Abstract
Objective
Rheumatoid arthritis (RA) is one of the most prevalent inflammatory arthritis worldwide. However, the genes and pathways associated with macrophages from synovial fluids in RA patients still remain unclear. This study aims to screen and verify differentially expressed genes (DEGs) related to identifying candidate genes related to synovial macrophages in rheumatoid arthritis by bioinformatics analysis.
Methods
We searched the Gene Expression Omnibus (GEO) database, and GSE97779 and GSE10500 with synovial macrophages expression profiling from multiple RA microarray dataset were selected to conduct a systematic analysis. GSE97779 included nine macrophage samples from synovial fluids of RA patients and five macrophage samples from primary human blood of HC. GSE10500 included five macrophage samples from synovial fluids of RA patients and three macrophage samples from primary human blood of HC. Functional annotation of DEGs was performed, including Gene Ontology (GO) analysis and Kyoto Encyclopedia of Genes and Genomes (KEGG) pathway enrichment analysis. Protein–protein interaction (PPI) network of DEGs was established using the STRING database. CytoHubba was used to identify hub genes. MCODE was used to determine gene clusters in the interactive network.
Results
There were 2638 DEGs (1425 upregulated genes and 1213 downregulated ones) and 889 DEGs (438 upregulated genes and 451 downregulated ones) selected from GSE97779 and GSE10500, respectively. Venn diagrams showed that 173 genes were upregulated and 106 downregulated in both two datasets. The top 10 hub genes, including FN1, VEGFA, HGF, SERPINA1, MMP9, PPBP, CD44, FPR2, IGF1, and ITGAM, were identified using the PPI network.
Conclusion
This study provides new insights for the potential biomarkers and the relevant molecular mechanisms in RA patients. Our findings suggest that the 10 candidate genes might be used in diagnosis, prognosis, and therapy of RA in the future. However, further studies are required to confirm the expression of these genes in synovial macrophages in RA and control specimen.
Introduction
Rheumatoid arthritis (RA) is a chronic and aggressive autoimmune disorder mainly characterized by destructive synovitis, cartilage destruction, and systemic organ involvement, with a prevalence of about 0.5–1% in the global population.Citation1,Citation2 Chronic pain, tenderness, swelling, deformation, and stiffness of the joints are the clinical manifestations of RA, which ultimately lead to a high incidence of disability and a heavy socio-economic burden.Citation3,Citation4
In RA patients, multiple chronic inflammatory cells infiltrate the synovium, usually bringing about pain and reducing joint functions. As we all know, fibroblast-like synoviocytes, macrophage-like synoviocytes, and macrophages are the main types of cells in the synovium, and synovitis can stimulate macrophages in synovial tissues to produce angiogenic factors, resulting in abnormal vascular formation in the synovium.Citation5 As we all know, macrophages are central to the pathophysiology of RA, and involved in the initiation and maintenance of inflammation, the adhesion and migration of leukocytes, the degradation of matrix and angiogenesis.Citation6,Citation7 Macrophages express adhesion molecules, chemokine receptors (CR), and other surface antigens, and also secrete chemokines, cytokines, growth factors, proteases, and other mediators, and these inflammatory mediators are important pathological mechanisms of inflammation and angiogenesis in arthritis.Citation6,Citation7 For example, in the early stage of RA, macrophages in the synovium mainly secrete chemokines CXCL4 and CXCL7 to recruit neutrophils and monocytes, and chemoattractants (CCL2 and IL-8) and alarmins S100A8/9 as well as tissue remodeling enzymes (MMP-3 and MMP-12) were produced throughout the active disease;Citation8 in the active stage of RA, macrophage population is characterized by M1-like inflammatory cells, which produce significant increase levels of proinflammatory cytokines TNF-α, IL-6, and IL-1β, while reduced expression of the M2 macrophage associated marker CD209.Citation9 In view of the fact that macrophages and their expression and secretion products play a key role in the pathogenesis of RA, they have become an ideal target for the treatment of RA.
A large number of studies have shown that genetic changes and environmental influences might promote the development of RA.Citation10 However, it is still unclear how the expression of gene and protein in the macrophage-like synoviocytes is involved in the development of RA.Citation11 Therefore, it is of great significance to reveal the underlying regulatory mechanisms of macrophage infiltration and activation, which might provide novel insights for more effective therapeutic and preventive strategies of RA.
