that families within the same community might be randomized to a different group, families in the usual care group might learn about the intervention from those assigned to the CTN group. The CTN trial was limited by insufficient resources and funding to employ a large-scale multicenter cluster design, which could protect against intervention contamination. Consequently, contamination of control children may have reduced the estimate of intervention effect and could have led to a type II error in the results.Citation38 Fourth, our findings were limited by a relatively short period of observation after the initial launch of the intervention. For chronically ill children, the effect of integrated care on health outcomes would often accumulate over time. Thus, an interventional gain might be observed over a longer period of follow-up as practitioners became more proficient and team functioning became more collaborative. Fifth, our results were limited by missing data due to loss of patients to follow-up. We assumed that those data were missing at random and employed multiple imputation in the analysis. However, the assumption of data being missing at random is inherently untestable. Finally, the population targeted by the CTN may represent a subset of the defined CSHCN population accessing the services. Thus, our results may not be generalizable to a broader population of CSHCN, some of whom may not be accessing the services. The complex model of care and the heterogeneous population under study are the main challenges in assessing integrated health service interventions.Citation39
Our findings should be interpreted in light of previously published evidence on the CTN intervention.Citation22,Citation23 For the subgroup of children who received complete CTN integrated care, their QoL was influenced by complex interactions between team integration and parenting factors, and overall, their QoL improved after 2 years.Citation22 In a cross-sectional analysis of the CTN children,Citation23 we found that symptoms of psychiatric distress experienced by parents were associated with level of social support, family functioning, child behavior, and parenting style. All those factors could be mediated through coordinated and family-centered care. Also, the costs of total health and social services were higher in those parents with more symptoms of psychiatric distress. From an economic perspective, continuous and comprehensive services can save costs because giving people the services they need not only improves their QoL but also reduces use of other duplicative or otherwise fruitless services.Citation40 A recent systematic review showed that coordinated and family-centered care was associated with improved outcomes for CSHCN.Citation41 A study by King et alCitation42 showed that services for children with neurodevelopmental disorders were most beneficial when they were delivered in a family-centered fashion and addressed parent-identified issues. The current evidence is primarily from nonrandomized studies. More randomized controlled trials are needed to assess the effect of integrative care that is continuous, comprehensive, coordinated, and family-centered for CSHCN. The CTN intervention is the first of its kind in Canada. It aligns with the advocacy of comprehensive care for children’s mental health,Citation43 family-centered rather than child-centered interventions,Citation44 and partnerships with community psychology in the system of care.Citation45 Considering that 13%–18% of Canadian children had special health care needs, the CTN appears to be a milestone in the improvement of QoL for CSHCN through integrative care. At the same time, multiple community, family, parent, and child factors around the management of CSHCN make it challenging to evaluate this type of intervention. The ongoing implementation and evaluation of the CTN is an important step forward for Canadian community-based child care programs targeting this vulnerable population. The methodologic limitations in our study inform further trials when evaluating similar interventions.
Conclusion
In this trial, we assessed the effect of CTN integrated care versus usual care on psychosocial QoL in CSHCN from Simcoe County and York Region over 2 years. We did not find a significant improvement in QoL for children in the CTN group compared with those in the usual care group. The value of continuous, comprehensive, coordinated, and family-centered care for CSHCN is increasingly being recognized. At the same time, more research is needed to demonstrate the magnitude of the effect of integrated care on those children and their parents. Given that noncompliance is a common issue in randomized controlled trials, especially pragmatic trials, a better understanding of the performance of different analytical approaches is also crucial. Future simulation studies are needed to provide insights into this question.
Author contributions
CY conceived the sensitivity analysis, proposed statistical methods, performed all analyses, interpreted the results, and drafted and revised the manuscript. GB advised on important intellectual content and revised the manuscript. GB was also commissioned by the CTN to design, carry out, analyze, and report the original trial. LT and JB contributed to the statistical design of the sensitivity analysis and revision of the manuscript. All authors have read and approved the final manuscript.
