Abstract
Background
To date, no specific vaccine or drug has been proven to be effective against SARS-CoV-2 infection. Therefore, we implemented an immunoinformatic approach to design an efficient multi-epitopes vaccine against SARS-CoV-2.
Results
The designed-vaccine construct consists of several immunodominant epitopes from structural proteins of spike, nucleocapsid, membrane, and envelope. These peptides promote cellular and humoral immunity and interferon-gamma responses. Also, these epitopes have a high antigenic capacity and are not likely to cause allergies. To enhance the vaccine immunogenicity, we used three potent adjuvants: Flagellin of Salmonella enterica subsp. enterica serovar Dublin, a driven peptide from high mobility group box 1 as HP-91, and human beta-defensin 3 protein. The physicochemical and immunological properties of the vaccine structure were evaluated. The tertiary structure of the vaccine protein was predicted and refined by Phyre2 and Galaxi refine and validated using RAMPAGE and ERRAT. Results of ElliPro showed 246 sresidues from vaccine might be conformational B-cell epitopes. Docking of the vaccine with toll-like receptors (TLR) 3, 5, 8, and angiotensin-converting enzyme 2 approved an appropriate interaction between the vaccine and receptors. Prediction of mRNA secondary structure and in silico cloning demonstrated that the vaccine can be efficiently expressed in Escherichia coli.
Conclusion
Our results demonstrated that the multi-epitope vaccine might be potentially antigenic and induce humoral and cellular immune responses against SARS-CoV-2. This vaccine can interact appropriately with the TLR3, 5, and 8. Also, it has a high-quality structure and suitable characteristics such as high stability and potential for expression in Escherichia coli .
Introduction
Severe acute respiratory syndrome coronavirus 2 (SARS-CoV-2) which the cause of COVID-19 disease was first reported as a pneumonia epidemic in the Chinese city of Wuhan (Hubei province) on December 31, 2019, belongs to the Beta coronavirus genus.Citation1–Citation3 Coronaviruses are positive-sense single-stranded RNA viruses. They belong to the order of Nidovirales and superfamily of Orthocoronaviridae. This superfamily has four genus including alpha, beta, gamma, and delta coronaviruses. Gamma and delta coronaviruses infect birds, while alpha and beta coronaviruses generally infect mammals such as humans. In immunocompetent individuals, they generally cause mild respiratory infections, such as common cold, while in some individuals, coronavirus infections cause serious diseases, such as SARS (Severe Acute Respiratory Syndrome) and MERS (Middle East Respiratory Syndrome) epidemics.
SARS-CoV-2 has a large mRNA genome, 26 to 32 kb in length, meaning a 5ʹ cap cover structure and a 3ʹ polyadenylated. This encodes several structural and nonstructural proteins. Among structural proteins, spike (S), envelope (E), membrane (M), and nucleocapsid (N) proteins are important in inducing immune responses.Citation3–Citation5 Spike protein is the main tool for virus entry into the cells, which interacts with host cell receptor, angiotensin-converting enzyme 2 (ACE2). ACE2 is a metallopeptidase expressed in variant tissues, including alveolar epithelial cells, fibroblasts, endothelial cells, and enterocytes.Citation6–Citation10 The N protein is an essential RNA-binding protein for transcription and replication of the viral RNA. It has significant roles in forming of the helical ribonucleoproteins during packaging the RNA genome, regulating of viral RNA synthesis during replication, transcription, and modulating of metabolism in the infected cells.Citation11–Citation14 T cell responses against the S and N proteins of SARS-CoV virus are most dominant and long-lived than other structural proteins.Citation15 E protein has an important role in the assembly of the viral genome.Citation16,Citation17 The M protein plays a pivotal role in virus assembly, budding, and replication of virus particles in the host cells.Citation18
SARS-CoV-2 is transmitted quickly and causes a considerable fatality rate, so that World Health Organization (WHO) reported over 14,562 550 cases globally and at least 9898 deaths because of this disease until July 21, 2020.Citation19 It can be controlled when medical resources are sufficient but, there is currently no vaccine or approved treatment for this disease. One of the major challenges for scientists in dealing with the new coronavirus pandemic will be to get a useful and effective vaccine. For this reason, trying to develop an effective vaccine to control the virus has been the subject of research by many scientists around the world.Citation4,Citation20-24 Previous studies on vaccine production against infectious diseases proved that achieving such a vaccine with conventional methods is time consuming and very expensive. As such, in silico prediction of the vaccine targets is very important because the targets can be selected with an open eye in a limited time and resources. Bioinformatic approaches are very helpful to identify the effective epitopes and developing vaccines. Several vaccine candidates for viral diseases have been reported based on this approach that include effective vaccines in Human papilloma viridea (HPV),Citation25 Ebola,Citation26 ZikaCitation27 and MERSCitation28,Citation29 viruses. There are few reports of COVID-19 vaccine.Citation30–Citation32 They contained multiple cytotoxic T lymphocytes (CTL) and B-cell epitopes against several proteins of SARS-CoV-2 virus. However, those vaccines did not cover most of the immunodominant viral proteins. Therefore, to overwhelm these limitations, we designed a multi-epitope peptide vaccine consisted of S, M, N, and E viral proteins. The vaccine has appropriate physicochemical properties such as stability at room temperature, more antigenic capability, and no possibility of allergy. It consists of three epitopes from S protein and one epitope from each of M, N, and E proteins, respectively. Each of the epitopes has a high ability to stimulate humoral and cellular immune responses, especially the proper production of Interferon gamma (IFN-γ). The selected epitopes aligned between SARS-CoV-2 and SARS species. We used appropriate adjuvants in the vaccine structure to potentiate the immunogenicity of the antigens. Therefore, we also incorporated the potent adjuvants of N and C terminus of Flagellin of Salmonella enterica subsp. enterica serovar Dublin as a toll-like receptor (TLR) 5, a driven peptide from high mobility group box 1 (HMGB1) as HP-91, and human beta-defensin 3 (HBD-3) in the construct of the vaccine. The vaccine segments were connected to each other by appropriate linkers. Physicochemical properties, structural stability, and immunological characterizations of the vaccine were evaluated. Modeling, refinement, and validation were performed to access a high-quality three-dimensional (3D) structure of the vaccine protein. Docking evaluation showed an appropriate interaction between the vaccine and TLRs 3, 5, 8, and ACE-2. In silico cloning showed that the vaccine could be effectively expressed in E. coli. Totally, a potential vaccine candidate with proper immunological and stable physicochemical properties against SARS-CoV-2 was designed. It is expected the vaccine could be capable to protect humans from COVID-19 disease.
Methods
Protein Sequence Retrieval
The protein sequences of spike glycoprotein (accession number of QIC53213.1), nucleocapsid phosphoprotein (QHU79211.1), membrane glycoprotein (QIC53207.1) and envelope protein (QIC53215.1) were driven using NCBI (https://www.ncbi.nlm.nih.gov/) databases and saved in FASTA format for subsequent analysis.
