Abstract
Introduction
Atrial fibrillation (AF) is the most prevalent sustained cardiac arrhythmia, but the molecular mechanisms underlying AF are not known. We aimed to identify the pivotal genes and pathways involved in AF pathogenesis because they could become potential biomarkers and therapeutic targets of AF.
Methods
The microarray datasets of GSE31821 and GSE41177 were downloaded from the Gene Expression Omnibus database. After combining the two datasets, differentially expressed genes (DEGs) were screened by the Limma package. MicroRNAs (miRNAs) confirmed experimentally to have an interaction with AF were screened through the miRTarBase database. Target genes of miRNAs were predicted using the miRNet database, and the intersection between DEGs and target genes of miRNAs, which were defined as common genes (CGs), were analyzed. Functional and pathway-enrichment analyses of DEGs and CGs were performed using the databases DAVID and KOBAS. Protein–protein interaction (PPI) network, miRNA- messenger(m) RNA network, and drug-gene network was visualized. Finally, reverse transcription quantitative real-time polymerase chain reaction (RT-qPCR) was used to validate the expression of hub genes in the miRNA–mRNA network.
Results
Thirty-three CGs were acquired from the intersection of 65 DEGs from the integrated dataset and 9777 target genes of miRNAs. Fifteen “hub” genes were selected from the PPI network, and the miRNA-mRNA network, including 82 miRNAs and 9 target mRNAs, was constructed. Furthermore, with the validation by RT-qPCR, macrophage migration inhibitory factor (MIF), MYC proto-oncogene, bHLH transcription factor (MYC), inhibitor of differentiation 1 (ID1), and C-X-C Motif Chemokine Receptor 4 (CXCR4) were upregulated and superoxide Dismutase 2 (SOD2) was downregulated in patients with AF compared with healthy controls. We also found MIF, MYC, and ID1 were enriched in the transforming growth factor (TGF)-β and Hippo signaling pathway.
Conclusion
We identified several pivotal genes and pathways involved in AF pathogenesis. MIF, MYC, and ID1 might participate in AF progression through the TGF-β and Hippo signaling pathways. Our study provided new insights into the mechanisms of action of AF.
Introduction
Atrial fibrillation (AF) is a type of sustained arrhythmia characterized by rapid and disordered atrial electrical activity. AF is one of the leading causes of stroke, heart failure, cardiovascular disease, and sudden death.Citation1,Citation2 With the increasing age of populations, AF incidence is increasing worldwide.Citation3,Citation4 However, the pathophysiologic mechanisms of AF are incompletely understood.
Bioinformatic analysis and studies using microarrays to measure gene expression can be employed to screen molecular markers in patients and healthy individuals. This strategy can help to provide novel insights into diseases at multiple levels, ranging from alterations in the copy number at the genome level to gene expression at the transcriptome level (and even epigenetic alterations).Citation5,Citation6 Microarray studies are used commonly to obtain gene-expression profiles to uncover the pathogenesis of complicated diseases and for biomarker identification.
Using bioinformatics analysis, Wang et al discovered that Zinc finger and BTB domain containing 20 (ZBTB20), Erb-B2 receptor tyrosine kinase 4 (ERBB4), yin yang 1 (YY1), CAMP responsive element binding protein 1 (CREB1), BCL2 interacting protein 3 like (BNIP3L), AT-rich interactive domain-containing protein 1A (ARID1A), protein inhibitor of activated STAT 1 (PIAS1) and forkhead box O3 (FOXO3) may be beneficial for the early diagnosis and future treatment of AF.Citation7 Li and coworkers found that cyclin dependent kinase 1 (CDK1), CDK6, and cyclin D3 (CCND3) might be potential biomarkers for the diagnosis and targets for treatment of AF.Citation8 Nevertheless, the genes involved in AF pathogenesis have not been reported fully.
In the present study, datasets on the gene-expression profile of AF were downloaded from the Gene Expression Omnibus (GEO) database. Several pivotal differentially expressed genes (DEGs) and pathways were revealed. This information could help to identify biomarkers with high sensitivity in the diagnosis and treatment of AF.
Materials and Methods
Microarray Data
Gene-expression profiles of AF were collected from the GEO database (www.ncbi.nlm.nih.gov/geo).Citation9 The GSE31821 dataset includes the atrial tissues of two samples from healthy controls (HCs) and four patients with AF. The GSE41177 dataset contains 38 samples (32 patients with AF and six samples from HCs). The two datasets were both generated using GPL570 (HG-U133_Plus_2) Human Genome U133 Plus 2.0 Array (Affymetrix, Santa Clara, CA, USA).Citation10 The search term “atrial fibrillation” and the microRNAs (miRNAs) associated with AF were used in the miRTarBase database (http://mirtarbase.mbc.nctu.edu.tw/php/index.php). This is a database on interactions between miRNAs and targets that has been validated experimentally.Citation11 All data in the present study were collected from public databases, so ethical approval from our institution was not required. The study flowchart was presented in .
