Abstract
Objective
This study aimed to develop and validate a machine learning model for predicting bone metastases (BM) in prostate cancer (PCa) patients.
Methods
Demographic and clinicopathologic variables of PCa patients in the Surveillance, Epidemiology and End Results (SEER) database from 2010 to 2017 were retrospectively analyzed. We used six different machine learning algorithms, including Decision tree (DT), Random forest (RF), Multilayer Perceptron (MLP), Logistic regression (LR), Naive Bayes classifiers (NBC), and eXtreme gradient boosting (XGB), to build prediction models. External validation using data from 644 PCa patients of the First Affiliated Hospital of Nanchang University from 2010 to 2016. The performance of the models was evaluated using the area under receiver operating characteristic curve (AUC), accuracy score, sensitivity (recall rate) and specificity. A web predictor was developed based on the best performance model.
Results
A total of 207,137 PCa patients from SEER were included in this study. Of whom, 6725 (3.25%) developed BM. Gleason score, Prostate-specific antigen (PSA) value, T, N stage and age were found to be the risk factors of BM. The XGB model offered the best predictive performance among these 6 models (AUC: 0.962, accuracy: 0.884, sensitivity (recall rate): 0.906, and specificity: 0.879). An XGB model-based web predictor was developed to predict BM in PCa patients.
Conclusion
This study developed a machine learning model and a web predictor for predicting the risk of BM in PCa patients, which may help physicians make personalized clinical decisions and treatment strategy for patients.
Introduction
Prostate cancer (PCa) is the most common non-skin cancer among men globally, with approximately 1.6 million cases and 366,000 deaths reported each year.Citation1,Citation2 Metastatic prostate cancer has important clinical implications, and metastatic disease may occur on the occasion of the initial clinical diagnosis.Citation3,Citation4 Bone metastases (BM) take up a great proportion of patients with metastases, accounting for approximately 16.7% of all metastatic cases, and these patients had significantly reduced 5-year survival and quality of life.Citation5,Citation6
Recently, prostate-specific membrane antigen (PSMA) ligands have presented good results in the diagnosis and treatment of BM from PCa.Citation7 However, this method is still at the stage of clinical trial and is not acceptable for most patients due to the radiation injury and the high cost.Citation8,Citation9 The current method for detecting BM in PCa patients is mainly bone scan. But it just suggested for patients with suspected skeletal-related events (SRE) due to the severe radiation injury. In addition, the median time to SRE has been reported to be 5 months after bone metastases.Citation10 An assisted decision-making system that can help determining which cancer patients should receive bone scan will provide help for addressing these issues. And with the development of precision medicine, the diagnosis and treatment of cancer should be individualized. The Surveillance, Epidemiology and End Results (SEER) database is a publicly obtainable, federally funded cancer reporting system that brings raw material to our insights into complex diseases.Citation11 With the increasing level of computer hardware, machine learning can facilitate the diagnosis of cancer metastasis by processing and analyzing large, heterogeneous and complex clinical data and building predictive models. And there are already studies that have been conducted in this area with good results.Citation12–Citation14
Therefore, in this study, we aim to build a prediction model to evaluate the risk of BM in PCa patients based on machine learning techniques, and develop a web-based predictor that can be easily manipulated by physicians and patients. This study may provide some help for clinicians to make personalized decisions for the treatment of patients with PCa BM.
Materials and Methods
Study Population
The study is carried out based on the SEER database. Patients’ data were obtained from the “SEER Research Plus Data, 18 Registries, Nov 2019 Sub (2000–2017)” and downloaded using SEER*stat 8.3.9 software. Patients who were diagnosed with PCa from 2010 to 2017 were included in this study. Exclusion criteria were as follows:Citation1 PCa was not the first tumor.Citation2 The information of race, grade, Prostate-specific antigen (PSA), Gleason score, T, N stage, metastatic status and marital status missed or unknown. Additionally, 644 PCa patients from the First Affiliated Hospital of Nanchang University from 2010 to 2016 were included for external validation. The case screening process is shown in .
