Abstract
The high prevalence of type 2 diabetes mellitus in the world as well as the increasing reports about the adverse side effects of the existing diabetes treatment drugs have made developing new and effective drugs against the disease a very high priority. In this study, we report ten novel compounds found by targeting peroxisome proliferator-activated receptors (PPARs) using virtual screening and core hopping approaches. PPARs have drawn increasing attention for developing novel drugs to treat diabetes due to their unique functions in regulating glucose, lipid, and cholesterol metabolism. The reported compounds are featured with dual functions, and hence belong to the category of dual agonists. Compared with the single PPAR agonists, the dual PPAR agonists, formed by combining the lipid benefit of PPARα agonists (such as fibrates) and the glycemic advantages of the PPARγ agonists (such as thiazolidinediones), are much more powerful in treating diabetes because they can enhance metabolic effects while minimizing the side effects. This was observed in the studies on molecular dynamics simulations, as well as on absorption, distribution, metabolism, and excretion, that these novel dual agonists not only possessed the same function as ragaglitazar (an investigational drug developed by Novo Nordisk for treating type 2 diabetes) did in activating PPARα and PPARγ, but they also had more favorable conformation for binding to the two receptors. Moreover, the residues involved in forming the binding pockets of PPARα and PPARγ among the top ten compounds are explicitly presented, and this will be very useful for the in-depth conduction of mutagenesis experiments. It is anticipated that the ten compounds may become potential drug candidates, or at the very least, the findings reported here may stimulate new strategies or provide useful insights for designing new and more powerful dual-agonist drugs for treating type 2 diabetes.
Introduction
Type 2 diabetes mellitus (T2DM) is the most common type of diabetes mellitus, which is characterized by insulin resistance and combined with relatively reduced insulin secretion.Citation1 It is a chronic metabolic disease that affects the body’s ability to turn food into energy. People developing T2DM may suffer from blindness, renal failure, coronary artery disease, and so forth.Citation2 Because of its dramatic increase worldwide, T2DM has reached an epidemic scale that is anticipated to affect over 360 million people by 2030.Citation3,Citation4
Belonging to the nuclear hormone receptor family, peroxisome proliferator-activated receptors (PPARs) are the ligand-activated transcription factors.Citation5,Citation6 PPARs, particularly the subtypes PPARα and PPARγ, played a central role in regulating the expression of genes involved in the control of lipid and lipoprotein metabolism, glucose homeostasis, and inflammatory processes. Accordingly, activating these receptors has become a promising therapeutic strategy for designing drugs against T2DM.Citation7
The activated PPARα receptors have a beneficial effect on lipid metabolism by regulating genes on fatty acid and cholesterol metabolism.Citation8 PPARα agonists belong to the class of lipid-lowering drugs (such as fenofibrate and gemfibrozil), and are widely prescribed to reduce triglycerides.Citation9 The PPARγ receptors, the most widely studied subtype of PPARs, are located in adipocytes, muscles, and macrophages, where they have a direct influence on T2DM, dyslipidemia, atherosclerosis, and cardiovascular diseases.Citation10,Citation11 Thiazolidinediones are a class of PPARγ agonists used in clinical practice to reduce plasma glucose level in type 2 diabetic patients, or to regulate glucose homeostasis by increasing insulin sensitivity and glucose disposal.Citation12
Unfortunately, if the drug were used alone, the undesirable side effects such as weight gain, edema, and anemia would be caused;Citation13 therefore, it is an urgent and challenging task to develop new dual agonists. In this regard, the novel PPARα/PPARγ dual agonist, formed by combining the lipid benefit of PPARα agonists (such as fibrates) with the glycemic advantages of the PPARγ agonists (such as thiazolidinediones), has drawn considerable attention.Citation14 In developing the dual agonists, a critical problem surrounds how to identify the selectivity ratio of the receptor subtype,Citation15 because it may provide useful insights for finding the new drug candidates. There is more information available about designing potential dual treatments for treating T2DM.Citation16
Many studies have indicated that computational approaches, such as structural bioinformatics,Citation17 molecular docking,Citation18 pharmacophore modeling,Citation19 Quantitative Structure–Activity Relationship (QSAR) techniques,Citation20–Citation24 and a series of user-friendly web server predictors developed recently, such as G-protein-coupled receptors-grey incident degree analysis (GPCR-GIA) and G-protein-coupled receptors -2-layer predictor (GPCR-2L) for identifying G protein-coupled receptors and their types,Citation25,Citation26 iLoc-Euk and iLoc-Hum for predicting subcellular localization of eukaryotic and human proteins,Citation27,Citation28 NR-2L for identifying nuclear receptors and their subfamilies,Citation29 ProtIdent for identifying proteases and their types,Citation30 and HIVcleave for predicting human immunodeficiency virus protease cleavage sites in proteins,Citation31,Citation32 can provide timely and very useful information, as well as insights for drug development. In addition to the aforementioned approaches, the Virtual Screening technique and Core Hopping technique are also very useful.Citation33–Citation36 The former can be used to screen for novel molecular scaffolds,Citation37,Citation38 while the latter can be used to generate new lead compounds with improved core properties.Citation37,Citation38
Encouraged by the aforementioned successful studies, the present study was initiated in an attempt to screen the fragment database in hopes to find a new antidiabetic compound. Meanwhile, the techniques of the core hopping associated with the glide docking and molecular dynamic simulation were utilized to analyze the binding interactions between the agonist and PPARs.Citation39,Citation40 The absorption, distribution, metabolism, and excretion (ADME) predictions were also used to evaluate whether the new agonist found to date possesses great potential to become a promising drug candidate for treating diabetes mellitus.Citation41,Citation42
Materials and methods
The representative complex crystal structures of PPARα (Protein Data Bank [PDB] ID 1k7l) and PPARγ (PDB ID 1k74) were downloaded from the PDB,Citation43,Citation44 and were to be used for the molecular modeling studies.