Currently, the identification of differentially expressed genes (DEGs) between abnormal and normal samples, as a high-throughput technique, can be used to determine various disease-related target genes, potential molecular mechanisms, diagnosis, and prognosis.Citation12 Although there have been studies using Gene Expression Omnibus (GEO) to explore the mRNA expression profiles of pancreatic islets in RA, there is still a lack of research exploring pathogenic genes related to RA macrophages based on bioinformatics analysis.Citation13,Citation14 We used DAVID database to perform Gene Ontology (GO) functional annotation analysis and Kyoto Encyclopedia of Genes and Genomes (KEGG) pathway enrichment analysis of DEGs, then constructed protein–protein interaction (PPI) network, and finally used cytoHubba and Cytotype MCODE to identify significant modules and key genes.
Therefore, our study aimed to identify hub genes related to synovial macrophages to further explore the pathogenesis of RA by Gene Ontology (GO) functional annotation analysis, Kyoto Encyclopedia of Genes and Genomes (KEGG) pathway enrichment analysis, the construction of protein–protein interaction (PPI) network, and the analysis of cytoHubba and Cytotype MCODE, thereby providing key synovial macrophages-related biomarkers of RA, and new insights for the pathogenesis of RA, which might improve the treatment strategies, and diagnostic approaches in the future.
Materials and Methods
Microarray Expression Data
We downloaded the microarray expression data of GSE97779 and GSE10500 from the GEO database (https://www.ncbi.nlm.nih.gov/geo/). GSE97779 included nine macrophage samples from synovial fluids of RA patients and five macrophage samples from primary human blood of HC. This GSE97779_series_matrix was established using the platform GPL570 (HG-U133_Plus_2) Affymetrix Human Genome U133 Plus 2.0 Array. GSE10500 included five macrophage samples from synovial fluids of RA patients and three macrophage samples from primary human blood of HC. This GSE10500_series_matrix was established using platform GPL8300 (HG-U95Av2) Affymetrix Human Genome U95 Version 2 Array.
Identification of DEGs
We used LIMMA package (http://www.bioconductor.org/packages/release/bioc/html/limma.html) of R language (https://www.r-project.org/; version 3.4.0) to screen out DEGs related to synovial macrophages between RA samples and control samples. Genes with more than 1 probe set were averaged, and genes with probe sets lacking gene symbols were eliminated. Adjusted P-value <0.05 and Log [Fold Change] (Log [FC])>1, as the screening criteria, were considered statistically significant. Venn diagram (http://bioinformatics.psb.ugent.be/webtools/Venn/) was drawn to show intersections of the two data sets.
KEGG Pathway Enrichment Analysis and GO Functional Enrichment Analysis
We used DAVID (Database for Annotation, Visualization and Integrated Discovery, https://david.ncifcrf.gov/) to perform KEGG pathway enrichment analysis and GO functional enrichment analysis to explore the underlying functions of the DEGs and the main pathways involved. We analyzed the DEGs in the GSE97779 and GSE10500 datasets by DAVID online program and used the “ggplot” package of R language to illustrate the results of GO and KEGG analysis. P value <0.05 and counts ≥2 were considered statistically significant.
Construction of the PPI Network and Identification of Hub Genes
We used the STRING website (https://string-db.org) to construct the PPI network. The cutoff value was set to a confidence score ≥0.7. We analyzed the interaction relationship of DEGs using Cytoscape software (version 3.4.0; http://www.Cytoscape.org). The plug-in Molecular Complex Detection (MCODE) was used to obtain significant gene clusters and the scores of the clusters (selection criteria: degree cutoff=2; max depth=100; k-core=2; node score cutoff=0.2). The nodes were the genes with high degree which was classified by using CytoHubba. We used the algorithm Degree to select the top 10 hub genes that might have important roles in the pathogenesis of RA. P < 0.05 was considered to be statistically significant.
Results
Identification of DEGs
There were 2638 DEGs (1425 upregulated genes and 1213 downregulated genes) and 889 DEGs (438 upregulated genes and 451 downregulated genes) were selected from GSE97779 and GSE10500, respectively ( and ). Venn diagrams showed that 173 upregulated genes and 106 down-regulated genes existed in the both data sets. ( and ).
Figure 1 Differentially expressed genes (DEGs) expression Volcano plots and Venn diagram between rheumatoid patients and healthy controls. (A and B) Volcano plots of GSE97779 and GSE10500 Data points in red represent upregulated and in green represent downregulated genes. (C and D) Venn diagram show the Co-expression of the upregulated and downregulated genes of the GSE97779 and GSE10500 databases.
