Abstract
Purpose
This study aimed to provide new biomarkers for predicting the disease course of COVID-19 by analyzing the dynamic changes of microRNA (miRNA) and its target gene expression in the serum of COVID-19 patients at different stages.
Methods
Serum samples were collected from all COVID-19 patients at three time points: the acute stage, the turn-negative stage, and the recovery stage. The expression level of miRNA and the target mRNA was measured by Quantitative Real-Time Polymerase Chain Reaction (RT-qPCR). The classification tree model was established to predict the disease course, and the prediction efficiency of independent variables in the model was analyzed using the receiver operating characteristic (ROC) curve.
Results
The expression of miR-125b-5p and miR-155-5p was significantly up-regulated in the acute stage and gradually decreased in the turn-negative and recovery stages. The expression of the target genes CDH5, STAT3, and TRIM32 gradually down-regulated in the acute, turn-negative, and recovery stages. MiR-125b-5p, miR-155-5p, STAT3, and TRIM32 constituted a classification tree model with 100% accuracy of prediction and AUC >0.7 for identification and prediction in all stages.
Conclusion
MiR-125b-5p, miR-155-5p, STAT3, and TRIM32 could be useful biomarkers to predict the time nodes of the acute, turn-negative, and recovery stages of COVID-19.
Introduction
Severe acute respiratory syndrome coronavirus 2 (SARS-CoV-2), also known as 2019-nCoV, has been identified as the pathogen that causes coronavirus disease 2019 (COVID-19).Citation1 As of February 1, 2022, the COVID-19 pandemic caused by SARS-CoV-2 infection has led to more than 370 million confirmed cases and 5.6 million deaths worldwide.Citation2 Some patients with COVID-19 exhibit mild clinical manifestations, such as fever, dry cough, headache, and muscle soreness, while others experience serious complications, such as dyspnea, acute respiratory distress syndrome, and the cytokine storm, which may lead to death.Citation3 COVID-19 patients have a different course of disease due to individual differences, and the disease trend cannot be evaluated. Accurate course prediction is helpful for clinicians to formulate reasonable treatment plans. Nucleic acid detection is the “gold standard” for the diagnosis of COVID-19, but this method is only a qualitative analysis and cannot dynamically show the progress of COVID-19 patients. At present, there is a lack of reasonable biomarkers to dynamically display and predict the disease development of COVID-19 patients. It is very necessary for clinical patients and doctors to find appropriate biomarkers.
MicroRNA (miRNA) is a type of non-coding RNA with a length of 17–25 nucleotides, which plays an important role in gene expression in organisms. miRNAs exist widely in biological body fluids, such as in serum, plasma, urine, saliva, and cerebrospinal fluid.Citation4 miRNA directly affects the cellular stability of messenger RNA, thus forming complex networks that regulate transcriptional genes, cell proliferation, differentiation, apoptosis, homeostasis, and the stress response.Citation5 Some studies suggest that in COVID-19 disease, dysregulated miRNAs are related to virus replication, the proliferation of infected cells, immune response, and inflammation.Citation6–8 Some studies have compared miRNA expression in patients with COVID-19 with miRNA expression in healthy people to provide potential diagnostic biomarkers;Citation9,Citation10 Moreover, some studies have analyzed the expression of miRNAs in patients with COVID-19 of different severities, which is helpful for the diagnosis and prediction of COVID-19;Citation11,Citation12 However, few studies have analyzed the expression levels of miRNA and its target genes in different stages of COVID-19.
This study focused on the disease course staging of COVID-19 patients. We conducted an extensive reading of articles on miRNA assays in COVID-19 before the study was conducted and identified hundreds of miRNAs that are differentially expressed in COVID-19 patients. Considering that the sample size required for the method of this study could not be measured in its totality, 22 miRNAs that are stimulated earlier in the immune activation system or are associated with viral replication, T cell or B cell differentiation, development and maturation, targeting of macrophages, and regulation of cytokine and chemokine synthesis in the inflammatory process were selected for this study.Citation13–24 Our study included COVID-19 patients and healthy people in the same period. The serum of all COVID-19 patients in the acute stage, the turn-negative stage, and the recovery stage were collected continuously. The dynamic changes of miRNA and its target mRNA expression at different stages of COVID-19 course were tracked. Based on this, we screened biomarkers that could distinguish the disease course and constructed a disease course prediction model based on the results, expecting to provide new biomarkers for the prediction of COVID-19 disease course.