Our calculations are operated with the Schrödinger software package (version 9.0.111; Schrödinger, LLC, New York, NY, USA), which runs on a Dell Precision™ T5500 computer (Dell, Round Rock, TX, USA). The molecular dynamic simulation was performed using the Gromacs 4.0 package (www.gromacs.com) for the Linux system.
Protein structures and databases
We downloaded the crystal structures of PPARα (1k71.pdb) and PPARγ (1k74.pdb) from the Protein Data Bank,Citation43,Citation44 and used them as receptors for the current modeling. The reasons behind the selection of the two proteins are: (1) the two proteins contain the same ligand, GW409544, which is used to easily identify the binding site for comparison; (2) the resolutions of the two crystal structures are quite similar with one (1k7l.pdb) having 2.50 Å and the other (1k74.pdb) having 2.30 Å; (3) the source organism of both structures was humans.Citation43
The protein-ligand binding site was identified by the SiteMap tool embedded in the Schrodinger Suite 2009 (Schrödinger, LLC), as described previously.Citation45–Citation47 The binding site encompassed the ligand GW409544, which was observed in the crystal structures of both PPARα (1k7l) and PPARγ (1k74). The binding site of PPARs is composed of three arms: arm 1, arm 2, and arm 3, as marked in the panels A and B of . These three segments play an important role in the interactions between the receptor and ligand.
Figure 1 Illustration to show the superimposed conformations obtained by docking ragaglitazar and the ten derivative compounds (Comp#1–#10) to PPARα and PPARγ receptors, respectively. (A) Ragaglitazar and Comp#1–#10 to PPARα (1k7l). (B) Ragaglitazar and Comp#1–#10 to PPARγ (1k74). (C) Ragaglitazar and Comp#1 to PPARα (1k7l). (D) Ragaglitazar and Comp#1 to PPARγ (1k74).
Abbreviations: PPARα, peroxisome proliferator-activated receptor-alpha; PPARγ, peroxisome proliferator-activated receptor-gamma.
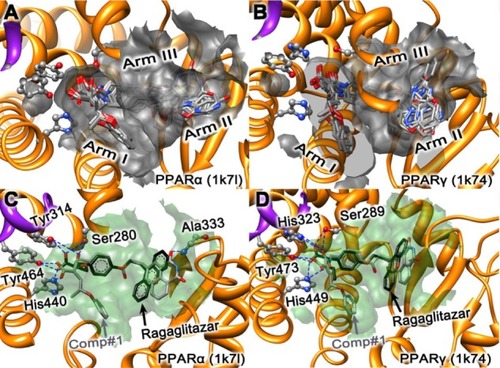
Several hydrophobic interactions between the three “arms” and the Y-shaped ligand were deemed as the key points for designing the new PPARs agonist.Citation48
Arm 1 has a predominately polar character and includes the AF2-helix (shown as the purple ribbon in ), which is responsible for the function of transcriptional activation. The vital conformation of AF2-helix could generate a charge clamp formed by the network hydrogen bonds, thus significantly reducing the mobility of AF2 and regulating gene expression.Citation7 The hydrophobic tail of the agonist interacts with either arm 2 or arm 3 to improve binding affinity.Citation7
The lead compounds and the fragment databases derived from ZINC (Shoichet Laboratory, Department of Pharmaceutical Chemistry, University of California, San Francisco, CA, USA) were used for virtual screening and core hopping searching,Citation49 as described below, respectively.
Virtual screening
ZINC (Shoichet Library, Department of Pharmaceutical Chemistry, University California) was a free database of commercially available compounds and contained over 13 million purchasable compounds in three-dimensional formats.Citation49 The lead compound (or “lead-now”) database, one subdatabase of the ZINC, was chosen for virtual screening to find the optimal molecular scaffold by the Glide5 docking program (Schrödinger, LLC),Citation35,Citation36 which was interfaced with Schrödinger Suite 2009 (Schrödinger, LLC). The preparation and refinement protocols for the protein receptor and all compound structures were performed on the Protein Preparation Wizard and LigPrep modules embedded in the Schrödinger Suite (2009; Schrödinger, LLC),Citation35 respectively. For protein preparation, the process included assigning bond orders, adding hydrogen, treating metals, treating disulfides, deleting waters, alleviating potential steric hindrance, adjusting bond order, and assigning the formal charges by protein minimization with the OPLS2005 force field;Citation50 the constrained refinement value of RMSD (root mean square deviation) for the protein was limited to 0.3 Å. Meanwhile, for the compounds of the databases, the preparation consisted of generating possible states by ionization at a target pH of 7.0 ± 2.0, desalting, retaining specified chiralities from the three-dimensional structure, and completing geometry minimization with the OPLS2005 force field.Citation50 When the above steps were accomplished, all the investigated compounds were docked into the receptor pocket through the rigid docking model with a high throughput virtual screening scoring function to estimate the binding affinities.Citation39,Citation40
Molecular docking with core hopping method
Many useful insights for drug design could be gained by conducting molecular docking studies.Citation17,Citation18,Citation51–Citation53 In this study, a new docking algorithm called “core hopping” was employed.Citation35,Citation36 Core hopping offers function of being able to perform both the fragment-based replacing and molecular docking,Citation36 which makes it a very powerful and cutting-edge technique for de novo drug design because it allows for rapidly screening novel cores to help overcome unwanted properties by generating new lead compounds with improved core properties, as demonstrated by a series of previous studies.Citation37,Citation38
The procedures of the core hopping computation can be briefly described as follows. The first step was to define the possible points to which the cores were attached; this could be done by using the program “Define Combinations” from the Combinatorial Screening panel in Schrodinger 2009 (Schrödinger, LLC). The second step was to define the “receptor grid file,” which could be done in the “Receptor Preparation” panel. The third step was the cores preparation; this could be done by operating the “Protocore Preparation” module to find the cores attached to the scaffold and using the fragment database derived from ZINC.Citation49