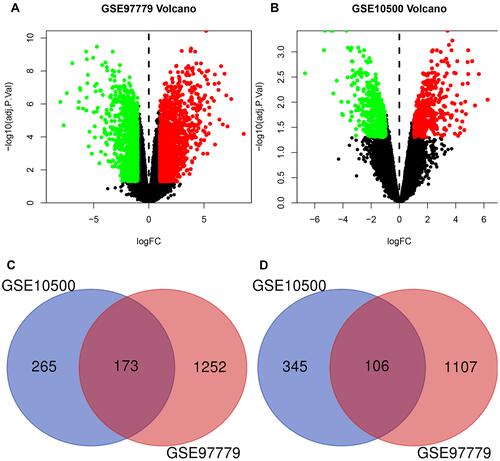
GO and KEGG Enrichment Analyses of the 173 Upregulated Genes and 106 Downregulated Genes
The results of GO functional analysis showed that DEGs were mainly enriched in inflammatory response, immune response, cell adhesion, positive regulation of gene expression, and extracellular matrix disassembly of biological process (BP), plasma membrane, extracellular exosome, extracellular space, extracellular region, and integral component of plasma membrane of CC (cellular component), and protein binding, protein homodimerization activity, identical protein binding, GTPase activator activity, and catalytic activity of MF (Molecular function) ().
Figure 2 Gene Ontology (GO) analysis of DEGs in the biological process (BP), molecular function (MF) and cellular component (CC). Select important GO entries with P < 0.05.
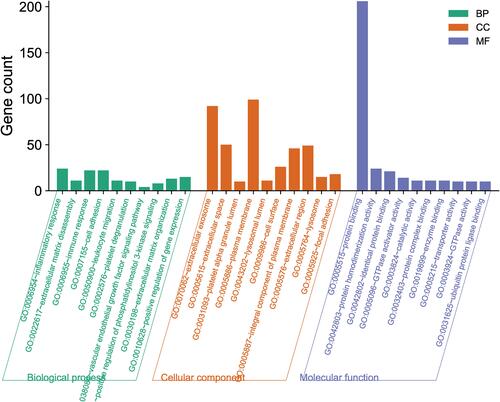
The results of KEGG pathway analysis suggested that DEGs were mainly enriched in PI3K-AKT signaling pathway, focal adhesion, lysosomes, transcriptional misregulation in cancer, hematopoietic cell lineage, acute myeloid leukemia, apoptosis, bladder cancer, prostate cancer, and HIF-1 signaling pathway (). The important signaling pathways—PI3K/AKT pathway is presented in .
Hub Genes and PPI Network Analysis
We used the STRING website to establish a PPI network, and Cytoscape to visualize the results. shows that the PPI network had a total of 168 nodes and 391 edges. and present the top two modules of the PPI network. illustrates the interactions with each other among the top 10 hub genes. The top 10 hub genes included FN1 (Fibronectin 1, degree: 24), VEGFA (Vascular Endothelial Growth Factor A, degree: 24), HGF (Hepatocyte Growth Factor, degree: 17), MMP9 (Matrix Metallopeptidase 9, degree: 17), PPBP (Pro-Platelet Basic Protein, degree: 16), CD44 (CD44 Molecule, Indian Blood Group, degree: 16), ITGAM (Integrin Subunit Alpha M, degree: 16), IGF1 (Insulin Like Growth Factor 1, degree: 15), SERPINA1 (Serpin Family A Member 1, degree: 15) and FPR2 (Formyl Peptide Receptor 2, degree: 15). Detailed information about the genes is listed in .
Table 1 Function and Connectivity of the 10 Hub Genes
Figure 4 PPI network of DEGs and four cluster modules extracted by MCODE. (A) The interaction network between proteins coded by DEGs was comprised of 188 nodes and 391 edges. Red nodes represent upregulated genes, blue nodes represent downregulated. Two significant modules identified from the PPI network using the MCODE with a score>5.0. Cluster 1 (B) had the highest cluster score (score = 8.125, 17 nodes and 65 edges), followed by cluster 2 (C) (score = 5.778, 19 nodes and 52 edges). The nodes represented as ellipse (green) and edges as lines (gray).
