Abstract
Background
The heterogeneity and dynamic changes of endometrial cells have a significant impact on health as they determine the normal function of the endometrium during the menstrual cycle. Dysfunction of the endometrium can lead to the occurrence of various gynecological diseases. Therefore, deconvolution of immune microenvironment that drives transcriptional programs throughout the menstrual cycle is key to understand regulatory biology of endometrium.
Methods
Herein, we comprehensively analyzed single-cell transcriptome of 59,397 cells across ten human endometrium samples and revealed the dynamic cellular heterogeneity throughout the menstrual cycle.
Results
We identified two perivascular cell subtypes, four epithelial subtypes and four fibroblast cell types in endometrium. Moreover, we inferred the cell type-specific transcription factor (TF) activities and linked critical TFs to transcriptional output of diverse immune cell types, highlighting the importance of transcriptional regulation in endometrium. Dynamic interactions between various types of cells in endometrium contribute to a range of biological pathways regulating differentiation of secretory. Integration of the molecular biomarkers identified in endometrium and bulk transcriptome of 535 endometrial cancers (EC), we revealed five RNA-based molecular subtypes of EC with highly intratumoral heterogeneity and different clinical manifestations. Mechanism analysis uncovered clinically relevant pathways for pathogenesis of EC.
Conclusion
In summary, our results revealed the dynamic immune microenvironment of endometrium and provided novel insights into future development of RNA-based treatments for endometriosis and endometrial carcinoma.
Introduction
The endometrium is the layer forming the inner wall of the uterus of mammals. It reacts to both estrogen and progesterone, and therefore changes significantly with the estrus cycle and menstrual cycle. Dysfunctions of endometrium had been associated with various human diseases, including abnormal uterine bleeding, infertility, pre-eclampsia, endometriosis and endometrial carcinoma (EC).Citation1,Citation2 Thus, understanding the gene expression regulation during menstrual cycle in humans is crucial for understanding normal functions of endometrium and the mechanism of EC. It also provides important insights for precision medicine in endometrium-related diseases.
Significant efforts have been made to understand the pathogenesis and identify biomarkers for endometrium-related diseases, such as endometrial cancer. Anna et al found that ncRNA plays a significant role in endometrial cancer and is associated with different clinical prognostic outcomes.Citation3 These results suggested that, in addition to clinicopathological stratification, the inherent genetic and epigenetic characteristics of EC patients are also crucial factors in the precision medicine of oncology.Citation4 With the development of high throughput sequencing technologies, single-cell RNA sequencing (scRNA-seq) as a revolutionary technology can uncover novel cell types, explore genetic and functional heterogeneity in various cellular contexts. Compared to traditional bulk RNA sequencing data, which only assesses the average expression levels of all cells in a mixed tissue, scRNA-seq performs sequencing at the level of individual cells, offering higher resolution. This not only reveals cell types and heterogeneity but also offers a deeper understanding of specific cell functions and dynamic changes, delivering more detailed and precise insights than traditional approaches. Recently, scRNA-seq has been used to understandthe potential mechanisms of reproductive diseases. Wang et al utilized scRNA-seq to analyze the cellular and molecular signatures of decidual and peripheral leukocytes in normal and unexplained recurrent miscarriage.Citation5 Liu et al performed a scRNA-seq analysis of adenomyosis and supported the theory of adenomyosis derived from the invasion and migration of the endometrium.Citation6 Ma et al performed single-cell analysis and identified nine cell types, and determined a potential developmental trajectory associated with endometriosis.Citation7 One recent study mapped the temporal and spatial dynamics of the human endometrium in vivo and in vitro.Citation8 Guo et al presented a view of endometrial carcinoma at single-cell resolution and revealed the characteristics of endometrial epithelial cells in the endometrium.Citation9 However, the dynamic immune microenvironment in human endometrium, including cellular composition, cellular states, and cell–cell interactions, remains unclear.
Moreover, EC is the most commonly diagnosed gynecologic malignancy.Citation10 Traditional classification of EC is primary based either on clinical and endocrine features.Citation10,Citation11 Histological subtyping is commonly used in clinical to guide prognosis and treatment decisions for EC patients, while ongoing researches are evaluating the potential molecular subtyping. An integrated genomic analysis had resulted in the molecular classification of endometrioid and serous carcinomas into four distinct subgroups.Citation12 The subtypes identified by the different classification systems correlate to some extent; however, the potential molecular pathways of different subtypes of EC are still unknown.
Therefore, we interrogate the immune microenvironment of human endometrial cells during the proliferative and secretory phases of women menstrual cycle. We identified the diverse cell types in human endometrial and characterization of the RNA expression patterns in different cell types. In particular, we explored the transcriptional regulation and revealed the transcription factors (TFs) that exhibited high activities in cell types. Cell–cell communications were examined and further uncovered five RNA-based molecular subtypes of EC.
Materials and Methods
Transcriptome of Human Endometrium and Endometrial Cancers
Single-cell RNA-sequencing was used to create a cell census of the human endometrium. We downloaded the raw sequencing data from ArrayExpress under the accession number E-MTAB-10287.Citation8 In total, 11 samples obtained from five patients were sequenced, including four menstrual cycle stages (proliferative stage, early-secretory stage, mid-secretory stage and late-secretory stage). We selected 10 samples obtained from endometrium for further analysis.
Gene expression profiles and clinical information of human endometrial cancers were obtained from The Cancer Genome Atlas (TCGA) project (https://portal.gdc.cancer.gov/). The expressions of genes were measured by Fragments Per Kilobase of exon model per Million mapped fragments (FPKM). In total, 535 human endometrial cancers were included in our analysis.
Processing of Single Cell Sequencing Data
The 10x Genomics scRNA-seq data were first analyzed using Cellranger 6.1.1 with the raw fastq files as input. The GRCh38 genome was used as the reference genome and default parameters were used. For each sample, the feature-barcode matrix was then converted into a Seurat object using the Seurat R package.Citation13 To enrich for high-quality cells in each sample, we performed quality control (QC) for each sample dataset individually. First, we filtered cells that expressed less than 200 genes. For A13 and A30 patients, we excluded the cells with >10% mitochondrial reads. For E1, E2 and E3, cells with >20% mitochondrial reads were excluded. In addition, we filtered cells with hemoglobin protein-related reads >5%.