Identification of DEGs
We employed the robust multiarray average (RMA) algorithm in R 3.6.3 (www.R-project.org/). Datasets were analyzed with the Affymetrix platform to convert raw microarray data into expression values. This strategy was followed by background correction, quintile normalization, and probe summarization.Citation12 After merging the microarray datasets (GSE31821 and GSE41177), batch effects were adjusted by the “combat” function of the “sva” package of R using empirical Bayes frameworks.Citation13 The DEGs between patients with AF and HCs were screened using the Linear Models for Microarray Data (LIMMA, http://www.bioconductor.org/packages/release/bioc/html/limma.html) package in R Bioconductor.Citation14 Gene-expression values of | log2 (fold change, FC) | > 1 and p-value < 0.05 were chosen as cut-off criteria. Volcano maps and heatmaps of DEGs were constructed, respectively.
Analyses of Pathway Enrichment Using the Gene Ontology (GO) and Kyoto Encyclopedia of Genes and Genomes (KEGG) Databases
Database for Annotation, Visualization and Integration Discovery (DAVID; https://david.ncifcrf.gov/) is an online database that integrates considerable biological data and analytical tools.Citation15 KEGG Orthology-Based Annotation System (KOBAS, http://kobas.cbi.pku.edu.cn) is an online tool for functional annotation of genes/proteins and functional enrichment of gene sets.Citation16 For enrichment analyses, KOBAS can accept a gene list or gene-expression data as the input. Then, it generates enriched gene sets, the corresponding name, p-value, or a probability of enrichment and enrichment score based on the results of multiple methods. The GO database is used widely in bioinformatics analysis, and covers three aspects of biology: Biological Process (BP), Cellular Components (CC) and Molecular Function (MF).Citation17 GO annotations were made using DAVID on DEGs. Pathway analyses of DEGs were done using KOBAS. GO terms and KEGG pathways of biological functions associated with p-value < 0.05 and count number ≥ 2 were considered to be enriched significantly.
Construction of Protein-Protein Interaction (PPI) Network
The miRTarBase database (http://mirtarbase.mbc.nctu.edu.tw/php/index.php) is an experimentally validated database on miRNA–target interactions.Citation18 The miRNet database (http://www.mirnet.ca) contains information about miRNA–target interactions and displays the association in a visual network.Citation19 Target genes of miRNAs obtained from the miRTarBase database were selected within the miRNet database. An online tool (https://bioinfogp.cnb.csic.es/tools/venny/index.html) was used to present the intersection of DEGs and the target genes of miRNAs in the form of a Venn diagram. The Search Tool for the Retrieval of Interacting Genes/Proteins (STRING) database (http://string-db.org/) consolidates known data on predicted PPIs for many organisms. This strategy helps to uncover the direct (physical) and indirect (functional) relationships of DEGs.Citation20 Commonly expressed genes were uploaded to the STRING database. Then, a PPI network was established with the minimum required interaction score set as the medium confidence (>0.4). Subsequently, the PPI network was visualized by CytoScape 3.2.1 (https://cytoscape.org/).Citation21 The intersection of DEGs and target genes of miRNAs was selected for analyses of the regulation of the miRNA–mRNA network.
Construction of miRNA–mRNA Network in AF
The target miRNAs of commonly expressed genes (CGs) were predicted using the miRTarBase database. The miRNAs of CGs were included once they were found in the reporter assay. Western blotting or real-time reverse transcription-quantitative polymerase chain reaction (RT-qPCR) can also provide strong experimental evidence. Following screening, the miRNA–mRNA network was established further by CytoScape.
Drug-Gene Network Analysis
Consolidating data on gene druggability and drug-gene interactions is the purpose of the Drug-Gene Interaction database. The DGIdb database (http://www.dgidb.org/) was used to predict drug-gene pairings for the co-regulated genes.Citation22 Finally, the drug-gene network was developed using Cytoscape software.
Clinical Experimental Design
We recruited 5 patients with AF and 5 HCs between October 15, 2021, and October 30, 2021 to further confirm the reliability of 9 hub genes selected from the miRNA–mRNA network. The inclusion and exclusion criteria for AF patients and HCs were listed in Appendix 1. The basic characteristic information of patients and HCs were displayed in Supplementary Table 1. Blood samples of all the participants were taken on an empty stomach in the morning on the first day of admission. The clinical blood collection scheme was approved by the Ethics Committee of the Affiliated Hospital of Xuzhou Medical University [Approval number: XYFY2021-KL302]. All volunteers were recruited by the Affiliated Hospital of Xuzhou Medical University and provided written informed consent to the blood samples used in the experiment. The informed consent details are available in Appendix 2.