Materials and Methods
Research Objects
The study population was drawn from 16 patients with COVID-19 admitted to Changchun Infectious Disease Hospital and Changchun Hospital of Traditional Chinese Medicine from January 2021 to March 2021 and 16 health check-ups at the First Hospital of Jilin University during the same period. According to the Protocol for the Treatment of COVID-19 in the People’s Republic of China (Trial Version 9), all 16 COVID-19 patients were categorized as common type (Clinical manifestations such as fever or respiratory symptoms and pneumonia visible on imaging) and exclusion criteria: (a) malignant tumors and malignant hematological diseases (b) severe complications (c) autoimmune diseases (d) diabetes mellitus. Matching healthy checkers with COVID-19 patients according to age and sex, exclusion criteria: (a) chronic digestive system diseases (b) routine physical examination exceeds the upper or lower limit of the reference value (Blood count, urine count, coagulation count, liver and kidney function tests, chest computed tomography (CT) scan). The blood specimens were collected from healthy checkers and three stages of each COVID-19 patient: (a) the acute stage samples were collected from the peripheral blood of COVID-19 patients within 3 days after the diagnosis of SARS-CoV-2 infection by RT-PCR (1~3 days after the onset of symptoms); (b) the turn-negative stage samples were collected from the peripheral blood of COVID-19 patients within 3 days after the conversion to negative by RT-PCR (Return of body temperature to normal for more than 3 days, significant improvement in respiratory symptoms, and significant improvement in acute exudative lesions on lung imaging); (c) the recovery stage samples were collected from peripheral blood of COVID-19 patients 14–16 days after discharge from the hospital (three consecutive days of negative RT-PCR results to meet the discharge criteria) (normal body temperature, no significant respiratory symptoms, mild or no significant pulmonary imaging changes). The peripheral blood samples were centrifuged at 3000 g at 4°C for 10 min, and the unqualified samples such as fat blood and hemolysis were excluded, and the separated serum was stored at −80°C. Other laboratory data related to COVID-19 patients and healthy people were retrieved by Laboratory Information Management System.
Total RNA Extraction and Reverse Transcription
Total RNA was isolated and extracted from 200 μL of serum using the miRcute miRNA Isolation Kit (Tiangen Biochemical Technology Co. Ltd., Beijing, China). The concentration and purity of total RNA were measured by NanoDrop™ 2000C (Thermo, Waltham, USA). miRNA reverse transcription was performed using miRcute plus miRNA First-strand cDNA kit (Tiangen Biochemical Technology Co. Ltd.) with the total reaction system of 20µL (10µL Buffer, 2µL Mix, 8µL Total RNA) and the reaction program of 60 min at 42°C and 3 min at 95°C. mRNA reverse transcription was performed using MonScriptTM RTlll All-in-one Mix with dsDNase (Monad Biochemical Technology Co. Ltd, Wuhan, China) with the total reaction system of 20µL (1 µL dsDNase, 4 µL Mix, 8 µL Total RNA, 7 µL Nuclease-Free Water) and the reaction procedure was 2 min at 37°C, 15 min at 55°C and 5 min at 85°C. The instrument was 100TM Thermal Cycler (BIO-RAD, Singapore). All reverse transcription cDNAs were placed at −20°C for use.
Quantitative Real-Time Polymerase Chain Reaction (RT-qPCR) Detection of miRNA and Target mRNA
The miRcute Plus miRNA qPCR Kit (SYBR Green) (Tiangen Biochemical Technology Co. Ltd.) was used to detect the expression level of miRNA. The primers for U6 and miRNAs were purchased from Tiangen Biochemical Technology Co., Ltd and the primer sequences are shown in Supplementary Table 1. U6 was used as an endogenous control gene to normalize the expression levels of the studied miRNAs. The expression levels of U6 were not statistically different between all groups in this study (P=0.271) (Supplementary Table 2). The total reaction volume was 20 μL (10µL 2×miRcute Plus miRNA Premix, 0.4µL Forward Primer, 0.4µL Reverse Primer, 0.4µL cDNA, 8.8µL Nuclease-Free Water) and the reaction procedure was 15 min at 95°C for 1 cycle, 20 sec at 94 °C and 34 sec at 60 °C for 40 cycles.
The MonAmpTM ChemoHS qPCR Mix (SYBR Green) (Monad Biochemical Technology Co. Ltd) was used to detect the expression level of mRNA. The primers of β- Actin and mRNAs were purchased from Sangon Bioengineering Co. Ltd (Shanghai, China) and the primer sequences are shown in Supplementary Table 1. Normalize the expression levels of the studied mRNAs. The total reaction volume was 20 μL (10µL qPCR mix, 0.4µL Forward Primer, 0.4µL Reverse Primer, 0.4µL cDNA, 0.2µL Low ROX Dye, 8.6µL Nuclease-Free Water) and the reaction procedure was 10 min at 95°C for 1 cycle, 10 sec at 95 °C and 30 sec at 60 °C for 40 cycles.
The RT-qPCR testing instrument was Agilent Mx3005p (Stratagene, California, America), which has undergone strict quality control tests before testing. The specificity of RT-qPCR was confirmed by melting curve analysis, undetectable data were assigned a default threshold cycle value (Ct) of 35. In the course of the experiment, three complex holes were set up in each sample. Relative expression of target gene =.Citation25
Target Gene Prediction
This study used the miRWalk database (which is a comprehensive database that can cover all three databases, TargetScan, miRDB, and miRTarBase) to perform target gene prediction for miRNAs with statistically different differences in each disease course, and the target score for prediction was set at 1. Since the number of target genes detectable using RT-qPCR was limited in this study, inflammatory genes or target mRNAs associated with viral replication, regulation of inflammatory cell function, macrophage polarization, and apoptosis were selected in this study based on the literature.Citation26–37
Statistical Analysis
GraphPad Prism 8.0 and MedCalc 20.0 were used for plotting, and SPSS 22.0 was used for data statistics and classification tree modeling. Four periods were used as dependent variables of the classification tree, and the expression levels of differentially expressed genes in each period were used as independent variables of the classification tree, and the growth method was CRT. 5-fold cross-validation was used for internal validation to evaluate the performance of the model. The process was repeated 5 times and the final performance of the internal validation was averaged over the 5 cross-validations. After preprocessing the RT-qPCR data, the Kolmogorov–Smirnov test and the Shapiro–Wilk test were used to test the normality. The independent-samples t-test or the Mann–Whitney U-test was used to compare the miRNA and mRNA expression between COVID-19 group and the healthy control group. In addition, the paired-samples t-test or Wilcoxon’s signed-rank test was used to compare miRNA and mRNA expression between the COVID-19 groups. The relationship between differentially expressed miRNAs, mRNA and clinical parameters was analyzed by Spearman correlation coefficient. The predictive efficacy of miRNA and mRNA as biomarkers for predicting the course of COVID-19 was measured by receiver operating characteristic (ROC) curve.
