Abstract
Background
The aim of the study was to investigate potential prognostic microRNA (miRNA) biomarkers for patients with early stage pancreatic ductal adenocarcinoma (PDAC) after pancreaticoduodenectomy using a miRNA-sequencing (miRNA-seq) data set from The Cancer Genome Atlas (TCGA). A miRNA expression-based prognostic signature was generated, and the potential role of target genes in overall survival (OS) in patients with PDAC was examined.
Methods
A miRNA-seq data set of 112 PDAC patients who underwent pancreaticoduodenectomy was obtained from TCGA. Survival analysis was performed to identify potential prognostic biomarkers.
Results
Eleven miRNAs (hsa-mir-501, hsa-mir-4521, hsa-mir-5091, hsa-mir-24-1, hsa-mir-126, hsa-mir-30e, hsa-mir-3157, hsa-let-7a-3, hsa-mir-133a-1, hsa-mir-4709, and hsa-mir-421) were used to construct a prognostic signature using the step function. The 11-miRNA prognostic signature showed good performance for prognosis prediction (adjusted P<0.0001, adjusted hazard ratio =4.285, 95% confidence interval =2.146–8.554), and the time-dependent receiver operating characteristic analysis showed an area under the curve of 0.864, 0.877, and 0.787 for 1-, 2-, and 3-year PDAC OS predictions, respectively. Comprehensive survival analysis suggested that the prognostic signature could serve as an independent prognostic factor for PDAC OS and performs better in prognosis prediction than other traditional clinical indicators. Functional assessment of the target genes of the miRNAs indicated that they were significantly enriched in multiple biological processes and pathways, including cell proliferation, cell cycle biological processes, the forkhead box O, mitogen-activated protein kinase, Janus kinase/signal transducers and activators of transcription signaling pathways, pathways in cancer, and the ErbB signaling pathway. Several target genes of these miRNAs were also associated with PDAC OS.
Conclusion
The present study identified a novel miRNA expression signature that showed potential as a prognostic biomarker for PDAC after pancreaticoduodenectomy.
Introduction
Pancreatic cancer (PC) is a highly aggressive malignant tumor of the digestive tract that is difficult to diagnose and treat. In >80% of PC cases, the histological type is pancreatic ductal adenocarcinoma (PDAC).Citation1–Citation3 According to Chinese National Cancer Center statistics, the morbidity and mortality of PC increased significantly in recent years, and ~90,100 newly diagnosed PC cases and 79,400 deaths were reported in People’s Republic of China in 2015.Citation4 The age-standardized 5-year survival rate of PC in People’s Republic of China is 11.7%, whereas the overall 5-year survival rate of PC in the United States is <7%.Citation5,Citation6 In addition to the highly malignant characteristics of PC, it is associated with a low rate of early diagnosis and high surgical mortality; the efficacy of existing treatments is limited and the cure rate is very low. Therefore, novel reliable biomarkers and molecular targets are urgently needed to combat this highly malignant and deadly cancer.
Despite improvements in our understanding of the pathogenesis of PC, currently there are no effective strategies for the early diagnosis and clinical treatment of PC. MicroRNAs (miRNAs) are closely related to tumorigenesis, and miRNAs associated with PC are being identified.Citation7–Citation9 Differentially expressed miRNAs are tissue-specific and disease-specific. These characteristics are helpful for designing new diagnostic methods, and identifying treatment targets and strategies for the differential diagnosis and treatment of PC.Citation7,Citation9 Clinical and pathological biomarkers of prognosis have not successfully guided clinicians in selecting adjuvant therapies for PDAC patients after surgical resection. Growing evidence suggests that the whole genome miRNA sequencing data sets provide potential tumor-specific prognostic information to assist clinicians in appropriately selecting patients for adjuvant therapy.Citation10 These potential molecules are often dysregulated and play oncogenic and/or tumor suppressor functions in PDAC. The government of United States has initiated a project The Cancer Genome Atlas (TCGA) to map out the genome variations of human cancers by applying genomic analysis techniques, especially using large-scale genome sequencing. The aim of this project was to systematically analyze all cancer-causing and inhibitory, small variations in oncogenes to understand the mechanism of cancer cell tumorigenesis and development and to help designing cancer prevention strategies.Citation11,Citation12 TCGA has yielded single-nucleotide variation data, RNA-seq data, copy number variation data, methylation data, clinical data, miRNA-sequencing (miRNA-seq) data, and biological sample data. These genome-wide data sets are open access and allow researchers to conduct comprehensive cancer studies.
In our previous study, we identified genes related to prognosis in patients with early stage PDAC after pancreaticoduodenectomy based on RNA-seq data from TCGA and proposed a risk score model for PDAC prognosis prediction.Citation13 In addition, we also used TCGA RNA-seq data set to investigate the potential prognostic value of the alcohol dehydrogenase genes in PC.Citation14 The aim of the present study was to identify potential prognostic miRNA biomarkers for patients with early stage PDAC after pancreaticoduodenectomy using the miRNA-seq data set from TCGA. An miRNA expression-based prognostic signature was generated, and the potential role of the miRNA target genes in the overall survival (OS) in patients with PDAC was investigated.
Materials and methods
Data source and pre-processing
TCGA PDAC level 3 miRNA expression data set was downloaded from TCGA data portal (https://portal.gdc.cancer.gov/, accessed January 30, 2018).Citation15 The corresponding clinical information was obtained from the University of California Santa Cruz Xena browser (UCSC Xena: http://xena.ucsc.edu/, accessed April 20, 2017). The raw miRNA data set of PDAC was normalized using the DESeq package in the R platform, and miRNAs with a mean value of >0 were included in the subsequent analysis.Citation16 The inclusion criteria for patients were listed in our previous study which are as follows: 1) complete survival data available; 2) the histology type was PDAC; 3) American Joint Committee on Cancer (AJCC 7th) pathological stage I or II; and 4) patients who underwent pancreaticoduodenectomy.Citation13 PDAC patients with pathological stage III or IV disease and who underwent other types of surgery were excluded.Citation13 Additional approval by an ethics committee was not necessary because the data set used in the current study was downloaded from TCGA, and data acquisition and application were performed according to TCGA publication guidelines and data access policies.