Reverse Transcription-Quantitative Real-Time Polymerase Chain Reaction (RT-qPCR)
Total RNA was extracted from peripheral blood using TRIzol® Reagent according to the manufacturer’s instructions (TaKaRa Biotechnology, Shiga, Japan). The iScriptTM cDNA Synthesis Kit (Bio-Rad Laboratories, Hercules, CA, USA) was then used to reverse transcription 600ng of extracted total RNA to synthesis complementary DNA (cDNA).
The ABI-384 Real-Time PCR Detection System (Applied Biosystems, Foster City, CA, USA) was used to quantify mRNA expression with the appropriate proportions of SYBR™ Green qPCR Master Mix kit (Bio-Rad Laboratories), cDNA and deionized water using the comparative quantification method (2−ΔΔCT). β-actin was used as the internal reference. A list of the primer sequences for the genes are shown in Supplementary Table 2.
Statistical Analyses
All data were performed using SPSS 22.0 (IBM Corporation, Armonk, NY) and GraphPad Prism 7 (GraphPad, San Diego, CA). Continuous variables were expressed as mean ± standard deviation, and classified variables were represented by N (%). The chi-square test was used to calculate the differences in the rates between patients and HCs. Independent samples t test was used to analyze differences in general characteristics and the hub gene expression levels between patients and HCs. P-value < 0.05 was selected to indicate a statistically.
Results
Identification of DEGs
A total of 65 DEGs were screened from the integrated dataset: expression of 49 genes was upregulated and expression of 16 genes was downregulated between AF patients and HCs (). The cluster heatmaps of all DEGs are shown in , and all DEGs are displayed in Supplementary Table 3.
Figure 2 Volcano plots and Heatmap for the DEGs identified from the integrated dataset.
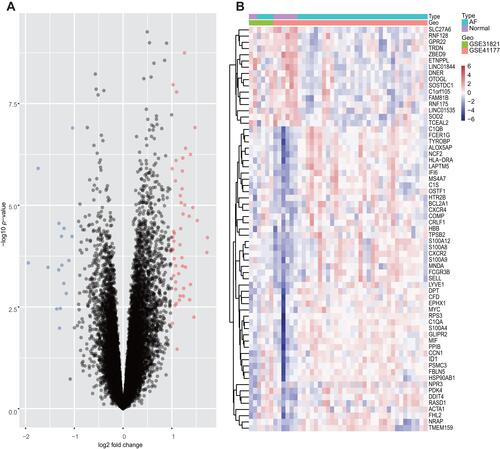
Pathway Enrichment of DEGs Using GO and KEGG Databases
Analyses using the GO database showed that the DEGs were enriched mainly in BPs, including “innate immune response”, “neutrophil chemotaxis”, and “negative regulation of the apoptotic process”. With regard to CC, DEGs were enriched in “extracellular exosome”, “extracellular region”, and “cell surface”. With respect to MF, the DEGs were enriched significantly in “RAGE receptor binding”, “arachidonic acid binding”, and “protein binding” (Supplementary Table 4). Pathway enrichment-analyses using the KEGG database showed that DEGs were expressed mainly in the “TGF-β signaling pathway” and “Hippo signaling pathway” (, Supplementary Table 5).
Figure 3 Terms of BP, CC and MF in pathway-enrichment analyses for DEGs using the GO database.
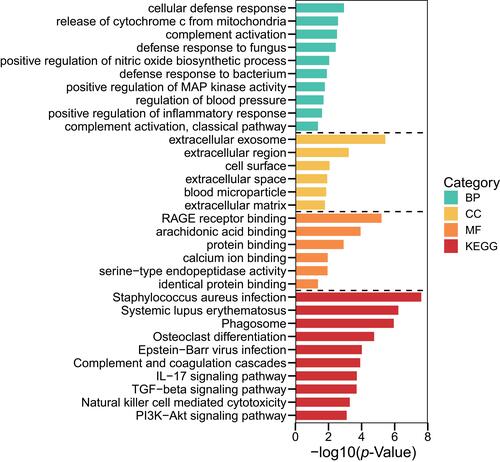
Analyses of PPI Networks
To further explore the molecular mechanism of AF, target genes of mRNAs were predicted using the miRNet database. A total of 9777 genes were predicted as target genes of miRNAs, of which 33 genes overlapped with DEGs (). For a combined score > 0.4, the STRING database was utilized to construct a PPI network with the intersection of 33 DEGs. After removal of isolated nodes, the PPI network was constructed with 15 nodes and 13 edges using CytoScape (). – and Supplementary Tables 6 and 7 reveals that these 33 common genes were enriched mainly in the TGF-β signaling pathway and Hippo signaling pathway.