Results
Inclusion Characteristics
A total of 16 COVID-19 patients and 16 healthy subjects were included in this study. There was no significant difference in gender and age between COVID-19 patients and healthy people (P>0.05). In the initial stage of COVID-19 patient’s infection, 62.5% of patients will have symptoms of Fever, Headache, and Chills, 50% of patients will have symptoms of Cough, and a few people will have symptoms of Confusion, Chest pain, and Dyspnea, more information can be found in .
Table 1 Clinical and Demographical Characteristics of the Study Participants
Dynamic Changes of miRNAs Expression in Different Stages of COVID-19 Disease
MiR-21-3p, miR-451a, and miR-486-5p had Ct ≥ 35 in more than 80% of samples from COVID-19 patients and healthy individuals and therefore were not analyzed further. There was no significant difference in the expression of miR-16-5p, miR-24-3p, miR-27a-3p, miR-27b-3p, miR-125a-5p, miR-142-5p, miR-146a-3p, miR-148a-3p, miR-148a-5p, miR-181a-5p, miR-221-3p, miR-222-3p, or miR-223-3p among four groups (P>0.05) (). The expression of miR-29a-3p and miR-92a-3p in the acute stage of COVID-19 was significantly increased than those in healthy controls (P<0.05); the expression of miR-142-3p and miR-150-5p in the acute stage of COVID-19 was significantly decreased than those in healthy controls (P<0.05); the expression level of miR-125b-5p and miR-155-5p was the highest in the acute stage of COVID-19 and decreased gradually in the turn-negative stage and the recovery stage and the differences in expression levels between each stage were statistically significant (P<0.05) ().
Figure 1 miRNAs and mRNAs with no statistically significant differences in the expression levels in different groups. The horizontal line in the middle of the figure represents the median, and the horizontal lines at both ends are Q25 and Q75. (A–C) miRNAs with no statistically significant differences in the expression levels in different groups. (D) mRNAs with no statistically significant differences in the expression levels in different groups.
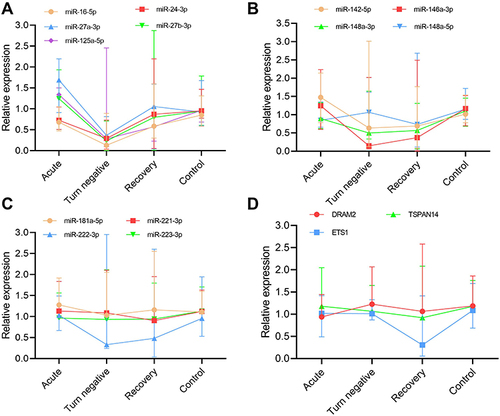
Prediction and Expression of Target Genes of miR-125b-5p and miR-155-5p
After detecting and analyzing the expression levels of 22 miRNAs selected in advance, only miR-125b-5p and miR-155-5 had statistically significant differences among each stage of COVID-19 (P < 0.05). Therefore, this study only predicted the target mRNA of those two miRNAs. The target genes finally determined for miR-125b-5p were BCL2, CDH5, DRAM2, ETS1, LIFR, STAT3, SUV39H1, TET2 and miR-155-5p were MSI2, TRIM32, TSPAN14, AAK1.
AAK1, MSI2, and SUV39H1 had Ct ≥ 35 in more than 80% of samples from COVID-19 patients and healthy individuals and therefore were not analyzed further. There was no significant difference in the expression of DRAM2, ETS1, and TSPAN14 among the four groups (P>0.05) (). The expression of BCL2 and LIFR in the turn-negative stage and the recovery stage of COVID-19 was significantly decreased than those in healthy controls (P<0.05); the expression of TET2 in the acute stage and the recovery stage of COVID-19 was significantly decreased than those in healthy controls (P<0.05); the expression level of CDH5, STAT3, and TRIM32 was decreased gradually in the acute stage, the turn-negative stage and the recovery stage, and the differences in expression levels between each stage were statistically significant (P<0.05) ().
Correlation Analysis of miRNA, mRNA and Laboratory Parameters
In this study, the correlation between miR-125b-5p, miR-155-5p, CDH5, STAT3, and TRIM32 of COVID-19 patients in three periods and laboratory parameters in corresponding periods was analyzed. Firstly, there was a significant correlation between miR-125b-5p and target genes CDH5, STAT3, miR-155-5p and target genes TRIM32 (P < 0.01). MiR-125b-5p, miR-155-5p, CDH5, STAT3, and TRIM32 were positively correlated with ALP, WBC, NE, CREA, IBIL, and TBIL (P < 0.05), and negatively correlated with PLT and LY (P < 0.05) ().
Figure 4 Correlations between differential expression miRNA-mRNA and laboratory parameters. *P < 0.05, **P < 0.01, -: Not analyzed.
