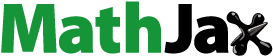
Abstract
Introduction
Chemokines are closely related with tumor immunity, progression, and metastasis. We aimed to construct a multi-RNA classifier of chemokine family genes for predicting tumor recurrence in stage I–III patients with colorectal cancer (CRC) after operation.
Patients and methods
By analyzing microarray data, the Cox regression analysis was conducted to determine survival-related chemokine family genes and develop a multi-RNA classifier in the training set. The prognostic value of this multi-RNA classifier was further validated in the internal validation and external independent sets. Receiver operating characteristic curves were used to compare the prediction ability of the combined model of this multi-RNA classifier and stage, and this multi-RNA classifier and stage alone.
Results
Nine survival-related chemokines were identified in the training set. We identified a nine-chemokine classifier and classified the patients as high-risk or low-risk. Compared with CRC patients with high-risk scores, CRC patients with low-risk scores had longer disease-free survival in the training (HR=2.353, 95% CI=1.480–3.742, P<0.001), internal validation (HR=2.389, 95% CI=1.428–3.996, P<0.001), and external independent (HR=3.244, 95% CI=1.813–5.807, P<0.001) sets. This nine-chemokine classifier was an independent prognostic factor in these datasets (P<0.05). The combined model of this nine-chemokine classifier and tumor stage may tend to have higher accuracy than stage alone in the training (area under curve 0.727 vs 0.626, P<0.01), internal validation (0.668 vs 0.584, P=0.03), and external independent (0.704 vs 0.678, P>0.05) sets. This nine-chemokine classifier may only be applied in Marisa’s C2, C5, and C6 subtypes patients.
Conclusion
Our nine-chemokine classifier is a reliable prognostic tool for some specific biological subtypes of CRC patients. It might contribute to guide the personalized treatment for high-risk patients.
Introduction
CRC is the third most common malignancy and the fourth leading cause of mortality worldwide.Citation1 Risk stratification of CRC may require the combined multiple approaches, including analysis of molecular biomarkers and clinical data, and thorough experimental studies. In the past two decades, study results have confirmed that tumor is a heterogeneity disease.Citation2 The combined model of multiple clinical and molecular parameters could be a much more objective prognostic tool for CRC patients.
Chemokines are a group of small molecular weight proteins that bind to the G protein-coupled chemokine receptors.Citation3 Chemokines and their receptors are divided into four subfamilies (CXC, CC, CX3C, and C) based on the pattern of cysteine residues, where C represents the cysteine and X represents noncysteine amino acids.Citation4,Citation5 Chemokine family genes are closely related with senescence, angiogenesis, epithelial mesenchymal transition, proliferation, immune evasion, and tumor metastasis.Citation6 The altered expression of chemokines and their receptors is found in many malignancies and may lead to aberrant chemokine receptor signaling.Citation7–Citation20 The chemokine ligand–receptor interactions were closely related with tumor immunity, progression, and metastasis in CRC patients, including the CXCL1– CXCR2,Citation7 CXCL9/10–CXCR3,Citation8,Citation9 CXCL12–CXCR4,Citation10–Citation14 CCL2–CCR2,Citation15,Citation16 CCL5–CCR5,Citation17 CCL15–CCR1,Citation18 CCL20– CCR6,Citation19 and CX3CL1–CX3CR1Citation20 axes. Although small sample studies showed that some chemokines could be used as the single biomarker for CRC, the values of these genes were not further validated in other validation sets.Citation8,Citation11,Citation13,Citation16,Citation21–Citation23 Until now, there is no multi-RNA classifier of chemokine family genes for predicting tumor recurrence in CRC patients. As a widely used microarray platform, Affymetrix human genome U133 plus 2.0 array included all probes of human genome U133 set and about 6,500 new genes.Citation24 Almost all the mRNA-level data of chemokine family genes could be obtained from this microarray platform. Laetitia Marisa defined six molecular subtypes for CRC based on the main biological characteristics, including one subtype with downregulated immune pathways (C1), one deficient mismatch repair subtype (C2), one KRAS mutant subtype (C3), one cancer stem cell subtype (C4), one subtype with the upregulated Wnt pathway (C5), and one subtype with a normal-like gene expression profile (C6).Citation25 Therefore, we performed this study to develop and validate a multi-RNA prognostic classifier of chemokine family genes for predicting recurrence in stage I–III patients with CRC by analyzing the microarray data. These multi-RNA signatures were further validated in CRC patients with different Marisa’s biological subtypes (C1–C6).Citation25
Materials and methods
Datasets preparation
Gene expression of chemokine family genes and corresponding clinical data were obtained from the GEO database. All log2-transformed expression data of chemokines and their receptors were obtained from Affymetrix human genome U133 plus 2.0 array (GSE39582 and GSE14333). The expression values of genes with multiple probes were calculated by using the median values of multiple probes. After filtering out CRC patients without DFS and clinical data, there were a total of 718 stage I–III patients with CRC, including 492 from GSE39582 and 226 from GSE14333. The 492 patients from GSE39582 were randomly divided into training (n=246, GSE39582) and internal validation (n=246, GSE39582) sets. The 226 patients from GSE14333 were used for the external independent set.