Figure 4 Venn diagram, PPI network and the functional terms and pathways enriched for common genes.
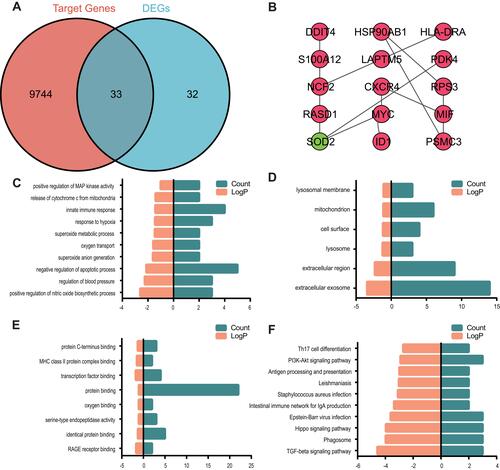
Analyses of Regulatory miRNA–mRNA Networks
A regulatory miRNA–mRNA network was constructed. It consisted of 91 nodes and 120 edges, including 9 target mRNAs and 82 miRNAs (). Nodes of degree ≥ 2 in the mRNA–miRNA network are shown in . Also, hsa-miRNA-212-3p, hsa-miRNA-494-3, hsa-miRNA-335-5, hsa-miRNA-451a and hsa-miRNA-146a-5p interacted with ≥2 mRNAs simultaneously ().
Table 1 The Nodes with Degree of ≥ 2 in the mRNA-miRNA Network
Drug-Gene Network Analysis
For the 9 common genes that interact with predicted miRNAs, 79 drug-gene pairs were acquired, including 7 common genes (including Macrophage migration inhibitory factor (MIF), MYC proto-oncogene, bHLH transcription factor (MYC), Pyruvate Dehydrogenase Kinase 4 (PDK4), C-X-C Motif Chemokine Receptor 4 (CXCR4), S100 Calcium Binding Protein A12 (S100A12), DNA Damage Inducible Transcript 4 (DDIT4) and Superoxide Dismutase 2 (SOD2) and 77 drugs ().
Validation of Hub Genes
The RT-qPCR was used to confirm the expression levels of MIF, MYC, CXCR4, SOD2, inhibitor of differentiation 1 (ID1), DDIT4, S100A12, Ras related dexamethasone induced 1 (RASD1), and PDK4. As demonstrated in , the expression levels of MIF, MYC, ID1, and CXCR4 were significantly higher in AF samples, as compared to HCs (all p-value <0.05). The expression of SOD2 was significantly downregulated in AF patients when compared to HCs (p-value <0.05). The findings of the RT-qPCR analysis in our research were consistent with the results of bioinformatics analysis. However, there was no difference in the expression of PDK4, S100A12, RASD1, or DDIT4 between AF patients and HCs.
Figure 7 Validation of hub genes in AF patients.
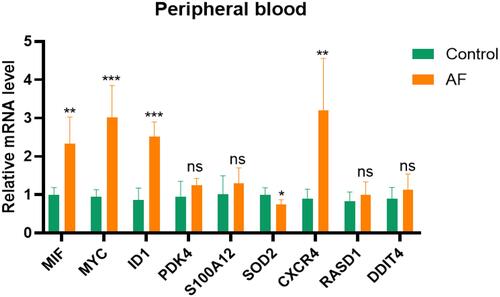
Discussion
The mechanism of AF is incompletely understood. Therefore, the early diagnosis of AF is the focus of basic scientific and clinical research.Citation1 Bioinformatics analysis of AF has focused mainly on DEGs using GEO datasets. However, there are few datasets on AF. We concentrated on the miRNAs related to AF as demonstrated by clinical trials, RT-qPCR, Western blotting, and luciferase assays. Moreover, the samples we used were from human atrial tissues. To research the biological function of common genes, we conducted enrichment analysis, constructed PPI, miRNA–mRNA and drug-gene networks. We speculated that the TGF-β and the Hippo signaling pathway might have vital roles in AF progression revealing from the KOBAS database. The genes associated with the TGF-β signaling pathway and the Hippo signaling pathway included MIF, MYC, and ID1, which were illustrated in the PPI network as well.