Screening of Potential Epitopes
Screening of Major Histocompatibility Complex Class I (MHC-I) Epitopes
MHC-I humans alleles with 9 mer length were selected using IEDB (www.iedb.org) database through IEDB recommended 2.22 method and Net-MHC 4.0 online server (http://www.cbs.dtu.dk/services/NetMHC/). IEDB (Instructor/Evaluator Database) is a repository of web-based tools which predicts and analysis of immune epitopes. It uses a consensus method based on artificial neural network (ANN), stabilized matrix method (SMM), and Combinatorial Peptide Libraries (CombLib), if any corresponding predictor is available for the peptide sequence.Citation33–Citation51 NetMHC 4.0 software is a free server for the prediction of peptide-MHCI binding affinity by gapped sequence alignment. Prediction methods of this server are based on alignments that include insertions and deletions and have significantly higher performance than those based on peptides of single lengths methods.Citation35
Screening of Cytotoxic T Lymphocytes (CTL) Epitopes
The prediction of CTL epitopes was done by an online server CTL Pred. This method is based on quantitative matrix (QM) and machine learning techniques, for example, artificial neural network (ANN) and support vector machine (SVM). The server also uses the consensus and combined prediction approaches. The consensus and combined prediction approaches are more specific and sensitive, respectively, in comparison with individual approaches such as ANN and SVM.Citation52
Screening of Human Leukocyte Antigen (HLA)-II Epitopes
IEDB database (IEDB recommended 2.22 method)Citation43Citation53–Citation56 (www.iedb.org) and RANKPEP online server (http://imed.med.ucm.es/Tools/rankpep.html) were employed for screening of HLA-II epitope. The database of IEDB was described previously. The RANKPEP server predicts MHC-II binding epitope by position-specific scoring matrices (PSSMs) that are structurally consistent with the binding mode of MHC-II ligands.Citation57
Screening of Linear B-Cell Epitopes
Prediction of linear B-cell epitope was done by IEDBCitation58 (Emini surface accessibility method) and BepiPred software. BepiPred 2.0 predicts the location of linear B-cell epitopes by a combination of a hidden Markov model and a propensity scale method. The epitope Threshold of this server was selected 0.5.Citation59
Selection of the Epitope Segments
The results of all predictions were collected and compared together, and the regions with the highest overlaps were determined. These immunodominant regions were employed for future analyses to finally select the most appropriate epitope domains for vaccine construct.
Alignment of Selected Epitopes Between SARS-CoV-2 and SARS
The alignment of the selected epitopes in SARS-CoV-2 and SARS was performed to understand the selected epitopes that are conserved in two viruses. The amino acid sequences of structural proteins of SARS were retrieved from the NCBI (https://www.ncbi.nlm.nih.gov/) database and their epitopes blasted using CLC sequence viewer 8.0.Citation60
In silico Analyzing of IFN-γ Inducing Epitopes
IFN epitope server (http://crdd.osdd.net/raghava/ifnepitope) was employed to determine the ability of IFN-γ production in the selected epitopes. This web server classifies MHC binder epitopes into IFN-γ inducing (positive numbers) and non-inducing IFN-γ (negative numbers) using several methods such as; motifs-based search, machine learning technique, and a hybrid approach. Accuracy of Best prediction based on hybrid approach in this software is 82.10%.Citation61
Evaluation of Antigenicity
Antigenic properties of the predicted-epitopes were assessed by ANTIGENpro (http://scratch.proteomics.ics.uci.edu/) and VaxiJen v2.0 (http://www.ddg-pharmfac.net/vaxijen/VaxiJen/VaxiJen.html) servers. Web server of ANTIGENpro is the first sequence-based, alignment-free, and pathogen independent predictor, using protein antigenicity microarray data for predicting the protein antigenicity.Citation62 VaxiJen is also the first server for the prediction of antigens. This server applies a new alignment-independent approach that is based on auto-cross covariance (ACC) transformation of protein sequences into uniform vectors of principal amino acid properties. Depending on the target organism (bacteria, virus, or tumor) accuracy of this server varies from 70% to 89%. The Threshold of this server was selected at 0.5.Citation63,Citation64
Allergenicity Assessment of Predicted Epitopes
To accurately predict the possibility of allergenicity, the software of AllergenFP v.1.0 (http://ddg-pharmfac.net/AllergenFP/) was used. This is an online server that identifies allergens based on amino acid principle properties such as hydrophobicity, helix, and β-strand forming with accuracy of about 88%.Citation40
Vaccine Engineering, Evaluation of Physicochemical Properties, Antigenicity, and Allergenicity
According to the results of the previous steps, three epitopes from S protein and one epitope from each protein of N, E, and M were selected to be incorporated in the vaccine construction. The multi-epitope vaccine construct was joined to the carboxyl and amino terminals of the flagellin adjuvant. In addition, HP-91 and HBD-3 synthetic peptide adjuvants were respectively incorporated at the N- and C-terminal of the epitopes. The felagellin molecule was derived from Salmonella enterica subsp. enterica serovar Dublin. Various segments of the designed-vaccine connected by suitable linkers.
After designing the vaccine, several physicochemical parameters including molecular weight, theoretical isoelectric point (pI), total number of positive and negative residues, extinction coefficient, Instability index, half-life, aliphatic index, and grand average hydropathy (GRAVY) were computed using Expasy’s ProtParam at http://us.expasy.org/tools/protparam.html.Citation65 Antigenicity of the final vaccine construct was evaluated using vaxijen v2.0 and ANTIGENpro and allergenicity were assayed by AllergenFP v.1.0 server.
Tertiary Structure Development
Three-dimensional (3D) structural modeling of the designed construct was predicted using Phyre2 server (http://www.sbg.bio.ic.ac.uk/phyre2). It is a suite of a free web server for prediction and analysis of protein structure, function, and mutations.Citation66 The predicted model by Phyre2 was refined using Galaxy Refine web services. It successfully tested in CASP10 (Critical assessment of techniques for protein structure prediction) experiments. This server refines the whole protein with gentle and aggressive relaxation methods. This first reconstructs all side-chain conformations and repeatedly relaxes the structure with short molecular dynamics simulations after side-chain repacking perturbations.Citation67 Finally, Galaxy Refine showed five structures as refined models of vaccine structure. The RAMPAGE (http://mordred.bioc.cam.ac.uk/~rapper/rampage.php), and ERRAT (http://services.mbi.ucla.edu/ERRAT/) servers were used to validate the refined 3D structures obtained from Galaxy Refine web service.Citation68,Citation69 Finally, the refined and high-quality 3D structure of the vaccine was observed by PyMOL software v2.1.1.PyMOL is an open-source that widely used for bimolecular function.Citation70
Prediction of Discontinuous B-Cell Epitope
Discontinuous B-cell epitopes were predicted from the 3D vaccine structure using ElliPro in IEDB database (http://tools.immuneepitope.org/tools/ElliPro). ElliPro is a useful research tool for identifying immune epitopes in protein antigens which implements Thornton’s method and with a residue clustering algorithm. The MODELLER program and the Jmol viewer allow the prediction and visualization of immune epitopes in a given protein sequence or structure. In comparison with six other structure-based methods that are using for epitope prediction, ElliPro performs the best prediction and gave an AUC value of 0.732, when the most significant prediction is considered for each protein.Citation71
Molecular Docking with TLR 3, 5, 8, and ACE-2
The first, tertiary structure of the human TLR 3, 5, 8 and ACE-2 were obtained from PDB database (www.rcsb.org) with codes of 3J0A, 5GS0, 3w3g, and 1R42, respectively. Next, protein–protein docking of the vaccine structure (as a ligand) and each receptor was performed by CLUSPRO 2.0 online server (cluspro.bu.edu/login.php). CLUSPRO 2.0 is a fully automated and fast rigid-body protein–protein docking server. This server evaluates docking of two interacting proteins based on three techniques: first, the Fast Fourier Transform (FFT) correlation approach, second, clustering of the best energy conformations, third, refining the obtained model using short Monte Carlo simulations and the medium-range optimization method SDU.Citation72–Citation74 The interaction between molecules was observed using Discovery studio 4.5.
Codon Optimization, in silico Cloning and Prediction of the mRNA Secondary Structure
The reverse translation of the designed-vaccine gene sequence was performed by Sequence Manipulation Suite (https://www.bioinformatics.org/sms2/rev_trans.html) to prepare a suitable vaccine sequence for cloning and expression in an appropriate expression system. The properties of sequence genes such as Codon Adaptation Index (CAI), GC content, and Codon Frequency and Distribution (CFD) have the key roles in attaining a high level of protein expression in the host were evaluated using GenScript online server (https://www.genscript.com/tools/rare-codon-analysis).Citation24 Finally, the restriction sites of NcoI and XhoI were, respectively, added to the N- and C-terminus of the vaccine DNA sequence using CLC Sequence viewer v8.0 (http://www.cacbio.com) to facilitate the cloning in E. coli expression system.