Next, we used the “isOutlier” function in scater package to detect the outliers. The outlier cells were defined in each of the following metrics: log(UMI counts) (>2 MADs, both), log(number of genes expressed) (>2 MADs, both) and log(percent mitochondrial read count) (>2 MADs, high end). We used the “CellCycleScoring” in Seurat to calculate the cell cycle score for each cell and regression with the “vars.to.regress”. Cell cycle genes (G2/M and S) of were obtained from Seurat package. To reduce the false positive rate in doublet calling, only cells marked as doublets by both scDblFinder and doubletFinderCitation14 were removed from our analysis.
Data Normalization, Feature Selection and Clustering
The quality control was performed for single sample and the “CCA” function in Seurat was used to integrate all samples. The read count matrices were normalized using “NormalizedData” with “LogNormalize” as the normalization method. Feature selection was performed by “FindVariableFeatures” using the “vst” method and the top 2000 variable genes were identified. The top 2000 most variable genes were summarized by principal component analysis (PCA). To identify groups of distinct cells, graph-based Louvain clustering was performed based on top 20 PCs. The “FindClusters” function with a resolution of 0.2 was used to identify the cell clusters and UMAP plots were generated in R for visualization.
Cell Type Annotation
We performed cell types annotation based on two methods. One was gene signature enrichment and another one was reference-based annotation with the SingleR package.Citation15 First, the cell types were annotated based on signatures from ESTIMATECitation16 and PangladoDB.Citation17 The “AddModuleScore” was used to calculate the signature scores. The median scores of each cell clusters were calculated and if the median >0.1, we considered the clusters as corresponding cell types. The primary annotations were performed by SingleR using the “HumanPrimaryCellAtlasData” as reference dataset. The marker genes of cell types were obtained from literature or CellMarker database.Citation18
Identification of Differentially Expressed Genes and Functional Annotations
Differential gene expression analysis was performed by “FindAllMarkers” in Seurat with the min.pct set to 0.25 and Wilcoxon’s rank sum test. Genes with p.adiust<0.05 and log2FoldChange>0.25 were considered as up-regulated. For functional enrichment analysis, we selected top 50 highly expressed genes in each main cell type. For the cell subtypes, we used all differentially expressed genes. The functional enrichment analysis was performed by clusterProfiler,Citation19 and heat maps were generated by ComplexHeatmap.Citation20
Gene Sets Functional Scores
To calculate the gene set functional scores, we used AUCell to perform this analysis.Citation21 The functional gene sets were obtained from the Molecular Signatures Database (MSigDB) hallmark gene set collection.Citation22 Wilcoxon’s rank sum test was used to compare the functional scores in different cell subtypes. P-values were adjusted by false discovery rate (FDR). The gene sets with log2FoldChange>0 and p.adjust<0.05 were considered as enriched in corresponding cell subtypes.
Co-Expression Analysis of Genes
To investigate the expression correlation among differentially expressed genes in each cell type, we calculated the Spearman correlation coefficient (SCC) among top 50 differentially expressed genes based on the expression across cell types. The SCC matrix was visualized by heat map.
Analysis of Transcription Factor Activities
We used pySCENIC to identify the TF regulators in each cell types identified in human endometrium.Citation21,Citation23 We first downloaded the motifs that allow using RcisTarget (mc9nr) from cisTarget database.Citation24 The input UMI count matrix was normalized (CPM) and log-transformed. Only genes in RcisTarget were included in further analysis. GENIE3 was performed to identify TF-modules in each cell type.Citation25 TF-modules having less than 10 genes were filtered out. The top 1 percentile of the number of detected genes per cell was used to calculate the AUCell enrichment of each TF regulon in each cell. ComplexHeatmap was used to generate the heat map of the activity matrix.
Cell–Cell Interactions
Cell–cell communication analysis was performed using CellChat (Version 1.1.0), based on the known ligand-receptor pairs in CellChatDB.Citation26 Briefly, the normalized genes expression matrix and cell type labels generated by Seurat were subjected as input for CellChat. For the main analyses, the core functions “computeCommunProb”, “computeCommunProbPathway” and “mergeCellChat” were applied using default parameters. The “computeNetSimilarityPairwise” function was used to calculate the similarity between pathways and pathways were clustered into different groups based on functional similarities.
RNA-Based Molecular Subtypes of Human Endometrial Cancers
To identify the molecular subtypes of human endometrial cancer, we performed clustering based on the gene expression. First, the ligand-receptor pairs prioritized in single-cell data analysis were used in the clustering. Nonnegative matrix factorization (NMF) was performed based on R package.Citation27 The parameters “rank=2:6, method=brunet” were used in this analysis. Five molecular subtypes were identified based on the cophenetic curve. The survival analysis was performed by R packages (survival and survminer). Log rank test was used to evaluate the difference of survival rates among subtypes.
Results
A Single Cell Map of Human Endometrium
To analyze the scRNA-seq cells from human endometrium through four menstrual cycle stages, we performed principal-component analysis (PCA) using the top 2000 most variably expressed genes across 59,397 cells. Cells were clustered into transcriptionally distinct clusters with top 20 principal components (PCs). The cells were visualized using UMAP plot and revealed eight clusters that could be annotated to known cell types (). Moreover, we found that cells were clustered together based on cell types but not based on patient identify (). We then used well-known marker genes to define the identity of each cell cluster. For example, epithelial cells expressed KRT8 and PAEP, endothelial cells expressed VWF, fibroblast cells expressed APOD, DCN and COL3A1, perivascular (PV) cells expressed RGS5, smooth muscle cells expressed ACTG2 and MYH11, multi-potent stromal cells (MSC) expressed TOP2A and UBE2C, lymphoid and myeloid cells expressed CD74, NKG7 and GNLY (, and and Figure S1).
Figure 1 Diversity of cell types in human endometrium delineated by single-cell transcriptomic analysis. (A and B) UMAP projection of cells from 10 human endometrium samples. (A) colored by cell types and (B) colored by patients. (C) The cell fractions of different cell types originating from different phases of menstrual cycle. (D) The cell fractions of different cell types originating from different patients. (E) Bubble plots showing the expression levels of genes highly expressed in corresponding cell types.