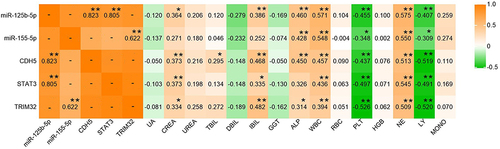
Establishment of the Classification Tree Model
A classification tree model for predicting the course of COVID-19 was constructed with miR-125b-5p, miR-155-5p, CDH5, STAT3, and TRIM32 as independent variables, and three disease courses of COVID-19 and healthy individuals as dependent variables, with the growth method CAT. The final independent variables incorporated into the model were miR-125b-5p, miR-155-5p, STAT3, and TRIM32, and the accuracy of the model’s predictions was 100% (). The classification tree model includes 5 layers, 11 ordinary nodes, and 6 terminal nodes (). The AUC values of the models in the five validation sets were 0.873, 0.880, 0.878, 0.884, and 0.866, respectively, with a mean AUC of 0.876. The results indicated that the classification tree model was able to reliably distinguish the different disease courses.
Table 2 Accuracy Evaluation of Classification Tree Model
ROC Curve Analysis
In order to evaluate the predictive efficacy of the expression levels of miR-125b-5p, mir-155-5p, STAT3, and TRIM32 as markers for predicting the course of COVID-19 in the model, ROC curve analysis was carried out in this study (). Our results showed that when used as a biomarker to predict the acute phase of COVID-19 disease, the AUCs of miR-125b-5p, miR-155-5p, STAT3, and TRIM32 were 1.000, 1.000, 0.824, and 0.707, respectively; when used to predict COVID-19 turn-negative, the AUC were 0.977, 0.816, 0.945, and 0.898, respectively; and when used to predict the end of recovery, the AUC is 0.984, 0.816, 0.902, and 1.000, respectively. More information can be found in .
Table 3 ROC Curve Analysis of miR-125b-5p, miR-155-5p, STAT3 and TRIM32 in Predicting the Course of COVID-19
Discussion
In this study, the course of COVID-19 disease was divided into three stages. The serum miRNA expression levels of 16 patients with COVID-19 in the acute stage, the turn-negative stage, and the recovery stage were dynamically tracked, and the target genes of differentially expressed miRNAs in each stage were predicted, and the expression levels of miRNA target genes were also analyzed. In this study, the expression of miR-29a-3p, miR-92a-3p, and miR-155-5p in serum from patients with COVID-19 was upregulated, while the expression of miR-142-3p was downregulated, which verified the results of previous COVID-19 studies,Citation15,Citation38,Citation39 however, these studies did not track the changes in miRNA expression during different stages of COVID-19. Our study found that the dysregulated miRNAs were differentially expressed in the acute stage of COVID-19, and then gradually tended to healthy people in the turn-negative stage and the recovery stage of COVID-19. This may be because the dysregulated miRNAs are involved in the regulation of inflammation and viral response in patients. It was shown that miR-125b-5p targets STAT3 in Japanese encephalitis virus infection, thereby coordinating viral replication and host antiviral response and that when the miR-125b-5p expression was upregulated, the expression levels of target genes in persistently infected cells were both decreased,Citation40 it is consistent with the expression of miR-125b-5p and STAT3 in this study. STAT3 regulates a variety of biological functions and plays a key role in many cellular processes including cell growth and apoptosis, STAT3 can be up- or down-regulated in viral infections, depending on the type of virus involved. For example, EBV, HIV-1, HBV, and HCV activate STAT3 phosphorylation, while IAV and hMPV inhibit STAT3 phosphorylation.Citation41 miR-155 has been identified as a major regulator of inflammation and can be involved in regulating CD8+ T cell activity and affecting B cell-mediated antibody production.Citation42,Citation43 In HIV infection, miR-155 binds to the 3ʹUTR of TRIM32 thereby inhibiting TRIM32 translation,Citation44 TRIM family has the ability to inhibit viral replication.Citation45 The inhibition of TRIM32 expression in this study may be caused by enhanced expression of miR-155-5p, and therefore inhibition of miR-155 expression may be an effective way to alleviate COVID-19 disease.
We analyzed the reasons for the differential expression levels of miRNAs in this study compared with other studies, such as differential expression in other studies of COVID-19 with no change in expression in this study or the opposite expression profile to this study. The first reason may be the different disease severity and stage of disease in the COVID-19 patients included in the study, resulting in different levels of differential expression of immune-related miRNAs.Citation46,Citation47 And in severe COVID-19 patients serious complications often occur in vivo, such as coagulation disorders and liver and kidney impairment. Studies have shown that these complications lead to changes in miRNA expression in vivo.Citation48–50 The second reason may be that the samples taken in our study were serum, whereas in other studies the samples were cells, and studies have shown that the expression patterns of extracellular miRNAs do not necessarily correlate with the expression patterns of intracellular miRNAs.Citation51 The last reason may be caused by the individual differences of COVID-19 patients, which are from different countries and regions, resulting from differences in ethnicity, dietary habits, and living environment.