Identification of prognosis-related miRNAs
The prognostic value of miRNAs was first assessed using the univariate Cox proportional hazards regression model, and the evaluation was performed using a survival package. A P-value of <0.05 in the survival analysis was considered statistically significant, and prognosis-related miRNAs regarding PDAC OS were identified.
Construction of the prognostic model and receiver operating characteristic (ROC) curve
Prognosis-related miRNAs that were included in the prognostic signature combination screening were assessed by a “step” function to select the optimal combination. Then, the combination leading to the most significant P-value was used for the construction of the prognostic model. The relative contribution of these prognostic miRNAs to PDAC survival prediction was assessed by fitting the selected miRNAs to a multivariate Cox regression analysis with OS as the dependent variable. The miRNA expression-based prognostic risk score model was constructed by the linear combination of the expression levels of miRNAs with the multivariate Cox regression coefficient (β) as the weight. The risk score formula was as follows: risk score = expression of miRNA1 × β1miRNA1 + expression of miRNA2 × β2miRNA2 +… expression of miRNAn × βnmiRNAn. Citation13,Citation17,Citation18 High- and low-risk patients were grouped by the median value of the risk score. The predictive accuracy of the miRNA expression-based prognostic signature for PDAC OS was assessed using the survivalROC package in the R platform.Citation13,Citation17
Stratified and joint effect survival analysis
A stratified and joint effect survival analysis was performed to investigate the association between the risk score and clinical characteristics of patients with PDAC with respect to the miRNA expression-based prognostic signature. A nomogram was constructed to assess the individualized prognosis prediction model based on the clinical characteristics and risk score.
Functional assessment
The TargetScan (http://www.targetscan.org/, accessed February 28, 2018),Citation19,Citation20 miRDB (http://www.mirdb.org/, accessed February 28, 2018),Citation21,Citation22 and miRTarBase (http://mirtarbase.mbc.nctu.edu.tw/, accessed February 28, 2018)Citation23,Citation24 algorithms were used to predict the target genes of these prognostic miR-NAs. The overlapping target genes in these three databases were identified as miRNA target genes and used for further enrichment analysis. The miRNA target genes interaction networks were constructed using Cytoscape v3.4.0. The functional enrichment of these miRNA target genes was performed using the Database for Annotation, Visualization and Integrated Discovery v6.8 (DAVID v6.8; https://david.ncifcrf.gov/home.jsp, accessed February 28, 2018)Citation25,Citation26 and visualized using the ggplot2 package.
Statistical analysis
False discovery rate (FDR) in DESeq was adjusted for multiple testing using the Benjamini–Hochberg procedure.Citation27–Citation29 Univariate analysis of clinical features and OS was performed using the log-rank test; clinical features with a P-value <0.05 were entered into the multivariate Cox proportional hazards regression model for adjustment. A P-value <0.05 was considered statistically significant. All statistical analyses were performed using SPSS version 20.0 and R 3.3.0.
Results
Study population
A total of 178 cases in the miRNA-seq data set were downloaded from TCGA, and the corresponding survival profiles were obtained from the UCSC Xena browser. A total of 112 early stage PDAC patients met the inclusion and exclusion criteria and were further analyzed. Most of the early stage PDAC patients were at stage II, whereas eight patients were at stage I. Survival analysis showed no significant difference in OS between stage I and stage II patients in the current study (log-rank, P=0.943; hazard ratio [HR] =1.038; 95% CI =0.375–2.872; ); because of the reason that only eight patients with stage I were included in the survival analysis. Univariate analysis identified the following clinical features as significantly associated with PDAC OS: histological grade (log-rank P=0.01, HR =1.919, 95% CI =1.156–3.185; ), radical resection (log-rank P=0.009, HR =0.514, 95% CI =0.310–0.852; ), radiation therapy (log-rank P=0.029, HR =0.527, 95% CI =0.293–0.947; ), and targeted molecular therapy (log-rank P<0.0001, HR =0.168, 95% CI =0.095–0.296; ). These features were included in the multivariate Cox proportional hazard regression model.
Table 1 Table FootnoteΨAssociation between OS and clinicopathologic features of PDAC patients
Prognosis-related miRNA screening
After normalization by the DESeq package in the R platform, 1457 miRNAs met the criterion of mean expression values >0. The normalized data were used for univariate Cox regression survival analysis using the survival package in the R platform. There were 83 miRNAs significantly associated with PDAC OS (Table S1). Further analysis of these prognostic miRNAs showed low expression in PDAC tumor tissues, and prognostic miRNAs with expression values of 0, are present in more than half of the samples or mean expression values of <1 were excluded. Finally, 26 prognostic miRNAs were included in the prognostic signature combination screening using the “step” function.