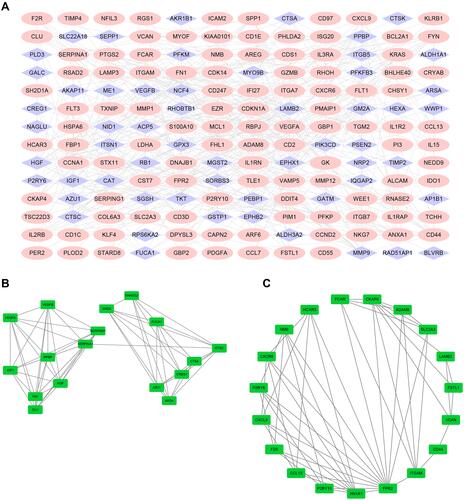
Discussion
In terms of pathogenesis, rheumatoid arthritis is related to chronic inflammation, and its severity is correlated with the levels of the pro-inflammatory cytokines derived from the macrophages in the synovium.Citation9 Increasing evidence shows that the infiltration of macrophages in the synovium is related to the degree of joint erosion, and macrophages can mediate arthritis together with other cells.Citation15,Citation16
The present study might provide a new insight into the phenotype of synovial macrophages and molecular mechanisms involved in RA. There were 279 DEGs in these two datasets, of which 173 were upregulated and 106 were downregulated. In order to further understand the interactions among these DEGs, we performed GO functional enrichment analysis and KEGG pathway enrichment analysis. GO analysis showed that DEGs were mostly enriched in inflammatory response, immune response, cell adhesion, positive regulation of gene expression, and extracellular matrix disassembly of biological process (BP). KEGG analysis suggested that DEGs participated in pathways related to immune regulation, such as PI3K-Akt pathway, focal adhesion, and lysosomes. These results were in accordance with previous findings in other research that immune abnormalities and inflammatory responses could contribute to autoimmune diseases like RA. One of the significantly enriched pathways, PI3K-Akt signaling pathway, was proved to be strongly related to the breakdown of synovium macrophages in RA.
In the pathogenesis of RA, the activation of PI3K can induce the formation of a cancer-like environment, which promotes the survival of multiple cells in the immune system, including macrophages/monocytes, FLS, and dendritic cells in the joint space.Citation17 The Akt pathway regulates cell survival, and the activation of Akt pathway in the macrophages of RA patients can inhibit apoptosis via upregulating MCL1 (myeloid cell leukemia sequence 1).Citation18 In addition, PI3K/Akt pathway can regulate the proliferation, survival, and migration of macrophages, and participate in the response to various inflammatory and metabolic signals in macrophages.Citation19,Citation20 Therefore, activating the PI3K-Akt signaling pathway can further promote cell proliferation, inhibit cell apoptosis, and elevate the expression levels of cytokines, leading to synovial hyperplasia in RA. Nonetheless, in-vitro and in-vivo research proved that different isoforms of PI3K/Akt had different functions in macrophage activation.Citation21 In addition, it was reported that inhibiting PI3K-Akt pathway not only played a role in ameliorating the injuries to the joints, but also led to apoptosis and death of synovial macrophages in RA patients, suggesting that PI3K/Akt pathway served as a potential treatment target for RA,Citation22 and the PI3K inhibitor ZSTK474 could inhibit articular inflammation in vivo.Citation23
Furthermore, in our study, we performed bioinformatics analysis to identify the top 10 genes highly associated with RA, including FN1, VEGFA, HGF, MMP9, PPBP, CD44, ITGAM, IGF1, SERPINA1 and FPR2, and the top 10 hub genes that might play important roles in the diagnosis, treatment, and prognosis of RA in the future.