Figure 1 Flow diagram of the study population selected from the Surveillance, Epidemiology, and End Results (SEER) database and the First Affiliated Hospital of Nanchang University. According to the inclusion and exclusion criteria, a total of 207,137 patients of SEER were included in this study, and they were randomly cut into the training and internal test sets in a 7:3 ratio. Data from the First Affiliated Hospital of Nanchang University as an external test set.
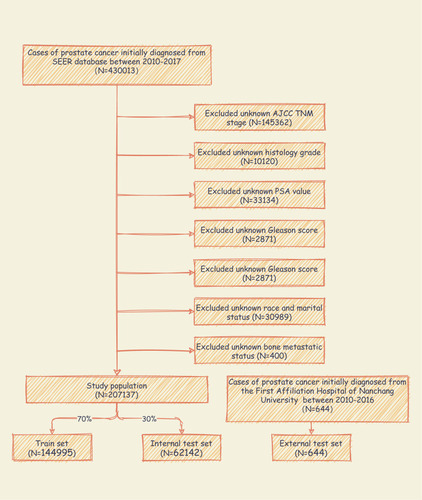
Data Selection
Eight variables from the SEER database that may affect BM in PCa patients were selected in this study, including age at diagnosis, race, grade, PSA value, Gleason score, T, N stage and marital status. Besides, we only included patients with PSA values between 0.1 and 98.0ng/mL. Because no specific values over 98.0 ng/mL were provided by the SEER database. All patients enrolled in this study were staged using the 7th edition of the AJCC TNM staging system and relevant guidelines of the SEER program.
Statistical Analyses and Model Establishment
All statistical analysis was performed in Python (version 3.8, Python Software Foundation) and SPSS (version 26, IBM, USA).Citation15 All machine learning algorithms were built based on scikit-learn (version 0.24.1). The patient data were randomly sliced into training and internal test sets in a ratio of 7:3 using python. Training set is used to build the model, and the internal test set is used for model validation and evaluation.
In order to determine the variables included in the machine learning model, we conducted a univariate analysis to compare these variables between patients with and without BM. The Chi-square test was utilized for categorical data, and the Wilcoxon rank-sum test was used for continuous non-normally distributed data. Variables with a P < 0.05 in univariate analysis were enclosed within the construction of machine learning models and multivariate logistic regression was performed to identify the risk factor for BM. Meanwhile, based on the Permutation Importance principle,Citation16 we performed feature importance analysis on the variables in each machine learning model. Correlation analysis was performed on the screened variables to test whether the variables would affect each other.
This study used six different machine learning algorithms to model the data, including Decision tree (DT), Random Forest (RF), Multilayer Perceptron (MLP), Logistic regression (LR), Naive Bayes classifiers (NBC) and eXtreme gradient boosting (XGB).Citation17–Citation22 The ML algorithms were trained by using Python software to predict the BM in PCa patients. Model parameter settings are detailed in the Supplementary Materials. Parameter settings link is accessible from https://share.streamlit.io/liuwencaincu/prostate-cancer/main/prostate.py. Then, the predictive power of the machine learning models was evaluated in internal ten-fold cross-validation of the train set, internal test set and external test set. The area under the receiver operating characteristic curve (AUC), the sensitivity (recall rate), specificity, and accuracy score were calculated. The best-performing model was selected to build a web predictor.
Results
Demographic Baseline Characteristics
A total of 207,137 PCa patients from SEER database were included in the study. Of whom, 6725 (3.25%) developed BM and 200,412 (96.75%) had no BM. All demographic and clinicopathological characteristics of these patients were demonstrated in detail in . All patients were randomly cut into a training set (n = 144,995) and an internal test set (n = 62,142) in a ratio of 7:3. External validation was conducted by using data of 644 PCa patients from the First Affiliated Hospital of Nanchang University. The details of the training and test sets are shown in .