Prognostic model construction and ROC curve analysis
After investigation of the “step” function for these prognostic miRNAs, the most significant candidate combinations based on the expression of these candidate prognostic miRNAs were selected. The following 11 prognostic miRNAs were used for the construction of the prognostic signature: hsa-mir-501, hsa-mir-4521, hsa-mir-5091, hsa-mir-24-1, hsa-mir-126, hsa-mir-30e, hsa-mir-3157, hsa-let-7a-3, hsa-mir-133a-1, hsa-mir-4709, and hsa-mir-421. The results of the Kaplan–Meier analysis of these prognostic-related miRNAs are shown in . The relative contribution of these prognostic miRNAs was assessed by multivariate Cox regression, with the multivariate Cox regression coefficient (β) as the weight. The risk score formula was as follows: risk score = hsa-mir-501 × (−0.554) + hsa-mir-4521 × (0.594) + hsa-mir-5091 × (0.440) + hsa-mir-24-1 × (0.511) + hsa-mir-126 × (0.670) + hsa-mir-30e × (−0.562) + hsa-mir-3157 × (−0.575) + hsa-let-7a-3 × (0.439) + hsa-mir-133a-1 × (−0.477) + hsa-mir-4709 × (−0.532) + hsa-mir-421 × (0.590). Patients were divided into low- and high-risk groups according to the median risk scores, and the survival analysis indicated that patients with high risk scores were significantly associated with a poor clinical outcome (median survival time, high risk vs low risk: 308 vs 695 days) and increased risk of death (adjusted P<0.0001; adjusted HR =4.285; 95% CI =2.146–8.554; ). Time-dependent ROC curve analysis was used to evaluate the predictive accuracy of this prognostic signature, and the results suggested that the prognostic signature constructed in the current study performed well in 1-, 2-, and 3-year survival predictions. The area under the curve for 1-, 2-, and 3-year survival was 0.864, 0.877, and 0.787 (), respectively. The distribution of the expression of the miRNAs in the high- and low-risk groups is shown in .
Figure 1 The Kaplan–Meier curves of 11 prognostic miRNAs in PDAC.
Notes: The order of Kaplan–Meier curves of 11 prognostic miRNAs were as follows: hsa-mir-501 (A), hsa-mir-4521 (B), hsa-mir-5091 (C), hsa-mir-24-1 (D), hsa-mir-126 (E), hsa-mir-30e (F), hsa-mir-3157 (G), hsa-let-7a-3 (H), hsa-mir-133a-1 (I), hsa-mir-4709 (J), and hsa-mir-421 (K).
Abbreviations: miRNA, microRNA; PDAC, pancreatic ductal adenocarcinoma.
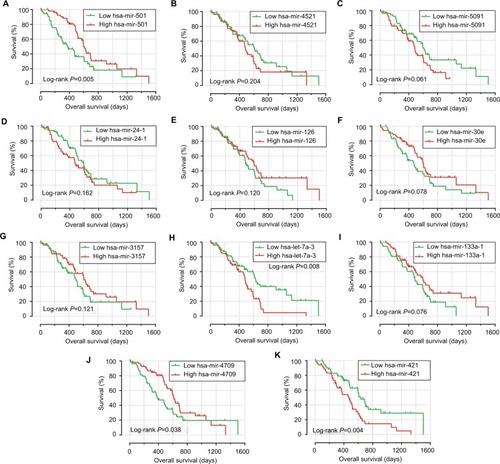
Figure 2 Prognostic risk score model analysis of 11 prognostic miRNAs in PDAC patients.
Notes: (A) From top to bottom are the risk score, patients’ survival status distribution, and 11 prognostic miRNA expression heat maps for low- and high-risk groups. (B) Kaplan–Meier curves for low- and high-risk groups. (C) ROC curve for predicting survival in PDAC patients by the risk score.
Abbreviations: miRNA, microRNA; PDAC, pancreatic ductal adenocarcinoma; ROC, receiver operating characteristic; AUC, area under curve.
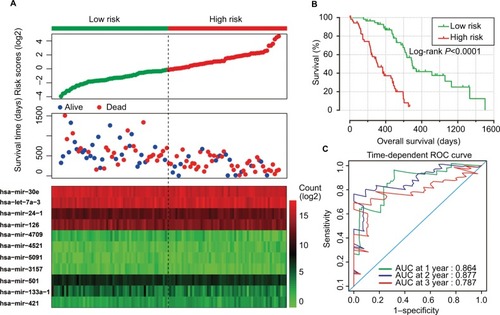
Stratified and joint effect analysis
Further investigation of the relation between the prognostic signature and the clinical characteristics associated with PDAC OS was performed by a comprehensive analysis of the nomogram and stratified and joint effect survival analysis. The stratified analysis indicated that patients with a high risk score showed a significantly increased risk of death in all favorable strata except in patients at stage I and in those younger than 60 years, and in all adverse strata except grade G3/G4 and patients who did not receive targeted molecular therapy (). A nomogram was visualized by rms and its auxiliary packages based on the clinical characteristics of PDAC and risk scores, and it demonstrated that the 11-miRNA prognostic signature contributed the most risk points, whereas the other clinical characteristics contributed much less ().
Figure 4 The relationship between risk score and clinical information.
Notes: (A) Stratified analysis of association between risk score and OS in PDAC. (B) Nomogram for predicting the 1-, 2-, and 3-year event (death) with risk score and clinical information.
Abbreviations: PDAC, pancreatic ductal adenocarcinoma; OS, overall survival.
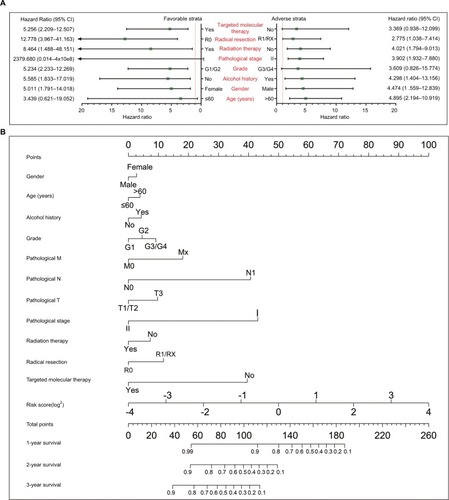
Joint effect survival analysis between the 11-miRNA prognostic signature and clinical parameters suggested that this prognostic signature performed well in PDAC OS predictions, and combination with clinical parameters significantly associated with PDAC OS showed more significant predictive values for PDAC OS (; ).
Table 2 Joint effect survival analysis of clinical factors and the risk score with OS in PDAC patients
Figure 5 Joint effect analysis of OS stratified by risk score and PDAC clinical parameters.
Notes: Joint effect analysis stratified by risk score and following clinical parameters: histological grade (A), radiation therapy (B), radical resection (C), and targeted molecular therapy (D).