Statistical analysis
The survival analysis was performed by the “survival” package of R software (version 3.4.3). In the training set, the association between the expression level of chemokine family genes and CRC patients’ DFS was evaluated using a univariate Cox regression analysis. Those chemokine family genes were considered to be significant if their P-values were less than 0.05. Then, the selected chemokine family genes were fitted in a multivariate Cox regression analysis in the training set. Risk scores were calculated by the selected chemokine family genes and their regression coefficients in the multivariate Cox regression analysis,Citation26–Citation28 as follows:
Functional enrichment analysis
To evaluate the functional implication of these nine chemokines, functional enrichment analyses for GO and KEGG category were performed with the GeneCodis web tool (http://genecodis.cnb.csic.es/).Citation29–Citation31 GO and KEGG category enrichments were based on the threshold of P-value <0.05. Significant enrichment results were visualized using R software (version 3.4.3).
Results
Identification of survival-related chemokines in the training set
shows the study flow for the development and validation of the nine-chemokine classifier. By using microarray data, we identified 59 chemokine family genes from GSE39582 and GSE14333. We further analyzed these 59 genes by the univariate Cox regression analysis in the training set (n=246, GSE39582; ). Consequently, we identified nine chemokines that were significantly correlated with DFS in CRC patients (shown in ). The positive coefficients for three chemokines (CCL1, CCL14, and CXCL14) indicated that their higher levels of expression were associated with worse prognosis. The negative coefficients for the remaining six genes (CXCL1, CXCL3, CXCL9, CXCL10, CXCL11, and CXCL13) indicated that their higher levels of expression were associated with better prognosis.
Table 1 The characteristics of nine chemokines associated with DFS in the training set of 246 CRC patients (n=246, GSE39582)
Survival comparisons between low-risk and high-risk groups in the training, internal validation, and external independent sets
According to these chemokines and their regression coefficients in the multivariate Cox model, we calculated the risk scores for every patient in the training (n=246, GSE39582), internal validation (n=246, GSE39582), and external independent (n=226, GSE14333) sets. Using the cutoff value of risk scores (1.559), CRC patients were classified into low-risk group and high-risk group for the training (low-risk/high-risk: 185/61), internal validation (low-risk/high-risk: 196/50), and external independent (low-risk/high-risk: 185/41) sets. shows the distributions of this risk score and the DFS status in these three sets. As shown in , CRC patients with high-risk scores tended to have higher risk of treatment relapse.
Figure 2 The distributions of risk score and DFS status in the training (n=246, GSE39582), internal validation (n=246, GSE39582), and external independent (n=226, GSE14333) sets.
Abbreviation: DFS, disease-free survival.
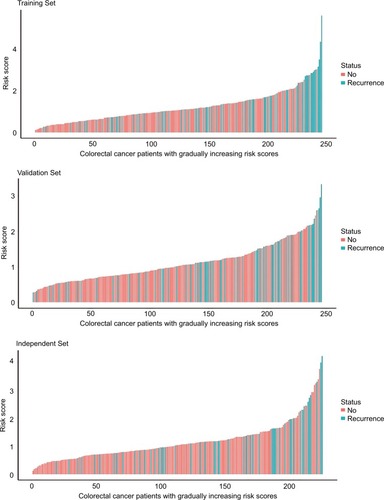
The clinical characteristics of low-risk group and high-risk group patients with CRC in these three sets are shown in . There were no differences for clinical characteristics (age, gender, stage, and adjuvant chemotherapy) between low-risk group and high-risk group (all P>0.05).