Table 1 Clinical and Pathological Characteristics of Study Population
Table 2 Clinical and Pathological Characteristics of Training Set and Test Set
Univariate and Multivariate Logistic Regression Analysis
Based on the univariate analysis, age, race, grade, T, N stage, PSA value, Gleason score and marital status were significantly associated with the BM in PCa patients (P < 0.05) (). Variables with a P values <0.05 between these two groups were selected for multivariate logistic regression analysis. Based on the analysis, T, N stage, Gleason score and PSA value were found to be the independent risk factors for BM in PCa patients ().
Table 3 Univariate Analysis and Multivariate Logistic Regression Analysis of Variables
Variable Influence and Feature Importance on Prediction
Correlation tests were performed among different variables identified from the univariate analysis. The correlation heat map showed that variables were not significantly correlated with each other (), which indicated that they were mutually independent.
Figure 2 Results of correlation analysis between all variables. The heat map shows the correlation between the variables.
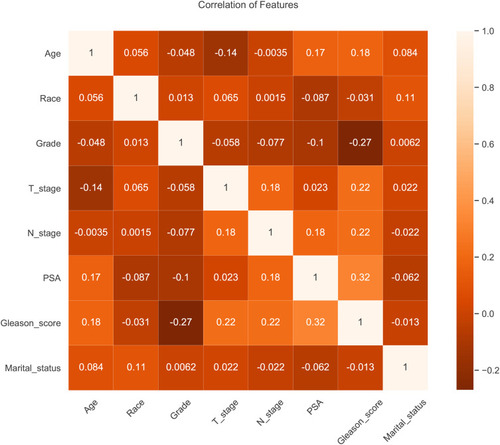
The importance of features in each machine learning model for predicting BM is shown in . Although the importance of features varied slightly among different machine learning algorithms, PSA value, Gleason score and N stage ranked at the top three of five models, which had similarities with the results of multivariate logistic analysis. In contrast, marital status ranked at the last place in most algorithms, but it also made some contributions to the models. In the XGB model, the importance of features were sorted in descending order by PSA value, Gleason score, N stage, T stage, age, grade, race and marital status.
Model Performance
The predictive performance of different models was compared using the internal ten-fold cross-validation of training set, internal and external test sets, which were detailed in – and . Among these models, the XGB model showed the best performance with an average AUC of 0.951 in the internal ten-fold cross-validation (). In the internal test set, the XGB model gained the best score with an AUC of 0.955, an accuracy of 0.881, a sensitivity (recall rate) of 0.905 and a specificity of 0.880. In the external test set, the XGB model also showed excellent performance with an AUC of 0.962, an accuracy of 0.884, a sensitivity (recall rate) of 0.906 and a specificity of 0.879 ( and and ). Meanwhile, the prediction results of different models were presented with a heat map in .
Table 4 Comparison Prediction Performances of Different Models for Bone Metastasis
Figure 4 Ten-fold cross-validation results of different machine learning models in the training set.
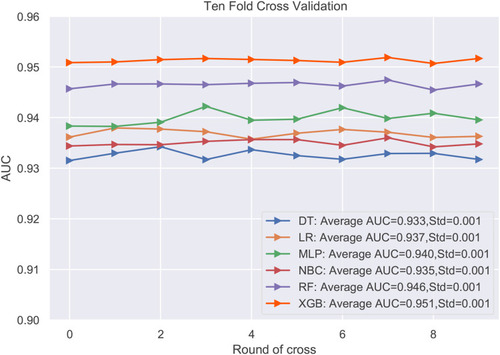
Figure 5 The roc curves of different machine learning models in internal test set and external test set.
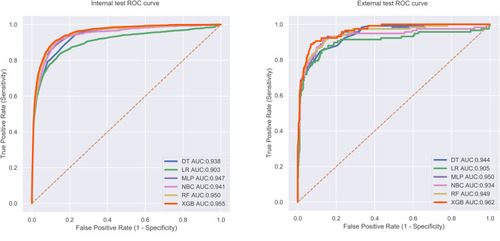
Figure 7 Prediction results of the different models. The heat map shows the predicted results of all models versus the actual situation in internal test set and external test set. Each column in the heat map represents the models’ predicted results of bone metastases for all patients in the dataset. Dark colors represent bone metastases cases and light colors are non-bone metastases.
