Abstract
Background
Immunotherapy has gradually played a significant role in treating cancer over the past 10 years. Meanwhile, significant connections have been found between infiltrating immune cells and prognosis, as well as the development of immunotherapy in hepatocellular carcinoma (HCC). Thus, analyses of the immune cell landscape are urgently needed for the future development of immunotherapy in HCC.
Methods
Expression data of HCC and normal liver tissue were searched and downloaded from The Cancer Genome Atlas. The fraction of various immune cells was estimated by CIBERSORT, which is a computational analysis tool. Comparisons of the fractions of 22 types of immune cells were performed between HCC and normal tissues, as well as survival analyses and clinical significance. xCell was used to validate the results.
Results
The fractions of 12 types of immune cells, including follicular helper T (Tfh) cells, monocytes, M0, and M2 macrophages, were significantly different between tissues. In survival analyses, higher fractions of Tfh cells (P=0.037), M0 (P=0.001), and M2 macrophages (P=0.045) were associated with a poorer prognosis, and monocytes with a better prognosis (P=0.040). Furthermore, a higher fraction of M0 macrophages (P=0.001), lower fraction of monocytes (P=0.031), and M2 macrophages (P=0.018) were found as risk factors of a poorer histological grade of HCC. Only M0 macrophages showed a significant association with the clinical stage of HCC. Meanwhile, xCell showed monocytes and M2 macrophages significantly reduced in tumor tissues, which validated the results.
Conclusion
Tfh cells, monocytes, M0 and M2 macrophages may play an indicator role in carcinogenesis, progression, and clinical outcomes of HCC. Our research can serve as a reference contributing to future immunotherapy strategies of HCC.
Background
Hepatocellular carcinoma (HCC) is the leading cause of cancer-related deaths worldwide with significant influences on patient’s quality of life, as well as massive economic costs.Citation1–Citation3 The global burden of HCC is gradually raising and about to reach an annual incidence of one million cases.Citation4 For early-stage HCC, the treatments are based on curative approaches: image-guided ablation, liver resection, liver transplantation and etc.Citation5,Citation6 However for intermediate and advanced stage HCC, there are limited treatments with relatively poor prognosis even after decades of development of molecular therapies.Citation7 As a brand-new cancer treatment, immunotherapy has attracted much attention in recent years. Up to now, there are various solid tumors where immunotherapy is developed and approved as an advanced therapy,Citation8 including HCC.Citation9–Citation11
The development of immunotherapy vastly depends on the recognition of immune microenvironment of the tumor. Rohr-Udilova et al investigated deviations of immune cell landscape between healthy liver and HCC using Gene Expression Omnibus database, showing different compositions of immune cells between healthy liver and HCC tissues.Citation12 On the other hand, the functions of T cells in treating HCC were well elucidated by PD-1-associated therapies.Citation13 However, these studies failed to investigate the associations between the immune cell landscape and clinical characteristics or outcomes of HCC.
CIBERSORT is a computational technique to characterize cell composition from gene expression data of tissues,Citation14 which is proven to be effective and accurate for identifying infiltrating immune cells with high-resolution properties from various tumor tissue.Citation15 Unlike immunohistochemistry or flow cytometry suffering from limitations in phenotypic markers practical implement and standardization, CIBERSORT is a versatile computational method with higher feasibility. Furthermore, the accuracy of CIBERSORT has been verified by flow cytometry.Citation16
To better recognize the role of the various types of immune cells in the tumor microenvironment, in this study, we analyzed the differences of the infiltrating immune cell landscape as well as its clinical significance in HCC.
Methods
RNA-sequencing (RNA-Seq) data and clinical data of HCC tissues and normal adjacent tissues were searched and downloaded from The Cancer Genome Atlas (TCGA, http://cancergenome.nih.gov/). A total of 374 HCC tissues and 50 normal adjacent tissue were finally found and included in this study.
RNA-Seq datasets of HCC and normal adjacent tissues were analyzed using CIBERSORT (https://cibersort.stanford.edu) to identify immune cell composition through LM22, an original CIBERSORT gene signature file defining 22 types of immune cells including naïve B cells, memory B cells, plasma cells, CD8+ T cells, naïve CD4+ T cells, resting CD4+ memory T cells, activated CD4+ memory T cells, follicular helper T (Tfh) cells, regulatory T cells (Tregs), gamma delta T cells, resting NK cells, activated NK cells, monocytes, M0 macrophages, M1 macrophages, M2 macrophages, resting dendritic cells, activated dendritic cells, resting mast cells, activated mast cells, eosinophils, neutrophils.