The TGF-β signaling pathway regulates the proliferation and differentiation of cells as well as fibrosis development.Citation23 Studies have demonstrated AF pathogenesis is related mainly to structural remodeling of atria involving cardiomyocyte apoptosis, inflammation, and activation of fibrotic pathways via fibroblasts.Citation24,Citation25 Moreover, the relationship among TGF-β, fibrosis and AF, as well as partial regulatory mechanisms, have been shown. Wang et alCitation26 demonstrated that long non-coding RNA predicting cardiac remodeling (lnc LIPCAR) might regulate atrial fibrosis via the TGF-β signaling pathway in samples from AF patients. Besides, Qiu and collaboratorsCitation27 found that salvianolate could suppress atrial interstitial fibrosis by inhibiting the TGF-β signaling pathway in AF rats. Our results confirmed the function of the TGF-β signaling pathway in AF pathogenesis.
The Hippo signaling pathway regulates the survival, proliferation, and apoptosis of cells. It has an important role in development/regeneration and diseases of the heart.Citation28,Citation29 However, few researchers have focused on the function of the Hippo signaling pathway in AF. Zhang et alCitation30 and Li and coworkersCitation31 suggested the role of the Hippo signaling pathway in AF regulation by bioinformatics analysis. Dysregulation of the Hippo signaling pathway in the cardiovascular system has been reported to be associated with myocardial infarction (MI), cardiac hypertrophy and vascular remodeling.Citation32 Ramjee et alCitation33 found that deficiency of two core effectors of the Hippo signaling pathway, Yes-associated protein (YAP) and transcription regulator protein 1 (TAZ), could lead to the post-MI pericardial inflammation and myocardial fibrosis in mice, which suggested the profound effect of the Hippo signaling pathway in MI. Yang and collaboratorsCitation34 found that YAP could promote cardiomyocyte growth, and cause hypertrophy in postnatal hearts. He et alCitation35 discovered that the Hippo/YAP signaling pathway alters the growth, death, and migration of vascular smooth muscle cells and endothelial cells, which contributes to vascular remodeling in cardiovascular diseases. In the progression of cardiac hypertrophy and vascular remodeling, fibrosis is vital.Citation36,Citation37 In this study, we showed that the Hippo signaling pathway participates in AF. Upon consideration of PPI networks, we found that MYC, ID1, and MIF were involved in the pathways mentioned above, thereby suggesting their roles in AF pathogenesis.
MIF, MYC, ID1, and CXCR4 had substantially higher levels of expression and SOD2 was downregulated in patients with AF compared to HCs. MIF is a pleiotropic inflammatory cytokine which shows high expression in patients with AF. Several studies have demonstrated its detailed mechanism of action in AF progression. Cheng et alCitation38 demonstrated that MIF might promote cardiac fibrosis in AF patients by activating the TGF-β signaling pathway. The relationship between MIF and Hippo is not known. We speculate that, because Hippo might be related to AF, and MIF was found to take part in the Hippo signaling pathway, then MIF might function in AF through the Hippo signaling pathway. MYC is a proto-oncogene implicated in regulation of the formation and metastasis of tumors. Yee and collaboratorsCitation39 discovered that polymorphisms in MYC-related genes lead to various bleeding complications in patients with a stable response to warfarin (which is used commonly in AF). Zhang and coworkersCitation40 found that TGF-β expression could be regulated by long noncoding RNA regulator of reprogramming through regulation of c-MYC expression. Xiao et alCitation41 found that Yap (a pivotal molecule in the Hippo signaling pathway) activates MYC directly in response to cardiac injury. Based on the relationship among genes and pathways in cardiac models, genes and AF, AF and pathways, we postulate that MYC might participate in AF pathogenesis. ID1 is a regulator of the cell cycle and cell differentiation.Citation42 ID1 expression in the heart is confined to non-myocardial layers. ID1 is essential for development of the neonatal heart and has important roles in embryogenesis.Citation43 Sato and collaboratorsCitation44 found that ID1 blocks TGF-β-induced apoptosis of mesangial cells. Lin et alCitation45 revealed that ID1 could mediate the fibroblast response to TGF-β in pulmonary fibrosis. Fibrosis is related to TGF-β and Hippo signaling pathways. Therefore, we speculate that ID1 might participate in TGF-β and Hippo signaling pathways in AF. Chen M found that by increasing antioxidant genes such as SOD2 and UCP3, fibroblast growth factor 21 successfully reduced atrial remodeling by lowering oxidative stress.Citation46 In addition, Wang et al revealed that CXCR4 expression is increased in chronic AF patients with mitral valve dysfunction, that it is related with atrial remodeling.Citation47
However, no difference in the expression of PDK4, S100A12, RASD1, or DDIT4 was seen between AF patients and HCs. Raman K demonstrated that PDK4 is a useful biomarker for discriminating between atrial fibrillation and sinus rhythm.Citation48 Via the least absolute shrinkage and selection operator model, Liu L identified that S100A12 is potentially involved in the molecular mechanisms of AF development.Citation49 The relationship between RASD1, DDIT4 and AF has not been reported. However, the involvement of the RASD1 and DDIT4 genes in AF requires more investigation.