Table 2 Clinical characteristics of CRC patients according to the nine-chemokine classifier in the training (n=246, GSE39582), internal validation (n=246, GSE39582), and external independent (n=226, GSE14333) sets
and show the DFS differences between high-risk and low risk groups in these three sets. The log-rank test showed that CRC patients with low-risk scores had significantly longer DFS than those with high-risk scores in the training set (HR=2.353, 95% CI=1.480–3.742, P<0.001) and internal validation (HR=2.389, 95% CI=1.428–3.996, P<0.001), and external independent (HR=3.244, 95% CI=1.813–5.807, P<0.001) sets.
Table 3 Log-rank test of disease-free survival according to the nine-chemokine classifier in the training (n=246, GSE39582), internal validation (n=246, GSE39582), and external independent (n=226, GSE14333) sets
Multivariate Cox regression analysis in the training, internal validation, and external independent sets
shows the multivariate Cox regression analysis results of the nine-chemokine classifier, gender, age, stage, adjuvant chemotherapy, and DFS in the training, internal validation, and external independent sets. Both the nine-chemokine classifier and stages were significantly associated with CRC patients’ DFS in these datasets (all P<0.05).
Table 4 Multivariate Cox regression analysis of the nine-chemokine classifier, gender, age, stage, adjuvant chemotherapy, and disease-free survival in the training (n=246, GSE39582), internal validation (n=246, GSE39582), and external independent (n=226, GSE14333) sets
ROC analysis in the training, internal validation, and external independent sets
shows the ROC curves for predicting DFS in the training, internal validation, and external independent sets. AUC of the nine-chemokine classifier is similar with that of stage alone in the training (0.673 vs 0.626, P=0.343), internal validation (0.651 vs 0.584, P=0.332), and external independent (0.609 vs 0.678, P=0.888) sets. AUC of the combined model (nine-chemokine classifier and tumor stage) may tend to be higher than that of stage alone in the training (0.727 vs 0.626, P=0.001), internal validation (0.668 vs 0.584, P=0.030), and external independent (0.704 vs 0.678, P=0.298) sets.
Figure 4 Receiver operating characteristics curves of the combined model of the nine-chemokine classifier and stage, the nine-chemokine classifier and stage alone for predicting disease-free survival in the training (n=246, GSE39582), internal validation (n=246, GSE39582), and external independent (n=226, GSE14333) sets.
Abbreviation: AUC, area under the curve.
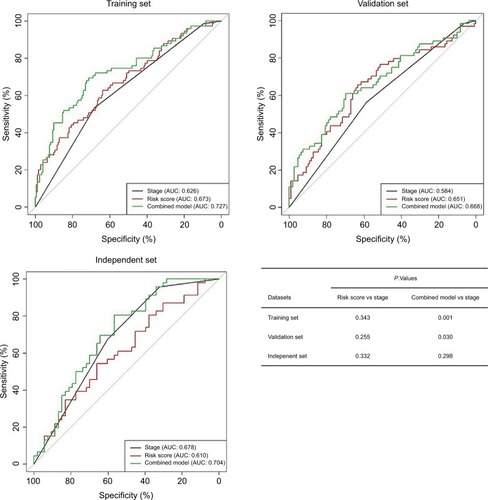
Survival analysis between low-risk and high-risk groups in the combined training and validation set
Using the same cutoff point (1.559), CCR patients were categorized into low-risk group (n=381) and high-risk group (n=111) in the combined training and validation set. CRC patients with low-risk scores had significantly longer DFS (HR=2.383, 95% CI=1.690–3.359, P<0.001) and OS (HR=1.603, 95% CI=1.115–2.305, P=0.010) than those with high-risk scores (shown in ). shows the multivariate Cox regression analysis results of the nine-chemokine classifier, gender, age, stage, adjuvant chemotherapy, and survival in the combined training and validation set (n=492, GSE39582). The multivariate Cox analysis showed that the nine-chemokine classifier was significantly associated with patients’ DFS (HR=2.292, 95% CI=1.622–3.239, P<0.001) and OS (HR=1.640, 95% CI=1.139–2.362, P=0.008; ). Subgroup analysis showed that stage II–III patients with low-risk scores had significantly longer DFS (P<0.05) than those with high-risk scores (shown in ).