To predict the mRNA folding and secondary structure of the vaccine, the optimized DNA sequence was converted to possible RNA sequence using DNA<->RNA<->Protein tool (http://biomodel.uah.es/en/lab/cybertory/analysis/trans.htm). Then, the mRNA secondary structure was predicted using RNAfold (http://rna.tbi.univie.ac.at/RNAWebSuite/help.html) server. This server predicts the mRNA secondary structure thermodynamically and provides minimum free energy (ΔG Kcal/mol) for structure.Citation75
Results
Immunoinformatic Analysis
Protein S, M, N, and E were used to predict HLA-І binding epitopes (HLA-A, B, and C) using IEDB and NetMHC 4. CTL Pred server was used to predict CTL epitopes of SARS-CoV-2 structural proteins. HLA-II binding epitope (DP, DQ, and DR) from these proteins were predicted using RANKEP and IEDB servers. Prediction of linear B-cell epitopes was performed by BepiPred and IEDB. Finally, the obtained epitopes from the comparison of the above analyses were applied to future selection ().
Table 1 The Final Immunodominant Epitopes Were Selected from S, M, N, and E Proteins of SARS-CoV-2
Antigenic Ability Screening and Avoid Allergenicity
All selected peptides were submitted to the IFN epitope server for evaluation of their ability to induce IFN-γ. Allergenicity probability of epitopes evaluated by AllergenFPE valuation of epitope antigenicity was performed by ANTIGEN pro and VaxiJen v2.0.The six epitopes (three from S and one from each of E, M, N proteins) that had the highest ability in IFN-γ induction, low allergenicity, and potent antigenic ability were chosen to be used in the vaccine structure ().
Alignment of the Selected Epitopes Between SARS-CoV-2 and SARS Viruses
The alignment of the selected epitopes between SARS-CoV-2 and SARS viruses showed the selected epitopes were conserved between two species (Table S1).
Designing a Multi-Epitopes Vaccine Construct
Six epitopes with high scores which selected to be incorporated in the final vaccine construct were the sequences of 20–49, 418–446, and 505–534 from S, 51–71 from E, 360–388 from N and 169–198 from M protein. Two epitopes, sequences 418–446 and 505–534, of S protein were located in RBD portion. Besides, three immunopotent adjuvants Flagellin of Salmonella enterica subsp. enterica serovar Dublin, HP-91, and HBD-3 were also added to the vaccine structure. The vaccine pieces were linked to each other using a short linker sequence of LE dipeptide repeats. The final multi-epitope peptide vaccine was 485 amino acid residues (). Figure S1 .
Figure 1 Schematic representation of the designed multi-epitope peptide based-vaccine. The vaccine consist of ten parts: Epitopes from structural proteins S, E, N, and M, adjuvants: Flagellin (in N-and C-terminus), HP-91 and HBD-3 that join to each other by linkers of repeat sequence of LE (A). Tertiary structure of the modeled multi-epitope vaccine construct (B). The 3D structure of the designed vaccine was predicted via homology modeling by Phyre2, then the best-predicted model was refined by Galaxy Refine and visualized using Discovery studio 4.5 software. N-and C-terminus of Flagellin is shown in green, HP-91 in gray, S epitopes in blue, E epitopes in red, N epitope in purple, M epitope in orange, HBD-3 in green, and linkers are shown in light pink.
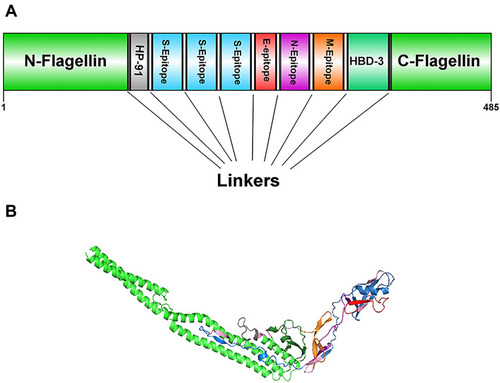
Immunological and Physicochemical Properties of the Vaccine Structure
To evaluate the antigenicity of the whole protein vaccine, the “virus” option was chosen as a target organism. The antigenicity of 0.5585 (probable antigen) was estimated at 0.5% threshold for the virus model. Assessment of antigenicity by Antigen pro showed this vaccine is high antigenic with antigenicity of 0.927820. AllergenFP showed that the T-cell epitopes in the vaccine protein are non-allergen. The molecular weight (MW) and theoretical isoelectric point (pI) of the vaccine protein were computed as 53.102 kDa and 7.92, respectively. The predicted half-lives were calculated as 30 hours (h) (mammalian reticulocytes, in vitro), 20 h (yeast, in vivo), and 10 h (E. coli, in vivo). The instability index was 36.41, indicating that the protein vaccine was stable enough ()
Table 2 Analysis of the Physicochemical and Immunological Properties of the SARS-CoV-2 Vaccine
Tertiary Structure Modeling, Refinement, and Validation
The primary 3D model of the protein vaccine construct was predicted using Phyre2. Then, the selected Phyre2 model was refined by GalaxyRefine software. The quality of the designed-vaccine was evaluated using the Ramachandran plot in the RAMPAGE server and characteristic atomic interaction in the ERRAT server. The RAMPAGE results of the final model showed that the majority of residues (95.9%) are located in the favored region and 2.1% are allowed, and only 2.1% of residues are the outlier. The ERRAT result showed that the refined model has the ERRAT score 89.362 (Figure S2). The outputs of the Ramachandran plot and ERRAT indicated that the refined 3D structure is good and therefore, can be utilized as a reliable model for further evaluations.
Conformational B-Cell Epitope Identification
The tertiary structure of the designed vaccine was used as an input for conformational (discontinuous) B-cell epitope prediction via ElliPro in IEDB. From 485 amino acid residues, 246 were defined as discontinued B-cell epitope ().
Table 3 Conformational B-Cell Epitopes Identified in the Refined Tertiary Structure of the Multi-Epitope Vaccine Using ElliPro
Identifying Binding Sites and Protein–Protein Docking
ClusPro server has been used to perform the docking of the vaccine with TLR3, 5, 8, and ACE-2 molecules. Cluspro generated 30 models for each interaction. The docked model was selected based on most atoms interacted in the vaccine and each of the receptors (–).
Figure 2 Docking model (cartoon representation) of human TLR3 in complex with the vaccine obtained using Cluspro server. TLR3 protein is shown in chocolate and vaccine was colored from N-to C-terminals (N-flagellin is shown in blue and C-flagellin is shown in red). As it shows some parts of two S, M, N, and E epitopes, HBD-3, and HP-91 interacted with TLR3. To more visualized interaction points, some of the interacting residues of the vaccine and TLR3 are magnified in 20 Angstrom. Docked model was visualized via Discovery studio 4.5 software.
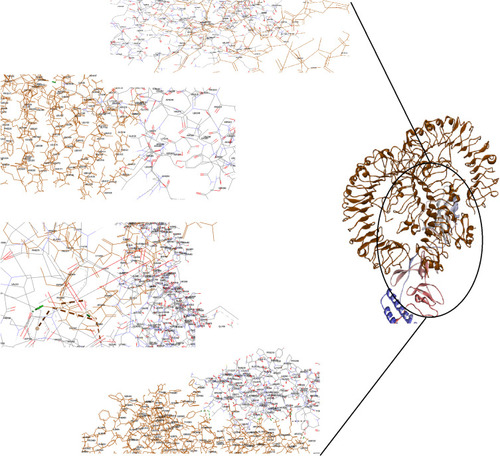
Figure 3 Docking model (cartoon representation) of human TLR5 in complex with the vaccine obtained using Cluspro server. TLR5 is shown in chocolate and vaccine was colored from N-to C-terminals (N-flagellin is shown in blue and C-flagellin is shown in red). As the figure shows some parts of Flagellin, S-epitope and HP-91 interacted with TLR5. In order to more visualized interaction points, some of the interacting residues of the vaccine and TLR5 are magnified in 20 Angstrom. Docked model was visualized by Discovery studio 4.5.