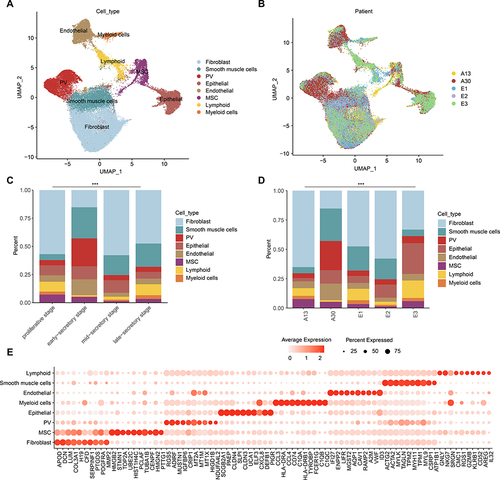
Next, we calculated the proportion of cells during four menstrual cycle stages and different patients. We found that the proportions of cell types among stages and patients were significantly different ( and , p-values < 2.2E-16). PV cells were predominated in early-secretory stage and in A30 patient. Fibroblast cells decreased in early-secretory stage and immune cells were enriched in proliferative stage and late-secretory stage (). MSC decreased during the menstrual cycle stages. Moreover, we identified the highly expressed marker genes in each cell types. We found that C1QA, C1QB and C1QC were highly expressed in myeloid cells (), indicating their phagocytic ability.Citation28 Together, our comprehensive analysis provided a comprehensive catalog of the major cell types together with their cellular position in endometrium.
Systematic Discovery of Cell Type-Specific RNAs in Human Endometrium
We next explored the cell type-specific RNAs that could help explain distinct biological states of these cell types. Functional enrichment analysis revealed that genes highly expressed in fibroblast cells were significantly enriched in wound healing, regulation of vasculature development and regulation of angiogenesis (). Genes highly expressed in smooth muscle cells were enriched in wound healing, extracellular matrix organization and extracellular structure organization, whereas PV cell-specific genes were enriched in response to corticosteroid, steroid hormone and glucocorticoid (). Epithelial cell-specific genes were significantly enriched in epithelial cell proliferation and tissue migration, and endothelial cell-specific genes were enriched in regulation of vasculature development and angiogenesis (). MSCs are a population of self-renewing multipotent cells in the perivascular regions of the endometrium in both the basalis and functionalis.Citation29,Citation30 We found that genes highly expressed in MSCs were enriched in sister chromatid segregation and nuclear division (). A large proportion of MSCs were in G2M stage of cell cycle (). In particular, we found that two proliferative marker genes, MKI67 and TOP2A, were highly expressed in MSC cells ( and ). These results suggested that enriched functional analysis of each cluster supported their functions in human endometrium.
Figure 2 Expression and functions of genes highly expressed in diverse cell types. (A) Heat map showing the expression levels of genes highly expressed in corresponding cell types. Enriched functions were indicated on the left. (B) UMAP plot, color-coded for cell cycle phases. (C and D) UMAP plot, color-coded for relative expression (lowest expression to highest expression, white to red) of marker genes. (C) for MKI67 and (D) for TOP2A.
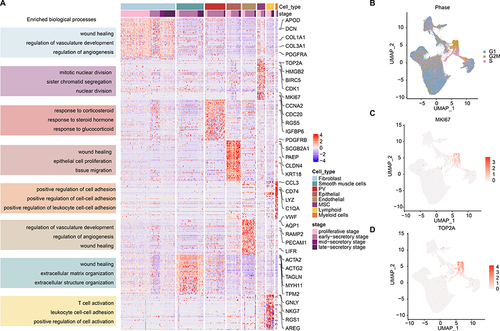
Two Newly Discovered Subtypes of Perivascular Cells
We analyzed the PV population (n=8,120) based on the known markers and re-clustered it into two distinct populations as indicated in the UMAP ( and Figure S2A). We analyzed the relative proportion of cells in each cluster and noted that two clusters exhibited similar proportion during menstrual cycle stages and patients (, p=1.862E-8 and Figure S2B). These observations were consistent with the results PV-MYH11+ are characteristic of myometrium, while PV-STEAP4+ are only present in the endometrium.Citation8 We next analyzed the gene expression profiles and identified the top differentially expressed genes in two PV populations (). In particular, STEAP4 and MYH11 were separately expressed in two PV populations ( and ). We found that several collagen-related genes (eg COL3A1, COL1A2, COL4A1 and COL1A1) were highly expressed in PV-STEAP4 populations (), which might be correlated with their roles in repair of endometrial damage and induced angiogenesis.Citation31 Moreover, IGFBP5 was highly expressed in PV-STEAP4+ subtypes, which is consistent with its roles in promoting angiogenic and neurogenic differentiation.Citation32,Citation33 In contrast, the PV-MYH11+ populations were characteristic of myometrium and highly expressed MUSTN1 and MYH11.
Figure 3 PV cell clusters in human endometrium. (A) UMAP plot of PV cells color-coded according to the assigned cell subtypes. (B) The cell fractions of different PV cell types originating from different phases of menstrual cycle. (C) Bubble plots showing the expression levels of genes highly expressed in corresponding PV subtypes. (D and E) UMAP plot, color-coded for relative expression (lowest expression to highest expression, white to red) of marker genes. (D) for STEAP4 and (E) for MYH11. (F) Heat map showing the expression levels of genes highly expressed in corresponding PV cell subtypes. Enriched functions were indicated on the left. (G) Lollipop plot showing the differences of pathway activities between two PV subtypes.
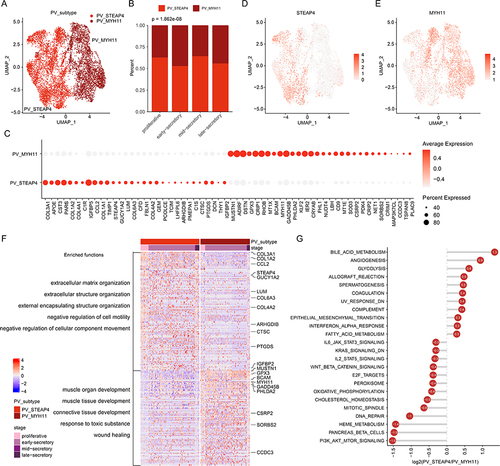
To further determine the specific roles of two PV populations that might contribute to human endometrium, we performed functional enrichment analysis based on the differentially expressed genes. We found that genes highly expressed in two PV populations were both significantly enriched in wound healing, response to oxidative stress and extracellular matrix organization (Figure S2C). However, the proportions of genes in PV-STEAP4+ were much higher. In particular, genes highly expressed in PV-STEAP4+ were significantly enriched in extracellular matrix organization and structure organization, while genes in PV-MYH11+ were significantly enriched in muscle development-related functions (). Cancer hallmark-related pathways also exhibited distinct activities in two PV populations. PV-STEAP4+ cells exhibited higher activities in immune and metabolism-related functions and PV-MYH11+ cells exhibited higher activities in signaling and proliferative pathways, such as PI3K-AKT-mTOR (). It has been demonstrated that glycolysis is beneficial to angiogenesis and plays an important role in endometrial decidualization.Citation34–36 In addition, PI3K-AKT-mTOR pathway plays an important role in the decidualization of endometrium.