Table 5 Comparison of the survival of colorectal cancer patients according to the nine-chemokine classifier in the combined training and validation set (n=492, GSE39582)
Table 6 Multivariate Cox regression analysis of the nine-chemokine classifier, gender, age, stage, adjuvant chemotherapy, and survival in the combined training and validation set (n=492, GSE39582)
The prediction values of the nine-chemokine classifier for different biological subtypes in the combined training and validation set
To verify the value of the nine-chemokine classifier, we further validated our findings in different molecular subtypes of Laetitia Marisa in the combined training and validation set (n=460, GSE39582).
We further validated the values of the nine-chemokine classifier for different biological subtypes in the combined training and validation set (n=492, GSE39582). Patients with high-risk scores had similar DFS (all P>0.05) with those with low-risk scores in the subtypes C1 (one subtype with downregulated immune pathways) and C3 (one KRAS mutant subtype) (shown in ). Patients with high-risk scores had significantly shorter DFS (all P>0.05) than those with low-risk scores in the subtypes C2 (one deficient mismatch repair subtype), C4 (one cancer stem cell subtype), C5 (one subtype with the upregulated Wnt pathway), and C6 (one subtype with a normal-like gene expression profile) (shown in ). But the Cox regression analysis showed that the integrated lncRNA-mRNA classifier was not significantly associated with the subtypes C1, C3, and C4 patients’ DFS (all P≥0.05). The integrated lncRNA-mRNA classifier was an independent prognostic factor for the subtypes C2, C5, and C6 patients’ DFS (all P<0.05).
Functional enrichment analysis
To explore the functional implication of nine chemokines, we performed functional category enrichment analysis. Functional enrichment analysis showed that the nine chemokine family genes were significantly enriched in 55 GO terms and 9 KEGG pathways (shown in ). The functional categories are mainly involved in eight GO terms, including immune response (GO:0006955), inflammatory response (GO:0006954), signal transduction (GO:0007165), chemotaxis (GO:0006935), cell–cell signaling (GO:0007267), extracellular space (GO:0005615), extracellular region (GO:0005576), and chemokine activity (GO:0008009). The mainly involved KEGG pathways included cytokine–cytokine receptor interaction (KEGG:04060), chemokine signaling pathway (KEGG:04062), and toll-like receptor signaling pathway (KEGG:04620).
Discussion
Although the TNM staging system is widely used as the risk stratification of CRC patients, it is insufficient in the prediction of prognosis and estimation for some patients.Citation32,Citation33 Conflict clinical outcomes may exist among some CRC patients with the same stage.Citation31,Citation32 To date, there are no clinically utilized prognostic biomarkers in CRC patients. An increasing amount of evidence demonstrates that chemokines and their receptors play an important role in tumor immunity, progression, and metastasis of CRC patients.Citation7–Citation20 The discovery and application of a multiple-chemokine biomarker will promote the evaluation and identification of potential high-risky recurrence in CRC patients. To identify the prognostic chemokines, we profiled chemokines by mining the existing microarray data of Affymetrix human genome U133 plus 2.0 array. We applied a univariate Cox regression analysis to select DFS-related chemokines. Based on this data-mining method, we have developed and validated a nine-chemokine classifier. The utility of this nine-chemokine classifier may add to the prognostic value of the TNM stage system. Furthermore, the clinical application of this nine-chemokine classifier might stratify CRC patients with the same stage into low-risk and high-risk groups of recurrence after operation. CRC patients of high-risk group had shorter DFS than those of low-risk group in stage II and III patients. The nine-chemokine classifier may provide an additional biomarker for identifying potential candidates for aggressive treatment strategies. The molecular subtype should be considered before the clinical application of the prognostic signatures. Subgroup showed that the nine-chemokine classifier was not an independent prognostic factor for DFS in Marisa’s C1, C3, and C4 subtypes patients. Therefore, the nine-chemokine classifier may only be applied in Marisa’s C2, C5, and C6 subtypes patients.
The nine-chemokine classifier included three risky genes (CCL1, CCL14, and CXCL14) and six protective genes (CXCL1, CXCL3, CXCL9, CXCL10, CXCL11, and CXCL13). The previous study showed that high tissue levels of CXCL14 was associated with increased risk of recurrence and mortality among CRC patients.Citation34 However, high expressions of CXCL1,CXCL9, CXCL10, and CXCL13 may be correlated with better prognosis of CRC patients.Citation23,Citation35–Citation37 Moreover, the prognostic values of these five chemokines were not further confirmed in another validation sets. The relationship of the remaining four chemokines (CCL1, CCL14, CXCL3, CXCL11) and CRC patients’ prognosis should be further studied.