The fourth step was to align and dock the entire molecular structure built up by the core and scaffold. After all the above four steps were accomplished, all the investigated compounds were redocked into the receptor pocket via the rigid protein docking model with the Stand-Precision scoring function to estimate the binding affinities.Citation39,Citation40
Molecular dynamics simulations
Many marvelous biological functions in proteins and deoxyribonucleic acid (DNA) and their profound dynamic mechanisms, such as switching between active and inactive states,Citation54,Citation55 cooperative effects,Citation56 allosteric transition,Citation57–Citation59 intercalation of drugs into DNA,Citation60 and assembly of microtubules,Citation61 can be revealed by studying their internal motions.Citation62 Likewise, to really understand the interaction mechanism of a receptor with its ligand, we should consider not only the static structures concerned, but also the dynamic information obtained by simulating their internal motions or dynamic processes.Citation63,Citation64
To examine whether the designed agonist remains bound by an explicit solvent from a dynamic point of view, the molecular dynamic simulation was performed with the periodic boundary conditions using the GROMACS 4.0 package under GROMACS 96-53a6 force fields for proteins.Citation65 The topology files and charges for the ligand atoms were generated by the Dundee PRODRG2.5 Server (beta).Citation66 Before starting the simulations, the systems were solvated in explicit water with a flexible simple point charge model in a cubic box, under the condition that the surface of the protein was covered with a water shell of 1.0 nm (nanometer or 10−9 of a meter). The minimum number of sodium and chloride ions needed to balance the system charge was placed randomly in the solvated system. Subsequently, the energy minimization was performed for the complex system by using the steepest descent method.Citation67,Citation68 Then, the 10 ns (or 10−9 of a second) MD simulations were carried out with a time step of 2 fs (femtosecond, or 10−15 of a second). The corresponding coordinates are stored every 2ps (picosecond, or 10−12 of a second). The Particle Mesh Ewald algorithm was used to calculate the electrostatic interactions.Citation69 All simulations were run under the periodic boundary condition with the Normal Pressure and Temperature (NPT) ensemble by using Berenson’s coupling algorithm for keeping the temperature at 310 K and the pressure at 1 atm. All bonds are constrained by using the Linear Constraint Solver algorithm.Citation70
ADME prediction
ADME is a very useful program to eliminate unfavorable compounds with undesired properties in the early stage of drug development via evaluating the proposed structural refinements prior to expensive synthesis.Citation27,Citation42,Citation71 Incorporating ADME predictions as a part of the drug development process can generate lead compounds that are more likely to exhibit satisfactory ADME performances during clinical trials.
The program of QikProp embedded in Schrödinger 2009 (Schrödinger, LLC) is a quick, accurate, and easy-to-use program for predicting the ADME properties of the compounds.Citation41,Citation72,Citation73 The QikProp program adopted here was featured by having the functions predict a total of 44 properties of compounds, including the principal descriptors and physiochemical properties.
All the investigated compounds need not be neutralized before using QikProp because it will be automatically done in QikProp. The normal mode of prediction of QikProp was used in this study. The property analysis for the molecular weight – partition coefficient (QP logP o/w) – predicted aqueous solubility (QP logS); in addition, apparent PMDCK permeability (QPP MDCK) was computed in the QikProp program to evaluate whether the compounds hold the potential to become drug candidates.Citation74
Results and discussion
Virtual screening and core hopping
The detailed procedure of discovering the desired dual agonists is shown in . The lead-now database from ZINC was screened by utilizing the Glide5 software for its optimal performance targeting the PPAR receptors (PPARα:1k7l and PPARγ:1k74).Citation35,Citation36,Citation49 The top hit, ZINC36728034 (see ) was selected as the optimal lead compound for further modification according to the well known structures of ragaglitazar and GW409544, as shown in .Citation43,Citation75 Consequently, the phenyl group rendered by red color in ZINC36728034 (see ) is modified into an acidic group colored with blue, as shown in Comp#0 (). The functional acidic group also exits in the following structures muraglitazar,Citation76 faraglitazar,Citation77 GW6471,Citation78 GW7845,Citation77 CHEMBL166572,Citation79 and so on. The new molecule Comp#0 was designed with the intention so it has stronger affinity than ZINC36728034. Subsequently, the core hopping method was used to search the fragment database for the desired chemical groups to replace the amide group (ie, the R0 group) (). Doing this was to help lengthen the Comp#0 structure so as to make it better fit the roomy space in arm 2 (see ). As a result, the amide group (ie, R0 group) of Comp#0 in was replaced by each of the best ten R groups (ie, the R1 to R10, as marked on the right side of ). Subsequently, they were used to generate the whole molecules (ie, Comp#1 to Comp#10) through the core hopping approach (see ). The ten compounds displayed the same chiral center (S) of the α carbon atom of the acidic group with the ragaglitazar and the GW409544 (see ), and the other compounds of Comp#5 to Comp#10 with the other preferred chiral centers in R groups allowed them to match the active pocket.
Figure 2 Illustration to show how to generate the best ten compounds from the ZINC36728034 structure through the core hopping method.