Subpopulations of Epithelial Cells Across Human Menstrual Cycle
The epithelial cell cluster (n=5,758) was then assigned to four epithelial subtypes based on known markers obtained from the published literatures.Citation37,Citation38 Three secretory glandular cells (secretory LGALS1+/PAEP+/MT+) and ciliated cells were identified (). We calculated the proportion of four subpopulations across menstrual cycle and found that ciliated epithelial cells were enriched in proliferative phase, secretory LGALS1+ cells were enriched in early and mid-secretory phases (, p<2.2E-16). By examining the gene expression patterns of four epithelial subpopulations, we revealed that ciliated cells expressed high levels of markers, such as the ciliated marker TPPP3, PIFO and FAM183A ( and ).
Figure 4 Epithelial cell clusters in human endometrium. (A) UMAP plot of epithelial cells color-coded according to the assigned cell subtypes. (B) The cell fractions of different epithelial cell types originating from different phases of menstrual cycle. (C) Bubble plots showing the expression levels of genes highly expressed in corresponding epithelial subtypes. (D) UMAP plot, color-coded for relative expression (lowest expression to highest expression, white to red) of marker genes. (E) Heat map showing the expression levels of genes highly expressed in corresponding epithelial cell subtypes. Enriched functions were indicated on the left. (F) Heat map showing the differences of pathway activities among epithelial subtypes.
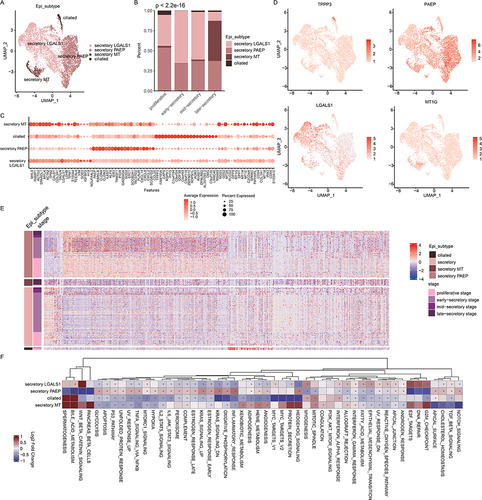
The gene set enrichment analyses showed that genes up-regulated in ciliated cells were mainly enriched for cilium organization, cilium assembly, cilium movement functions ( and Figure S3). This result is similar with one previous study.Citation9 The secretory MT+ cell populations highly expressed genes in metallothionein (MT) family, such as MT2A, MT1G, MT1E, MT1X and MT1H (), which might contribute to impaired endometrial receptivity.Citation39 Moreover, higher proportion of secretory MT+ cells was observed in late-secretory phase (). This is consistent with previous observations that MT genes were highly expressed in late-secretory phase.Citation40 The secretory PAEP+ cell populations were enriched during the menstrual cycle and highly expressed CLDN3, CLDN4 and CXCL family genes (). Functional analysis revealed that genes up-regulated in this cell population were enriched in regulation of cell–cell adhesion, cell migration and reproductive development (Figure S3).
We next performed functional analysis based on cancer hallmark-related pathways. By examining the pathway activities, we found that secretory populations exhibited higher pathway activities in numerous pathways (). The ciliate epithelial cells exhibited higher pathway activities in bile acid metabolism and mitotic spindle. The Wnt signaling pathway, DNA repair and G2M checkpoint pathways were enriched in secretory LGALS1+ cell populations (). In particular, epithelial mesenchymal transition was enriched in secretory LGALS1+, which was consistent with their roles in regeneration or differentiation of endometrium.Citation29 The inflammation-related pathways were significantly enriched in secretory PAEP+ cell populations, such as IL6-JAK-STAT3 and IL2-STAT5 signaling pathways (). These results extended the transcriptional signature and potential pathways underlying the human endometrial epithelial cells.
Fibroblast Cells Separate into Four Distinct Cell Types
Fibroblasts (n=21,865) were further separated into four clusters and annotated based on the highest expressing genes (). SPARACL1, ID4, MMP11 and EGR1 were highly expressed in corresponding clusters. We found that fibroblast ID4+ and SPARCL1+ cells were primarily observed in early- and late-secretory phases (). Fibroblast MMP11+ cells were primarily in the proliferative phase and EGR1+ cells were in mid-secretory phase (, p<2.2E-16). The proportions were variable in different patients (Figure S4A), suggesting the high heterogeneity among patients. Gene expression analysis revealed fibroblast SPARCL1+ highly expressed fibroblast-related genes, such as DCN, APOD, COL1A2 and COL15A1 ( and Figure S4B). In particular, four clusters highly expressed corresponding marker genes (). We next performed the functional enrichment analysis and found that genes up-regulated in fibroblast SPARCL1+ were significantly enriched in extracellular structure organization and negative regulation of locomotion (). Genes highly expressed in fibroblast ID4+ were enriched in epithelial cell proliferation, positive regulation of cytokine production and regulation of angiogenesis (). Numerous of transcription factors (TFs) were highly expressed in fibroblast EGR1+ sub-populations, such as JUNB, EGR1, FOS and JUN. We also observed high expression of GADD45B in this population, which plays important roles in DNA repair.Citation41 We also investigated the cancer hallmark pathway activities in different fibroblast populations and found that Wnt, MYC and peroxisome exhibited higher activities in ID4+ cell populations (Figure S4C). Notch and PI3K-AKT signaling pathways were active in SPARCL1+ cell populations (Figure S4C). Together, these results uncovered the potential pathways underlying different fibroblast populations in human endometrium.
Figure 5 Fibroblast cell clusters in human endometrium. (A) UMAP plot of fibroblast cells color-coded according to the assigned cell subtypes. (B) The cell fractions of different fibroblast cell types originating from different phases of menstrual cycle. (C) Bubble plots showing the expression levels of genes highly expressed in corresponding fibroblast subtypes. (D) UMAP plot, color-coded for relative expression (lowest expression to highest expression, white to red) of marker genes. (E) Heat map showing the differences of pathway activities among fibroblast subtypes.