Our study had three main limitations. First, we created the PPI network with the minimum required interaction score of medium confidence (>0.4) in order to obtain more proteins related with AF. Second, we concentrated only on reverse regulation of miRNA–mRNA pairs and ignored the more complicated mechanisms of miRNA–mRNA pairs. Finally, the miRNA and mRNA we obtained were not from identical samples.
Conclusions
In the study, we identified 9 pivotal genes and several related pathways involved in AF pathogenesis. We further verified experimentally that MIF, MYC, CXCR4, and ID1 were up-regulated and SOD2 was down-regulated in patients with AF. Based on the miRNA-mRNA network, 11 pairs of miRNA-mRNA pairs associated with AF were identified, including miR-335-5p/CXCR4, miR-335-5p/MYC, miR-494-3p/CXCR4, miR-494-3p/MYC, miR-451a/MYC, miR-451a/MIF, miR-146-5p/CXCR4, miR-146-5p/MIF, miR-146-5p/S100A12, miR-212-3p/SOD2, miR-212-3p/MYC, that might regulate development and progression of AF. In addition, MIF, MYC, and ID1 might participate in AF progression through TGF-β and Hippo signaling pathways. Our study provides new insights into AF mechanisms.
Data Sharing Statement
The data used to support the findings of this study are available from the corresponding author upon request.
Author Contributions
All authors contributed significantly to the conception and design, data acquisition, and data analysis and interpretation; participated in the drafting of the article or critically revised it for important intellectual content; agreed to submit to the current journal; gave final approval of the version to be published; and agreed to be accountable for all aspects of the work.
Disclosure
The authors declare that they have no competing interests.
Additional information
Funding
References
- Killu AM, Granger CB, Gersh BJ. Risk stratification for stroke in atrial fibrillation: a critique. Eur Heart J. 2019;40(16):1294–1302.
- Kirchhof P, Benussi S, Kotecha D, et al. [2016 ESC Guidelines for the management of atrial fibrillation developed in collaboration with EACTS]. Kardiol Pol. 2016;74(12):1359–1469. Polish. doi:10.5603/KP.2016.0172
- Lip GY, Fauchier L, Freedman SB, et al. Atrial fibrillation. Nat Rev Dis Primers. 2016;2:16016. doi:10.1038/nrdp.2016.16
- Xiao S, Zhou Y, Liu Q, Zhang T, Pan D. Identification of pivotal MicroRNAs and target genes associated with persistent atrial fibrillation based on bioinformatics analysis. Comput Math Methods Med. 2021;2021:6680211. doi:10.1155/2021/6680211
- Li N, Li L, Chen Y. The identification of core gene expression signature in hepatocellular carcinoma. Oxid Med Cell Longev. 2018;2018:3478305. doi:10.1155/2018/3478305
- Xiao S, Zhou Y, Liu A, et al. Uncovering potential novel biomarkers and immune infiltration characteristics in persistent atrial fibrillation using integrated bioinformatics analysis. Math Biosci Eng. 2021;18(4):4696–4712. doi:10.3934/mbe.2021238
- Wang T, Wang B. Identification of microRNA-mRNA interactions in atrial fibrillation using microarray expression profiles and bioinformatics analysis. Mol Med Rep. 2016;13(6):4535–4540. doi:10.3892/mmr.2016.5106
- Li Y, Tan W, Ye F, et al. Identification of microRNAs and genes as biomarkers of atrial fibrillation using a bioinformatics approach. J Int Med Res. 2019;47(8):3580–3589. doi:10.1177/0300060519852235
- Clough E, Barrett T. The gene expression omnibus database. Methods Mol Biol. 2016;1418:93–110.