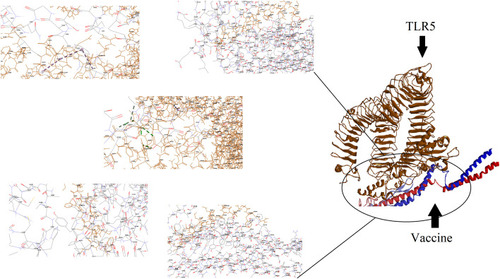
Figure 4 Docking model (cartoon representation) of human TLR8 in complex with the vaccine obtained using Cluspro server. TLR8 protein is shown in chocolate and vaccine was colored from N-to C-terminals (N-flagellin is shown in blue and C-flagellin is shown in red. As the figure shows some parts of S, M, N, and E epitopes, HBD-3 and HP-91 interacted with TLR8). In order to more visualized interaction points, some of the interacting residues of the vaccine and TLR8 are magnified in 20 Angstrom. Docked model was visualized using Discovery studio 4.5.
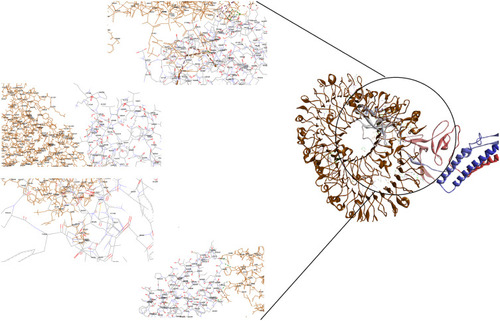
Figure 5 Docking model (cartoon representation) of human ACE-2 in complex with the vaccine obtained using Cluspro server. ACE-2 protein is shown in chocolate and vaccine was shown in purple. As the figure shows RBD epitopes of vaccine interacted with ACE-2. In order to more visualized interaction points, some of the interacting residues of the vaccine and ACE-2 are magnified in 20 Angstrom. Docked model was visualized using Discovery studio 4.5.
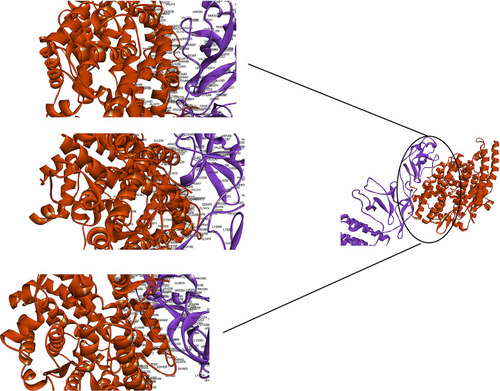
In silico Codon Optimization of the Vaccine Construct and Prediction of the mRNA Secondary Structure
The reverse translation of the protein vaccine into a nucleotide sequence was performed simultaneously using the Sequence Manipulation Suite server to express high-level protein in E. coli. Codon Adaptation Index (CAI), GC content, and Codon Frequency and Distribution (CFD) were evaluated using GenScript online server. A CAI of the vaccine optimized nucleotide sequence was 1. The GC content of the structure was in the ideal range of 58.73% (30–70%), and CFD 100 indicating the effective expression of the protein in the host. To clone the gene into E. coli vectors, NcoI and XhoI restriction sites were added into the N- and C-terminals of the sequence using CLC Sequence viewer v.8. Finally, the vaccine gene was inserted into the PET-28 vector (). Minimum free energy of the vaccine was determined using RNA fold server, the optimized mRNA construct showed ΔG value of −520 kcal/mol. Figure S2 illustrates the predicted secondary structure of the vaccine.
Figure 6 Evaluation of the three important parameters of the codon-optimized gene to express high-level protein in E. coli. (A) CAI of the gene sequence was 1. It is noted that a CAI of > 0.8 will be considered as good for expression in the selected host. (B) Average GC content of the sequence was 58.73%. (C) Codon frequency distribution (CFD) value of the gene sequence is 100. A CFD equal to100 supports maximum protein expression in the desired host. (D) Insertion of the vaccine gene in the PET28 vector by restriction enzymes of NcoI and XhoI.
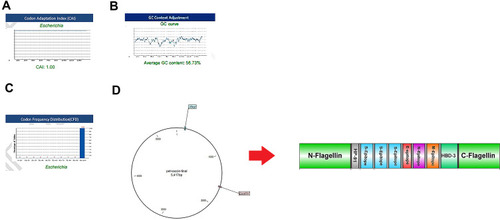
Discussion
Today, there is an urgent need to design effective vaccines to stop the indescribable spread of COVID-19. In light of recent advances in bioinformatic approaches, we can identify immunodominant T- and B-cell epitopes and design a potential vaccine to prevent the disease. We used an in silico analysis to design a potent multi-epitope peptide vaccine against SARS-CoV-2. The vaccine contains 485 amino acids which constructed from three specific epitopes from S (two epitopes from RBD section (and one epitope from each of the structural proteins of E, M, and N)). These proteins have essential roles in the infection of host cells and host immune modulation. Therefore, it is expected that the vaccine will have a high ability to induce the production of neutralizing antibodies by B lymphocytes and the production of IFN-γ by (T helper) Th and CTL cells.
Adjuvants are essential components of multi-epitope vaccines because they increase the immunologic properties of the vaccine structures. We used Flagellin of Salmonella enterica subsp. enterica serovar Dublin, a TLR5 agonist, in two N and C terminus of the vaccine construct. Flagellin induces innate immune effectors such as cytokines and nitric oxide,Citation76,Citation77 activates TLR5-positive dendritic cells (DCs), neutrophils, and epithelial cellsCitation78–Citation81 and stimulates the activation of adaptive immune responses mainly Th2-type and IgA production.Citation78,Citation79,Citation82–Citation85 Intranasal administration of Flagellin stimulates the signaling of TLR5 in lung epithelial cells and pneumonocytes.Citation86 It was used broadly as an adjuvant in vaccine structure designed against viral infections such as influenzaCitation85,Citation87,Citation88 and HPV.Citation25,Citation89 In addition, we incorporated HP-91 and HBD-3 in the final vaccine construct. The peptide of HP-91 derived from B-box domain amino acids of 89–108 from HMGB1 protein. This peptide induces high levels of IL-2 and IL-15, increases secretion of IL-12 and IFN-α in human DCs and augmented T cell activation.Citation90 Also, it activates DCs independent of TLR2, 4, and 9, and MyD88 pathways.Citation91 This is a potent immune adjuvant for inducing cellular and humoral immune responses and has been used in vaccines against viral infections such as HIV and HPV.Citation90–Citation93 HBD-3 is the third adjuvant in our vaccine, used as adjuvant to viruses such as influenza and MERS-CoV.Citation28,Citation94 This peptide blocks viral fusion using creating a protective barricade of immobilized surface proteins.Citation94 It activates APCs via TLR1 and TLR2Citation95 and induces IL-22,Citation96 TGF-αCitation97,Citation98 and IFN-γ.Citation99,Citation100 It has been implicated in the chemotaxis of immature DCs and T cells via its interaction with chemokine receptor 6 (CCR6) and the chemotaxis of monocytes via interaction with CCR2,Citation101 As well as this peptide activates natural killer (NK), promotes and activates myeloid DCs directly and dependent NK cells activity.Citation95,Citation100 To link different pieces of the vaccine, a repeated of a dipeptide linker of LE was used. This linker improves the expression of vaccine protein via the decreasing of a pI.Citation102,Citation103
An efficient vaccine should not only have stimulating ability immune response but also possess good physicochemical properties during production, formulation, storage, and consumption. According to the results of bioinformatic predictions, the designed vaccine was stable with a stability index of 36.41 and had a pI of 7.92. Conformational B-cell epitopes have a central role in induction humoral responses. The accessibility of a significant number of B-cell epitopes in the vaccine molecule indicates the high ability of this structure to stimulate B lymphocytes`. The binding affinity of the vaccine with the immune receptors (TLR3, 5, and 8) is necessary to effectively transport vaccine protein into antigen-presenting cells.