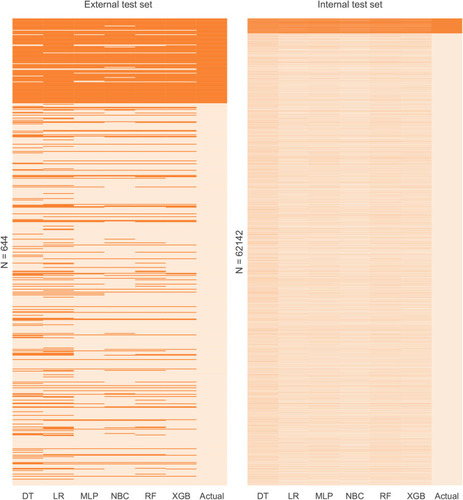
Web Predictor
A web predictor based on the best predictive performance of the XGB model was developed to predict BM in PCa patients. The risk of BM from PCa could be easily predicted by simply setting the variables in the sidebar of the web page (https://share.streamlit.io/liuwencaincu/prostate-cancer/main/prostate.py) ().
Discussion
Prostate cancer is the second leading cause of cancer-related death among men in the world. Bone-related events usually occur after bone metastases, which will result in reduced patient quality of life and survivorship.Citation23 The current diagnostic method for BM from PCa is bone scan and prostate-specific membrane antigen examination combined with nuclear imaging. However, there is radiation injury and high cost for the bone scan, and not all PCa patients are recommended for BM screening.Citation24–Citation26 Tissue biopsy is another method for the diagnosis. But it may increase the risk of further tumor invasion. Although skeletal-related events are considered to be a sign of BM, it would not be reasonable for screening BM from PCa because it may delay the treatment. Thus, it makes sense to develop a model to provide early attention and screening PCa patients at a high risk of BM. In this study, we built a predictive model using machine learning technologies to predict BM in PCa patients and identify patients at a high risk of BM.
With the development of the computer technique, machine learning technology has been widely used in different fields. And it also shows great promise for application in the biomedical science.Citation13,Citation27 Studies have already used machine learning technology to predict the development of diseases.Citation12,Citation28 In this study, several widely used machine learning algorithms were developed and validated to predict the risk of BM in PCa patients. After the comparison of algorithms with several evaluation indicators, the XGB algorithm-based prediction model showed the best performance among these models. The XGB model achieved better performance than others probably because it uses a number of strategies to prevent overfitting, exploits the second-order derivatives of the loss function and supports parallelization, and has a fast data processing speed.Citation29 These results can provide clinicians with more accurate prediction outcomes and help them to make personalized decisions for the treatment of PCa.
In the present study, four risk factors, including T, N stage, PSA and Gleason Score, were screened out of the pre-selected eight factors by univariate and multivariate analyses. They were in high agreement with the models’ feature importance ranking (, ). However, machine learning algorithms consider that other variables that are not statistically significant can also make some contribution to the prediction. This may be because the algorithms can make predictions by exploring intrinsic connections between data that cannot be discovered through traditional statistical methods.