Acknowledgments
CY is supported in part by funding from the Father Sean O’Sullivan Research Centre studentship award, the Canadian Institute of Health Research Training award in Bridging Scientific Domains for Drug Safety and Effectiveness, and the Canadian Network and Centre for Trials Internationally program.
Supplementary materials
Propensity score methods
The propensity score (PS) is the probability of receiving a treatment conditional on observed characteristics. Balancing on PS mimics randomization and produces comparable groups that are balanced on prognosis and observed confounding. We collected baseline variables that included child’s age, sex, admission diagnosis, parent’s age, marital status, education, distress, parenting style, family income, social support, and family functioning. Using those variables, we calculated the PS for receiving complete Children’s Treatment Network (CTN) integrated care by a logistic regression model. Four PS methods were used to balance between treated and untreated children in the AT and PP analyses, ie, matching, stratification, weighting, and covariate adjustment.
In PS matching, we created matched pairs of treated and untreated children by matching them within 0.2 of the standard deviation of the logit of the PS. We used a 1:1 ratio to match the nearest children without replacement. This matching algorithm produced the least bias.Citation1 We then used the generalized estimating equation model to analyze the matched data. An exchangeable correlation structure was used.
In PS stratification, children were divided into five equal strata by the quintiles of their PS.Citation2 The outcome between treated and untreated children was compared directly in each stratum. The overall treatment effect is given by
where βi is the estimated treatment effect in stratum i. The variance of βoverall is calculated by
where wi is the inverse of the estimated variance of βi for stratum i.
In the PS-weighted method, we balanced the children by the inverse probability of receiving CTN integrated care. The weight (wi) for each child can be calculated by
where Xi is the treatment indicator and ei is the estimated PS for child i. Confounding between the observed variables and treatment was eliminated in the weighted sample. We then directly compared the outcome between treated and untreated children using a weighted linear regression model.
In the covariate adjustment method, we adjusted the PS as the sole covariate in the regression model for estimating the treatment effect.
Calculating standard error for instrumental variable estimate
Suppose we have two random variables, X and Y. A Taylor series expansion of f(x, y) about the values (x0, y0) is given by
where o(⋅) represents the higher order terms which are omitted in this approximation. The instrumental variable (IV) estimator for the exposure X and the outcome Y is given by
where β(Z→Y) and β(Z→X) are the parameters of association between Z and Y and between Z and X, respectively. By the Taylor series expansion on βIV, we have
where b(Z→Y) and b(Z→X) are two values at which βIV is differentiable. The variance of the IV estimator can then be approximated as
Under the assumption that β(Z→χ) is independent of β(Z→Y), the variance of the IV estimator is then
We substitute b(Z→Y) and b(Z→X) by the estimates of ß(Z→Y) and ß(Z→X), respectively, and approximate the
and
by the associated standard error. Thus, we can obtain an approximate variance of the IV estimate. In our analysis, we used the least squares estimate of ß(Z→Y) and associated standard error obtained from the linear regression model. For the exposure X (a binary indicator of whether or not a patient received complete CTN integrated care), the association between X and the IV can be calculated by
where P(X = 1|Z = 1) represents the proportion of treated patients in the CTN group; and P(X = 1|Z = 0) is always zero because the children in the usual care group are deemed to be untreated. The variance of β(Z→X) is
where n is the number of children in the CTN group.
References
- AustinPCOptimal caliper widths for propensity-score matching when estimating differences in means and differences in proportions in observational studiesPharm Stat201110215016120925139
- RosenbaumPRRubinDBReducing bias in observational studies using subclassification on the propensity scoreJ Am Stat Assoc198479387516524
Disclosure
The authors report no conflicts of interest in this work.