Abbreviations: PDAC, pancreatic ductal adenocarcinoma; OS, overall survival.
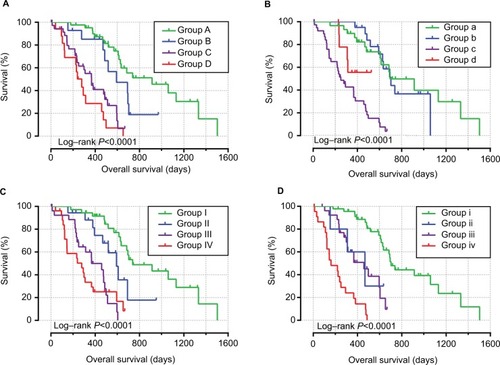
Functional assessment
The target genes of these 11 miRNAs were analyzed using three independent miRNA target gene prediction websites: TargetScan, miRDB, and miRTarBase. Target genes overlapping in the three websites were identified as miRNA target genes. Among the 11 miRNAs, hsa-mir-4709, hsa-mir-501, hsa-mir-30e, hsa-mir-126, and hsa-mir-3157 had overlapping target genes in the three websites (). Enrichment analysis of these target genes was performed using DAVID v6.8. Gene Ontology term enrichment results, which suggested that these target genes were significantly enriched in cell division, positive regulation of autophagy, G1/S transition of mitotic cell cycle, cell proliferation, and cell–cell adhesion mediated by integrin biological processes (). Kyoto Encyclopedia of Genes and Genomes (KEGG) enrichment analysis indicated that these target genes were significantly correlated with the forkhead box O (FOXO), mitogen-activated protein kinase (MAPK), Janus kinase/signal transducers and activators of transcription (JAK/STAT), pathways in cancer, ErbB, cell cycle, and PC (hsa05212, Figure S1) signaling pathways (). To further investigate the role of these target genes in PDAC OS, we performed a survival analysis of these target genes using the survival package. The mRNA expression data set of PDAC patients, which was the same as that in the present study, was generated from our previous studies of TCGA PDAC mRNA data analysis, and the data set was also normalized using the DESeq package in the R platform.Citation13 Among the 223 target genes, 28 were significantly correlated with PDAC OS in univariate analysis (Table S2), and the Kaplan–Meier curves of the top five significant target genes ranked by the P-values are shown in .
Figure 6 Interaction networks of the prognostic miRNAs and their target genes.
Notes: Red diamonds represent miRNAs, green circles represent target genes, and the link in black indicates a miRNA target genes relationship.
Abbreviation: miRNA, microRNA.
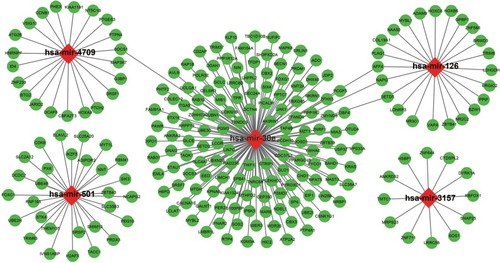
Figure 7 Functional assessment of the target genes of these 11 prognostic related miRNAs.
Notes: (A) GO term enrichment results of target genes; (B) KEGG enrichment results of target genes.
Abbreviations: GO, Gene Ontology; KEGG, Kyoto Encyclopedia of Genes and Genomes.
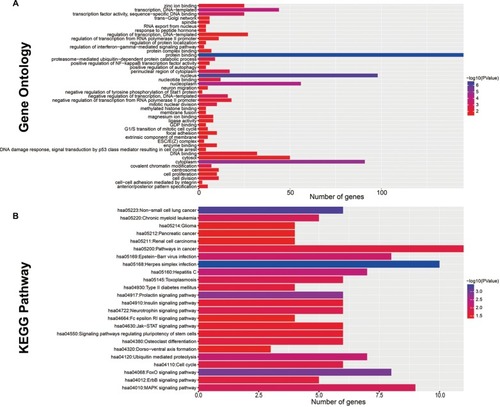
Figure 8 Survival analysis of the top five significant target genes.
Notes: The order of Kaplan–Meier curves of the top five significant target genes were as follows: CBX3 (A), ERGIC2 (B), STRIP1 (C), CCNB1 (D), and PTCH2 (E).
Abbreviations: CBX3, chromobox 3; ERGIC2, ERGIC and golgi 2; STRIP1, striatin interacting protein 1; CCNB1, cyclin B1; PTCH2, patched 2.
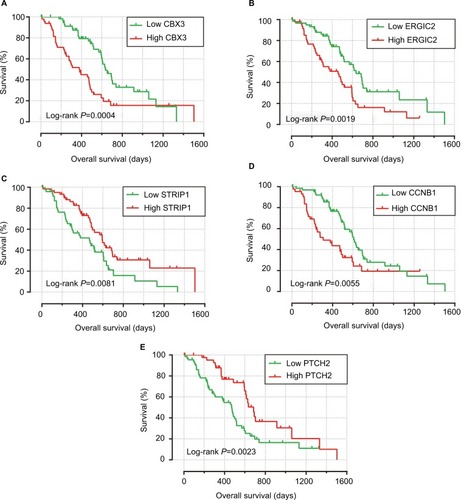
Discussion
Genome-wide data filtering is one of the most promising tools for the identification of biomarkers and therapeutic targets. The notion that miRNAs are closely related to the prognosis of cancers is increasingly recognized. Many cancer miRNA-seq data sets with complete clinical information has been shared on TCGA for researchers to download and investigate. The miRNAs of TCGA PC miRNA-seq data set were analyzed in previous studies. Zhou et al investigated a 13-miRNA expression-based prognostic signature for PC survival prediction including 167 PC patients from TCGA.Citation30 Liang et al identified 10 miRNAs as potential prognostic bio-markers using 175 pancreatic adenocarcinoma patients from TCGA.Citation31 However, the PC patients in TCGA included several histological types of PC other than PDAC, such as poorly differentiated pancreatic adenocarcinoma and pancreas-colloid (mucinous non-cystic) carcinoma. In addition, the surgical procedures of these patients included distal pancreatectomy, total pancreatectomy, and pancreaticoduodenectomy. The histological types and surgical procedures were important prognostic factors that may affect the survival outcome of PC patients.Citation32–Citation34 However, the studies by Zhou et al and Liang et al did not include these prognostic factors in the multivariate Cox proportional hazards model or stratified analysis.Citation30,Citation31 Therefore, the prognostic miRNAs identified in their studies may be unreliable. In the present study, we included only patients at early stage of PDAC who underwent pancreaticoduodenectomy and excluded patients at advanced pathological stages.