Although our study possessed the larger sample size for developing and validating this nine-chemokine classifier, we should acknowledge certain potential limitations. First, preliminary functional enrichment analysis indicated that the nine-chemokine classifier was mainly involved in immune response, inflammatory response, signal transduction, chemotaxis, and cell–cell signaling. But the mechanism of these nine chemokines has not been confirmed through experimental studies. Further experimental studies may provide potential therapeutic targets for CRC patients. Second, Affymetrix human genome U133 plus 2.0 array was used for obtaining the mRNA level data of chemokine family genes in this study. But the mRNA level data of some chemokines (CCL6, CCL12, CXCL7, and CXCL15) were not available in this microarray platform. The link between the mRNA levels of these chemokines and survival of CRC patients should be further investigated by experimental studies. Third, this multi-RNA classifier was only developed and validated by the mRNA-level data. The value of this multi-RNA classifier was not further confirmed by the protein-level data. Moreover, future analysis of protein level on additional independent datasets would contribute to determine the potential importance of population and geographical differences.
In conclusion, we performed a comprehensive analysis of chemokine expression levels and corresponding survival information of CRC patients. We have successfully developed and validated a nine-chemokine classifier that may be a useful prognostic biomarker for the personalized treatment. It was the first study demonstrating a link between a multiple-chemokine classifier and tumor recurrence in CRC patients. This nine-chemokine classifier may provide an effective risk stratification of disease-free survival in CRC patients with the same stage, especially for stage II–III patients. But we should acknowledge that the protein-level data of this nine-chemokine classifier should be further validated before its clinical application.
Abbreviations
AUC | = | area under the curve |
CRC | = | colorectal cancer |
DFS | = | disease-free survival |
GEO | = | Gene Expression Omnibus |
GO | = | Gene Ontology |
KEGG | = | Kyoto Encyclopedia of Genes and Genomes OS, overall survival |
ROC | = | receiver operating characteristics |
Acknowledgments
The funders had no role in study design, data collection and analysis, decision to publish, or preparation of the manuscript.
Supplementary material
Table S1 Univariate Cox regression analysis of chemokine family genes associated with disease-free survival in the training set (n=246, GSE39582)
Disclosure
The authors report no conflicts of interest in this work.
References
- SiegelRLMillerKDJemalACancer statistics, 2016CA Cancer J Clin201666173026742998
- AlizadehAAArandaVBardelliAToward understanding and exploiting tumor heterogeneityNat Med201521884685326248267
- RamanDSobolik-DelmaireTRichmondAChemokines in health and diseaseExp Cell Res2011317557558921223965
- ZlotnikAYoshieOChemokines: a new classification system and their role in immunityImmunity200012212112710714678
- MurphyPMBaggioliniMCharoIFInternational union of pharmacology. XXII. Nomenclature for chemokine receptorsPharmacol Rev2000521145176
- SarvaiyaPJGuoDUlasovIGabikianPLesniakMSChemokines in tumor progression and metastasisOncotarget20134122171218524259307
- YamamotoMKikuchiHOhtaMTSU68 prevents liver metastasis of colon cancer xenografts by modulating the premetastatic nicheCancer Res200868239754976219047154
- KawadaKHosogiHSonoshitaMChemokine receptor CXCR3 promotes colon cancer metastasis to lymph nodesOncogene200726324679468817297455
- MurakamiTKawadaKIwamotoMThe role of CXCR3 and CXCR4 in colorectal cancer metastasisInt J Cancer2013132227628722689289
- KimJTakeuchiHLamSTChemokine receptor CXCR4 expression in colorectal cancer patients increases the risk for recurrence and for poor survivalJ Clin Oncol200523122744275315837989
- OttaianoAFrancoRAiello TalamancaAOverexpression of both CXC chemokine receptor 4 and vascular endothelial growth factor proteins predicts early distant relapse in stage II-III colorectal cancer patientsClin Cancer Res20061292795280316675573
- SchimanskiCCSchwaldSSimiantonakiNEffect of chemokine receptors CXCR4 and CCR7 on the metastatic behavior of human colorectal cancerClin Cancer Res20051151743175015755995