R 3.3.0 and Statistical Package for Social Sciences 23.0 for Windows (SPSS Inc., Chicago, IL, USA) were used to perform statistical analyses, and GraphPad Prism software to generate pictures. One-way analysis of variance (ANOVA) was used, with homogeneous variance, to analyze differences in immune cell components between normal and HCC tissues, while Welch’s ANOVA was applied when variance was heterogeneous. Kaplan-Meier curve was used for survival analysis, where the Log rank test was for comparison between groups.
Results
Adaptive Immune Cells
Comparisons of adaptive immune cells landscape between HCC and normal adjacent tissues are shown in . The differences were statistically insignificant in the fraction of naive B cells, memory B cells, plasma cells or total B cells between tissues. Furthermore, no difference was detected in fraction of naive CD4+ T cells or CD8+ T cells. However, there was an increase of resting CD4+ memory T cells, activated CD4+ memory T cells, Tfh cell and Tregs in HCC tissue compared to normal control, while gamma delta T cells decreased in HCC. Overall, the total fraction of T cells was more frequent in HCC than in normal controls.
Figure 1 Comparisons of adaptive immune cells between HCC and normal tissues. Median with 95% confidence interval were shown as red lines. (A) naive B cells; (B) memory B cells; (C) plasma cells; (D) total B cells; (E) CD8+ T cells; (F) naive CD4+ T cells; (G) resting CD4+ memory T cells; (H) activated CD4+ memory T cells; (I) follicular helper T cells; (J) regulatory T cells; (K) gamma delta T cells; (L) total T cells. *P<0.05; **P<0.001.
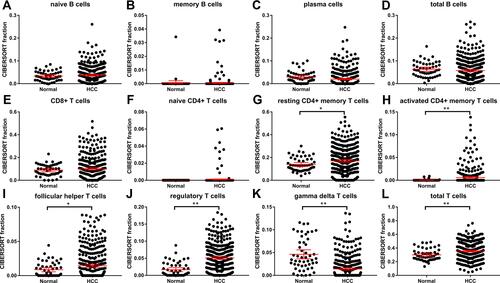
Innate Immune Cells
Comparisons of innate immune cells landscape between tissues were shown in . It showed statistically insignificant differences in resting NK cells, activated NK cells, M1 macrophages, activated dendritic cells or eosinophils. Meanwhile, monocytes, M2 macrophages, activated mast cells and neutrophils significantly reduced in tumor tissues, while M0 macrophages, resting dendritic cells and resting mast cells were upregulated in HCC tissues.
Figure 2 Comparisons of innate immune cells between HCC and normal tissues. Median with 95% confidence interval were shown as red lines. (A) resting NK cells; (B) activated NK cells; (C) monocytes; (D) M0 macrophages; (E) M1 macrophages; (F) M2 macrophages; (G) resting dendritic cells; (H) activated dendritic cells; (I) resting mast cells; (J) activated mast cells; (K) eosinophils; (L) neutrophils. *P<0.05; **P<0.001.
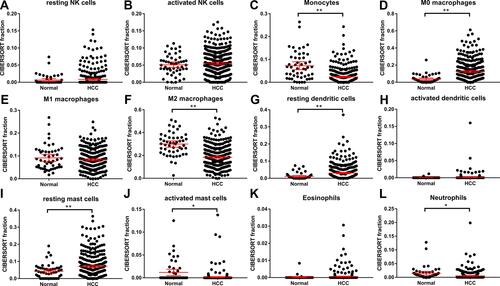
Immune Cell Patterns
The fractions of immune cells in both types of tissues are shown in and . Two leading types of immune cells were M2 macrophages and resting CD4+ memory T cells in both normal tissues (30.0% and 13.9%) and HCC tissues (18.4% and 17.0%). M1 macrophages were the third leading type in normal tissues (9.1%), while M0 macrophages in HCC tissues (12.4%). The heatmap and correlation heatmap of 22 immune cells were shown in and , respectively. The most positive correlation in HCC was detected between CD8+ T cells and activated CD4+ memory T cells (Pearson correlation coefficient = 0.46). On the other hand, CD8+ T cells and Tfh cells and were shown as having equally negative correlation with resting CD4+ memory T cells (Pearson correlation coefficient = −0.43).