- Barrett T, Wilhite SE, Ledoux P, et al. NCBI GEO: archive for functional genomics data sets–update. Nucleic Acids Res. 2013;41(Database issue):D991–5. doi:10.1093/nar/gks1193
- Chou CH, Shrestha S, Yang CD, et al. miRTarBase update 2018: a resource for experimentally validated microRNA-target interactions. Nucleic Acids Res. 2018;46(D1):D296–d302. doi:10.1093/nar/gkx1067
- Gautier L, Cope L, Bolstad BM, Irizarry RA. affy–analysis of Affymetrix GeneChip data at the probe level. Bioinformatics. 2004;20(3):307–315. doi:10.1093/bioinformatics/btg405
- Leek JT, Johnson WE, Parker HS, Jaffe AE, Storey JD. The sva package for removing batch effects and other unwanted variation in high-throughput experiments. Bioinformatics. 2012;28(6):882–883. doi:10.1093/bioinformatics/bts034
- Ritchie ME, Phipson B, Wu D, et al. limma powers differential expression analyses for RNA-sequencing and microarray studies. Nucleic Acids Res. 2015;43(7):e47. doi:10.1093/nar/gkv007
- Huang DW, Sherman BT, Tan Q, et al. The DAVID gene functional classification tool: a novel biological module-centric algorithm to functionally analyze large gene lists. Genome Biol. 2007;8(9):R183. doi:10.1186/gb-2007-8-9-r183
- Wu J, Mao X, Cai T, Luo J, Wei L. KOBAS server: a web-based platform for automated annotation and pathway identification. Nucleic Acids Res. 2006;34(WebServer issue):W720–W724. doi:10.1093/nar/gkl167
- Ashburner M, Ball CA, Blake JA, et al. Gene ontology: tool for the unification of biology. Gene Ontol Consortium Nat Genet. 2000;25(1):25–29.
- Huang HY, Lin YC, Li J, et al. miRTarBase 2020: updates to the experimentally validated microRNA-target interaction database. Nucleic Acids Res. 2020;48(D1):D148–d54. doi:10.1093/nar/gkz896
- Fan Y, Xia J. miRNet-functional analysis and visual exploration of miRNA-target interactions in a network context. Methods Mol Biol. 2018;1819:215–233.
- Szklarczyk D, Franceschini A, Wyder S, et al. STRING v10: protein-protein interaction networks, integrated over the tree of life. Nucleic Acids Res. 2015;43(Database issue):D447–52. doi:10.1093/nar/gku1003
- Kohl M, Wiese S, Warscheid B. Cytoscape: software for visualization and analysis of biological networks. Methods Mol Biol. 2011;696:291–303.
- Wagner AH, Coffman AC, Ainscough BJ, et al. DGIdb 2.0: mining clinically relevant drug-gene interactions. Nucleic Acids Res. 2016;44(D1):D1036–44. doi:10.1093/nar/gkv1165
- Zhang Y, Alexander PB, Wang XF. TGF-β family signaling in the control of cell proliferation and survival. Cold Spring Harb Perspect Biol. 2017;9(4):a022145. doi:10.1101/cshperspect.a022145
- Nattel S. Molecular and cellular mechanisms of atrial fibrosis in atrial fibrillation. JACC Clin Electrophysiol. 2017;3(5):425–435.
- Hu YF, Chen YJ, Lin YJ, Chen SA. Inflammation and the pathogenesis of atrial fibrillation. Nat Rev Cardiol. 2015;12(4):230–243. doi:10.1038/nrcardio.2015.2
- Wang H, Song T, Zhao Y, Zhao J, Wang X, Fu X. Long non-coding RNA LICPAR regulates atrial fibrosis via TGF-β/Smad pathway in atrial fibrillation. Tissue Cell. 2020;67:101440. doi:10.1016/j.tice.2020.101440
- Qiu H, Liu W, Lan T, et al. Salvianolate reduces atrial fibrillation through suppressing atrial interstitial fibrosis by inhibiting TGF-β1/Smad2/3 and TXNIP/NLRP3 inflammasome signaling pathways in post-MI rats. Phytomedicine. 2018;51:255–265. doi:10.1016/j.phymed.2018.09.238
- Zheng Y, Pan D. The hippo signaling pathway in development and disease. Dev Cell. 2019;50(3):264–282. doi:10.1016/j.devcel.2019.06.003
- Zhou Q, Li L, Zhao B, Guan KL. The hippo pathway in heart development, regeneration, and diseases. Circ Res. 2015;116(8):1431–1447. doi:10.1161/CIRCRESAHA.116.303311
- Zhang H, Yang G, Zhong N, et al. Possible key microRNAs and corresponding molecular mechanisms for atrial fibrillation. Anatol J Cardiol. 2020;23(6):324–333. doi:10.14744/AnatolJCardiol.2020.39483