- YoppACShiaJButteJMCXCR4 expression predicts patient outcome and recurrence patterns after hepatic resection for colorectal liver metastasesAnn Surg Oncol201219Suppl 3339346
- MatsusueRKuboHHisamoriSHepatic stellate cells promote liver metastasis of colon cancer cells by the action of SDF-1/CXCR4 axisAnn Surg Oncol20091692645265319588204
- BaileyCNegusRMorrisAChemokine expression is associated with the accumulation of tumour associated macrophages (TAMs) and progression in human colorectal cancerClin Exp Metastasis200724212113017390111
- HuHSunLGuoCTumor cell-microenvironment interaction models coupled with clinical validation reveal CCL2 and SNCG as two predictors of colorectal cancer hepatic metastasisClin Cancer Res200915175485549319706805
- ChangLYLinYCMahalingamJTumor-derived chemokine CCL5 enhances TGF-β-mediated killing of CD8(+) T cells in colon cancer by T-regulatory cellsCancer Res20127251092110222282655
- ItataniYKawadaKFujishitaTLoss of SMAD4 from colorectal cancer cells promotes CCL15 expression to recruit CCR1+ myeloid cells and facilitate liver metastasisGastroenterology201314551064107523891973
- LiuJZhangNLiQTumor-associated macrophages recruit CCR6+ regulatory T cells and promote the development of colorectal cancer via enhancing CCL20 production in micePLoS One201164e1949521559338
- ZhengJYangMShaoJChemokine receptor CX3CR1 contributes to macrophage survival in tumor metastasisMol Cancer201312114124245985
- ZengYJLaiWWuHNeuroendocrine-like cells -derived CXCL10 and CXCL11 induce the infiltration of tumor-associated macrophage leading to the poor prognosis of colorectal cancerOncotarget2016719273942740727034164
- StanisavljevićLAßmusJStorliKECXCR4, CXCL12 and the relative CXCL12-CXCR4 expression as prognostic factors in colon cancerTumour Biol20163767441745226678887
- JiangZXuYCaiSCXCL10 expression and prognostic significance in stage II and III colorectal cancerMol Biol Rep20103763029303619821051
- ZhangYSzustakowskiJSchinkeMBioinformatics analysis of micro-array dataMethods Mol Biol200957325928419763933
- MarisaLde ReynièsADuvalAGene expression classification of colon cancer into molecular subtypes: characterization, validation, and prognostic valuePLoS Med2013105e100145323700391
- LossosISCzerwinskiDKAlizadehAAPrediction of survival in diffuse large-B-cell lymphoma based on the expression of six genesN Engl J Med2004350181828183715115829
- HuZChenXZhaoYSerum microRNA signatures identified in a genome-wide serum microRNA expression profiling predict survival of non-small-cell lung cancerJ Clin Oncol201028101721172620194856
- KangJD’AndreaADKozonoDA DNA repair pathway-focused score for prediction of outcomes in ovarian cancer treated with platinum-based chemotherapyJ Natl Cancer Inst2012104967068122505474
- Tabas-MadridDNogales-CadenasRPascual-MontanoAGene-Codis3: a non-redundant and modular enrichment analysis tool for functional genomicsNucleic Acids Res201240Web Server issueW478W48322573175
- Nogales-CadenasRCarmona-SaezPVazquezMGeneCodis: interpreting gene lists through enrichment analysis and integration of diverse biological informationNucleic Acids Res200937Web Server issueW317W32219465387
- Carmona-SaezPChagoyenMTiradoFCarazoJMPascual-MontanoAGENECODIS: a web-based tool for finding significant concurrent annotations in gene listsGenome Biol200781R317204154
- ZinicolaRPedrazziGHaboubiNNichollsRJThe degree of extramural spread of T3 rectal cancer: a plea to the UICC and AJCCColorectal Dis201719331027418312
- LiJYangSHuJTumor deposits counted as positive lymph nodes in TNM staging for advanced colorectal cancer: a retrospective multicenter studyOncotarget2016714182691827926934317
- ZengJYangXChengLChemokine CXCL14 is associated with prognosis in patients with colorectal carcinoma after curative resectionJ Transl Med201311623294544
- Le RolleAFChiuTKFaraMThe prognostic significance of CXCL1 hypersecretion by human colorectal cancer epithelia and myofibroblastsJ Transl Med20151319926104296
- WuZHuangXHanXThe chemokine CXCL9 expression is associated with better prognosis for colorectal carcinoma patientsBiomed Pharmacother20167881326898419
- AgesenTHSveenAMerokMAColoGuideEx: a robust gene classifier specific for stage II colorectal cancer prognosisGut201261111560156722213796