In the current study, we identified 83 potential prognostic miRNAs, and 11 miRNAs were used for the construction of the prognostic signature. The 11-miRNA expression-based prognostic signature constructed in the present study classified the patients into high- and low-risk groups, and it performed well in 1-, 2-, and 3-year survival predictions. The nomogram and stratified and joint effect survival analysis also indicated that this prognostic signature performed better in PDAC OS prediction than other clinical parameters.
Among these 11 prognostic miRNAs, five (hsa-mir-4521, hsa-mir-5091, hsa-mir-3157, hsa-mir-133a-1 and hsa-mir-4709) had not been previously investigated for their functional roles in cancer, whereas the remaining six miRNAs (hsa-mir-501, hsa-mir-24-1, hsa-mir-126, hsa-mir-30e, hsa-let-7a-3 and hsa-mir-421) had been previously reported to be associated to cancer. Ling et al reported that miR-501-3p was highly expressed in PDAC tumor tissues, and low expression of miR-501-3p was significantly associated with a low risk of tumor recurrence based on a cohort of 64 PDAC patients.Citation35 Similar results were reported in hepatocellular carcinoma (HCC) and gastric cancer (GC), indicating that miR-501-5p was markedly upregulated in HCC and GC tumor tissues, and high expression of miR-501-5p had significantly increased the risk of death in patients with GC.Citation36,Citation37 However, comparing with the previous studies, the result of hsa-mir-501 in the current study obtained an opposite prognosis results, because both the current study and study by Ling et alCitation35 in PDAC prognosis were with a small sample size; therefore, further verification cohort is needed to verify our results. Another miRNA, hsa-mir-24-1, was shown to be downregulated in cutaneous malignant melanoma tumor tissues.Citation38 Regarding the third miRNA, hsa-mir-126, a study by Feng et al observed that the combined overexpression of miR-126 and miR-34a exerts a more effective antitumor effect than a single miRNA and may be a promising system for PC targeted therapy.Citation39 MiR- 126 is downregulated in thyroid cancer cells and esophageal cancer tissues and suggested to play a tumor suppressor role in renal cell carcinoma and thyroid cancer by targeting specific genes.Citation40–Citation42 Debernardi et al reported that miR-30e is significantly upregulated in urine samples in stage I PDAC patients compared with healthy subjects and performed well in discriminating these patients, as determined by ROC analysis.Citation43 However, miR-30e is downregulated in the sera of HCC and non–small cell lung cancer (NSCLC) patients compared with healthy volunteers.Citation44,Citation45 In terms of clinical outcome prediction and low serum levels of miR-30e-3p were significantly associated with poor disease-free survival (DFS), whereas high serum levels of miR-30e-5p were markedly correlated to a shorter OS in NSCLC.Citation45,Citation46 In other cancers, the potential prognostic role of miR-30e is complex. High expression of miR-30e showed a protective effect regarding the clinical outcomes of patients with ovarian carcinoma and breast cancer, which were consistent with our results in PDAC, whereas it served as a risk factor for esophageal cancer OS and DFS.Citation47–Citation49 Dysregulation of hsa-let-7a-3 is associated with the prognosis of cancers and regulated by DNA methylation.Citation50–Citation52 Hyper-methylation of let-7a-3 in acute myeloid leukemia (AML) patients with hypomethylated CCAAT enhancer-binding protein alpha (CEBPA) is significantly correlated with better OS, and overexpression of let-7a-3 is associated with poor OS in AML, which are consistent with our results in PDAC.Citation50,Citation53 Lu et al showed that methylation of let-7a-3 is inversely correlated with insulin-like growth factor-II (IGFII) expression, and let-7a-3 hypermethylation significantly reduces the risk of death in ovarian cancer.Citation52 However, a similar study by Lu et al in breast cancer demonstrated that epigenetic regulation of let-7a-3 may affect the activity of IGFs, whereas it is not associated with DFS or OS in patients with breast cancer.Citation51 MiR-421 is markedly upregulated in GC,Citation54–Citation56 HCC,Citation57,Citation58 PC,Citation59 biliary tract cancer,Citation60 osteosarcoma,Citation61 and nasopharyngeal carcinoma tumor tissues,Citation62 and high expression of miR421 is significantly associated with poor clinical outcomes in patients with GC,Citation55,Citation63 HCC,Citation58 and osteosarcoma.Citation61 MiR421 acts as an oncomiRNA in GC,Citation55 HCC,Citation57 PC,Citation59 and nasopharyngeal carcinoma,Citation62 and overexpression of miR421 in these cancer cells promotes cell proliferation. However, miR421 may play a tumor-suppressor role in prostate cancer cells, as overexpression of miR-421 significantly suppressed prostate cancer cell growth, and knockdown of miR-421 improved proliferation.Citation64 Despite the opposite roles of miR-421 in previous studies, the current study indicated that high expression of hsa-mir-421 significantly increased the risk of death in PDAC, which was consistent with previous PC studies and several cancer studies.