In this study, miR-125b-5p was significantly correlated with CDH5 and STAT3, and miR-155-5p was significantly correlated with TRIM32 before, further confirming the regulatory relationship between miRNA-mRNA. MiR-125b-5p and miR-155-5p expression in this study were significantly up-regulated, significantly positively correlated with WBC and NE, and significantly negatively correlated with PLT and LY. A study showed that elevated miR-155 expression levels in COVID-19 patients were significantly correlated with clinicopathological features (eg, chest CT, CRP, ferritin, D-dimer, WBC count, and lymphocyte and neutrophil percentages) in COVID-19 patients, with a significant increase in WBC count and neutrophil count and a significant decrease in lymphocyte count in COVID-19 patients.Citation52 It was found that knocking out the miR-155 gene in mice can reduce the pulmonary inflammatory response caused by influenza virus,Citation53 and inhibition of the miR-155/NF-κB pathway was found to be effective in alleviating the inflammatory response,Citation54 so the changes in the clinicopathological characteristics of COVID-19 patients may be controlled by the miR-155/NF-κB pathway. There was also a significant correlation between ALP, CREA, and DBIL and differentially expressed miRNAs, which may predict the involvement of these miRNAs in the regulation of liver and kidney function. It has been shown that COVID-19 is involved in liver and kidney injury,Citation55,Citation56 and it has been shown that miR-125b-5p expression is upregulated in samples from HBV-infected patients, and the degree of upregulation directly correlates with the severity of disease in HBV-infected patients.Citation57 In a mouse model of kidney injury, renal miR-155 levels are significantly elevated, and inhibition of miR-155 is followed by inhibition of the JAK2/STAT3 pathway, which attenuates inflammation and apoptosis in kidney tissue, suggesting that elevated miR-155 expression affects kidney function.Citation51
Logistic regression and decision tree models are commonly used to find risk factors associated with diseases and to predict the probability of occurrence of certain diseases. Logistic regression is a traditional prediction method that can present results graphically through a columnar line graph model. However, there is a correlation between the individual predictors in this study, so logistic regression does not apply to this study. The decision tree model is an easy-to-use nonparametric classifier that has the advantages of being computationally simple, easy to understand, and highly interpretable, with the categorical regression tree algorithm being the most widely used algorithm for this model. When the dependent variable is a categorical variable, the generated decision tree is a classification tree. The classification tree model is a tool to represent the processing logic with a binary tree diagram In recent years, scholars have successfully applied the classification tree model to predict COVID-19 in the elderly population, in which the number of sample cases is 41, the same small sample prediction model as this study.Citation58 In addition, the classification tree has been applied to predict hyperuricemia,Citation59 predict the survival rate of lung cancer patients,Citation60 analyze the cause of pediatric pneumoniaCitation61 and other medical and surgical diseases, and its application in medical-related fields has proven its effectiveness. In this study, miR-125b-5p together with miR-155-5p and target genes STAT3 and TRIM32 constituted a classification tree model. Firstly, the first level of classification by miR-155-5p can make the distinction between healthy individuals and COVID-19 patients, followed by the next level of classification by miR-125b-5p, which can infer that patients in the end stage of recovery of COVID-19 patients, and finally, the combination of STAT3 and TRIM32 can predict that COVID-19 patients are in the acute stage or in the turn-negative stage. The predicted sample stage in the model matches 100% with the sample stage given in advance, which has high accuracy. In the clinic, based on the expression levels of miR-125b-5p and miR-155-5p and the target genes STAT3 and TRIM32 in the classification tree, it is possible to determine that the patient is now in that period and also to predict the course of the patient’s disease.
Our study has certain strengths and limitations. The strengths of our study are that it is the first longitudinal study to assess the expression levels of miRNAs-mRNA in the serum of COVID-19 patients, and it is the first application to apply the classification tree model to the prediction of COVID-19 disease course, which provides a reliable biomarker for the prediction of COVID-19 disease course. However, there are some limitations to our study. First, the limited size of the cohort may result in poor stability of the classification tree model and the inability to validate the established model. In addition, the medication status of all included COVID-19 patients is unknown, but considering that the subjects included in this study all excluded patients with severe comorbidities and underlying diseases and that there was little difference in medication use between the same treatment sites, the effect of medication factors is considered to be quite low. Finally, the number of miRNAs and mRNAs included in the study is limited due to the characteristics of the methodology and the continuous emergence of new studies, but this research group will continue to improve in future work.
Conclusion
MiR-125b-5p, miR-155-5p, STAT3, and TRIM32 could be useful biomarkers to predict the time nodes of the acute, turn-negative, and recovery stages of COVID-19.
Ethical Issues
This study was approved by the institution alethics committee of the First Hospital of Jilin University (2016–306). Requirement for informed consent was waived by the approval body since the study was anonymous, involved very minimal risk to the subjects, did not include intentional deception, and did not involve sensitive populations or topics; this waiver does not adversely affect the rights and welfare of the subjects. All methods/experiments were carried out in accordance with relevant guidelines and regulations (Declaration of Helsinki).
Disclosure
The authors declare no conflict of interest.
Acknowledgments
We acknowledge TopEdit LLC for the linguistic editing and proofreading during the preparation of this manuscript.
Additional information
Funding
References
- Wu F, Zhao S, Yu B, et al. A new coronavirus associated with human respiratory disease in China. Nature. 2020;579(7798):265–269. doi:10.1038/s41586-020-2008-3
- World Health Organization. Coronavirus disease (COVID-19) outbreak situation; 2021. Available from: https://www.who.int/emergencies/diseases/novel-coronavirus-2019. Accessed September 1, 2021.