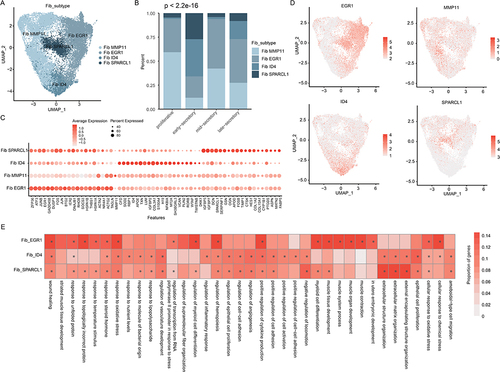
TF Regulators of Cell Types in Human Endometrium
It has been demonstrated that genes usually interact with each other to form a complex interaction network.Citation42 We thus analyzed the correlation between gene expressions and we found that genes highly expressed in cell types were co-expressed with each other (), indicating the modular programs associated with basic cellular functions of cells. TFs are important regulators and play important roles in regulating gene expression.Citation43 In addition, we calculated the module activities as the average expression of genes. We found that the expressions of several TFs (such as IRF8, SOX17, FOS and KLF2) were significantly correlated with the module activities (Figure S5). We thus identified the cell type-specific TFs based on the pySCENIC pipeline. In total, we identified 336 TFs exhibited high activities in different cell types and 62 TFs also exhibited differential expression (). We found that TFs exhibited high cell type- and phase-specificity. For example, SOX4 exhibited higher activity in proliferative phase (). SOX4 is closely associated with the development and progression of many malignant tumors and plays an important role in the cell growth and proliferation.Citation44 We also observed that the expressions of SOX4 in proliferative phase were significantly higher than secretory phases. In addition, MAF, KLF4, JUN, FOS and EGR1 exhibited higher activities in secretory phases ().
Figure 6 TF regulators of cell types. (A) Co-expression of genes differentially expressed in cell type. (B) Heat maps showing the TF activities in each cell types. Each row represents a TF and column represents a cell. Numbers in the brackets were the potential target genes of TFs. (C) Violin plots showing the expression of TFs during menstrual cycle. (D) Bar plots showing the enriched functions of TF target genes.
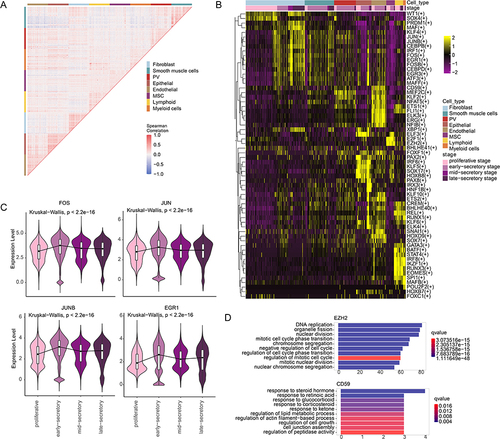
Moreover, we found that several TFs exhibited cell type-specific activities in human endometrium. CD59 exhibited higher activities in endothelial cells, which is highly expressed in endothelial cells of human endometrium (Figure S6). It has been demonstrated that CD59 may be important in protection of endothelial cells against C-mediated damage at local sites of inflammation, thereby maintaining the vascular integrity.Citation45 E2F1 and EZH2 exhibited higher activities in MSC cell populations (). E2F1 plays a pivotal role in driving cells out of a quiescent state and into the S phase of the cell cycleCitation46 and the activity of EZH2 influences cell fate regulation.Citation47 Functional analysis of the targets of EZH2 revealed that they were significantly enriched in DNA replication and cell cycle, which is consistent with the proliferative state of MSC cells. EGR1 exhibited higher activities in fibroblast cells (), which had been demonstrated to contribute to inflammatory factors and fibrosis reduction.Citation48 The potential targets of EGR1 were significantly enriched in Wnt signaling pathway and cell growth (). In addition, FOS exhibited higher activities in fibroblast and smooth muscle cell populations () and its targets were significantly enriched in muscle tissue development, Wnt signaling and cell differentiation (). It has been demonstrated that FOS plays an important role in control of fibroblast senescence and activation of programmed cell death.Citation49 Several TFs also exhibited higher activities in immune cells, such as STAT4, BATF, RUNX3 and EOMES (). These results suggest that the dynamic transcriptome during menstrual cycle was strictly regulated by TFs that were with dynamic activities.
Cell–Cell Interactions in Human Endometrium
The development of tissue and progression of complex diseases relies on a complex network of cell–cell interactions.Citation50 We thus inferred intercellular communications for human endometrium based on CellChat.Citation26 As a result, we found that the myeloid cells frequently interact with MSC and epithelial cells, while the fibroblast cells frequently interact with others (). Immune cells mainly function as signal input cells. As the signal output cells, epithelial and endothelial cells mainly interact with immune cells as targets (). Next, the 15 signaling pathways associated with inferred networks were mapped onto a two-dimensional manifold and clustered into four groups ( and ). MIF, IGF, PTN and MK pathways were grouped into cluster 1, while group 4 was formed by pathways of VEGF, CXCL and VISFATIN ().
Figure 7 Cell–cell communications in human endometrium. (A) Cell–cell communications. (B) Jointly projecting and clustering signaling pathways into a shared two-dimension manifold according to their functional similarity. The size is proportional to the total communication probability. (C) Magnified view of each pathway group. (D) The inferred MIF signaling pathway network in human endometrium. Left and right portions show the autocrine and paracrine signaling, respectively. Circle sizes are proportional to the number of cells in each cell group and edge width represents the communication probability. (E) Relative contribution of each ligand-receptor pair to the overall MIF signaling pathway network. (F) Expression distribution of MIF signaling genes. (G) The inferred SPP1 signaling pathway network in human endometrium. Left and right portions show the autocrine and paracrine signaling, respectively. Circle sizes are proportional to the number of cells in each cell group and edge width represents the communication probability. (H) Relative contribution of each ligand-receptor pair to the overall SPP1 signaling pathway network. (I) Expression distribution of SPP1 signaling genes. (J and K) The dot plot showing the comparison of incoming and outgoing signaling patterns of secreting cells. The dot size is proportional to the contribution score computed from pattern recognition analysis. Higher contribution score implies the signaling pathway is more enriched in the corresponding cell group.