In function enrichment analysis, these prognostic miRNA target genes were significantly enriched in the cell cycle, cell proliferation biological processes, and several signaling pathways such as FOXO, MAPK, JAK-STAT, and ErbB signaling pathways. Cell cycle and cell proliferation involve in maintaining the basic state of cells, and dysregulation of cell cycle components may lead to tumor formation.Citation65 These prognostic miRNAs may play a role in maintaining the stability of cellular states through the regulation of target genes. Roy et al demonstrated that inhibition of the PI3K/AKT and MAPK/ERK pathways in PC induces the activation of FOXO transcription factor, leading to cell cycle arrest and apoptosis.Citation66 The MAPK pathway is not only involved in the formation and development of PC but also mediates the sensitivity of PC cells to chemotherapy drugs.Citation67,Citation68 A similar function of ErbB signaling was reported in previous studies, and targeting the regulation of the ErbB pathway by sex-determining region Y-related high mobility group box 9 was involved in pancreatic tumorigenesis; dual targeting of ErbB-2 and ErbB-3 increases the therapeutic efficacy of trastuzumab.Citation69,Citation70 Similar anti-tumor drugs in PC target the JAK-STAT pathway and may have a potential application in chemotherapy and immunotherapy.Citation71,Citation72 Therefore, we can hypothesize that these prognostic miRNAs are involved in biological processes and signaling pathways by regulating their target genes and affecting PC tumorigenesis and treatment. Regarding the top five significant target genes, the present study is the first to identify a significant association between these mRNA expression levels and PDAC OS. The prognostic values for these miRNAs and most of their putative target genes seem to be in good agreement. Among the 28 target genes, which were significantly correlated with PDAC OS, only one target gene of hsa-mir-3157 and two target genes of hsa-mir-126 were significantly associated with PDAC OS, and the prognostic values between these miRNAs and target genes showed an opposite trend and in good agreement. In addition, three in six prognostic target genes of hsa-mir-4709, three in six prognostic target genes of hsa-mir-501, and five in 13 prognostic target genes of hsa-mir-30e also show the opposite trend in PDAC prognosis, which were in a good agreement with the miRNA-target gene regulation relationship. In one way, these results also support the validity of the prediction of presented miRNA–target genes interaction.
The present study had several limitations. First, the clinical information from TCGA database was not comprehensive, and we were unable to perform an accurate evaluation of the risk score model using the multivariate Cox proportional hazards model or stratified analysis. Second, because of the strict inclusion and exclusion criteria, the sample size in our study was relatively small, and a larger sample size with well-designed validation cohorts is needed to validate our results. Third, because of the relatively small sample size, patients with long-term survival of more than 3 years were rare; therefore, we were unable to assess the 5-year survival prediction accuracy or other long-term survival.
Despite these limitations, the present study identified an 11-miRNA expression-based prognostic signature for PDAC OS prediction, and it performed better than other clinical parameters. We also investigated the functions and potential prognostic roles of the target genes of these miRNAs in PDAC. These findings may provide insight into the roles of miRNAs in PDAC prognosis and have potential clinical application value in PDAC prognosis prediction, targeted therapy, and decision-making regarding treatment strategy and management.
Conclusion
Through an integrative analysis of the genome-wide miRNA-seq and clinical data of PDAC generated by TCGA, we found a miRNA expression-based signature including 11 miRNAs, which may serve as a potential biomarker for PDAC prognosis prediction. However, these results need further verification and investigation.
Acknowledgments
This work was supported in part by the National Nature Science Foundation of China (No. 81560535, 81072321, 30760243, 30460143, and 30560133), 2009 Program for New Century Excellent Talents in University (NCET), Guangxi Nature Sciences Foundation (No. GuiKeGong 1104003A-7), and Guangxi Health Ministry Medicine Grant (Key-Scientific Research-Grant Z201018). The present study is also partly supported by Self-raised Scientific Research Fund of the Health and Family Planning Commission of Guangxi Zhuang Autonomous Region (Z2016318), The Basic Ability Improvement Project for Middle-aged and Young Teachers in Colleges and Universities in Guangxi (2018KY0110), and Research Institute of Innovative Think-tank in Guangxi Medical University (the gene–environment interaction in hepatocarcinogenesis in Guangxi HCCs and its translational applications in the HCC prevention). We would also acknowledge the supported by the National Key Clinical Specialty Programs (General Surgery and Oncology) and the Key Laboratory of Early Prevention and Treatment for Regional High-Incidence-Tumor (Guangxi Medical University), Ministry of Education, People’s Republic of China. The authors also want to thank Prof Xinping Ye, who is from the Department of Hepatobiliary Surgery, The First Affiliated Hospital of Guangxi Medical University (Nanning, Guangxi, People’s Republic of China), for his contribution on data processing, manuscript design, and revision. This study was also supported in part by the funding from Prof Xinping Ye’s project: Innovation Project of Guangxi Graduate Education (JGY2018037). The authors thank the contributors of TCGA (https://portal.gdc.cancer.gov/) and UCSC Xena (http://xena.ucsc.edu/) for sharing the PDAC data on open access. In addition, we would also like to acknowledge the helpful comments on this manuscript received from our reviewers.
Disclosure
The authors report no conflicts of interest in this work.