MMP9 can degrade type IV collagen in the extracellular matrix and basement membrane. MMP9 can stimulate fibroblast-mediated inflammation, pannus formation, and cartilage degradation. In addition, it can aggravate the erosion of RA synovium and participate in the destruction of joints in RA.Citation24 In MMP-9 knockout mice, arthritis induced by antibody was ameliorated, implying that MMP-9 could promote arthritis in vivo.Citation25
CD44 can regulate cAMP-modulated signaling pathway, and under the stimulation of pro-inflammatory cytokines (TNF-α and IL-1), the level of CD44 in serum, plasma, and synovial fluid could be elevated.Citation26 Previous studies reported that CD44 was upregulated and accumulated on the surface of stimulated macrophages in RA.Citation3 Hyaluronic acid (HA) that can bind to CD44 is used as a target for RA treatment.Citation27 In addition, researchers found that monoclonal antibodies (mAbs) against CD44 could significantly ameliorate collagen-induced arthritis (CIA) in vivo models.Citation28
HGF can affect PI3K-AKT pathway in focal adhesion and ECM receptor pathways and compared with normal controls or patients with osteoarthritis, the expression of HGF in serum, synovium, and articular adipose significantly increases in RA patients.Citation29 In addition, HGF levels in the serum can be used to predict the severity of joint damage in RA patients.Citation30 Studies have shown that HGF can cause angiogenesis in articulation and pannus formation in patients with RA. Inhibition of HGF/c-Met pathway can significantly inhibit inflammation and bone loss in articulation of RA mice.Citation31
PPBP, a member of the CXC chemokine family, is a mitochondrial gene that plays a role in regulating the susceptibility to chronic inflammation-related diseases such as RA.Citation32 Beta-thromboglobulin (beta-TG), an alias for PPBP, was found to be markedly upregulated in female RA patients, compared with the control group.Citation33
IGF-1 is an important mediator in bone development and remodeling, which can promote the synthesis process and inhibit degradation activities of extracellular matrix.Citation34 Some research suggested that IGF1 could be used as a marker for RA-SF macrophages.Citation9 It has been reported that inflammation in hippocampus could interfere with the signal transduction of IGF1 receptors, resulting in neural complications of RA.Citation35 In clinical practice, the cachexia and critical condition of RA patients were related to the downregulation of IGF1,Citation36 while ameliorating inflammation by using anti-rheumatic therapies such as TNF inhibitors and corticosteroids rarely resulted in the recovery of IGF1 system.Citation37
VEGFA is a dominant endothelial growth factor, a core member of vascular formation, and can be stimulated by pro-inflammatory factors such as TNF-α, IL-1, and IL-6.Citation38 Studies have shown that compared with osteoarthritis or normal controls, the expression level of VEGFA increased significantly in synovial fluids in RA patients. In addition, VEGFA levels in the serum were correlated with the severity of RA, especially the number of swollen joints.Citation39 The VEGFA rs699947 C/A functional polymorphism may be related to the risk of elderly RA cases.Citation40
ALX (lipoxin A4 receptor), also known as FPR2, is a member of the Gi-protein coupled receptor (GiPCR) family and can be stimulated by Annexin A1-derived peptides, Annexin A1, lipoxin A4, or amyloid A, a reactant formed in the acute phase. Activated FPR can exert a powerful anti-inflammatory function in synovial tissues.Citation41 FPRs have an important part in CIA and antigen-induced arthritis.Citation42 An in-vivo experiment also indicated that the receptor had an inhibitory role in the FPR2-deficient mice with K/B × N serum transfer arthritis.Citation43
Although FN1, SERPINA1, and ITGAM have not been confirmed to be associated with RA, our results suggest that they might be potential biomarkers and are worthy of further investigation.
This research had some limitations. The sample size of this study was relatively small. The effects of comorbidity, gender, and medication (non-steroidal anti-inflammatory agents or methotrexate) were not eliminated, which might influence gene expression levels in the synovial macrophages in RA. In addition, macrophages extracted from RA samples should be used for in-vitro experiments to explore the molecular mechanisms related to gene expression. Preclinical in-vivo experiments should be conducted to explore the functions of the identified genes in the development of the disease. Future validation using polymerase chain reaction assays and Western blot and synovial macrophages in RA is required.
Conclusion
In summary, we identified 10 candidate genes associated with RA, including MMP9, VEGFA, HGF, PPBP, CD44, IGF1, FPR2, SERPINA1, FN1, and ITGAM, and explored the candidate genes and the relevant molecular mechanisms in RA patients. SERPINA1, FN1, and ITGAM, except for MMP9, VEGFA, HGF, PPBP, CD44, IGF1, and FPR2, were rarely mentioned in previous studies on RA. Our findings suggest that the 10 candidate genes might be used in diagnosis, prognosis, and therapy of RA in the future. Further studies are required to confirm the expression of these genes in synovial macrophages in RA and control specimen.
Highlights
● Integrated bioinformatics analysis of two profile datasets based on macrophage samples from RA patients and healthy controls was performed, and 173 upregulated and 106 downregulated common DEGs were identified.
● GO analysis showed that common DEGs were enriched in inflammatory response and immune response, and KEGG pathway illustrated that DEGs were mainly enriched in PI3K-Akt signaling pathway.