Moreover, it has been reported that TLR3 induces a strong type I IFN-dependent antiviral response in murine Coronavirus InfectionCitation104 and TLR8 has the inhibitory roles against viruses.Citation105,Citation106 These three TLRs were used in designing in previous bioinformatic-based vaccines against SARS-CoV-2.Citation30,Citation107,Citation108 The results of docking analysis showed the vaccine protein properly interacts with TLR3, 5, and 8. In addition, docking results of the vaccine and ACE-2 reveal the RBD epitopes of the vaccine can efficiently interact with ACE-2. This interaction indicates the vaccine will be attached to ACE-2 and therefore, induces humoral and cellular responses against SARS-CoV-2.
The codon optimization and in silico cloning illustrated the vaccine can efficiently transcript and translate in E. coli. Alignment of the selected epitopes of structural proteins showed these epitopes are conserved between SARS-CoV-2 and SARS, so it is also possible that the vaccine may induce neutralizing antibodies against SARS coronavirus.
Conclusion
In this study, using a variety of bioinformatic methods, we developed a multi-epitope subunit vaccine against the SARS-Cov-2 virus. The results of this study showed that it could be possible to predict vaccine candidates against new emerging viral diseases such as COVID-19 with the help of reverse vaccinology. The present study, with its effective vaccine design against SARS-CoV-2, showed that bioinformatic approaches could help to develop effective treatments for other emerging infectious diseases in a short time and at low cost. However, the in silico results of this study need to be verified using laboratory and animal models.
Abbreviations
ACE2, angiotensin-converting enzyme 2; ANN, artificial neural network; CAI, Codon Adaptation Index; CASP10, Critical assessment of techniques for protein structure prediction; CCR, C-C Motif Chemokine Receptor; CFD, Codon Frequency and Distribution; CombLib, Combinatorial Peptide Libraries; CTL, Cytotoxic T lymphocytes; DC, Dendritic cells; E, envelope; E. coli, Escherichia coli; h, hour; HBD-3, human beta-defensin 3; HMGB1, high mobility group box1; HLA, Human leukocyte antigen; KDa, Kilo Dalton; INF, Interferon; M, membrane; MHC, Major histocompatibility complex class; MW, molecular weight; N, nucleocapsid; NK, Natural killer; pI, isoelectric point; PSSMs, position specific scoring matrices; QM, Quantitative matrix; S, spike; SARS-CoV-2, severe acute respiratory syndrome coronavirus 2; SMM, stabilized matrix method; SVM, support vector machine; Th, T helper; TLR, Toll-Like Receptor; 3D, Three dimensional.
Ethics Approval and Consent to Participate
The protocol of this study approved by the Research Ethics committee of Mazandaran University of Medical Sciences IR.MAZUMS.REC.1398.1430.
Author Contributions
A.R conceived and designed this study; supervised the project and collected the data. Z.Y and M.Y contributed to performing the analysis and writing the draft. A.R and R.V edited the manuscript. All authors contributed to data analysis, drafting or revising the article, have agreed on the journal to which the article will be submitted, gave final approval of the version to be published, and agree to be accountable for all aspects of the work.
Disclosure
The authors report no conflicts of interest in this work.
References
- Huang C, Wang Y, Li X, et al. Clinical features of patients infected with 2019 novel coronavirus in Wuhan, China. Lancet. 2020;395(10223):497–506. doi:10.1016/S0140-6736(20)30183-531986264
- Wang C, Horby PW, Hayden FG, Gao GF. A novel coronavirus outbreak of global health concern. Lancet. 2020;395(10223):470–473. doi:10.1016/S0140-6736(20)30185-931986257
- Lu R, Zhao X, Li J, et al. Genomic characterisation and epidemiology of 2019 novel coronavirus: implications for virus origins and receptor binding. Lancet. 2020;395(10224):565–574. doi:10.1016/S0140-6736(20)30251-832007145
- Ahmed SF, Quadeer AA, McKay MR. Preliminary identification of potential vaccine targets for the COVID-19 coronavirus (SARS-CoV-2) based on SARS-CoV immunological studies. Viruses. 2020;12(3):254. doi:10.3390/v12030254
- Zhou P, Yang X-L, Wang X-G, et al. A pneumonia outbreak associated with a new coronavirus of probable bat origin. Nature. 2020;579(7798):270–3.32015507
- Stein RA. The 2019 Coronavirus: learning curves, lessons, and the weakest link. Int J Clin Pract. 2020;74:e13488.32052918
- Wan Y, Shang J, Graham R, Baric RS, Li F. Receptor recognition by novel coronavirus from Wuhan: an analysis based on decade-long structural studies of SARS. J. Virol. 2020;94. doi:10.1128/JVI.00127-20
- Li F. Structure, function, and evolution of coronavirus spike proteins. Annu Rev Virol. 2016;3:237–261. doi:10.1146/annurev-virology-110615-04230127578435
- Bosch BJ, van der Zee R, de Haan CA, Rottier PJ. The coronavirus spike protein is a class I virus fusion protein: structural and functional characterization of the fusion core complex. J Virol. 2003;77(16):8801–8811. doi:10.1128/JVI.77.16.8801-8811.200312885899
- Wrapp D, Wang N, Corbett KS, et al. Cryo-EM structure of the 2019-nCoV spike in the prefusion conformation. Science. 2020;367(6483):1260–1263. doi:10.1126/science.abb250732075877
- Nelson GW, Stohlman SA, Tahara SM. High affinity interaction between nucleocapsid protein and leader/intergenic sequence of mouse hepatitis virus RNA. J Gen Virol. 2000;81(1):181–188. doi:10.1099/0022-1317-81-1-18110640556
- Stohlman S, Baric R, Nelson G, Soe L, Welter L, Deans R. Specific interaction between coronavirus leader RNA and nucleocapsid protein. J Virol. 1988;62(11):4288–4295. doi:10.1128/JVI.62.11.4288-4295.19882845141
- Cong Y, Ulasli M, Schepers H, et al. Nucleocapsid protein recruitment to replication-transcription complexes plays a crucial role in coronaviral life cycle. J Virol. 2020;94(4).
- Kang S, Yang M, Hong Z, et al. Crystal structure of SARS-CoV-2 nucleocapsid protein RNA binding domain reveals potential unique drug targeting sites. bioRxiv. 2020.
- Li CK-F, Wu H, Yan H, et al. T cell responses to whole SARS coronavirus in humans. J Immunol. 2008;181(8):5490–5500. doi:10.4049/jimmunol.181.8.549018832706
- Westerbeck JW, Machamer CE. The infectious bronchitis coronavirus envelope protein alters Golgi pH to protect the spike protein and promote the release of infectious virus. J Virol. 2019;93(11):e00015–00019. doi:10.1128/JVI.00015-1930867314
- Gupta MK, Vemula S, Donde R, Gouda G, Behera L, Vadde R. In-silico approaches to detect inhibitors of the human severe acute respiratory syndrome coronavirus envelope protein ion channel. J Biomol Struct Dyn. 2020;1–17.