References
- SeufferleinTBachetJBVan CutsemERougierPGroup EGWPancreatic adenocarcinoma: ESMO-ESDO Clinical Practice Guidelines for diagnosis, treatment and follow-upAnn Oncol201223Suppl 7vii33vii4022997452
- SiegelRMaJZouZJemalACancer statistics, 2014CA Cancer J Clin201464192924399786
- TorreLABrayFSiegelRLFerlayJLortet-TieulentJJemalAGlobal cancer statistics, 2012CA Cancer J Clin20156528710825651787
- ChenWZhengRBaadePDCancer statistics in China, 2015CA Cancer J Clin201666211513226808342
- ZengHZhengRGuoYCancer survival in China, 2003–2005: a population-based studyInt J Cancer201513681921193025242378
- SiegelRLMillerKDJemalACancer statistics, 2016CA Cancer J Clin201666173026742998
- ChitkaraDMittalAMahatoRImiRNAs in pancreatic cancer: therapeutic potential, delivery challenges and strategiesAdv Drug Deliv Rev201581345225252098
- RachaganiSMachaMAHeimannNClinical implications of miRNAs in the pathogenesis, diagnosis and therapy of pancreatic cancerAdv Drug Deliv Rev201581163325453266
- CostelloEGreenhalfWNeoptolemosJPNew biomarkers and targets in pancreatic cancer and their application to treatmentNat Rev Gas-troenterol Hepatol201298435444
- WaldPLiuXSPettitCPrognostic value of microRNA expression levels in pancreatic adenocarcinoma: a review of the literatureOncotarget2017842733457336129069873
- TomczakKCzerwinskaPWiznerowiczMThe Cancer Genome Atlas (TCGA): an immeasurable source of knowledgeContemp Oncol2015191AA68A77
- Cancer Genome Atlas Research NetworkWeinsteinJNCollissonEAThe Cancer Genome Atlas Pan-Cancer analysis projectNat Genet201345101113112024071849
- LiaoXHuangKHuangRGenome-scale analysis to identify prognostic markers in patients with early-stage pancreatic ductal adenocarcinoma after pancreaticoduodenectomyOnco Targets Ther2017104493450628979141
- LiaoXHuangRLiuXDistinct prognostic values of alcohol dehydrogenase mRNA expression in pancreatic adenocarcinomaOnco Targets Ther2017103719373228769575
- Cancer Genome Atlas Research NetworkIntegrated genomic characterization of pancreatic ductal adenocarcinomaCancer Cell2017322185203.e11328810144
- AndersSHuberWDifferential expression analysis for sequence count dataGenom Biol20101110R106
- HuangRLiaoXLiQIdentification and validation of potential prognostic gene biomarkers for predicting survival in patients with acute myeloid leukemiaOnco Targets Ther2017105243525429138577
- LiaoXZhuGHuangRIdentification of potential prognostic microRNA biomarkers for predicting survival in patients with hepatocellular carcinomaCancer Manag Res20181078780329713196
- AgarwalVBellGWNamJWBartelDPPredicting effective microRNA target sites in mammalian mRNAseLife20154
- LewisBPBurgeCBBartelDPConserved seed pairing, often flanked by adenosines, indicates that thousands of human genes are microRNA targetsCell20051201152015652477
- WangXImproving microRNA target prediction by modeling with unambiguously identified microRNA-target pairs from CLIP-ligation studiesBioinformatics20163291316132226743510
- WongNWangXmiRDB: an online resource for microRNA target prediction and functional annotationsNucleic Acids Res201543Database issueD146D15225378301
- ChouCHShresthaSYangCDmiRTarBase update 2018: a resource for experimentally validated microRNA-target interactionsNucleic Acids Res201846D1D296D30229126174
- HsuSDLinFMWuWYmiRTarBase: a database curates experimentally validated microRNA-target interactionsNucleic Acids Res201139Database issueD163D16921071411
- Huang daWShermanBTLempickiRASystematic and integrative analysis of large gene lists using DAVID bioinformatics resourcesNat Protoc200941445719131956
- Huang daWShermanBTLempickiRABioinformatics enrichment tools: paths toward the comprehensive functional analysis of large gene listsNucleic Acids Res200937111319033363
- BenjaminiYHochbergYControlling the false discovery rate: a practical and powerful approach to multiple testingJ Royal Stat Society Series B1995571289300
- ReinerAYekutieliDBenjaminiYIdentifying differentially expressed genes using false discovery rate controlling proceduresBioinformatics200319336837512584122
- BenjaminiYDraiDElmerGKafkafiNGolaniIControlling the false discovery rate in behavior genetics researchBehav Brain Res20011251–227928411682119
- ZhouXHuangZXuLA panel of 13-miRNA signature as a potential biomarker for predicting survival in pancreatic cancerOncotarget2016743696166962427626307
- LiangLWeiDMLiJJPrognostic microRNAs and their potential molecular mechanism in pancreatic cancer: a study based on The Cancer Genome Atlas and bioinformatics investigationMol Med Reports2018171939951
- Mino-KenudsonMFernandez-del CastilloCBabaYPrognosis of invasive intraductal papillary mucinous neoplasm depends on histological and precursor epithelial subtypesGut201160121712172021508421
- StotzMEisnerFSzkanderaJClinico-pathological characteristics and clinical outcome of different histological types of pancreatic cancer in a large Middle European seriesJ Clin Pathol201366975375723750038
- CuillerierECellierCPalazzoLOutcome after surgical resection of intraductal papillary and mucinous tumors of the pancreasAm J Gastroenterol200095244144510685747
- LingQXuXYePThe prognostic relevance of primary tumor location in patients undergoing resection for pancreatic ductal adenocarcinomaOncotarget201789151591516728122349
- HuangDHWangGYZhangJWLiYZengXCJiangNMiR-501-5p regulates CYLD expression and promotes cell proliferation in human hepatocellular carcinomaJpn J Clin Oncol201545873874425917358
- FanDRenBYangXLiuJZhangZUpregulation of miR-501-5p activates the wnt/beta-catenin signaling pathway and enhances stem cell-like phenotype in gastric cancerJ Exp Clin Cancer Res201635117727846906