Table 1 Fraction of Immune Cells
Prognostic Assessment of Infiltrating Immune Cells
Clinical data of 377 patients retrieved from TCGA are shown in . Among them, a total of 370 patients with adequate clinical data were divided into the high group or low group according to the medium fraction of each variety of immune cell. Statistically significant differences in survival analyses were found in 4 types of immune cells including Tfh cell, Monocytes, M0 macrophages, and M2 macrophages, shown in , while the rest are shown in the Supplement Material. HCC patients with a lower fraction of Tfh cell, M0, and M2 macrophages tended to have a better prognosis, while HCC with a lower proportion of monocytes had a poorer prognosis.
Table 2 Patient Baseline and Tumor Characteristics
Relationship Between Clinical Features and Infiltrating Immune Cells
Toward the four types of immune cells associated with prognosis, further analyses were performed to investigate its potential relationships with clinical features. 366 patients with known histologic grades were enrolled and the results are shown in . M2 macrophages and Monocytes were decreasing, and M0 macrophages were increasing with histological grade deteriorating from G1 to G4. However, the fraction of Tfh cell was not associated with histological grade.
Figure 5 Differences of the fraction of follicular helper T cells, monocytes, M0 and M2 macrophages in each histological grade (A–D) and clinical stage (E–H) of HCC.
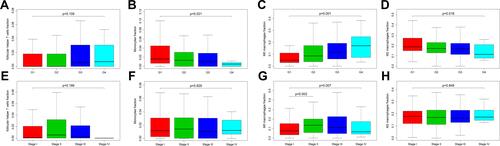
347 patients with known clinical classification (8th Edition of The AJCC Cancer Staging Manual) were enrolled and the results are shown in . The fractions of Tfh cell, M2 macrophages and Monocytes had no difference between various stages. M0 macrophages were significantly lower in stage I, while no difference was found between stage II, III, and IV.
xCell
In the meantime, xCell was used to confirm the differences of the fraction of these four prognostic immune cell types between two tissues.Citation17 However, Tfh cell and M0 macrophages were not contained by xCell. Thus, we compared the fractions of monocytes and M2 macrophages between two tissues, and the results are shown in . Monocytes and M2 macrophages significantly reduced in tumor tissues, which was consistent with the result from CIBERSORT.
Discussion
In the present study, we used CIBERSORT to identify and compare the infiltrating immune cells composition between HCC and normal adjacent tissues. Furthermore, associations of these immune cells with patient prognosis and tumor characteristics were also investigated. Our results suggested that Tfh cell, Monocytes, M0 macrophages, and M2 macrophages had significant roles in carcinogenesis, progression, and prognosis of HCC.
Follicular helper T cells, which prime B cells to initiate extrafollicular and germinal center antibody responses, are recognized as highly expressing CXCR5 and driven by a transcription factor, BCL6.Citation18 It has been suggested that infiltration of Tfh cells is associated with progression of lymphoid tumors,Citation19 however the role that Tfh cells have in solid tumors is little researched with incompatible results. Studies showed Tfh cells infiltrating was linked with improved survival in breast cancer and colorectal cancer.Citation20,Citation21 On the other hand, the high number of Tfh cells could cause further immunosuppression and lead to tumor development in non-small cell lung cancer,Citation22 which is consistent with our results. In addition, our results also suggested that the effect of Tfh cells on carcinogenesis and prognosis of HCC was independent of histological grade and clinical stage.
Monocytes, members of the mononuclear phagocyte system including macrophages and dendritic cells, are a crucial part of the human innate immune system with complex functions both within blood and after their migration into various tissues.Citation23 Currently, monocytes are mainly recognized as promoters in the development of cancer affecting widely from the initiation of vessel growth to immune escape and metastasis.Citation24 Interestingly, our results presented that the proportion of infiltrating monocytes were lower in HCC than normal tissues, and higher infiltrating monocytes was shown as a protective factor with better patient prognosis and histological grade of tumor, indicating monocytes as inhibitors in HCC. A few studies revealed that patrolling monocytes can contribute to cancer immunosurveillance and reduction of metastasis, however the associated mechanism of the protective effect remains unclear.Citation25,Citation26 It was considered to be a balance between the anti-tumor activity of monocytes and pro-tumor function of macrophages,Citation27 which may explain the situation. Future studies should be performed to confirm the results and explore further mechanisms.