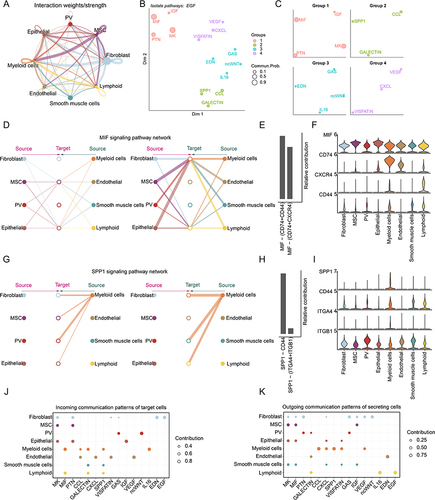
We specifically examined how macrophage migration inhibitory factor (MIF) communications among cell populations (). All cells function as signal output cells, although the intensity of interaction was different. When MSC and epithelial cells acted as signal input cells, the output intensity of cells was low. In this pathway, immune cells were mainly used as signal input cells, and the strength of intercellular interaction was high (). Macrophage migration inhibitory factor had been identified as a potential biomarker of endometriosis.Citation51,Citation52 We also examined ligand receptor pairs that play a major role in this pathway and identified two ligand-receptor pairs MIF−(CD74+CD44), and MIF−(CD74+CXCR4) (). MIF exhibited high expression in all cell types, while CXCR4, CD74 and CD44 exhibited higher expression in immune cells (). We also found that the SPP1 signaling pathway only took myeloid cells as signal output cells, and exported signals to fibroblasts, PV cells, SMC cells and lymphocytes in paracrine mode, and played a role in autocrine mode to a large extent (). The SPP1-(ITGA4+ITGB1) and SPP1-CD44 plays important roles in cell–cell communications (). SPP1 and CD44 exhibited higher expression in immune cells, while ITGB1 was highly expressed in all cell types ().
Next, we compared the information flow for each signaling pathway. In signal input, several pathways only functions in one cell type, such as EGF and EDN in fibroblasts, VEGF and CXCL in endothelial cells, and ncWNT in PV cells (). MIF signaling pathway only participates in the signal input of immune cells and most of these pathways are involved in signal input patterns of myeloid cells, fibroblasts, and endothelial cells (). In the signal output part, we found that IL6, EGF, SPP1 and CCL only played a role in the signal output of immune cells. EDN only plays a role in signal output of endothelial cells. As with signal input patterns, most of these pathways were involved in signal output by fibroblasts and myeloid cells (). Together, cell–cell communication analysis enables multifaceted assessment of intercellular communication patterns in human endometrium.
RNA-Based Molecular Subtypes of Human Endometrial Cancers
Endometrial cancer (EC) is the most common gynecologic malignancy. We next explored the expression patterns of the ligand-receptor in EC. We found that the patients can be grouped into five clusters based on the ligand-receptor of the 15 pathways identified in cell–cell communication (). The survival rates for patients in five clusters were significantly different, and patients in cluster-5 were with poor survival (, Log rank test p=0.00025). We found that patients in cluster-5 were with distinct expression of 17 genes, which were involved multiple cytokine-related pathways, including MIF, CXCL, SPP1 and VEGF. We next calculated the pathway activities for these pathways and found that the majority of pathway activities in cluster-5 were significantly higher than other clusters. For example, epidermal growth factor (EGF) was a conventional mitogenic factor that can stimulate the proliferation of various types of cells including epithelial cells and fibroblasts.Citation53 The activities of EGF in cluster-5 were significantly higher than that in cluster 1, 3, and 4 (). The EGFR family also plays an important role in maintaining epithelial homeostasisCitation54 and overexpressed in EC and ovarian cancers.Citation55,Citation56 Compared with other clusters, we found that ERBB2 was highly expressed in patients of cluster-5 ().
Figure 8 Molecular subtypes of EC. (A) Heat map showing the consensus matrix of EC patients. (B) The overall survival curves based on EC patients data, stratified by the expression patterns of ligand-receptor pairs. (C) Boxplots showing the EGF pathway activities in patients of different EC clusters. (D) Boxplots showing the expressions of ERBB2 in patients of different EC clusters. (E) Boxplots showing the IGF pathway activities in patients of different EC clusters. (F) Boxplots showing the expressions of IGF2 in patients of different EC clusters.
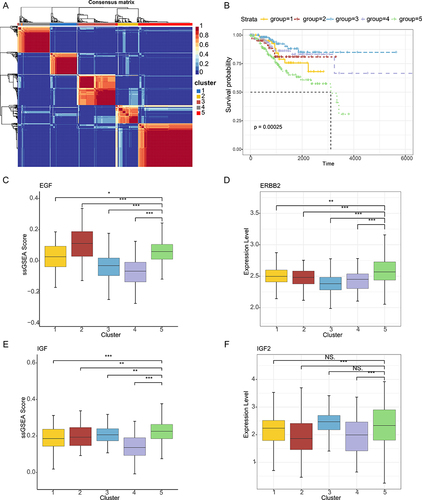
In addition, as a major ligand of IGF, IGF2 overexpression has been shown to play a role in many cancers.Citation57 However, there seem to be few reports about its role in endometrial cancer. We found that the activities of IGF pathway and expressions of IGF2 in patients of cluster-5 were significantly higher than cluster-2 and cluster-4 ( and ). In contrast, several pathways (ie CXCL) had lower pathway activities in cluster-5 and the expressions of CXCR4 were also lower in patients of cluster-5. These results suggested that the genes identified here can be used to predict the prognostic survival of patients with endometrial cancer.
Discussion
In this study, our single transcriptome analysis yields a comprehensive catalog of the major subsets of uterine cells in endometrium. We comprehensively identified the diverse cell types in human endometrium and found that there were a higher proportion of fibroblast, PV and epithelial cells. We further characterized the gene expression patterns in different cell types and identified the cell-type specific genes. In particular, we explored the transcriptional regulation and revealed the TFs that exhibited high activities in specific cell types. In addition, cell–cell communications were examined and revealed the critical ligand-receptor pairs. The expression patterns of identified ligand-receptor further uncovered five molecular subtypes of EC. All these results increased our understanding of the dynamic microenvironment of uterine cells in endometrium, as well as potential biomarkers for EC.