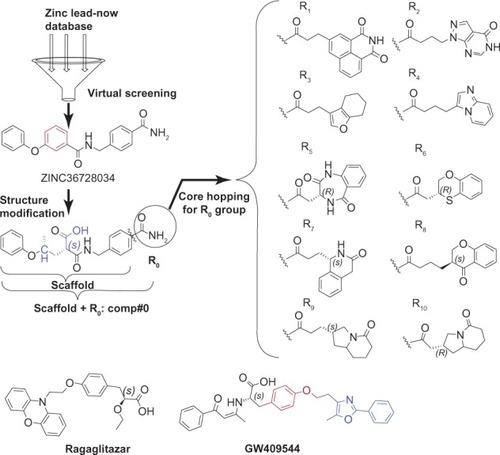
The above strategy of this study is very similar that in to our previous paper;Citation38 however, the starting point at which the study was carried out is very different. This current study used virtual screening as the first operation to find the novel scaffold against PPARs; we also used a previous study for this same purpose,Citation80 and we then developed new compounds with the core hopping method, while the previous study mainly modified the well known ligand GW409544 though the use of two steps of the core hopping method.Citation38 At first glance, the current study is quite similar to a previous study,Citation38 for we used the same protein targets, fragment database, core hopping method, and molecular dynamics modeling, but the detailed approaches are completely different. Therefore, it will be necessary to combine both studies that use the core hopping method for novel drug development in order to provide more comprehensive information.
Modeling of PPAR agonist complex
The best ten potential lead compounds or candidates obtained via the above step were redocked into the two receptors, PPARα (1k71) and PPARγ (1k74), respectively. As shown in the panels A and B of , the best docked poses of the ten compounds are superposed together in the active pockets of the two receptors, PPARα (1k71) and PPARγ (1k74), respectively. From the docking simulation results, it can be seen that all the compounds form good steric complementarity with the hydrophobic pocket of each receptor and produce strong van der Waals contacts and hydrophobic interactions. As shown in the black in panels C and D of , ragaglitazar (a novel dual agonist for PPARα and PPARγ that also includes the important polar acidic head group in its structure) was chosen as a positive control for its high affinity with the PPARα and PPARγ receptors in the previous research.Citation75,Citation81–Citation83
Listed in are the top ten compounds (ie, Comp#1 to Comp#10) that are much stronger than ragaglitazar and Comp#0 in binding to the two PPARα (1k71) and PPARγ (1k74) receptors, and these are ranked roughly according to their docking scores to the receptors. The best docked conformations of Comp#1 (carbon atoms colored in gray) and ragaglitazar (carbon atoms colored in black) are aligned together in panels C and D of . The results of receptor–ligand interactions obtained from the docking simulation had proved that the key residues for the binding interactions between Comp#1 and the receptor were fully consistent with the previous reports.Citation43,Citation71,Citation84 The conservative H-bonding network formed by the polar acidic head group in both ragaglitazar and Comp#1 to the four key residues of PPARα (or PPARγ), such as Ser280 (or Ser289), Tyr314 (or His323), Tyr464 (or Tyr473), and His440 (or His449) were observed in our docking simulation. It is noteworthy that Comp#1 has three oxygen atoms to serve as the polar acidic head, which allows Comp#1 to have more opportunities to interact with the AF2-helix. In addition, one more H-bond formed between the oxygen atom of the R1 group and Ala333 residue only in the PPARα (1k7l) receptor was observed in this study. The H-bonding networks made a major contribution in stabilizing the active conformation of AF2-helix in arm I1, which is extraordinarily important for the regulation of gene expression.Citation7 The hydrophobic part of Comp#1 derived from ZINC36728034 played an important role in complementing the hydrophobic arm 1 formed by the hydrophobic residues, as shown by the light green surface (see panels C and D of ). Compared with ragaglitazar, the Comp#1 compound has an extra hydrophobic part, making it more fitted to the hydrophobic arm 1, and hence resulting in a much better binding affinity than ragaglitazar, as clearly indicated by the docking scores shown in . Among the compounds listed in , Comp#1 has the strongest binding affinity with the two receptors PPARα and PPARγ, and hence it was singled out as the representative compound used for further investigation.
Table 1 The compound ragaglitazar was used as a positive control, and the ten compounds (Comp#1–#10) were ranked roughly according to their docking scores to the receptors PPARα and PPARγ
Molecular dynamics trajectory analysis
To acquire the relevant information from a dynamic point of view, the 10 ns molecular dynamic simulations were carried out, respectively, for the crystal structures of PPARα (1k7l), PPARγ (1k74), as well as their complexes with ragaglitazar and Comp#1: PPARα–ragaglitazar, PPARγ–ragaglitazar, PPARα–Comp#1, and PPARγ–Comp#1. As we can see from , all of the characters concerned reached the simulation equilibrium within the 5 ns.
Figure 3 Illustration to show the outcomes of molecular dynamic simulations for the interactions of the receptors with Comp#1 – the best derivative found in this study, as shown in . (A) The RMSD of all backbone atoms for the receptor PPARα. (B) The RMSD of all backbone atoms for the receptor PPARγ. (C) The RMSF of the side-chain atoms for the receptor PPARα. (D) The RMSF of the side-chain atoms for the receptor PPARγ.
Abbreviations: RMSD, root mean square deviation; PPARα, peroxisome proliferator-activated receptor-alpha; PPARγ, peroxisome proliferator-activated receptor-gamma; RMSF, root mean square fluctuation.