Macrophages are now recognized as the main component in microenvironment of tumors, with either promoting or inhibiting effects on carcinogenesis and metastasis according to their state.Citation28 In many solid tumor types, a number of studies suggest high densities of macrophage-associated markers have been reported to associate with progressing of tumor and poor clinical outcome, through affecting the regulation of tumor cell survival pathways, tumor angiogenesis, immune suppression, and tumor metastasis.Citation29 However, conflicting data are reported with both positive and negative prognosis associations in various tumors.Citation30 In the present study, M0 macrophages were undoubtedly connected with development, progression, and poor prognosis of HCC, while complicated results were shown about M2 macrophages. On the one hand, a lower fraction of M2 macrophages was detected in HCC compared with normal tissue, and associated with poorer histological grade. On the other hand, a better prognosis was found in the group with lower M2 macrophages, indicating the effect of M2 macrophages in promoting tumors may be independent of carcinogenesis and histological differentiation. Overall, macrophages function complicatedly in HCC with both promoting and potential inhibiting effects, which remains to be investigated in further researches.
CD4+ T cells were reported to be associated with hepatocarcinogenesis in non-alcoholic fatty liver disease.Citation31 Moreover, it has been suggested that HCC patients with a higher fraction of CD4+ T cells had a preferred survival data.Citation32 However, in our study, no significant difference was shown between patients with a lower fraction of CD4+ T cells and those with a higher fraction of CD4+ T cells. Meanwhile, the functions of Tregs were facing controversies. On the one hand, Tregs might upregulate checkpoint inhibitors and contribute to systemic immune dysfunction and antitumoural activity by several inhibitory pathways.Citation33 On the other hand, Tregs could also be recruited by tumor-associated neutrophils to promote progression of HCC and resistance to sorafenib.Citation34 These previous studies might explain that the Tregs were not associated with survival data in this research, because these effects of Tregs on HCC patient survival might cancel each other out.
There were some studies investigating impacts of immune cell landscape on HCC that showed consistent and inconsistent results. Rohr-Udilova et al investigated deviations of immune cell landscape between healthy liver and HCC using Gene Expression Omnibus database.Citation12 Their results showed gamma delta T cells, activated mast cells, monocytes were decreased in HCC while resting CD4+ memory T cells, activated CD4+ memory T cells, Tfh cells, M2 macrophages, resting mast cells were increased compared with normal adjacent tissues, which was most consistent with our results except macrophages. For studies involving clinical outcomes, Tang et al focused on immune cell landscape in HCC patients with different degrees of fibrosis and revealed that PVRIG involved in immune cells and significantly associated with the outcome of HCC patients was identified, however without investigations about impacts of various immune cells on survival data.Citation35 Furthermore, we also used xCell to confirm the results and explored the relationship between immune cell landscape and clinical characteristics to show the significances of immune cell landscape in HCC.
There were still limitations in the present study. Firstly, the relatively small cohort of normal control tissue might reduce the reliability of our results. Secondly, CIBERSORT was a convenient computational method to determine the fractions of infiltrating immune cells, but it was still less exact than immunohistochemistry or flow cytometry, which might cause inaccuracy of fractions of immune cells. Thirdly, myeloid-derived suppressor cells (MDSC), which suppresses T cell functions, could not be predicted by CIBERSORT. Fourthly, we failed to confirm the fraction of Tfh cells and M0 macrophages using xCell because it did not contain the two types of immune cells. Lastly, the immune cell landscape and its significance might alter in different subtypes of HCC. This study failed to classify subtypes of HCC, and subsequently failed to demonstrate the significances of immune cells in distinct subtypes of HCC, which should be a focus in future studies.
Conclusion
In summary, we analyzed tissue expression data using CIBERSORT to provide explicit information about infiltrating immune cells in HCC. Tfh cells, monocytes, M0, and M2 macrophages may play an indicator role in carcinogenesis, progression, and clinical outcomes of HCC. Our research can serve as a reference for future immunotherapy strategies of HCC.
Abbreviations
HCC, hepatocellular carcinoma; Tfh cells, follicular helper T cells; RNA-Seq, RNA-sequencing; TCGA, the cancer genome atlas; Tregs, regulatory T cells; NK cells, natural killer cells; ANOVA, one-way analysis of variance.
Data Sharing Statement
All data generated or analysed during this study are included in this published article and the Supplement Material.
Ethics Approval and Consent to Participate
Extra informed consent is not essential for the data were all obtained from public database. The authors can not assess to any identifying characteristics, which do not distort scientific meaning.