Studies have found that PCa patients with high PSA values have a higher likelihood of developing BM. It is recommended that PCa patients with PSA >20ng/mL need a bone scan to check for BM.Citation30,Citation31 In this study, we also found that PSA values were important in predicting BM from PCa. It has been shown that advanced age and clinical stage were both correlated with the BM from PCa and poor prognosis.Citation32,Citation33 Chen et alCitation34 found that age greater than 70 years was the threshold for significantly higher risk of BM for PCa patients. However, Stolzenbach et alCitation35 found that age and race were not informative in predicting the progression of PCa metastasis, which was in line with the results of our study. Patients diagnosed at the T4 stage had the highest risk of BM. The same trend was seen in Grade. These results were consistent with the findings of Lu et al and Guo et al.Citation32,Citation36 Patients with regional lymphoma metastases tend to be more likely to develop BM than those with N0 stage.Citation37 The Gleason score also played an important role in the prediction model. The degree of BM in patients varies greatly with the change of Gleason score.Citation38 It was reported that Gleason score greater than 6 had a high specificity (88.9%) for the diagnosis of BM.Citation39 In addition, unmarried male patients had a higher risk of BM from PCa, which was in line with previous studies.Citation36,Citation40 Our model adequately incorporates various risk factors that may affect BM in PCa patients and achieves excellent predictive performance.
Based on machine learning algorithms and the huge amount of data in the SEER database, a model was constructed to predict BM in PCa patients and a web page predictor was developed. However, there are still some limitations in this study. First, the population in this study was obtained from the SEER database and externally validated using data just from a single center, which will be limitations for the application. Second, due to the inherent black-box properties of machine learning algorithms, it may pose some difficulties for the interpretation of the model. Third, the SEER database just reports the initial diagnostic information of PCa patients, and further therapeutic information is missing. We cannot access this information for further analysis.
In conclusion, we developed a prediction model to predict the risk of BM in PCa patients based on the XGB algorithm with machine learning techniques, and developed a web predictor in this study. And those at high risk of BM were recommended for further detailed screening based on the web predictor. This may help physicians to individualize the treatment of BM in patients with PCa.
Data Sharing Statement
The datasets generated and/or analyzed during the current study are available in the SEER database (https://seer.cancer.gov/).
Ethics Approval and Consent to Participate
We received permission to access the research data file in the SEER program from the National Cancer Institute, US. Approval was waived by the local ethics committee, as SEER data is publicly available and de-identified. This study was approved by the Ethics Committee of the First Affiliated Hospital of Nanchang University, and cases from the First Affiliated Hospital of Nanchang University signed written informed consent form. This study followed the guidelines outlined in the Declaration of Helsinki.
Disclosure
The authors report no conflicts of interest in this work.
Acknowledgments
This work is supported by the Department of Science and Technology Program of Jiangxi Province, China (No. 20202BBGL73015, 20203BBG73045) and the project of Jiangxi Provincial Health Commission (No. 20161024).
References
- Siegel RL, Miller KD, Jemal A. Cancer statistics, 2020. CA Cancer J Clin. 2020;70(1):7–30. doi:10.3322/caac.2159031912902