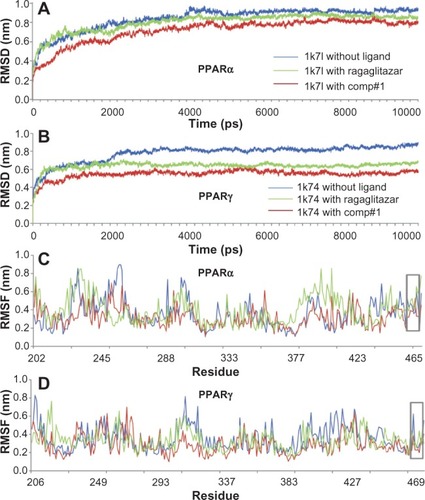
The RMSD from the initial conformation is a major criterion used to evaluate the stability of a protein system. Shown in the panels A and B of are the backbone RMSD curves for PPARα, PPARγ, PPARα–ragaglitazar, PPARγ–ragaglitazar, PPARα–Comp#1, and PPARγ–Comp#1. It is interesting to see that the RMSD values of PPARα–Comp#1 and PPARγ–Comp#1 are remarkably smaller than those of PPARα–ragaglitazar and PPARγ–ragaglitazar, indicating that the complexes of PPARα–Comp#1 and PPARγ–Comp#1 are more stable than PPARα–ragaglitazar and PPARγ–ragaglitazar.
In order to perform an in-depth study of the interactions of the AF2 helix with the agonist (), the root mean square fluctuations for all the side-chain atoms of the receptors were also computed, as shown in panels C and D of . It can be seen from the figure that the side-chain of the root mean square fluctuations of PPARα–Comp#1 or PPARγ–Comp#1 were very similar to that of PPARα–ragaglitazar or PPARγ–ragaglitazar, respectively. This is particularly true for the residues 459–465 of PPARα–Comp#1 and the residues 469–477 of PPARγ–Comp#1 (see the gray framed box marked with AF2 in panels C and D of ), indicating that the new designed Comp#1 is very likely to perform exactly the same function in activating the AF2 helix as the compound ragaglitazar did.
ADME prediction
Also listed in are the corresponding ADME properties that are directly relevant to pharmaceutical potentials. It can be seen from the table that, compared with ragaglitazar, the predicted ADME properties, such as the polar surface area, logPo/w, logS, PPCaco, and percent of human oral absorption for the newly designed agonists are either much better than or quite close to that of ragaglitazar. Actually, these percentages are all within the acceptable ranges for humans, indicating that the ten compounds found in this study, particularly the Comp#1 compound, hold very high potential to become a new drug candidate.
Binding pocket
In drug design, particularly for conducting mutagenesis studies,Citation17 the information of the binding pocket of a targeted receptor with its ligand is crucially important. According to a previous study,Citation85 such binding pockets were defined by those residues of the receptor that have at least one heavy atom (ie, an atom other than hydrogen) with a distance ≤ 5 Å from a heavy atom of the ligand. Similar criteria were also used to define the binding pockets of many other receptor–ligand interactions.Citation37,Citation51,Citation86–Citation91 The same criterion used in a previous study was also used in this study for defining the binding pockets of receptors PPARα and PPARγ with the ligand, GW409544.Citation85 From this point of view, the residues involved in forming the binding pocket of PPARα and PPARγ for the docked ligand Comp#1 are listed in , which are identical to the other nine compounds, where those marked by an asterisk are the key residues for forming the H-bonding network, as shown in .
Table 2 The residues involved in forming the binding pocket of PPARα and PPARγ for the ligand Comp#1Table Footnotea
Acknowledgments
The authors thank the three anonymous reviewers and the editor for their valuable comments, which were very helpful for strengthening the presentation of this study.
Disclosure
The authors report no conflicts of interest in this work.
References
- Chehade JM Mooradian AD A rational approach to drug therapy of type 2 diabetes mellitus Drugs 2000 60 1 95 113 10929931
- Carpino PA Goodwin B Diabetes area participation analysis: a review of companies and targets described in the 2008–2010 patent literature Expert Opin Ther 2010 20 12 1627 1651
- Wild S Roglic G Green A Sicree R King H Global prevalence of diabetes: estimates for the year 2000 and projections for 2030 Diabetes Care 2004 27 5 1047 1053 15111519
- DeFronzo RA Pathogenesis of type 2 diabetes mellitus Med Clin North Am 2004 88 4 787 835 ix 15308380
- Berger J Moller DE The mechanisms of action of PPARs Annu Rev Med 2002 53 409 435 11818483
- Berger JP Akiyama TE Meinke PT PPARs: therapeutic targets for metabolic disease Trends Pharmacol Sci 2005 26 5 244 251 15860371
- Markt P Schuster D Kirchmair J Laggner C Langer T Pharmacophore modeling and parallel screening for PPAR ligands J Comput Aided Mol Des 2007 21 10–11 575 590 17960326
- van Raalte DH Li M Pritchard PH Wasan KM Peroxisome proliferator-activated receptor (PPAR)-alpha: a pharmacological target with a promising future Pharm Res 2004 21 9 1531 1538 15497675
- Issemann I Prince RA Tugwood JD Green S The peroxisome proliferator-activated receptor:retinoid X receptor heterodimer is activated by fatty acids and fibrate hypolipidaemic drugs J Mol Endocrinol 1993 11 1 37 47 8240670
- Evans RM Barish GD Wang YX PPARs and the complex journey to obesity Nat Med 2004 10 4 355 361 15057233
- Semple RK Chatterjee VK O’Rahilly S PPAR gamma and human metabolic disease J Clin Invest 2006 116 3 581 589 16511590