- Song Z, Yang Y, Wang L, et al. EIF4A2 interacts with the membrane protein of transmissible gastroenteritis coronavirus and plays a role in virus replication. Res Vet Sci. 2019;123:39–46. doi:10.1016/j.rvsc.2018.12.00530583231
- Control ECfDPa. COVID-19; 2020 Available from: http://mdanderson.libanswers.com/faq/26219. AccessedJuly 21,2020.
- Pang J, Wang MX, Ang IYH, et al. Potential rapid diagnostics, vaccine and therapeutics for 2019 novel coronavirus (2019-nCoV): a systematic review. J Clin Med. 2020;9(3):623. doi:10.3390/jcm9030623
- ul Qamar MT, Alqahtani SM, Alamri MA, Chen -L-L. Structural basis of SARS-CoV-2 3CLpro and anti-COVID-19 drug discovery from medicinal plants. J Pharm Anal. 2020.
- Robson B. Computers and viral diseases. Preliminary bioinformatics studies on the design of a synthetic vaccine and a preventative peptidomimetic antagonist against the SARS-CoV-2 (2019-nCoV, COVID-19) coronavirus. Comput Biol Med. 2020;103670.32209231
- Amanat F, Krammer F. SARS-CoV-2 vaccines: status report. Immunity. 2020;52(4):583–589. doi:10.1016/j.immuni.2020.03.00732259480
- Prompetchara E, Ketloy C, Palaga T. Immune responses in COVID-19 and potential vaccines: lessons learned from SARS and MERS epidemic. Asian Pac J Allergy Immunol. 2020;38(1):1–9. doi:10.12932/AP-200220-077232105090
- Yazdani Z, Rafiei A, Valadan R, Ashrafi H, Pasandi M, Kardan M. Designing a potent L1 protein-based HPV peptide vaccine; a bioinformatics approach. Comput Biol Chem. 2020;85:107209. doi:10.1016/j.compbiolchem.2020.10720932120301
- Ahmad B, Ashfaq UA, Rahman M-U, Masoud MS, Yousaf MZ. Conserved B and T cell epitopes prediction of ebola virus glycoprotein for vaccine development: an immuno-informatics approach. Microb Pathog. 2019;132:243–253. doi:10.1016/j.micpath.2019.05.01031075428
- Shahid F, Ashfaq UA, Javaid A, Khalid H. Immunoinformatics guided rational design of a next generation multi epitope based peptide (MEBP) vaccine by exploring Zika virus proteome. Infect Genet Evol. 2020;80:104199. doi:10.1016/j.meegid.2020.10419931962160
- Srivastava S, Kamthania M, Singh S, Saxena AK, Sharma N. Structural basis of development of multi-epitope vaccine against middle east respiratory syndrome using in silico approach. Infect Drug Resist. 2018;11:2377. doi:10.2147/IDR.S17511430538505
- Ul Qamar MT, Saleem S, Ashfaq UA, Bari A, Anwar F, Alqahtani S. Epitope‐based peptide vaccine design and target site depiction against Middle East Respiratory Syndrome Coronavirus: an immune-informatics study. J Transl Med. 2019;17(1):362. doi:10.1186/s12967-019-2116-831703698
- Ul Qamar MT, Rehman A, Ashfaq UA, et al. Designing of a next generation multiepitope based vaccine (MEV) against SARS-COV-2: immunoinformatics and in silico approaches. BioRxiv. 2020.
- Behbahani M. In silico Design of novel Multi-epitope recombinant Vaccine based on Coronavirus surface glycoprotein. BioRxiv. 2020.
- Kalita P, Padhi A, Zhang KY, Tripathi T. Design of a peptide-based subunit vaccine against novel coronavirus SARS-CoV-2. Microb Pathog. 2020;145:104236. doi:10.1016/j.micpath.2020.10423632376359
- Zhang Q, Wang P, Kim Y, et al. Immune epitope database analysis resource (IEDB-AR). Nucleic Acids Res. 2008;36(suppl_2):W513–W518. doi:10.1093/nar/gkn25418515843
- Vita R, Mahajan S, Overton JA, et al. The immune epitope database (IEDB): 2018 update. Nucleic Acids Res. 2019;47(D1):D339–D343. doi:10.1093/nar/gky100630357391
- Andreatta M, Nielsen M. Gapped sequence alignment using artificial neural networks: application to the MHC class I system. Bioinformatics. 2016;32(4):511–517. doi:10.1093/bioinformatics/btv63926515819
- Lundegaard C, Lamberth K, Harndahl M, Buus S, Lund O, Nielsen M. NetMHC-3.0: accurate web accessible predictions of human, mouse and monkey MHC class I affinities for peptides of length 8–11. Nucleic Acids Res. 2008;36(suppl_2):W509–W512. doi:10.1093/nar/gkn20218463140
- Lundegaard C, Nielsen M, Lund O. The validity of predicted T-cell epitopes. Trends Biotechnol. 2006;24(12):537–538. doi:10.1016/j.tibtech.2006.10.00117045685
- Lundegaard C, Lund O, Nielsen M. Accurate approximation method for prediction of class I MHC affinities for peptides of length 8, 10 and 11 using prediction tools trained on 9mers. Bioinformatics. 2008;24(11):1397–1398. doi:10.1093/bioinformatics/btn12818413329
- Nielsen M, Lundegaard C, Worning P, et al. Reliable prediction of T‐cell epitopes using neural networks with novel sequence representations. Protein Sci. 2003;12(5):1007–1017. doi:10.1110/ps.023940312717023
- Buus S, Lauemøller S, Worning P, et al. Sensitive quantitative predictions of peptide‐MHC binding by a ‘Query by Committee’artificial neural network approach. Tissue Antigens. 2003;62(5):378–384. doi:10.1034/j.1399-0039.2003.00112.x14617044
- Peters B, Sette A. Generating quantitative models describing the sequence specificity of biological processes with the stabilized matrix method. BMC Bioinform. 2005;6(1):132. doi:10.1186/1471-2105-6-132
- Kim Y, Sidney J, Pinilla C, Sette A, Peters B. Derivation of an amino acid similarity matrix for peptide: MHC binding and its application as a Bayesian prior. BMC Bioinform. 2009;10(1):394. doi:10.1186/1471-2105-10-394
- Sidney J, Assarsson E, Moore C, et al. Quantitative peptide binding motifs for 19 human and mouse MHC class I molecules derived using positional scanning combinatorial peptide libraries. Immunome Res. 2008;4(1):2. doi:10.1186/1745-7580-4-218221540
- Moutaftsi M, Peters B, Pasquetto V, et al. A consensus epitope prediction approach identifies the breadth of murine T CD8+-cell responses to vaccinia virus. Nat Biotechnol. 2006;24(7):817–819. doi:10.1038/nbt121516767078
- Jurtz V, Paul S, Andreatta M, Marcatili P, Peters B, Nielsen M. NetMHCpan-4.0: improved peptide–MHC class I interaction predictions integrating eluted ligand and peptide binding affinity data. J Immunol. 2017;199(9):3360–3368. doi:10.4049/jimmunol.170089328978689
- Nielsen M, Andreatta M. NetMHCpan-3.0; improved prediction of binding to MHC class I molecules integrating information from multiple receptor and peptide length datasets. Genome Med. 2016;8(1):33. doi:10.1186/s13073-016-0288-x27029192
- Hoof I, Peters B, Sidney J, et al. NetMHCpan, a method for MHC class I binding prediction beyond humans. Immunogenetics. 2009;61(1):1. doi:10.1007/s00251-008-0341-z19002680