In particular, we identified two perivascular cell subtypes, four epithelial subtypes and four fibroblast cell types in endometrium. We showed that the ciliated epithelium is a typical endometrial cell type, which is consistent with the results of one recent study.Citation58 Although the existence of ciliated cells in human endometrium was observed long times ago,Citation59 the gene expression patterns and marker genes were not identified previously. We identified numerous of genes that showed higher expression in ciliated cells, such as TPPP3, CAPS, AGR3 and PIFO. AGR3 is a specialized member of the protein disulfide isomerase (PDI) family, and it has been found to play an unexpected role in the regulation of ciliary beat frequency and mucociliary clearance in the airway.Citation60
In total, we identified 4,038 immune cells, including monocyte, NK1, NK2 and T cells (Figure S7A). Immune cells were mainly enriched in proliferation and late secretory stage, NK2 cells were mainly enriched in late secretory stage, and T cells were mainly distributed in proliferative stage (Figure S7B). Monocyte cells highly expressed CD74 and LYZ, T cells highly expressed CD3D and IL7R, and NK cells highly expressed NKG7 and GNLY (Figure S7C). In addition, the high expression of antigen presentation and phagocytic genes were observed in monocyte, such as HLA−DRA, HLA−DRB1, HLA−DPA1, C1QA, C1QB, and C1QC, indicating that they play an important role in the clearance of apoptotic cells after endometrial shedding (Figure S7D). Functional enrichment analysis suggested that genes highly expressed in monocyte were significantly enriched in antigen processing and presentation, cell–cell adhesion and leukocyte migration (Figure S7E). Moreover, genes highly expressed in T cells were enriched in T cell differentiation, T cell activation and leukocyte cell−cell adhesion (Figure S7E). These results suggest that immune cells play an important role in the immune response, clearance of apoptotic cells and endometrial tissue remodeling after endometrium shedding.
In addition, we found that genes highly expressed in cell types showed expression perturbations in cancer. For example, APOD, DCN, LUM and CFD were significantly highly expressed in fibroblast cells (Figure S8A). DCN has been demonstrated to play tumor suppressive functions in cancer,Citation61,Citation62 and we found that it was lowly expressed in EC. CAV1 and VWF were highly expressed in endothelial cells but showed lower expression in EC (Figure S8B). Epithelial-related genes, such as SCGB2A1 and CLDN4, were highly expressed in cancer (Figure S8C). Moreover, we found that CCL3, C1QA, GZMB and IL32 were highly expressed in cancer (Figure S8D and E). SCGB2A1 was found to be perturbed in various cancer types, such as breast and ovarian cancers.Citation63,Citation64 We also found the expressions of several genes were associated with survival of cancer patients (Figure S8F). These results highlight the critical roles of these genes in endometrial cancer.
In summary, deconvolution of immune microenvironment that drives transcriptional programs throughout the menstrual cycle is important for understanding RNA regulatory biology of endometrium. Our dynamic immune microenvironment analyses provide novel insights into future development of RNA-based treatments for endometriosis and endometrial carcinoma. Our findings provide a theoretical foundation for personalized medicine research on endometrial diseases. However, further experimental validation is needed, including studies using mouse models or cell lines, to minimize the impact on human patients, especially those who wish to preserve their fertility. This emphasizes the importance of tailored approaches in improving treatment efficacy and patient outcomes, while also effectively reducing the occurrence of potential medical incidents.
Ethics Approval and Consent to Participate
All relevant ethical regulations were followed the original study of the datasets and the authors of the source studies had also obtained informed consent from participants. Ethic approval was exempted for this study by Hainan Medical University because the data were obtained from public resource.
Author Contributions
All authors made a significant contribution to the work reported, whether that is in the conception, study design, execution, acquisition of data, analysis and interpretation, or in all these areas; took part in drafting, revising or critically reviewing the article; gave final approval of the version to be published; have agreed on the journal to which the article has been submitted; and agree to be accountable for all aspects of the work.
Disclosure
The authors declare that they have no competing interests in this work.
Acknowledgments
This paper has been uploaded to ResearchSquare as a preprint: https://www.researchsquare.com/article/rs-2645136/v1.
Data Sharing Statement
All datasets used in our study are from previously published studies. All the data generated in this study can be downloaded from https://figshare.com/projects/scRNAseq-Endometrium/147556. All the scripts can be downloaded from the following link: https://figshare.com/projects/scRNAseq-Endometrium/147556.
Additional information
Funding
References
- Garrido-Gomez T, Dominguez F, Quinonero A, et al. Defective decidualization during and after severe preeclampsia reveals a possible maternal contribution to the etiology. Proc Natl Acad Sci U S A. 2017;114(40):E8468–E8477. doi:10.1073/pnas.1706546114
- Salker MS, Christian M, Steel JH, et al. Deregulation of the serum- and glucocorticoid-inducible kinase SGK1 in the endometrium causes reproductive failure. Nat Med. 2011;17(11):1509–1513. doi:10.1038/nm.2498
- Piergentili R, Zaami S, Cavaliere AF, et al. Non-coding RNAs as prognostic markers for endometrial cancer. Int J Mol Sci. 2021;23(1):22. doi:10.3390/ijms23010022
- Cavaliere AF, Perelli F, Zaami S, et al. Towards Personalized medicine: non-coding RNAs and endometrial cancer. Healthcare. 2021;10(1):9. doi:10.3390/healthcare10010009
- Wang F, Jia W, Fan M, et al. Single-cell immune landscape of human recurrent miscarriage. Genomics Proteomics Bioinf. 2021;19(2):208–222. doi:10.1016/j.gpb.2020.11.002