Research Involving Human Participants and/or Animals
This article does not contain any studies with human participants or animals performed by any of the authors.
Guarantor of the Article
The corresponding author is accessing to the data and have control of the decision to publish and accepting full responsibility for the conduct of the study.
Author Contributions
All authors contributed to data analysis, drafting or revising the article, gave final approval of the version to be published, agreed to the submitted journal, and agree to be accountable for all aspects of the work.
Disclosure
The authors declare that they have no competing interests.
References
- Jemal A, Bray F, Center MM, Ferlay J, Ward E, Forman D. Global cancer statistics. CA Cancer J Clin. 2011;61(2):69–90.
- Fu J, Li Y, Li Z, Li N. Clinical utility of decarboxylation prothrombin combined with α-fetoprotein for diagnosing primary hepatocellular carcinoma. Bioscience Rep. 2018;38(5):R20180044. doi:10.1042/BSR20180044
- Fu S, Li N, Zhou P, Huang Y, Zhou R, Fan X. Detection of HBV DNA and antigens in HBsAg-positive patients with primary hepatocellular carcinoma. Clin Res Hepatol Gas. 2017;41(4):415–423. doi:10.1016/j.clinre.2017.01.009
- Llovet JM, Montal R, Sia D, Finn RS. Molecular therapies and precision medicine for hepatocellular carcinoma. Nat Rev Clin Oncol. 2018;15(10):599–616. doi:10.1038/s41571-018-0073-4
- Zou H, Tao Y, Wang ZM. Integration of Child-Pugh score with future liver remnant yields improved prediction of liver dysfunction risk for HBV-related hepatocellular carcinoma following hepatic resection. Oncol Lett. 2017;13(5):3631–3637. doi:10.3892/ol.2017.5919
- Zou H, Xue H, Tao Y. Liver three-dimensional reconstruction accurately predicts remnant liver volume for HBV-related hepatocellular carcinoma prior to hepatectomy. Indian J Surg. 2018;80(5):488–493. doi:10.1007/s12262-017-1645-8
- Huang Y, Zhang Z, Zhou Y, Yang J, Hu K, Wang Z. Should we apply sorafenib in hepatocellular carcinoma patients with microvascular invasion after curative hepatectomy? Oncotargets Ther. 2019;12:541–548. doi:10.2147/OTT.S187357
- Dyck L, Mills KHG. Immune checkpoints and their inhibition in cancer and infectious diseases. Eur J Immunol. 2017;47(5):765–779. doi:10.1002/eji.201646875
- El-Khoueiry AB, Sangro B, Yau T, et al. Nivolumab in patients with advanced hepatocellular carcinoma (CheckMate 040): an open-label, non-comparative, Phase 1/2 dose escalation and expansion trial. Lancet. 2017;389(10088):2492–2502.
- El-Khoueiry AB, Melero I, Crocenzi TS, et al. Phase I/II safety and antitumor activity of nivolumab in patients with advanced hepatocellular carcinoma (HCC): CA209-040. J Clin Oncol. 2015;33(18_suppl):A101. doi:10.1200/jco.2015.33.18_suppl.lba101
- Zhu AX, Finn RS, Edeline J, et al. Pembrolizumab in patients with advanced hepatocellular carcinoma previously treated with sorafenib (KEYNOTE-224): a non-randomised, open-label Phase 2 trial. Lancet Oncol. 2018;19(7):940–952. doi:10.1016/S1470-2045(18)30351-6
- Rohr-Udilova N, Klinglmuller F, Schulte-Hermann R, et al. Deviations of the immune cell landscape between healthy liver and hepatocellular carcinoma. Sci Rep. 2018;8(1):6220. doi:10.1038/s41598-018-24437-5
- Sunshine J, Taube JM. PD-1/PD-L1 inhibitors. Curr Opin Pharmacol. 2015;23:32–38. doi:10.1016/j.coph.2015.05.011
- Newman AM, Liu CL, Green MR, et al. Robust enumeration of cell subsets from tissue expression profiles. Nat Methods. 2015;12(5):453–457. doi:10.1038/nmeth.3337
- Chen B, Khodadoust MS, Liu CL, Newman AM, Alizadeh AA. Profiling tumor infiltrating immune cells with CIBERSORT. Methods Mol Biol. 2018;1711:243.