- Li W, Wang L, Wu Y, Yuan Z, Zhou J. Weighted gene co‑expression network analysis to identify key modules and hub genes associated with atrial fibrillation. Int J Mol Med. 2020;45(2):401–416. doi:10.3892/ijmm.2019.4416
- Heallen T, Zhang M, Wang J, et al. Hippo pathway inhibits Wnt signaling to restrain cardiomyocyte proliferation and heart size. Science. 2011;332(6028):458–461. doi:10.1126/science.1199010
- Ramjee V, Li D, Manderfield LJ, et al. Epicardial YAP/TAZ orchestrate an immunosuppressive response following myocardial infarction. J Clin Invest. 2017;127(3):899–911. doi:10.1172/JCI88759
- Yang Y, Del Re DP, Nakano N, et al. miR-206 mediates YAP-induced cardiac hypertrophy and survival. Circ Res. 2015;117(10):891–904. doi:10.1161/CIRCRESAHA.115.306624
- He J, Bao Q, Yan M, et al. The role of Hippo/yes-associated protein signalling in vascular remodelling associated with cardiovascular disease. Br J Pharmacol. 2018;175(8):1354–1361. doi:10.1111/bph.13806
- Ni G, Wang K, Zhou Y, et al. Citri reticulatae Pericarpium attenuates Ang II-induced pathological cardiac hypertrophy via upregulating peroxisome proliferator-activated receptors gamma. Ann Transl Med. 2020;8(17):1064. doi:10.21037/atm-20-2118
- Li Y, Ren W, Wang X, et al. MicroRNA-150 relieves vascular remodeling and fibrosis in hypoxia-induced pulmonary hypertension. Biomed Pharmacother. 2019;109:1740–1749. doi:10.1016/j.biopha.2018.11.058
- Cheng WL, Kao YH, Chen YC, Lin YK, Chen SA, Chen YJ. Macrophage migration inhibitory factor increases atrial arrhythmogenesis through CD74 signaling. Transl Res. 2020;216:43–56. doi:10.1016/j.trsl.2019.10.002
- Yee J, Kim W, Chang BC, Chung JE, Lee KE, Gwak HS. Effects of polymorphisms in Myc-related genes on bleeding complications in patients with stable warfarin responses. Cardiovasc Ther. 2019;2019:1813747. doi:10.1155/2019/1813747
- Zhang L, Luo B, Dang YW, et al. The clinical significance of endothelin receptor type B in hepatocellular carcinoma and its potential molecular mechanism. Exp Mol Pathol. 2019;107:141–157. doi:10.1016/j.yexmp.2019.02.002
- Xiao Y, Hill MC, Li L, et al. Hippo pathway deletion in adult resting cardiac fibroblasts initiates a cell state transition with spontaneous and self-sustaining fibrosis. Genes Dev. 2019;33(21–22):1491–1505. doi:10.1101/gad.329763.119
- Zhao Z, Bo Z, Gong W, Guo Y. Inhibitor of differentiation 1 (Id1) in cancer and cancer therapy. Int J Med Sci. 2020;17(8):995–1005. doi:10.7150/ijms.42805
- Zhao Q, Beck AJ, Vitale JM, et al. Developmental ablation of Id1 and Id3 genes in the vasculature leads to postnatal cardiac phenotypes. Dev Biol. 2011;349(1):53–64. doi:10.1016/j.ydbio.2010.10.004
- Sato AY, Antonioli E, Tambellini R, Campos AH. ID1 inhibits USF2 and blocks TGF-β-induced apoptosis in mesangial cells. Am J Physiol Renal Physiol. 2011;301(6):F1260–9. doi:10.1152/ajprenal.00128.2011
- Lin L, Zhou Z, Zheng L, et al. Cross talk between Id1 and its interactive protein Dril1 mediate fibroblast responses to transforming growth factor-beta in pulmonary fibrosis. Am J Pathol. 2008;173(2):337–346. doi:10.2353/ajpath.2008.070915
- Chen M, Zhong J, Wang Z, et al. Fibroblast growth factor 21 protects against atrial remodeling via reducing oxidative stress. Front Cardiovasc Med. 2021;8:720581. doi:10.3389/fcvm.2021.720581
- Wang XX, Zhang FR, Zhu JH, Xie XD, Chen JZ. Up-regulation of CXC chemokine receptor 4 expression in chronic atrial fibrillation patients with mitral valve disease may be attenuated by renin-angiotensin system blockers. J Int Med Res. 2009;37(4):1145–1151. doi:10.1177/147323000903700419
- Raman K, Aeschbacher S, Bossard M, et al. Whole blood gene expression differentiates between atrial fibrillation and sinus rhythm after cardioversion. PLoS One. 2016;11(6):e0157550. doi:10.1371/journal.pone.0157550
- Liu L, Yu Y, Hu LL, et al. Potential target genes in the development of atrial fibrillation: a comprehensive bioinformatics analysis. Med Sci Monit. 2021;27:e928366. doi:10.12659/MSM.928366