- Nielsen M, Lundegaard C, Blicher T, et al. NetMHCpan, a method for quantitative predictions of peptide binding to any HLA-A and-B locus protein of known sequence. PLoS One. 2007;2(8):e796. doi:10.1371/journal.pone.000079617726526
- Karosiene E, Lundegaard C, Lund O, Nielsen M. NetMHCcons: a consensus method for the major histocompatibility complex class I predictions. Immunogenetics. 2012;64(3):177–186. doi:10.1007/s00251-011-0579-822009319
- Zhang H, Lund O, Nielsen M. The PickPocket method for predicting binding specificities for receptors based on receptor pocket similarities: application to MHC-peptide binding. Bioinformatics. 2009;25(10):1293–1299. doi:10.1093/bioinformatics/btp13719297351
- Rasmussen M, Fenoy E, Harndahl M, et al. Pan-specific prediction of peptide–MHC class I complex stability, a correlate of T cell immunogenicity. J Immunol. 2016;197(4):1517–1524. doi:10.4049/jimmunol.160058227402703
- Bhasin M, Raghava G. Prediction of CTL epitopes using QM, SVM and ANN techniques. Vaccine. 2004;22(23–24):3195–3204. doi:10.1016/j.vaccine.2004.02.00515297074
- Nielsen M, Lund O. NN-align. An artificial neural network-based alignment algorithm for MHC class II peptide binding prediction. BMC Bioinform. 2009;10(1):296. doi:10.1186/1471-2105-10-296
- Nielsen M, Lundegaard C, Lund O. Prediction of MHC class II binding affinity using SMM-align, a novel stabilization matrix alignment method. BMC Bioinform. 2007;8(1):238. doi:10.1186/1471-2105-8-238
- Sturniolo T, Bono E, Ding J, et al. Generation of tissue-specific and promiscuous HLA ligand databases using DNA microarrays and virtual HLA class II matrices. Nat Biotechnol. 1999;17(6):555–561. doi:10.1038/985810385319
- Andreatta M, Karosiene E, Rasmussen M, Stryhn A, Buus S, Nielsen M. Accurate pan-specific prediction of peptide-MHC class II binding affinity with improved binding core identification. Immunogenetics. 2015;67(11–12):641–650. doi:10.1007/s00251-015-0873-y26416257
- Reche PA, Glutting J-P, Zhang H, Reinherz EL. Enhancement to the RANKPEP resource for the prediction of peptide binding to MHC molecules using profiles. Immunogenetics. 2004;56(6):405–419. doi:10.1007/s00251-004-0709-715349703
- Emini EA, Hughes JV, Perlow D, Boger J. Induction of hepatitis A virus-neutralizing antibody by a virus-specific synthetic peptide. J Virol. 1985;55(3):836–839. doi:10.1128/JVI.55.3.836-839.19852991600
- Jespersen MC, Peters B, Nielsen M, Marcatili P. BepiPred-2.0: improving sequence-based B-cell epitope prediction using conformational epitopes. Nucleic Acids Res. 2017;45(W1):W24–W29. doi:10.1093/nar/gkx34628472356
- Sarwar MA, Rehman A, Ferzund J. Database Search, Alignment Viewer and Genomics Analysis Tools: big Data for Bioinformatics. Int J Comput Sci Netw. 2016;14(12):317.
- Dhanda SK, Vir P, Raghava GP. Designing of interferon-gamma inducing MHC class-II binders. Biol Direct. 2013;8(1):30. doi:10.1186/1745-6150-8-3024304645
- Magnan CN, Baldi P. SSpro/ACCpro 5: almost perfect prediction of protein secondary structure and relative solvent accessibility using profiles, machine learning and structural similarity. Bioinformatics. 2014;30(18):2592–2597. doi:10.1093/bioinformatics/btu35224860169
- Agostini F, Cirillo D, Livi CM, Ponti RD, Tartaglia GG. ccSOL omics: a webserver for large-scale prediction of endogenous and heterologous solubility in E. coli. Bioinformatics. 2014;30(20):2975–2977. doi:10.1093/bioinformatics/btu42024990610
- Doytchinova IA, Flower DR. Identifying candidate subunit vaccines using an alignment-independent method based on principal amino acid properties. Vaccine. 2007;25(5):856–866. doi:10.1016/j.vaccine.2006.09.03217045707
- Gasteiger E, Hoogland C, Gattiker A, Wilkins MR, Appel RD, Bairoch A. Protein identification and analysis tools on the ExPASy server In: Walker J.M. (eds) The Proteomics Protocols Handbook. Springer; 2005:571–607.
- Kelley LA, Mezulis S, Yates CM, Wass MN, Sternberg MJ. The Phyre2 web portal for protein modeling, prediction and analysis. Nat Protoc. 2015;10(6):845. doi:10.1038/nprot.2015.05325950237
- Ko J, Park H, Heo L, Seok C. GalaxyWEB server for protein structure prediction and refinement. Nucleic Acids Res. 2012;40(W1):W294–W297. doi:10.1093/nar/gks49322649060
- Lovell SC, Davis IW, Arendall WB III, et al. Structure validation by Cα geometry: ϕ, ψ and Cβ deviation. Proteins. 2003;50(3):437–450. doi:10.1002/prot.1028612557186
- Colovos C, Yeates TO. Verification of protein structures: patterns of nonbonded atomic interactions. Protein Sci. 1993;2(9):1511–1519. doi:10.1002/pro.55600209168401235
- Lill MA, Danielson ML. Computer-aided drug design platform using PyMOL. J Comput Aided Mol. 2011;25(1):13–19. doi:10.1007/s10822-010-9395-8
- Ponomarenko J, Bui -H-H, Li W, et al. ElliPro: a new structure-based tool for the prediction of antibody epitopes. BMC Bioinform. 2008;9(1):514. doi:10.1186/1471-2105-9-514
- Vajda S, Yueh C, Beglov D, et al. New additions to the ClusPro server motivated by CAPRI. Proteins. 2017;85(3):435–444. doi:10.1002/prot.2521927936493
- Kozakov D, Hall DR, Xia B, et al. The ClusPro web server for protein–protein docking. Nat Protoc. 2017;12(2):255. doi:10.1038/nprot.2016.16928079879
- Kozakov D, Beglov D, Bohnuud T, et al. How good is automated protein docking? Proteins. 2013;81(12):2159–2166. doi:10.1002/prot.2440323996272
- Mathews DH, Disney MD, Childs JL, Schroeder SJ, Zuker M, Turner DH. Incorporating chemical modification constraints into a dynamic programming algorithm for prediction of RNA secondary structure. Proc Natl Acad Sci. 2004;101(19):7287–7292. doi:10.1073/pnas.040179910115123812
- Mizel SB, Honko AN, Moors MA, Smith PS, West AP. Induction of macrophage nitric oxide production by Gram-negative flagellin involves signaling via heteromeric Toll-like receptor 5/Toll-like receptor 4 complexes. J Immunol. 2003;170(12):6217–6223. doi:10.4049/jimmunol.170.12.621712794153
- Moors MA, Li L, Mizel SB. Activation of interleukin-1 receptor-associated kinase by gram-negative flagellin. Infect Immun. 2001;69(7):4424–4429. doi:10.1128/IAI.69.7.4424-4429.200111401982
- Didierlaurent A, Ferrero I, Otten LA, et al. Flagellin promotes myeloid differentiation factor 88-dependent development of Th2-type response. J Immunol. 2004;172(11):6922–6930. doi:10.4049/jimmunol.172.11.692215153511
- Lee SE, Kim SY, Jeong BC, et al. A bacterial flagellin, Vibrio vulnificus FlaB, has a strong mucosal adjuvant activity to induce protective immunity. Infect Immun. 2006;74(1):694–702. doi:10.1128/IAI.74.1.694-702.200616369026