- SandMSkryganMSandDComparative microarray analysis of microRNA expression profiles in primary cutaneous malignant melanoma, cutaneous malignant melanoma metastases, and benign melanocytic neviCell Tissue Res20133511859823111773
- FengSDMaoZLiuCSimultaneous overexpression of miR-126 and miR-34a induces a superior antitumor efficacy in pancreatic adenocarcinomaOnco Targets Ther2017105591560429200874
- LiuSGQinXGZhaoBSDifferential expression of miRNAs in esophageal cancer tissueOncol Lett2013551639164223761828
- QianYWangXLvZMicroRNA126 is downregulated in thyroid cancer cells, and regulates proliferation, migration and invasion by targeting CXCR4Mol Med Rep201614145345927175968
- ZhangGMLuoLDingXMMicroRNA-126 inhibits tumor cell invasion and metastasis by downregulating ROCK1 in renal cell carcinomaMol Med Rep20161365029203627108693
- DebernardiSMassatNJRadonTPNoninvasive urinary miRNA biomarkers for early detection of pancreatic adenocarcinomaAm J Cancer Res20155113455346626807325
- BhattacharyaSSteeleRShrivastavaSChakrabortySDi BisceglieAMRayRBSerum miR-30e and miR-223 as novel noninvasive biomarkers for hepatocellular carcinomaAm J Pathol2016186224224726776075
- SilvaJGarciaVZaballosAVesicle-related microRNAs in plasma of nonsmall cell lung cancer patients and correlation with survivalEur Respir J201137361762320595154
- MarkouASourvinouIVorkasPAYousefGMLianidouEClinical evaluation of microRNA expression profiling in non small cell lung cancerLung Cancer201381338839623756108
- HuYCorreaAMHoqueAPrognostic significance of differentially expressed miRNAs in esophageal cancerInt J Cancer2011128113214320309880
- LeeHParkCSDeftereosGMicroRNA expression in ovarian carcinoma and its correlation with clinicopathological featuresWorld J Surg Oncol20121017422925189
- D’AiutoFCallariMDugoMmiR-30e* is an independent subtype-specific prognostic marker in breast cancerBr J Cancer2015113229029826057454
- KoYCFangWHLinTCMicroRNA let-7a-3 gene methylation is associated with karyotyping, CEBPA promoter methylation, and survival in acute myeloid leukemiaLeuk Res201438562563124703161
- LuLKatsarosDZhuYLet-7a regulation of insulin-like growth factors in breast cancerBreast Cancer Res Treat2011126368769420848182
- LuLKatsarosDde la LongraisIASochircaOYuHHypermethylation of let-7a-3 in epithelial ovarian cancer is associated with low insulin-like growth factor-II expression and favorable prognosisCancer Res20076721101171012217974952
- LiYLinJYangJOverexpressed let-7a-3 is associated with poor outcome in acute myeloid leukemiaLeuk Res201337121642164724138945
- JiangZGuoJXiaoBIncreased expression of miR-421 in human gastric carcinoma and its clinical associationJ Gastroenterol2010451172319802518
- GeXLiuXLinFMicroRNA-421 regulated by HIF-1alpha promotes metastasis, inhibits apoptosis, and induces cisplatin resistance by targeting E-cadherin and caspase-3 in gastric cancerOncotarget2016717244662448227016414
- ZhaoGXuLHuiLZhaoJLevel of circulated microRNA-421 in gastric carcinoma and related mechanismsInt J Clin Exp Pathol2015811142521425626823741
- ZhangYGongWDaiSDownregulation of human farnesoid X receptor by miR-421 promotes proliferation and migration of hepatocellular carcinoma cellsMol Cancer Res201210451652222446874
- LuMKongXWangHHuangGYeCHeZA novel microRNAs expression signature for hepatocellular carcinoma diagnosis and prognosisOncotarget2017858775878428060739
- HaoJZhangSZhouYLiuCHuXShaoCMicroRNA 421 suppresses DPC4/Smad4 in pancreatic cancerBiochem Biophys Res Commun2011406455255721352803
- ZhongXYYuJHZhangWGMicroRNA-421 functions as an oncogenic miRNA in biliary tract cancer through down-regulating farnesoid X receptor expressionGene20124931445122146319
- ZhouSWangBHuJmiR-421 is a diagnostic and prognostic marker in patients with osteosarcomaTumour Biol20163779001900726758431
- ChenLTangYWangJYanZXuRmiR-421 induces cell proliferation and apoptosis resistance in human nasopharyngeal carcinoma via downregulation of FOXO4Biochem Biophys Res Commun2013435474575023707940
- LiuHGaoYSongDLiuTFengYCorrelation between microRNA-421 expression level and prognosis of gastric cancerInt J Clin Exp Pathol2015811151281513226823855
- MengDYangSWanXA transcriptional target of androgen receptor, miR-421 regulates proliferation and metabolism of prostate cancer cellsInt J Biochem Cell Biol201673304026827675
- Champeris TsanirasSKanellakisNSymeonidouIENikolopoulouPLygerouZTaravirasSLicensing of DNA replication, cancer, pluripotency and differentiation: an interlinked world?Semin Cell Dev Biol20143017418024641889
- RoySKSrivastavaRKShankarSInhibition of PI3K/AKT and MAPK/ERK pathways causes activation of FOXO transcription factor, leading to cell cycle arrest and apoptosis in pancreatic cancerJ Mol Signal201051020642839
- CollinsMAYanWSebolt-LeopoldJSPasca di MaglianoMMAPK signaling is required for dedifferentiation of acinar cells and development of pancreatic intraepithelial neoplasia in miceGastroenterology20141463822834.e82724315826
- KopperFBinkowskiAMBierwirthCDobbelsteinMThe MAPK-activated protein kinase 2 mediates gemcitabine sensitivity in pancreatic cancer cellsCell Cycle201413688488924556918
- GhasemiRRapposelliIGCaponeEDual targeting of ErbB-2/ErbB-3 results in enhanced antitumor activity in preclinical models of pancreatic cancerOncogenesis20143e11725133484
- GrimontAPinhoAVCowleyMJSOX9 regulates ERBB signalling in pancreatic cancer developmentGut201564111790179925336113
- DoiTIshikawaTOkayamaTThe JAK/STAT pathway is involved in the upregulation of PD-L1 expression in pancreatic cancer cell linesOncol Rep20173731545155428112370
- ChenLZhouDLiuZCombination of gemcitabine and erlotinib inhibits recurrent pancreatic cancer growth in mice via the JAK-STAT pathwayOncol Rep20183931081108929328487