- Steele CB, Li J, Huang B, Weir HK. Prostate cancer survival in the United States by race and stage (2001‐2009): findings from the concord‐2 study. Cancer. 2017;123:5160–5177. doi:10.1002/cncr.3102629205313
- McDougall JA, Bansal A, Goulart BH, et al. The clinical and economic impacts of skeletal-related events among medicare enrollees with prostate cancer metastatic to bone. Oncologist. 2016;21(3):320. doi:10.1634/theoncologist.2015-032726865591
- Nørgaard M, Jensen AØ, Jacobsen JB, Cetin K, Fryzek JP, Sørensen HT. Skeletal related events, bone metastasis and survival of prostate cancer: a population based cohort study in Denmark (1999 to 2007). J Urol. 2010;184(1):162–167. doi:10.1016/j.juro.2010.03.03420483155
- Sanjaya I, Mochtar CA, Umbas R. Correlation between low Gleason score and prostate specific antigen levels with incidence of bone metastases in prostate cancer patients: when to omit bone scans? Asian Pac J Cancer Prev. 2013;14(9):4973–4976. doi:10.7314/APJCP.2013.14.9.497324175761
- Saad F, Lipton A, Cook R, Chen YM, Smith M, Coleman R. Pathologic fractures correlate with reduced survival in patients with malignant bone disease. Cancer. 2007;110(8):1860–1867. doi:10.1002/cncr.2299117763372
- Haberkorn U, Eder M, Kopka K, Babich JW, Eisenhut M. New strategies in prostate cancer: prostate-specific membrane antigen (PSMA) ligands for diagnosis and therapy. CliNl Cancer Res. 2016;22(1):9–15. doi:10.1158/1078-0432.CCR-15-0820
- Fendler WP, Rahbar K, Herrmann K, Kratochwil C, Eiber M. 177Lu-PSMA radioligand therapy for prostate cancer. J Nucl Med. 2017;58(8):1196–1200. doi:10.2967/jnumed.117.19102328663195
- Barrio M, Fendler WP, Czernin J, Herrmann K. Prostate specific membrane antigen (PSMA) ligands for diagnosis and therapy of prostate cancer. Expert Rev Mol Diagn. 2016;16(11):1177–1188. doi:10.1080/14737159.2016.124305727679869
- Farooki A, Leung V, Tala H, Tuttle RM. Skeletal-related events due to bone metastases from differentiated thyroid cancer. J Clin Endocrinol Metab. 2012;97(7):2433–2439. doi:10.1210/jc.2012-116922564664
- Doll KM, Rademaker A, Sosa JA. Practical guide to surgical data sets: surveillance, epidemiology, and end results (SEER) database. JAMA Surg. 2018;153(6):588–589. doi:10.1001/jamasurg.2018.050129617544
- Liu WC, Li ZQ, Luo ZW, Liao WJ, Liu ZL, Liu JM. Machine learning for the prediction of bone metastasis in patients with newly diagnosed thyroid cancer. Cancer Med. 2021;10(8):2802–2811. doi:10.1002/cam4.377633709570
- Goecks J, Jalili V, Heiser LM, Gray JW. How machine learning will transform biomedicine. Cell. 2020;181(1):92–101. doi:10.1016/j.cell.2020.03.02232243801
- Darcy AM, Louie AK, Roberts LW. Machine learning and the profession of medicine. JAMA. 2016;315(6):551–552. doi:10.1001/jama.2015.1842126864406
- Oliphant TE. Python for scientific computing. Comput Sci Eng. 2007;9(3):10–20. doi:10.1109/MCSE.2007.58
- Altmann A, Toloşi L, Sander O, Lengauer T. Permutation importance: a corrected feature importance measure. Bioinformatics. 2010;26(10):1340–1347. doi:10.1093/bioinformatics/btq13420385727
- Chen T, He T, Benesty M, Khotilovich V, Tang Y, Cho H. Xgboost: extreme gradient boosting. R Package Version 04-2. 2015;1(4):1–4.
- Qi Y. Random forest for bioinformatics. In: Ensemble Machine Learning. Springer; 2012:307–323.
- Tang J, Deng C, Huang G-B. Extreme learning machine for multilayer perceptron. IEEE Trans Neural Netw Learn Syst. 2015;27(4):809–821. doi:10.1109/TNNLS.2015.242499525966483
- Sperandei S. Understanding logistic regression analysis. Biochem Med. 2014;24(1):12–18. doi:10.11613/BM.2014.003
- Myles AJ, Feudale RN, Liu Y, Woody NA, Brown SD. An introduction to decision tree modeling. J Chemom. 2004;18(6):275–285. doi:10.1002/cem.873
- Rish I, editor. An empirical study of the naive Bayes classifier. In: IJCAI 2001 Workshop on Empirical Methods in Artificial Intelligence; 2001.