- Pan Y, Li X, Yang G, et al. Serological immunochromatographic approach in diagnosis with SARS-CoV-2 infected COVID-19 patients. J Infect. 2020;81(1):e28–e32. doi:10.1016/j.jinf.2020.03.051
- Xu L, Cai Y, Chen X, Zhu Y, Cai J. Circulating MiR-1290 as a potential diagnostic and disease monitoring biomarker of human gastrointestinal tumors. BMC Cancer. 2021;21(1):989. doi:10.1186/s12885-021-08729-0
- Feng RM, Zong YN, Cao SM, Xu RH. Current cancer situation in China: good or bad news from the 2018 Global Cancer Statistics? Cancer Commun. 2019;39(1):22. doi:10.1186/s40880-019-0368-6
- Liu Z, Wang J, Ge Y, et al. SARS-CoV-2 encoded microRNAs are involved in the process of virus infection and host immune response. J Biomed Res. 2021;35(3):216–227. doi:10.7555/JBR.35.20200154
- Houshmandfar S, Saeedi-Boroujeni A, Rashno M, Khodadadi A, Mahmoudian-Sani MR. miRNA-223 as a regulator of inflammation and NLRP3 inflammasome, the main fragments in the puzzle of immunopathogenesis of different inflammatory diseases and COVID-19. Naunyn Schmiedebergs Arch Pharmacol. 2021;394(11):2187–2195. doi:10.1007/s00210-021-02163-6
- Visacri MB, Nicoletti AS, Pincinato EC, et al. Role of miRNAs as biomarkers of COVID-19: a scoping review of the status and future directions for research in this field. Biomark Med. 2021;15(18):1785–1795. doi:10.2217/bmm-2021-0348
- Li CX, Chen J, Lv SK, Li JH, Li LL, Hu X. Whole-transcriptome RNA sequencing reveals significant differentially expressed mRNAs, miRNAs, and lncRNAs and related regulating biological pathways in the peripheral blood of COVID-19 patients. Mediators Inflamm. 2021;2021:6635925. doi:10.1155/2021/6635925
- Gonzalo-Calvo D, Benitez ID, Pinilla L, et al. Circulating microRNA profiles predict the severity of COVID-19 in hospitalized patients. Transl Res. 2021;236:147–159.
- Keikha R, Hashemi-Shahri SM, Jebali A. The relative expression of miR-31, miR-29, miR-126, and miR-17 and their mRNA targets in the serum of COVID-19 patients with different grades during hospitalization. Eur J Med Res. 2021;26(1):75. doi:10.1186/s40001-021-00544-4
- Keikha R, Hashemi-Shahri SM, Jebali A. [The miRNA neuroinflammatory biomarkers in COVID-19 patients with different severity of illness]. Neurologia. 2021. Spanish. doi:10.1016/j.nrl.2021.06.005
- Askari N, Hadizadeh M, Rashidifar M. A new insight into sex-specific non-coding RNAs and networks in response to SARS-CoV-2. Infect Genet Evol. 2022;97:105195. doi:10.1016/j.meegid.2021.105195
- Bonilla-Muro MG, Hernandez de la Cruz ON, Gonzalez-Barrios JA. EsxA mainly contributes to the miR-155 overexpression in human monocyte-derived macrophages and potentially affect the immune mechanism of macrophages through miRNA dysregulation. J Microbiol Immunol Infect. 2021;54(2):185–192. doi:10.1016/j.jmii.2019.07.007
- Donyavi T, Bokharaei-Salim F, Baghi HB, et al. Acute and post-acute phase of COVID-19: analyzing expression patterns of miRNA-29a-3p, 146a-3p, 155-5p, and let-7b-3p in PBMC. Int Immunopharmacol. 2021;97:107641. doi:10.1016/j.intimp.2021.107641
- Farr RJ, Rootes CL, Stenos J, Foo CH, Cowled C, Stewart CR. Detection of SARS-CoV-2 infection by microRNA profiling of the upper respiratory tract. PLoS One. 2022;17(4):e0265670. doi:10.1371/journal.pone.0265670
- Garcia-Hidalgo MC, Gonzalez J, Benitez ID, et al. Identification of circulating microRNA profiles associated with pulmonary function and radiologic features in survivors of SARS-CoV-2-induced ARDS. Emerg Microbes Infect. 2022;11(1):1537–1549. doi:10.1080/22221751.2022.2081615
- Iravani Saadi M, Ramzi M, Hesami Z, et al. MiR-181a and -b expression in acute lymphoblastic leukemia and its correlation with acute graft-versus-host disease after hematopoietic stem cell transplantation, COVID-19 and torque teno viruses. Virusdisease;2021. 32(4):727-736. doi:10.1007/s13337-021-00653-y
- Li C, Wang R, Wu A, et al. SARS-COV-2 as potential microRNA sponge in COVID-19 patients. BMC Med Genomics. 2022;15(Suppl 2):94. doi:10.1186/s12920-022-01243-7
- Martinez-Fleta P, Vera-Tome P, Jimenez-Fernandez M, et al. A differential signature of circulating miRNAs and cytokines between COVID-19 and community-acquired pneumonia uncovers novel physiopathological mechanisms of COVID-19. Front Immunol. 2021;12:815651. doi:10.3389/fimmu.2021.815651