● FN1, VEGFA, HGF, SERPINA1, MMP9, PPBP, CD44, FPR2, IGF1, and ITGAM might be used in diagnosis, prognosis, and therapy of RA in the future.
Abbreviations
RA, rheumatoid arthritis; DEGs, differentially expressed genes; GEO, Gene Expression Omnibus; GO, Gene Ontology; KEGG, Kyoto Encyclopedia of Genes and Genomes; PPI, protein–protein interaction; HA, hyaluronic acid; mAbs, monoclonal antibodies; CIA, collagen-induced arthritis; GiPCR, Gi-protein coupled receptor.
Data Sharing Statement
The datasets used and/or analysed during the current study are available from the corresponding author on reasonable request.
Ethics Approval and Consent to Participate
Not applicable because GEO belongs to public databases, the patients involved in the database have obtained ethical approval, users can download relevant data for free for research and publish relevant articles, and our study is based on open-source data, and Guangzhou University of Chinese Medicine and the First Affiliated Hospital of Guangzhou University of Chinese Medicine specifically do not require research using publicly available data to be submitted for review to their ethics committee, so there are no ethical issues and other conflicts of interest.
Author Contributions
All authors made a significant contribution to the work reported, whether that is in the conception, study design, execution, acquisition of data, analysis, and interpretation, or in all these areas; took part in drafting, revising, or critically reviewing the article; gave final approval of the version to be published; have agreed on the journal to which the article has been submitted; and agree to be accountable for all aspects of the work.
Acknowledgments
Thanks to the support from the funding, and GEO database for providing their platforms and contributors for uploading their meaningful datasets.
Disclosure
The authors report no conflicts of interest in this work.
Additional information
Funding
References
- Scott DL, Wolfe F, Huizinga TW. Rheumatoid arthritis. Lancet. 2010;376(9746):1094–1108. doi:10.1016/S0140-6736(10)60826-4
- Safiri S, Kolahi AA, Hoy D, et al. Global, regional and national burden of rheumatoid arthritis 1990–2017: a systematic analysis of the global burden of disease study 2017. Ann Rheum Dis. 2019;78(11):1463–1471. doi:10.1136/annrheumdis-2019-215920
- Yang M, Feng X, Ding J, Chang F, Chen X. Nanotherapeutics relieve rheumatoid arthritis. J Control Release. 2017;252:108–124. doi:10.1016/j.jconrel.2017.02.032
- Firestein GS, McInnes IB. Immunopathogenesis of rheumatoid arthritis. Immunity. 2017;46(2):183–196. doi:10.1016/j.immuni.2017.02.006
- Fujiwara N, Kobayashi K. Macrophages in inflammation. Curr Drug Targets Inflamm Allergy. 2005;4(3):281–286. doi:10.2174/1568010054022024
- Xi ZD, Ge HL. Role of macrophages and their products in the pathogenesis of rheumatoid arthritis. Chin J Nat. 2009;31:262–267. in Chinese. doi:10.3969/j.issn.0253-9608.2009.05.004
- Siouti E, Andreakos E. The many facets of macrophages in rheumatoid arthritis. Biochem Pharmacol. 2019;165:152–169. PMID: 30910693. doi:10.1016/j.bcp.2019.03.029
- Yeo L, Adlard N, Biehl M, et al. Expression of chemokines CXCL4 and CXCL7 by synovial macrophages defines an early stage of rheumatoid arthritis. Ann Rheum Dis. 2016;75(4):763–771. doi:10.1136/annrheumdis-2014-206921
- Soler Palacios B, Estrada-Capetillo L, Izquierdo E, et al. Macrophages from the synovium of active rheumatoid arthritis exhibit an activin A-dependent pro-inflammatory profile. J Pathol. 2015;235(3):515–526. doi:10.1002/path.4466
- Okada Y, Eyre S, Suzuki A, Kochi Y, Yamamoto K. Genetics of rheumatoid arthritis: 2018 status. Ann Rheum Dis. 2019;78(4):446–453. doi:10.1136/annrheumdis-2018-213678