- Ali HR, Chlon L, Pharoah PD, Markowetz F, Caldas C. Patterns of immune infiltration in breast cancer and their clinical implications: a gene-expression-based retrospective study. Plos Med. 2016;13(12):e1002194. doi:10.1371/journal.pmed.1002194
- Aran D, Hu Z, Butte AJ. xCell: digitally portraying the tissue cellular heterogeneity landscape. Genome Biol. 2017;18(1):220. doi:10.1186/s13059-017-1349-1
- Vinuesa CG, Linterman MA, Yu D, MacLennan IC. Follicular helper T cells. Annu Rev Immunol. 2016;34:335–368. doi:10.1146/annurev-immunol-041015-055605
- Ame-Thomas P, Le Priol J, Yssel H, et al. Characterization of intratumoral follicular helper T cells in follicular lymphoma: role in the survival of malignant B cells. Leukemia. 2012;26(5):1053–1063. doi:10.1038/leu.2011.301
- Bindea G, Mlecnik B, Tosolini M, et al. Spatiotemporal dynamics of intratumoral immune cells reveal the immune landscape in human cancer. Immunity. 2013;39(4):782–795. doi:10.1016/j.immuni.2013.10.003
- Gu-Trantien C, Loi S, Garaud S, et al. CD4(+) follicular helper T cell infiltration predicts breast cancer survival. J Clin Invest. 2013;123(7):2873–2892. doi:10.1172/JCI67428
- Qiu L, Yu Q, Zhou Y, et al. Functionally impaired follicular helper T cells induce regulatory B cells and CD14(+) human leukocyte antigen-DR(-) cell differentiation in non-small cell lung cancer. Cancer Sci. 2018;109(12):3751–3761. doi:10.1111/cas.13836
- Jakubzick CV, Randolph GJ, Henson PM. Monocyte differentiation and antigen-presenting functions. Nat Rev Immunol. 2017;17(6):349–362. doi:10.1038/nri.2017.28
- Guilliams M, Mildner A, Yona S. Developmental and functional heterogeneity of monocytes. Immunity. 2018;49(4):595–613. doi:10.1016/j.immuni.2018.10.005
- Qian BZ, Li J, Zhang H, et al. CCL2 recruits inflammatory monocytes to facilitate breast-tumour metastasis. Nature. 2011;475(7355):222–225. doi:10.1038/nature10138
- Hanna RN, Cekic C, Sag D, et al. Patrolling monocytes control tumor metastasis to the lung. Science. 2015;350(6263):985–990. doi:10.1126/science.aac9407
- Murray PJ. Immune regulation by monocytes. Semin Immunol. 2018;35:12–18. doi:10.1016/j.smim.2017.12.005
- Guerriero JL. Macrophages: the road less traveled, changing anticancer therapy. Trends Mol Med. 2018;24(5):472–489. doi:10.1016/j.molmed.2018.03.006
- Ruffell B, Coussens LM. Macrophages and therapeutic resistance in cancer. Cancer Cell. 2015;27(4):462–472. doi:10.1016/j.ccell.2015.02.015
- Zhang QW, Liu L, Gong CY, et al. Prognostic significance of tumor-associated macrophages in solid tumor: a meta-analysis of the literature. PLoS One. 2012;7(12):e50946. doi:10.1371/journal.pone.0050946
- Ma C, Kesarwala AH, Eggert T, et al. NAFLD causes selective CD4(+) T lymphocyte loss and promotes hepatocarcinogenesis. Nature. 2016;531(7593):253–257. doi:10.1038/nature16969
- Zheng C, Zheng L, Yoo JK, et al. Landscape of infiltrating T cells in liver cancer revealed by single-cell sequencing. Cell. 2017;169(7):1342–1356. doi:10.1016/j.cell.2017.05.035
- Langhans B, Nischalke HD, Krämer B, et al. Role of regulatory T cells and checkpoint inhibition in hepatocellular carcinoma. Cancer Immunol Immunother. 2019;68(12):2055–2066. doi:10.1007/s00262-019-02427-4
- Zhou SL, Zhou ZJ, Hu ZQ, et al. Tumor-associated neutrophils recruit macrophages and T-regulatory cells to promote progression of hepatocellular carcinoma and resistance to sorafenib. Gastroenterology. 2016;150(7):1646–1658. doi:10.1053/j.gastro.2016.02.040
- Tang X, Shu Z, Zhang W, et al. Clinical significance of the immune cell landscape in hepatocellular carcinoma patients with different degrees of fibrosis. Ann Transl Med. 2019;7(20):528. doi:10.21037/atm.2019.09.122