- Lehmann JM Moore LB Smith-Oliver TA Wilkison WO Wilson TM Klieweer SA An antidiabetic thiazolidinedione is a high affinity ligand for peroxisome proliferator-activated receptor gamma (PPAR gamma) J Biol Chem 1995 270 22 12953 12956 7768881
- Rosen ED Spiegelman BM PPARgamma: a nuclear regulator of metabolism, differentiation, and cell growth J Biol Chem 2001 276 41 37731 37734 11459852
- Henke BR Peroxisome proliferator-activated receptor alpha/gamma dual agonists for the treatment of type 2 diabetes J Med Chem 2004 47 17 4118 4127 15293980
- Mochizuki K Suruga K Yagi E Takase S Goda T The expression of PPAR-associated genes is modulated through postnatal development of PPAR subtypes in the small intestine Biochim Biophys Acta 2001 1531 1–2 68 76 11278173
- Jones RM New Therapeutic Strategies for Type 2 Diabetes: Small Molecule Approaches London, UK RSC Publishing 2012
- Chou KC Structural bioinformatics and its impact to biomedical science Curr Med Chem 2004 11 16 2105 2134 15279552
- Chou KC Wei DQ Zhong WZ Binding mechanism of coronavirus main proteinase with ligands and its implication to drug design against SARS Biochem Biophys Res Commun 2003 308 1 148 151 12890493
- Sirois S Wei DQ Du QS Chou KC Virtual Screening for SARS-CoV protease based on KZ7088 pharmacophore points J Chem Inf Comput Sci 2004 44 3 1111 1122 15154780
- Dea-Ayuela MA Pérez-Castillo Y Meneses-Marcel A HP-Lattice QSAR for dynein proteins: experimental proteomics (2D-electrophoresis, mass spectrometry) and theoretic study of a Leishmania infantum sequence Bioorg Med Chem 2008 16 16 7770 7776 18662882
- Prado-Prado FJ González-Díaz H de la Vega OM Ubeira FM Chou KC Unified QSAR approach to antimicrobials. Part 3: First multi-tasking QSAR model for input-coded prediction, structural back-projection, and complex networks clustering of antiprotozoal compounds Bioorg Med Chem 2008 16 11 5871 5880 18485714
- Prado-Prado FJ Martinez de la Vega O Uriarte E Ubeira FM Chou KC González-Díaz H Unified QSAR approach to antimicrobials. 4. Multitarget QSAR modeling and comparative multi-distance study of the giant components of antiviral drug-drug complex networks Bioorg Med Chem 2009 17 2 569 575 19112024
- Du QS Huang RB Wei YT Pang ZW Du LQ Chou KC Fragment-based quantitative structure-activity relationship (FB-QSAR) for fragment-based drug design J Comput Chem 2009 30 2 295 304 18613071
- Hou X Du J Fang H Li M 3D-QSAR study on a series of Bcl-2 protein inhibitors using comparative molecular field analysis Protein Pept Lett 2011 18 5 440 449 21171944
- Lin WZ Xiao X Chou KC GPCR-GIA: a web-server for identifying G-protein coupled receptors and their families with grey incidence analysis Protein Eng Des Sel 2009 22 11 699 705 19776029
- Xiao X Wang P Chou KC GPCR-2L: predicting G protein-coupled receptors and their types by hybridizing two different modes of pseudo amino acid compositions Mol Biosyst 2011 7 3 911 919 21180772
- Chou KC Wu ZC Xiao X iLoc-Euk: a multi-label classifier for predicting the subcellular localization of singleplex and multiplex eukaryotic proteins PLoS One 2011 6 3 e18258 21483473
- Chou KC Wu ZC Xiao X iLoc-Hum: using accumulation-label scale to predict subcellular locations of human proteins with both single and multiple sites Mol Biosyst 2012 8 2 629 641 22134333
- Wang P Xiao X Chou KC NR-2L: a two-level predictor for identifying nuclear receptor subfamilies based on sequence-derived features PLoS One 2011 6 8 e23505 21858146
- Chou KC Shen HB ProtIdent: a web server for identifying proteases and their types by fusing functional domain and sequential evolution information Biochem Biophys Res Commun 2008 376 2 321 325 18774775
- Shen HB Chou KC HIVcleave: a web-server for predicting human immunodeficiency virus protease cleavage sites in proteins Anal Biochem 2008 375 2 388 390 18249180
- Chou KC Prediction of human immunodeficiency virus protease cleavage sites in proteins Anal Biochem 1996 233 1 1 14 8789141
- Richards WG Virtual screening using grid computing: the screensaver project Nat Rev Drug Discov 2002 1 7 551 555 12120261
- Roche O Schneider P Zuegge J Development of a virtual screening method for identification of “frequent hitters” in compound libraries J Med Chem 2002 45 1 137 142 11754585
- Senger S Using Tversky similarity searches for core hopping: finding the needles in the haystack J Chem Inf Model 2009 49 6 1514 1524 19453147
- Henke BR Consler TG Go N A new series of estrogen receptor modulators that display selectivity for estrogen receptor beta J Med Chem 2002 45 25 5492 5505 12459017
- Li XB Wang SQ Xu WR Wang RL Chou KC Novel inhibitor design for hemagglutinin against H1N1 influenza virus by core hopping method PLoS One 2011 6 11 e28111 22140516
- Ma Y Wang SQ Xu WR Wang RL Chou KC Design novel dual agonists for treating type-2 diabetes by targeting peroxisome proliferator-activated receptors with core hopping approach PLoS One 2012 7 6 e38546 22685582