- Fornetti J, Welm AL, Stewart SA. Understanding the bone in cancer metastasis. Bone Miner Res. 2018;33(12):2099–2113. doi:10.1002/jbmr.3618
- Kuten J, Fahoum I, Savin Z, et al. Head-to-head comparison of 68Ga-PSMA-11 with 18F-PSMA-1007 PET/CT in staging prostate cancer using histopathology and immunohistochemical analysis as a reference standard. J Nucl Med. 2020;61(4):527–532. doi:10.2967/jnumed.119.23418731562225
- Afshar-Oromieh A, Babich JW, Kratochwil C, et al. The rise of PSMA ligands for diagnosis and therapy of prostate cancer. J Nucl Med. 2016;57(Supplement3):79S–89S. doi:10.2967/jnumed.115.17072027694178
- Lenzo NP, Meyrick D, Turner JH. Review of gallium-68 PSMA PET/CT imaging in the management of prostate cancer. Diagnostics. 2018;8(1):16. doi:10.3390/diagnostics8010016
- Camacho DM, Collins KM, Powers RK, Costello JC, Collins JJ. Next-generation machine learning for biological networks. Cell. 2018;173(7):1581–1592. doi:10.1016/j.cell.2018.05.01529887378
- Zhu J, Zheng J, Li L, et al. Application of machine learning algorithms to Predict central lymph node metastasis in T1-T2, non-invasive, and clinically node negative papillary thyroid carcinoma. Front Med. 2021;8. doi:10.3389/fmed.2021.635771
- Ogunleye A, Wang Q-G. XGBoost model for chronic kidney disease diagnosis. IEEE/ACM Trans Comput Biol Bioinform. 2019;17(6):2131–2140. doi:10.1109/TCBB.2019.2911071
- Greene KL, Albertsen PC, Babaian RJ, et al. Prostate specific antigen best practice statement: 2009 update. J Urol. 2013;189(1):S2–S11. doi:10.1016/j.juro.2012.11.01423234625
- Network NCC. NCCN clinical practice guidelines in oncology. Prostate cancer V. 4; 2011. Available from: http://www.nccn.org/professionals/physician_gls/pdf/prostate.pdf. Accessed November 17, 2021.
- Guo X, Zhang C, Guo Q, et al. The homogeneous and heterogeneous risk factors for the morbidity and prognosis of bone metastasis in patients with prostate cancer. Cancer Manag Res. 2018;10:1639. doi:10.2147/CMAR.S16857929970963
- Briganti A, Suardi N, Gallina A, et al. Predicting the risk of bone metastasis in prostate cancer. Cancer Treat Rev. 2014;40(1):3–11. doi:10.1016/j.ctrv.2013.07.00123896177
- Chen S, Wang L, Qian K, et al. Establishing a prediction model for prostate cancer bone metastasis. Int J Biol Sci. 2019;15(1):208. doi:10.7150/ijbs.2753730662360
- Stolzenbach LF, Rosiello G, Deuker M, et al. The impact of race and age on distribution of metastases in patients with prostate cancer. J Urol. 2020;204(5):962–968. doi:10.1097/JU.000000000000113132396050
- Lu YJ, Duan WM. Establishment and validation of a novel predictive model to quantify the risk of bone metastasis in patients with prostate cancer. Transl Androl Urol. 2021;10(1):310–325. doi:10.21037/tau-20-113333532320
- Wilczak W, Wittmer C, Clauditz T, et al. Marked prognostic impact of minimal lymphatic tumor spread in prostate cancer. Eur Urol. 2018;74(3):376–386. doi:10.1016/j.eururo.2018.05.03429908878
- Battisti V, Maders LD, Bagatini MD, et al. Ectonucleotide pyrophosphatase/phosphodiesterase (E-NPP) and adenosine deaminase (ADA) activities in prostate cancer patients: influence of Gleason score, treatment and bone metastasis. Biomed Pharmacother. 2013;67(3):203–208. doi:10.1016/j.biopha.2012.12.00423433854
- Zaman MU, Fatima N, Sajjad Z. Metastasis on bone scan with low prostate specific antigen (≤ 20 ng/mL) and Gleason’s score (< 8) in newly diagnosed Pakistani males with prostate cancer: should we follow Western guidelines. Asian Pac J Cancer Prev. 2011;12(6):1529–1532.22126493
- Vaarala MH, Hirvikoski P, Kauppila S, Paavonen TK. Identification of androgen-regulated genes in human prostate. Mol Med Rep. 2012;6(3):466–472. doi:10.3892/mmr.2012.95622735730