- Tang CH. Research of pathogenesis and novel therapeutics in arthritis. Int J Mol Sci. 2019;20(7):1646. doi:10.3390/ijms20071646
- Petryszak R, Burdett T, Fiorelli B, et al. Expression atlas update–a database of gene and transcript expression from microarray- and sequencing-based functional genomics experiments. Nucleic Acids Res. 2014;42(7):D926–932. doi:10.1093/nar/gkt1270
- Chen Y, Qiu F, Yu B, et al. Metformin, an AMPK activator, inhibits activation of FLSs but promotes HAPLN1 secretion. Mol Ther Methods Clin Dev. 2020;17:1202–1214. doi:10.1016/j.omtm.2020.05.008
- Cheng Q, Chen X, Wu H, Du Y. Three hematologic/immune system-specific expressed genes are considered as the potential biomarkers for the diagnosis of early rheumatoid arthritis through bioinformatics analysis. J Transl Med. 2021;19(1):18. doi:10.1186/s12967-020-02689-y
- Yanni G, Whelan A, Feighery C, Bresnihan B. Synovial tissue macrophages and joint erosion in rheumatoid arthritis. Ann Rheum Dis. 1994;53(1):39–44. doi:10.1136/ard.53.1.39
- Kinne RW, Bräuer R, Stuhlmüller B, Palombo-Kinne E, Burmester GR. Macrophages in rheumatoid arthritis. Arthritis Res. 2000;2(3):189–202. doi:10.1186/ar86
- Rana AK, Li Y, Dang Q, Yang F. Monocytes in rheumatoid arthritis: circulating precursors of macrophages and osteoclasts and, their heterogeneity and plasticity role in RA pathogenesis. Int Immunopharmacol. 2018;65:348–359. doi:10.1016/j.intimp.2018.10.016
- Vergadi E, Ieronymaki E, Lyroni K, Vaporidi K, Tsatsanis C. Akt signaling pathway in macrophage activation and M1/M2 polarization. J Immunol. 2017;198(3):1006–1014. doi:10.4049/jimmunol.1601515
- Li J, Hsu HC, Mountz JD. Managing macrophages in rheumatoid arthritis by reform or removal. Curr Rheumatol Rep. 2012;14(5):445–454. doi:10.1007/s11926-012-0272-4
- Hamerman JA, Pottle J, Ni M, He Y, Zhang ZY, Buckner JH. Negative regulation of TLR signaling in myeloid cells–implications for autoimmune diseases. Immunol Rev. 2016;269(1):212–227. doi:10.1111/imr.12381
- Chan TO, Rittenhouse SE, Tsichlis PN. AKT/PKB and other D3 phosphoinositide-regulated kinases: kinase activation by phosphoinositide-dependent phosphorylation. Annu Rev Biochem. 1999;68(1):965–1014. doi:10.1146/annurev.biochem.68.1.965
- Qi W, Lin C, Fan K, et al. Hesperidin inhibits synovial cell inflammation and macrophage polarization through suppression of the PI3K/AKT pathway in complete Freund’s adjuvant-induced arthritis in mice. Chem Biol Interact. 2019;306:19–28. doi:10.1016/j.cbi.2019.04.002
- Haruta K, Mori S, Tamura N, et al. Inhibitory effects of ZSTK474, a phosphatidylinositol 3-kinase inhibitor, on adjuvant-induced arthritis in rats. Inflamm Res. 2012;61(6):551–562. doi:10.1007/s00011-012-0444-8
- Daghestani HN, Pieper CF, Kraus VB. Soluble macrophage biomarkers indicate inflammatory phenotypes in patients with knee osteoarthritis. Arthritis Rheumatol. 2015;67(4):956–965. doi:10.1002/art.39006
- Itoh T, Matsuda H, Tanioka M, Kuwabara K, Itohara S, Suzuki R. The role of matrix metalloproteinase-2 and matrix metalloproteinase-9 in antibody-induced arthritis. J Immunol. 2002;169(5):2643–2647. doi:10.4049/jimmunol.169.5.2643
- Thorne RF, Wang Y, Zhang Y, et al. Evaluating nuclear translocation of surface receptors: recommendations arising from analysis of CD44. Histochem Cell Biol. 2020;153(2):77–87. doi:10.1007/s00418-019-01835-y
- Heo R, Park JS, Jang HJ, et al. Hyaluronan nanoparticles bearing γ-secretase inhibitor: in vivo therapeutic effects on rheumatoid arthritis. J Control Release. 2014;192:295–300. doi:10.1016/j.jconrel.2014.07.057