- Eldridge MD Murray CW Auton TR Paolini GV Mee RP Empirical scoring functions: I. The development of a fast empirical scoring function to estimate the binding affinity of ligands in receptor complexes J Comput Aided Mol Des 1997 11 5 425 445 9385547
- Halgren TA Murphy RB Friesner RA Glide: a new approach for rapid, accurate docking and scoring. 2. Enrichment factors in database screening J Med Chem 2004 47 7 1750 1759 15027866
- Jorgensen WL Duffy EM Prediction of drug solubility from structure Adv Drug Deliv Rev 2002 54 3 355 366 11922952
- Tian S Li Y Wang J Zhang J Hou T ADME evaluation in drug discovery. 9. Prediction of oral bioavailability in humans based on molecular properties and structural fingerprints Mol Pharm 2011 8 3 841 851 21548635
- Xu HE Lambert MH Montana VG Structural determinants of ligand binding selectivity between the peroxisome proliferator-activated receptors Proc Natl Acad Sci USA 2001 98 24 13919 13924 11698662
- Berman HM Battistuz T Bhat TN The Protein Data Bank Acta Crystallogr D Biol Crystallogr 2002 58 Pt 61 899 907 12037327
- Husslein T Moore PB Zhong Q Newns DM Pattnaik PC Klein ML Molecular dynamics simulation of a hydrated diphytanol phosphatidylcholine lipid bilayer containing an alpha-helical bundle of four transmembrane domains of the influenza A virus M2 protein Faraday Discuss 1998 111 201 208 discussion 225 246 10822610
- Kochendoerfer GG Salom D Lear JD Wilk-Orescan R Kent SB DeGrado WF Total chemical synthesis of the integral membrane protein influenza A virus M2: role of its C-terminal domain in tetramer assembly Biochemistry 1999 38 37 11905 11913 10508393
- Gandhi CS Shuck K Lear JD Cu(II) inhibition of the proton translocation machinery of the influenza A virus M2 protein J Biol Chem 1999 274 9 5474 5482 10026160
- Feige JN Gelman L Tudor C Engelborghs Y Wahli W Desvergne B Fluorescence imaging reveals the nuclear behavior of peroxisome proliferator-activated receptor/retinoid X receptor heterodimers in the absence and presence of ligand J Biol Chem 2005 280 18 17880 17890 15731109
- Irwin JJ Schoichet BK ZINC – a free database of commercially available compounds for virtual screening J Chem Inf Model 2005 45 1 177 182 15667143
- Banks JL Beard HS Cao Y Integrated Modeling Program, Applied Chemical Theory (IMPACT) J Comput Chem 2005 26 16 1752 1780 16211539
- Liao QH Gao QZ Wei J Chou KC Docking and molecular dynamics study on the inhibitory activity of novel inhibitors on epidermal growth factor receptor (EGFR) Med Chem 2011 7 1 24 31 21235516
- Cai L Wang Y Wang JF Chou KC Identification of proteins interacting with human SP110 during the process of viral infections Med Chem 2011 7 2 121 126 21222611
- Liu XY Wang RL Xu WR Tang LD Wang SQ Chou KC Docking and molecular dynamics simulations of peroxisome proliferator activated receptors interacting with pan agonist sodelglitazar Protein Pept Lett 2011 18 10 1021 1027 21592078
- Chou KC The biological functions of low-frequency vibrations (phonons). 4. Resonance effects and allosteric transition Biophys Chem 1984 20 1–2 61 71 6487745
- Wang JF Gong K Wei DQ Li YX Chou KC Molecular dynamics studies on the interactions of PTP1B with inhibitors: from the first phosphate-binding site to the second one Protein Eng Des Sel 2009 22 6 349 355 19380334
- Chou KC Low-frequency resonance and cooperativity of hemoglobin Trends Biochem Sci 1989 14 6 212 213 2763333
- Chou KC The biological functions of low-frequency vibrations (phonons). III. Helical structures and microenvironment Biophys J 1984 45 5 881 889 6428481
- Lian P Wei DQ Wang JF Chou KC An allosteric mechanism inferred from molecular dynamics simulations on phospholamban pentamer in lipid membranes PLoS One 2011 6 4 e18587 21525996
- Chou KC The biological functions of low-frequency vibrations (phonons): VI. A possible dynamic mechanism of allosteric transition in antibody molecules Biopolymers 1987 26 2 285 295 3828475
- Chou KC Mao B Collective motion in DNA and its role in drug intercalation Biopolymers 1988 27 11 1795 1815 3233332
- Chou KC Zhang CT Maggiora GM Solitary wave dynamics as a mechanism for explaining the internal motion during microtubule growth Biopolymers 1994 34 1 143 153 8110966
- Chou KC Low-frequency collective motion in biomacromolecules and its biological functions Biophys Chem 1988 30 1 3 48 3046672
- Schnell JR Chou JJ Structure and mechanism of the M2 proton channel of influenza A virus Nature 2008 451 7178 591 595 18235503
- Huang RB Du QS Wang CH Chou KC An in-depth analysis of the biological functional studies based on the NMR M2 channel structure of influenza A virus Biochem Biophys Res Commun 2008 377 4 1243 1247 18996090
- Oostenbrink C Soares TA van der Vegt NF van Gunsteren WF Validation of the 53A6 GROMOS force field Eur Biophys J 2005 34 4 273 284 15803330
- Schüttelkopf AW van Aalten DM PRODRG: a tool for high-throughput crystallography of protein-ligand complexes Acta Crystallogr D Biol Crystallogr 2004 60 Pt 8 1355 1363 15272157