References
- McPhersonMArangoPFoxHA new definition of children with special health care needsPediatrics199810211371399714637
- BethellCDReadDBlumbergSJNewacheckPWWhat is the prevalence of children with special health care needs? Toward an understanding of variations in findings and methods across three national surveysMatern Child Health J200812111417566855
- GurneyJGMcPheetersMLDavisMMParental report of health conditions and health care use among children with and without autism: National Survey of Children’s HealthArch Pediatr Adolesc Med2006160882516894082
- GhandourRMKoganMDBlumbergSJPerryDFPrevalence and correlates of internalizing mental health symptoms among CSHCNPediatrics20101252e269e27720083529
- PatelVFlisherAJHetrickSMcGorryPMental health of young people: a global public-health challengeLancet200736995691302131317434406
- IngoldsbyEMKohlGOMcMahonRJLenguaLConduct problems, depressive symptomatology and their co-occurring presentation in childhood as predictors of adjustment in early adolescenceJ Abnorm Child Psychol2006345602620
- CossetteLDuclosÉA profile of disability in Canada 2001 (PALS)Minister of IndustryStatistics Canada2001 Available from: http://ww.statcan.gc.ca/pub/89-577-x/Accessed May 20, 2013
- SimpsonGACohenRABloomBBlumbergSJThe impact of children’s emotional and behavioural difficulties on their lives and their use of mental health servicesPaediatr Perinat Epidemiol200923547248119689498
- RosenzweigJMBrennanEMHuffstutterKBradleyJRChild care and employed parents of children with emotional or behavioral disordersJ Emot Behav Disord20081627889
- BallTLearning from our past successesChildren’s Treatment Network Updates2013 Available from: http://www.ctn-simcoeyork.ca/resources/Learning%20From%20Our%20Past%20Successes.pdfAccessed May 20, 2013
- CarnevaleFARehmRSKirkSMcKeeverPWhat we know (and do not know) about raising children with complex continuing care needsJ Child Health Care20081214618287180
- The Well-being of Canada’s Young Children ReportGovernment of Canada2011 Available from: http://www.dpe-agje-ecd-elcc.ca/eng/ecd/well-being/page11.shtmlAccessed May 20, 2013
- HartleySLBarkerETSeltzerMMThe relative risk and timing of divorce in families of children with an autism spectrum disorderJ Fam Psychol201024444945720731491
- MillerARRecskyMAArmstrongRWResponding to the needs of children with chronic health conditions in an era of health services reformCan Med Assoc J2004171111366136715557591
- GhandourRMPerryDFKoganMDStricklandBBThe medical home as a mediator of the relation between mental health symptoms and family burden among children with special health care needsAcad Pediatr201111216116921306969
- Medical Home Initiatives for Children With Special Needs Project Advisory CommitteeAmerican Academy of Pediatrics. The medical homePediatrics20021101 Pt 118418612093969
- StricklandBBSinghGKKoganMDMannMYvan DyckPCNewacheckPWAccess to the medical home: new findings from the 2005–2006 national survey of children with special health care needsPediatrics20091236e996e100419482751
- StarfieldBShiLThe medical home, access to care, and insurance: a review of evidencePediatrics2004113Suppl 41493149815121917
- ColpeLJEstimates of mental and emotional problems, functional impairments, and associated disability outcomes for the US child population in households Available from: http://www.mentalhealth.org/publications/allpubs/SMA01%2D3537/chapter19.aspAccessed August 15, 2013
- PalfreyJSSofisLADavidsonEJLiuJFreemanLGanzMLThe Pediatric Alliance for Coordinated Care: evaluation of a medical home modelPediatrics2004113Suppl 41507151615121919
- ThurstonSPaulLBrowneGEarly results: the 2-year comparative effect and expense of more and less integration of services that provide treatment and rehabilitation for children with multiple disabilities: a randomized controlled trial Children’s Treatment Network of Simcoe York, Final research report32010