- Molinero M, Benitez ID, Gonzalez J, et al. Bronchial aspirate-based profiling identifies microRNA signatures associated with COVID-19 and fatal disease in critically ill patients. Front Med. 2021;8:756517. doi:10.3389/fmed.2021.756517
- O’Connell RM, Rao DS, Baltimore D. microRNA regulation of inflammatory responses. Annu Rev Immunol. 2012;30:295–312. doi:10.1146/annurev-immunol-020711-075013
- Park JH, Choi Y, Lim CW, et al. Potential therapeutic effect of microRNAs in extracellular vesicles from mesenchymal stem cells against SARS-CoV-2. Cells. 2021;10(9):2393. doi:10.3390/cells10092393
- Wang Y, Zhu X, Jiang XM, et al. Decreased inhibition of exosomal miRNAs on SARS-CoV-2 replication underlies poor outcomes in elderly people and diabetic patients. Signal Transduct Target Ther. 2021;6(1):300. doi:10.1038/s41392-021-00716-y
- Schmittgen TD, Livak KJ. Analyzing real-time PCR data by the comparative C(T) method. Nat Protoc. 2008;3(6):1101–1108. doi:10.1038/nprot.2008.73
- Chu M, Fan Y, Wu L, et al. Knockdown of lncRNA BDNF-AS inhibited the progression of multiple myeloma by targeting the miR-125a/b-5p-BCL2 axis. Immun Ageing. 2022;19(1):3. doi:10.1186/s12979-021-00258-5
- Cull AH, Snetsinger B, Buckstein R, Wells RA, Rauh MJ. Tet2 restrains inflammatory gene expression in macrophages. Exp Hematol. 2017;55(56–70):e13. doi:10.1016/j.exphem.2017.08.001
- Fu B, Wang L, Ding H, Schwamborn JC, Li S, Dorf ME. TRIM32 senses and restricts Influenza A virus by ubiquitination of PB1 polymerase. PLoS Pathog. 2015;11(6):e1004960. doi:10.1371/journal.ppat.1004960
- Jablonski KA, Gaudet AD, Amici SA, Popovich PG, Guerau-de-Arellano M. Control of the inflammatory macrophage transcriptional signature by miR-155. PLoS One. 2016;11(7):e0159724. doi:10.1371/journal.pone.0159724
- Kar P, Saleh EIMM, Jaishee N, et al. Computational profiling of natural compounds as promising inhibitors against the spike proteins of SARS-CoV-2 wild-type and the variants of concern, viral cell-entry process, and cytokine storm in COVID-19. J Cell Biochem. 2022;123(5):964–986. doi:10.1002/jcb.30243
- Liu G, Wan Q, Li J, Hu X, Gu X, Xu S. Silencing miR-125b-5p attenuates inflammatory response and apoptosis inhibition in mycobacterium tuberculosis-infected human macrophages by targeting DNA damage-regulated autophagy modulator 2 (DRAM2). Cell Cycle. 2020;19(22):3182–3194. doi:10.1080/15384101.2020.1838792
- Muller MB, Hubner M, Li L, et al. Cell-crossing functional network driven by microRNA-125a regulates endothelial permeability and monocyte trafficking in acute inflammation. Front Immunol. 2022;13:826047. doi:10.3389/fimmu.2022.826047
- Nguyen HN, Noss EH, Mizoguchi F, et al. Autocrine loop involving IL-6 family member LIF, LIF receptor, and STAT4 drives sustained fibroblast production of inflammatory mediators. Immunity. 2017;46(2):220–232. doi:10.1016/j.immuni.2017.01.004
- Sudhahar V, Das A, Horimatsu T, et al. Copper transporter ATP7A (Copper-Transporting P-Type ATPase/Menkes ATPase) limits vascular inflammation and aortic aneurysm development: role of MicroRNA-125b. Arterioscler Thromb Vasc Biol. 2019;39(11):2320–2337. doi:10.1161/ATVBAHA.119.313374
- Xu J, Liu Y, Deng M, et al. MicroRNA221-3p modulates Ets-1 expression in synovial fibroblasts from patients with osteoarthritis of temporomandibular joint. Osteoarthritis Cartilage. 2016;24(11):2003–2011. doi:10.1016/j.joca.2016.06.011
- Zeng H, Zhao B, Zhang D, et al. Viola yedoensis Makino formula alleviates DNCB-induced atopic dermatitis by activating JAK2/STAT3 signaling pathway and promoting M2 macrophages polarization. Phytomedicine. 2022;103:154228. doi:10.1016/j.phymed.2022.154228
- Zhang D, Yao X, Teng Y, et al. Adipocytes-derived exosomal microRNA-1224 inhibits M2 macrophage polarization in obesity-induced adipose tissue inflammation via MSI2-mediated Wnt/beta-catenin axis. Mol Nutr Food Res;2022. e2100889. doi:10.1002/mnfr.202100889
- Fayyad-Kazan M, Makki R, Skafi N, et al. Circulating miRNAs: potential diagnostic role for coronavirus disease 2019 (COVID-19). Infect Genet Evol. 2021;94:105020. doi:10.1016/j.meegid.2021.105020
- Tang H, Gao Y, Li Z, et al. The noncoding and coding transcriptional landscape of the peripheral immune response in patients with COVID-19. Clin Transl Med. 2020;10(6):e200. doi:10.1002/ctm2.200
- Huang CW, Tsai KN, Chen YS, Chang RY. Differential miRNA expression profiling reveals correlation of miR125b-5p with persistent infection of Japanese encephalitis virus. Int J Mol Sci. 2021;22(8):4218.