- Naor D, Nedvetzki S, Walmsley M, et al. CD44 involvement in autoimmune inflammations: the lesson to be learned from CD44-targeting by antibody or from knockout mice. Ann NY Acad Sci. 2007;1110(1):233–247. doi:10.1196/annals.1423.025
- Kontny E, Prochorec-Sobieszek M. Articular adipose tissue resident macrophages in rheumatoid arthritis patients: potential contribution to local abnormalities. Rheumatology. 2013;52(12):2158–2167. doi:10.1093/rheumatology/ket287
- Grandaunet B, Syversen SW, Hoff M, et al. Association between high plasma levels of hepatocyte growth factor and progression of radiographic damage in the joints of patients with rheumatoid arthritis. Arthritis Rheum. 2011;63(3):662–669. doi:10.1002/art.30163
- Hosonuma M, Sakai N, Furuya H, et al. Inhibition of hepatocyte growth factor/c-Met signalling abrogates joint destruction by suppressing monocyte migration in rheumatoid arthritis. Rheumatology. 2021;60(1):408–419. doi:10.1093/rheumatology/keaa310
- Strieter RM, Burdick MD, Mestas J, Gomperts B, Keane MP, Belperio JA. Cancer CXC chemokine networks and tumour angiogenesis. Eur J Cancer. 2006;42(6):768–778. doi:10.1016/j.ejca.2006.01.006
- Karatoprak C, Uyar S, Abanonu GB, Pehlevan SM, Okuroglu N, Demirtunc R. The levels of β-thromboglobulin in female rheumatoid arthritis patients as activation criteria. Rheumatol Int. 2013;33(5):1229–1232. doi:10.1007/s00296-012-2511-4
- Szeremeta A, Jura-Półtorak A, Komosińska-Vassev K, Zoń-Giebel A, Kapołka D, Olczyk K. The association between insulin-like growth factor 1 (IGF-1), IGF-binding proteins (IGFBPs), and the carboxyterminal propeptide of type I procollagen (PICP) in pre- and postmenopausal women with rheumatoid arthritis. Scand J Rheumatol. 2017;46(3):171–179. doi:10.1080/03009742.2016.1203020
- Andersson KME, Wasén C, Juzokaite L, et al. Inflammation in the hippocampus affects IGF1 receptor signaling and contributes to neurological sequelae in rheumatoid arthritis. Proc Natl Acad Sci USA. 2018;115(51):E12063–E12072. doi:10.1073/pnas.1810553115
- Erlandsson MC, Lyngfelt L, Åberg ND, et al. Low serum IGF1 is associated with hypertension and predicts early cardiovascular events in women with rheumatoid arthritis. BMC Med. 2019;17(1):141. doi:10.1186/s12916-019-1374-x
- Desai RJ, Solomon DH, Schneeweiss S, Danaei G, Liao KP, Kim SC. Tumor necrosis factor-α inhibitor use and the risk of incident hypertension in patients with rheumatoid arthritis. Epidemiology. 2016;27(3):414–422. doi:10.1097/EDE.0000000000000446
- Estrada CC, Maldonado A, Mallipattu SK. Therapeutic inhibition of VEGF signaling and associated nephrotoxicities. J Am Soc Nephrol. 2019;30(2):187–200. doi:10.1681/ASN.2018080853
- Zetterberg E, Palmblad J, Wallensten R, Morfini M, Melchiorre D, Holmström M. Angiogenesis is increased in advanced haemophilic joint disease and characterised by normal pericyte coverage. Eur J Haematol. 2014;92(3):256–262. doi:10.1111/ejh.12227
- Zhang Y, Qiu H, Zhang H, Wang L, Zhuang C, Liu R. Vascular endothelial growth factor A (VEGFA) polymorphisms in Chinese patients with rheumatoid arthritis. Scand J Rheumatol. 2013;42(5):344–348. doi:10.3109/03009742.2013.787454
- Lee HY, Lee M, Bae YS. Formyl peptide receptors in cellular differentiation and inflammatory diseases. J Cell Biochem. 2017;118(6):1300–1307. doi:10.1002/jcb.25877
- Odobasic D, Jia Y, Kao W, et al. Formyl peptide receptor activation inhibits the expansion of effector T cells and synovial fibroblasts and attenuates joint injury in models of rheumatoid arthritis. Int Immunopharmacol. 2018;61:140–149. doi:10.1016/j.intimp.2018.05.028
- Park YJ, Park B, Lee M, et al. A novel antimicrobial peptide acting via formyl peptide receptor 2 shows therapeutic effects against rheumatoid arthritis. Sci Rep. 2018;8(1):14664. doi:10.1038/s41598-018-32963-5