- Bhaya A Kaszkurewicz E Steepest descent with momentum for quadratic functions is a version of the conjugate gradient method Neural Netw 2004 17 1 65 71 14690708
- Deift P Venakides S Zhou X An extension of the steepest descent method for Riemann-Hilbert problems: the small dispersion limit of the Korteweg-de Vries (KdV) equation Proc Natl Acad Sci USA 1998 95 2 450 454 11038618
- Cerutti DS Duke RE Darden TA Lybrand TP Staggered Mesh Ewald: An extension of the Smooth Particle-Mesh Ewald method adding great versatility J Chem Theory Comput 2009 5 9 2322 20174456
- Hess B Bekker H Berendsen HJC Fraaije JGEM LINCS: a linear constraint solver for molecular simulations J Comput Chem 1998 18 12 1463 1472
- Liu XY Wang RL Xu WR Tang LD Wang SQ Chou KC Docking and molecular dynamics simulations of peroxisome proliferator activated receptors interacting with pan agonist sodelglitazar Protein Pept Lett 2011 18 10 1021 1027 21592078
- Liu Y Hong L Yu LS The role of ADME evaluation in translation research of innovative drug Yao Xue Xue Bao 2011 46 1 19 29 Chinese 21465804
- Jorgensen WL Duffy EM Prediction of drug solubility from Monte Carlo simulations Bioorg Med Chem Lett 2000 10 11 1155 1158 10866370
- Singh KHD Kirubakaran P Nagarajan S Homology modeling, molecular dynamics, e-pharmacophore mapping and docking study of Chikungunya virus nsP2 protease J Mol Model 2012 18 1 39 51 21445710
- Pickavance LC Brand CL Wassermann K Wilding JP The dual PPARalpha/gamma agonist, ragaglitazar, improves insulin sensitivity and metabolic profile equally with pioglitazone in diabetic and dietary obese ZDF rats Br J Pharmacol 2005 144 3 308 316 15655531
- Waites CR Dominick MA Sanderson TP Schilling BE Nonclinical safety evaluation of muraglitazar, a novel PPARalpha/gamma agonist Toxicol Sci 2007 100 1 248 258 17675651
- Heppner TJ Bonev AD Eckman DM Gomez MF Petkov GV Nelson MT Novel PPARgamma agonists GI 262570, GW 7845, GW 1929, and pioglitazone decrease calcium channel function and myogenic tone in rat mesenteric arteries Pharmacology 2005 73 1 15 22 15452359
- Ding L Liang XG Lou YJ Time-dependence of cardiomyocyte differentiation disturbed by peroxisome proliferator-activated receptor alpha inhibitor GW6471 in murine embryonic stem cells in vitro Acta Pharmacol Sin 2007 28 5 634 642 17439719
- Sauerberg P Pettersson I Jeppesen L Novel tricyclic-alpha-alkyloxyphenylpropionic acids: dual PPARalpha/gamma agonists with hypolipidemic and antidiabetic activity J Med Chem 2002 45 4 789 804 11831892
- Scarsi M Podvinec M Roth A Sulfonylureas and glinides exhibit peroxisome proliferator-activated receptor gamma activity: a combined virtual screening and biological assay approach Mol Pharmacol 2007 71 2 398 406 17082235
- Pavankuamr VV Vinu CA Mullangi R Srinivas NR Preclinical pharmacokinetics and interspecies scaling of ragaglitazar, a novel biliary excreted PPAR dual activator Eur J Drug Metab Pharmacokinet 2007 32 1 29 37 17479541
- Skrumsager BK Nielsen KK Müller M Pabst G Drake PG Edsberg B Ragaglitazar: the pharmacokinetics, pharmacodynamics, and tolerability of a novel dual PPAR alpha and gamma agonist in healthy subjects and patients with type 2 diabetes J Clin Pharmacol 2003 43 11 1244 1256 14551179
- Chakrabarti R Vikramadithyan RK Misra P Ragaglitazar: a novel PPAR alpha PPAR gamma agonist with potent lipid-lowering and insulin-sensitizing efficacy in animal models Br J Pharmacol 2003 140 3 527 537 12970088
- Ebdrup S Pettersson I Rasmussen HB Synthesis and biological and structural characterization of the dual-acting peroxisome proliferator-activated receptor alpha/gamma agonist ragaglitazar J Med Chem 2003 46 8 1306 1317 12672231
- Chou KC Watenpaugh KD Heinrikson RL A model of the complex between cyclin-dependent kinase 5 and the activation domain of neuronal Cdk5 activator Biochem Biophys Res Commun 1999 259 2 420 428 10362524
- Chou KC Molecular therapeutic target for type-2 diabetes J Proteome Res 2004 3 6 1284 1288 15595739
- Pielak RM Schnell JR Chou JJ Mechanism of drug inhibition and drug resistance of influenza A M2 channel Proc Natl Acad Sci USA 2009 106 18 7379 7384 19383794
- Wang JF Chou KC Insights from modeling the 3D structure of New Delhi metallo-β-lactamase and its binding interactions with antibiotic drugs PLoS One 2011 6 4 e18414 21494599
- Housaindokht MR Bozorgmehr MR Bahrololoom M Analysis of ligand binding to proteins using molecular dynamics simulations J Theor Biol 2008 254 2 294 300 18599089
- Wang JF Chou KC Insights into the mutation-induced HHH syndrome from modeling human mitochondrial ornithine transporter-1 PLoS One 2012 7 1 e31048 22292090
- Chou KC Insights from modeling the 3D structure of the extracellular domain of alpha7 nicotinic acetylcholine receptor Biochem Biophys Res Commun 2004 319 2 433 438 15178425
- Chou KC Watenpaugh KD Heinrikson RL A model of the complex between cyclin-dependent kinase 5 and the activation domain of neuronal Cdk5 activator Biochem Biophys Res Commun 1999 259 2 420 428 10362524