- Kuchipudi SV. The complex role of STAT3 in viral infections. J Immunol Res. 2015;2015:272359. doi:10.1155/2015/272359
- Alivernini S, Gremese E, McSharry C, et al. MicroRNA-155-at the critical interface of innate and adaptive immunity in arthritis. Front Immunol. 2017;8:1932. doi:10.3389/fimmu.2017.01932
- Vigorito E, Kohlhaas S, Lu D, Leyland R. miR-155: an ancient regulator of the immune system. Immunol Rev. 2013;253(1):146–157. doi:10.1111/imr.12057
- Fatima M, Kumari R, Schwamborn JC, et al. Tripartite containing motif 32 modulates proliferation of human neural precursor cells in HIV-1 neurodegeneration. Cell Death Differ. 2016;23(5):776–786. doi:10.1038/cdd.2015.138
- Uchil PD, Hinz A, Siegel S, et al. TRIM protein-mediated regulation of inflammatory and innate immune signaling and its association with antiretroviral activity. J Virol. 2013;87(1):257–272. doi:10.1128/JVI.01804-12
- de Gonzalo-Calvo D, Benitez ID, Pinilla L, et al. Circulating microRNA profiles predict the severity of COVID-19 in hospitalized patients. Transl Res. 2021;236:147–159. doi:10.1016/j.trsl.2021.05.004
- Li S, Duan X, Li Y, et al. Differentially expressed immune response genes in COVID-19 patients based on disease severity. Aging. 2021;13(7):9265–9276. doi:10.18632/aging.202877
- Luo Y, Fu Y, Tan T, et al. Screening of lncRNA-miRNA-mRNA coexpression regulatory networks involved in acute traumatic coagulation dysfunction based on CTD, GeneCards, and PharmGKB databases. Oxid Med Cell Longev. 2022;2022:7280312. doi:10.1155/2022/7280312
- Zhao Y, Lang Y, Zhang M, Liang S, Zhu X, Liu Z. miR-125b disrupts mitochondrial dynamics via targeting Mitofusin 1 in cisplatin-induced acute kidney injury. Kidney Dis. 2022;8(2):137–147. doi:10.1159/000520140
- Feng X, Bao J, Song C, et al. Functional role of miR155 in physiological and pathological processes of liver injury (Review). Mol Med Rep. 2021;24(4):714. doi:10.3892/mmr.2021.12353
- de Gonzalo-Calvo D, Cenarro A, Garlaschelli K, et al. Translating the microRNA signature of microvesicles derived from human coronary artery smooth muscle cells in patients with familial hypercholesterolemia and coronary artery disease. J Mol Cell Cardiol. 2017;106:55–67. doi:10.1016/j.yjmcc.2017.03.005
- Haroun RA, Osman WH, Amin RE, Hassan AK, Abo-Shanab WS, Eessa AM. Circulating plasma miR-155 is a potential biomarker for the detection of SARS-CoV-2 infection. Pathology. 2022;54(1):104–110. doi:10.1016/j.pathol.2021.09.006
- Pociask DA, Robinson KM, Chen K, et al. Epigenetic and transcriptomic regulation of lung repair during recovery from influenza infection. Am J Pathol. 2017;187(4):851–863. doi:10.1016/j.ajpath.2016.12.012
- Koranteng RD, Swindle EJ, Davis BJ, et al. Differential regulation of mast cell cytokines by both dexamethasone and the p38 mitogen-activated protein kinase (MAPK) inhibitor SB203580. Clin Exp Immunol. 2004;137(1):81–87. doi:10.1111/j.1365-2249.2004.02510.x
- Zhang H, Liao YS, Gong J, Liu J, Zhang H. Clinical characteristics and risk factors for liver injury in COVID-19 patients in Wuhan. World J Gastroenterol. 2020;26(31):4694–4702. doi:10.3748/wjg.v26.i31.4694
- Kudose S, Batal I, Santoriello D, et al. Kidney biopsy findings in patients with COVID-19. J Am Soc Nephrol. 2020;31(9):1959–1968. doi:10.1681/ASN.2020060802
- Coppola N, Potenza N, Pisaturo M, et al. Liver microRNA hsa-miR-125a-5p in HBV chronic infection: correlation with HBV replication and disease progression. PLoS One. 2013;8(7):e65336. doi:10.1371/journal.pone.0065336
- Sacco G, Foucault G, Briere O, Annweiler C. COVID-19 in seniors: findings and lessons from mass screening in a nursing home. Maturitas. 2020;141:46–52. doi:10.1016/j.maturitas.2020.06.023
- Shi JC, Chen XH, Yang Q, et al. A simple prediction model of hyperuricemia for use in a rural setting. Sci Rep. 2021;11(1):23300. doi:10.1038/s41598-021-02716-y
- Tohme S, Yazdani HO, Rahman A, et al. The use of machine learning to create a risk score to predict survival in patients with hepatocellular carcinoma: a TCGA cohort analysis. Can J Gastroenterol Hepatol. 2021;2021:5212953. doi:10.1155/2021/5212953
- Valim C, Ahmad R, Lanaspa M, et al. Responses to bacteria, virus, and malaria distinguish the etiology of pediatric clinical pneumonia. Am J Respir Crit Care Med. 2016;193(4):448–459. doi:10.1164/rccm.201506-1100OC