- Han S, Yang K, Ozen Z, et al. Enhanced differentiation of splenic plasma cells but diminished long-lived high-affinity bone marrow plasma cells in aged mice. J Immunol. 2003;170(3):1267–1273. doi:10.4049/jimmunol.170.3.126712538685
- Pino O, Martin M, Michalek SM. Cellular mechanisms of the adjuvant activity of the flagellin component FljB of Salmonella enterica Serovar Typhimurium to potentiate mucosal and systemic responses. Infect Immun. 2005;73(10):6763–6770. doi:10.1128/IAI.73.10.6763-6770.200516177354
- Honko AN, Mizel SB. Mucosal administration of flagellin induces innate immunity in the mouse lung. Infect Immun. 2004;72(11):6676–6679. doi:10.1128/IAI.72.11.6676-6679.200415501801
- Cunningham AF, Khan M, Ball J, et al. Responses to the soluble flagellar protein FliC are Th2, while those to FliC on Salmonella are Th1. Eur J Immunol. 2004;34(11):2986–2995. doi:10.1002/eji.20042540315384042
- McSorley SJ, Ehst BD, Yu Y, Gewirtz AT. Bacterial flagellin is an effective adjuvant for CD4+ T cells in vivo. J Immunol. 2002;169(7):3914–3919. doi:10.4049/jimmunol.169.7.391412244190
- Wang B-Z, Quan F-S, Kang S-M, Bozja J, Skountzou I, Compans RW. Incorporation of membrane-anchored flagellin into influenza virus-like particles enhances the breadth of immune responses. J Virol. 2008;82(23):11813–11823. doi:10.1128/JVI.01076-0818786995
- López-Boado YS, Cobb LM, Deora R. Bordetella bronchiseptica flagellin is a proinflammatory determinant for airway epithelial cells. Infect Immun. 2005;73(11):7525–7534. doi:10.1128/IAI.73.11.7525-7534.200516239555
- Huleatt JW, Nakaar V, Desai P, et al. Potent immunogenicity and efficacy of a universal influenza vaccine candidate comprising a recombinant fusion protein linking influenza M2e to the TLR5 ligand flagellin. Vaccine. 2008;26(2):201–214. doi:10.1016/j.vaccine.2007.10.06218063235
- Skountzou I, Del Pilar Martin M, Wang B, et al. Salmonella flagellins are potent adjuvants for intranasally administered whole inactivated influenza vaccine. Vaccine. 2010;28(24):4103–4112. doi:10.1016/j.vaccine.2009.07.05819654062
- Negahdaripour M, Eslami M, Nezafat N, et al. A novel HPV prophylactic peptide vaccine, designed by immunoinformatics and structural vaccinology approaches. Infect Genet Evol. 2017;54:402–416. doi:10.1016/j.meegid.2017.08.00228780192
- Telusma G, Datta S, Mihajlov I, et al. Dendritic cell activating peptides induce distinct cytokine profiles. Int Immunol. 2006;18(11):1563–1573. doi:10.1093/intimm/dxl08916966494
- Saenz R, Futalan D, Leutenez L, et al. TLR4-dependent activation of dendritic cells by an HMGB1-derived peptide adjuvant. J Transl Med. 2014;12(1):211. doi:10.1186/1479-5876-12-21125123824
- Talebi S, Bolhassani A, Sadat SM, Vahabpour R, Agi E, Shahbazi S. Hp91 immunoadjuvant: an HMGB1-derived peptide for development of therapeutic HPV vaccines. Biomed Pharmacother. 2017;85:148–154. doi:10.1016/j.biopha.2016.11.11527930979
- Milani A, Bolhassani A, Shahbazi S, Motevalli F, Sadat SM, Soleymani S. Small heat shock protein 27: an effective adjuvant for enhancement of HIV-1 Nef antigen-specific immunity. Immunol Lett. 2017;191:16–22. doi:10.1016/j.imlet.2017.09.00528917624
- Leikina E, Delanoe-Ayari H, Melikov K, et al. Carbohydrate-binding molecules inhibit viral fusion and entry by crosslinking membrane glycoproteins. Nat Immunol. 2005;6(10):995–1001. doi:10.1038/ni124816155572
- Funderburg N, Lederman MM, Feng Z, et al. Human β-defensin-3 activates professional antigen-presenting cells via Toll-like receptors 1 and 2. Proc Natl Acad Sci. 2007;104(47):18631–18635. doi:10.1073/pnas.070213010418006661
- Wolk K, Kunz S, Witte E, Friedrich M, Asadullah K, Sabat R. IL-22 increases the innate immunity of tissues. Immunity. 2004;21(2):241–254. doi:10.1016/j.immuni.2004.07.00715308104
- Sørensen OE, Cowland JB, Theilgaard-Mönch K, Liu L, Ganz T, Borregaard N. Wound healing and expression of antimicrobial peptides/polypeptides in human keratinocytes, a consequence of common growth factors. J Immunol. 2003;170(11):5583–5589.12759437
- Ferris LK, Mburu YK, Mathers AR, et al. Human beta-defensin 3 induces maturation of human Langerhans cell–like dendritic cells: an antimicrobial peptide that functions as an endogenous adjuvant. J Invest Dermatol. 2013;133(2):460–468. doi:10.1038/jid.2012.31922951718
- Joly S, Organ CC, Johnson GK, McCray PB Jr, Guthmiller JM. Correlation between β-defensin expression and induction profiles in gingival keratinocytes. Mol Immunol. 2005;42(9):1073–1084.15829297
- Judge CJ, Reyes-Aviles E, Conry SJ, et al. HBD-3 induces NK cell activation, IFN-γ secretion and mDC dependent cytolytic function. Cell Immunol. 2015;297(2):61–68. doi:10.1016/j.cellimm.2015.06.00426302933
- Röhrl J, Yang D, Oppenheim JJ, Hehlgans T. Human β-defensin 2 and 3 and their mouse orthologs induce chemotaxis through interaction with CCR2. J Immunol. 2010;184(12):6688–6694. doi:10.4049/jimmunol.090398420483750
- Chen X, Zaro JL, Shen W-C. Fusion protein linkers: property, design and functionality. Adv Drug Deliv Rev. 2013;65(10):1357–1369. doi:10.1016/j.addr.2012.09.03923026637
- Chen X, Lee H-F, Zaro JL, Shen W-C. Effects of receptor binding on plasma half-life of bifunctional transferrin fusion proteins. Mol Pharm. 2011;8(2):457–465. doi:10.1021/mp100306421291258
- Mazaleuskaya L, Veltrop R, Ikpeze N, Martin-Garcia J, Navas-Martin S. Protective role of Toll-like receptor 3-induced type I interferon in murine coronavirus infection of macrophages. Viruses. 2012;4(5):901–923. doi:10.3390/v405090122754655
- Campbell GR, Spector SA. Toll-like receptor 8 ligands activate a vitamin D mediated autophagic response that inhibits human immunodeficiency virus type 1. PLoS Pathog. 2012;8(11):e1003017. doi:10.1371/journal.ppat.100301723166493
- Hammerbeck DM, Burleson GR, Schuller CJ, et al. Administration of a dual toll-like receptor 7 and toll-like receptor 8 agonist protects against influenza in rats. Antiviral Res. 2007;73(1):1–11. doi:10.1016/j.antiviral.2006.07.01116959331
- Bhatnager R, Bhasin M, Arora J, Dang AS. Epitope based peptide vaccine against SARS-COV2: an immune-informatics approach. J Biomol Struct Dyn. 2020;1–16. doi:10.1080/07391102.2020.1787227
- Bhattacharya M, Sharma AR, Patra P, et al. Development of epitope‐based peptide vaccine against novel coronavirus 2019 (SARS‐COV‐2): immunoinformatics approach. J Med Virol. 2020;92(6):618–631. doi:10.1002/jmv.2573632108359