- ThurstonSPaulLYeCSystem integration and its influence on the quality of life of children with complex needsInt J Pediatr2010201057020920976132
- ThurstonSPaulLLoneyPYeCWongMBrowneGAssociations and costs of parental symptoms of psychiatric distress in a multi-diagnosis group of children with special needsJ Intellect Disabil Res201155326328021199042
- SchulzKFAltmanDGMoherDCONSORT 2010 statement: updated guidelines for reporting parallel group randomised trialsBMC Med2010811820334633
- Simcoe York District Health Council Profile II42002 Available from: http://www.ontla.on.ca/library/repository/mon/10000/250806.pdfAccessed May 20, 2013
- Disability in Canada: A 2001 profileOffice of Disabilities, Human Resources Development Canada2003 Available from: http://publications.gc.ca/collections/Collection/RH37-34-4-2001E.pdfAccessed May 20, 2013
- WeissESAndersonRMLaskerRDMaking the most of collaboration: exploring the relationship between partnership synergy and partnership functioningHealth Educ Behav200229668369812456129
- VarniJWSeidMRodeCAThe PedsQL (TM): measurement model for the pediatric quality of life inventoryMed Care199937212613910024117
- KesslerRCMcGonagleKAZhaoSLifetime and 12-month prevalence of DSM-III-R psychiatric disorders in the United States: results from the National Comorbidity SurveyArch Gen Psychiatry19945118198279933
- StrayhornJMWeidmanCSA parent practices scale and its relation to parent and child mental healthJ Am Acad Child Adolesc Psychiatry19882756136183182627
- CutronaCERussellDWThe provisions of social relationships and adaptation to stressAdvances in Personal Relationships1987113767
- BylesJByrneCBoyleMHOffordDROntario Child Health Study: reliability and validity of the general functioning subscale of the McMaster Family Assessment DeviceFam Process1988271971043360100
- VarniJWSeidMKurtinPSPedsQL (TM) 4.0: reliability and validity of the pediatric quality of life Inventory (TM) version 4.0 generic core scales in healthy and patient populationsMed Care200139880081211468499
- RubinDBMultiple Imputation for Nonresponse in SurveysNew York, NYJohn Wiley and Sons2009
- GreenlandSAn introduction to instrumental variables for epidemiologistsInt J Epidemiol200029472272910922351
- ShefferLCHanleyCBagleyAMolitorFJamesMAComparison of self-reports and parent proxy-reports of function and quality of life of children with below-the-elbow deficiencyJ Bone Joint Surg Am200991122852285919952247
- OwensPLHoagwoodKHorwitzSMBarriers to children’s mental health servicesJ Am Acad Child Adolesc Psychiatry200241673173812049448
- TorgersonDJContamination in trials: is cluster randomisation the answer?BMJ2001322728235535711159665
- EnglishMSchellenbergJToddJAssessing health system interventions: key points when considering the value of randomizationBull World Health Organ2011891290791222271948
- YeCBrowneGGrdisaVSBeyeneJThabaneLMeasuring the degree of integration for an integrated service networkInt J Integr Care201212e13723593050
- KuhlthauKABloomSVan CleaveJEvidence for family-centered care for children with special health care needs: a systematic reviewAcad Pediatr201111213621396616
- KingGKingSRosenbaumPGoffinRFamily-centered caregiving and well-being of parents of children with disabilities: linking process with outcomeJ Pediatr Psychol19992414153
- TolanPHDodgeKAChildren’s mental health as a primary care and concern: a system for comprehensive support and serviceAm Psychol200560660161416173893
- Briggs-GowanMJOwensPLSchwab-StoneMELeventhalJMLeafPJHorwitzSMPersistence of psychiatric disorders in pediatric settingsJ Am Acad Child Adolesc Psychiatry200342111360136914566174
- CookJRKilmerRPSystems of care: new partnerships for community psychologyAm J Community Psychol2012493–439340322538407