- Liu Z, Sun Z, Liu H, et al. Single-cell transcriptomic analysis of eutopic endometrium and ectopic lesions of adenomyosis. Cell Biosci. 2021;11(1):51. doi:10.1186/s13578-021-00562-z
- Ma J, Zhang L, Zhan H, et al. Single-cell transcriptomic analysis of endometriosis provides insights into fibroblast fates and immune cell heterogeneity. Cell Biosci. 2021;11(1):125. doi:10.1186/s13578-021-00637-x
- Garcia-Alonso L, Handfield LF, Roberts K, et al. Mapping the temporal and spatial dynamics of the human endometrium in vivo and in vitro. Nat Genet. 2021;53(12):1698–1711. doi:10.1038/s41588-021-00972-2
- Guo YE, Li Y, Cai B, et al. Phenotyping of immune and endometrial epithelial cells in endometrial carcinomas revealed by single-cell RNA sequencing. Aging. 2021;13(5):6565–6591. doi:10.18632/aging.202288
- Bell DW, Ellenson LH. Molecular genetics of endometrial carcinoma. Annu Rev Pathol. 2019;14(1):339–367. doi:10.1146/annurev-pathol-020117-043609
- Murali R, Soslow RA, Weigelt B. Classification of endometrial carcinoma: more than two types. Lancet Oncol. 2014;15(7):e268–278. doi:10.1016/S1470-2045(13)70591-6
- Kandoth C, Schultz N, Cherniack AD, et al.; Cancer Genome Atlas Research N. Integrated genomic characterization of endometrial carcinoma. Nature. 2013;497(7447):67–73. doi:10.1038/nature12113
- Stuart T, Butler A, Hoffman P, et al. Comprehensive Integration of Single-Cell Data. Cell. 2019;177(7):1888–1902e1821. doi:10.1016/j.cell.2019.05.031
- McGinnis CS, Murrow LM, Gartner ZJ. DoubletFinder: doublet detection in single-cell RNA sequencing data using artificial nearest neighbors. Cell Syst. 2019;8(4):329–337e324. doi:10.1016/j.cels.2019.03.003
- Aran D, Looney AP, Liu L, et al. Reference-based analysis of lung single-cell sequencing reveals a transitional profibrotic macrophage. Nat Immunol. 2019;20(2):163–172. doi:10.1038/s41590-018-0276-y
- Yoshihara K, Shahmoradgoli M, Martinez E, et al. Inferring tumour purity and stromal and immune cell admixture from expression data. Nat Commun. 2013;4(1):2612. doi:10.1038/ncomms3612
- Franzen O, Gan LM, Bjorkegren JLM. PanglaoDB: a web server for exploration of mouse and human single-cell RNA sequencing data. Database. 2019;2019:2. doi:10.1093/database/baz046
- Zhang X, Lan Y, Xu J, et al. CellMarker: a manually curated resource of cell markers in human and mouse. Nucleic Acids Res. 2019;47(D1):D721–D728. doi:10.1093/nar/gky900
- Yu G, Wang LG, Han Y, He QY. clusterProfiler: an R package for comparing biological themes among gene clusters. OMICS. 2012;16(5):284–287. doi:10.1089/omi.2011.0118
- Gu Z, Hubschmann D, Pei J, Houwing-Duistermaat J, Uh H-W. Make Interactive Complex Heatmaps in R. Bioinformatics. 2021;22(1). doi:10.1186/s12859-021-03958-3
- Van de Sande B, Flerin C, Davie K, et al. A scalable SCENIC workflow for single-cell gene regulatory network analysis. Nat Protoc. 2020;15(7):2247–2276. doi:10.1038/s41596-020-0336-2
- Liberzon A, Birger C, Thorvaldsdottir H, Ghandi M, Mesirov JP, Tamayo P. The Molecular Signatures Database (MSigDB) hallmark gene set collection. Cell Syst. 2015;1(6):417–425. doi:10.1016/j.cels.2015.12.004
- Aibar S, Gonzalez-Blas CB, Moerman T, et al. SCENIC: single-cell regulatory network inference and clustering. Nat Methods. 2017;14(11):1083–1086. doi:10.1038/nmeth.4463
- Imrichova H, Hulselmans G, Atak ZK, Potier D, Aerts S. i-cisTarget 2015 update: generalized cis-regulatory enrichment analysis in human, mouse and fly. Nucleic Acids Res. 2015;43(W1):W57–64. doi:10.1093/nar/gkv395
- Huynh-Thu VA, Irrthum A, Wehenkel L, Geurts P. Inferring regulatory networks from expression data using tree-based methods. PLoS One. 2010;2:5.
- Jin S, Guerrero-Juarez CF, Zhang L, et al. Inference and analysis of cell-cell communication using CellChat. Nat Commun. 2021;12(1):1088. doi:10.1038/s41467-021-21246-9
- Gaujoux R, Seoighe C. A flexible R package for nonnegative matrix factorization. BMC Bioinf. 2010;11(1):367. doi:10.1186/1471-2105-11-367
- Zou G, Wang J, Xu X, et al. Cell subtypes and immune dysfunction in peritoneal fluid of endometriosis revealed by single-cell RNA-sequencing. Cell Biosci. 2021;11:98.
- Owusu-Akyaw A, Krishnamoorthy K, Goldsmith LT, Morelli SS. The role of mesenchymal-epithelial transition in endometrial function. Hum Reprod Update. 2019;25(1):114–133. doi:10.1093/humupd/dmy035
- Schwab KE, Gargett CE. Co-expression of two perivascular cell markers isolates mesenchymal stem-like cells from human endometrium. Hum Reprod. 2007;22(11):2903–2911. doi:10.1093/humrep/dem265
- Li Z, Yan G, Diao Q, et al. Transplantation of human endometrial perivascular cells with elevated CYR61 expression induces angiogenesis and promotes repair of a full-thickness uterine injury in rat. Stem Cell Res Ther. 2019;10(1):179. doi:10.1186/s13287-019-1272-3
- Li J, Diao S, Yang H, Cao Y, Du J, Yang D. IGFBP5 promotes angiogenic and neurogenic differentiation potential of dental pulp stem cells. Dev Growth Differ. 2019;61(9):457–465. doi:10.1111/dgd.12632
- Lofqvist C, Willett KL, Aspegren O, et al. Quantification and localization of the IGF/insulin system expression in retinal blood vessels and neurons during oxygen-induced retinopathy in mice. Invest Ophthalmol Vis Sci. 2009;50(4):1831–1837. doi:10.1167/iovs.08-2903
- He X, Zeng H, Chen ST, et al. Endothelial specific SIRT3 deletion impairs glycolysis and angiogenesis and causes diastolic dysfunction. J Mol Cell Cardiol. 2017;112:104–113. doi:10.1016/j.yjmcc.2017.09.007
- Deng F, Zhou R, Lin C, et al. Tumor-secreted dickkopf2 accelerates aerobic glycolysis and promotes angiogenesis in colorectal cancer. Theranostics. 2019;9(4):1001–1014. doi:10.7150/thno.30056
- Lv H, Tong J, Yang J, et al. Dysregulated pseudogene HK2P1 may contribute to preeclampsia as a competing endogenous RNA for hexokinase 2 by impairing decidualization. Hypertension. 2018;71:648–658. doi:10.1161/HYPERTENSIONAHA.117.10084
- Haider S, Gamperl M, Burkard TR, et al. Estrogen signaling drives ciliogenesis in human endometrial organoids. Endocrinology. 2019;160(10):2282–2297. doi:10.1210/en.2019-00314
- Fitzgerald HC, Dhakal P, Behura SK, Schust DJ, Spencer TE. Self-renewing endometrial epithelial organoids of the human uterus. Proc Natl Acad Sci U S A. 2019;116(46):23132–23142. doi:10.1073/pnas.1915389116
- Zhai J, Li S, Hu J, et al. In silico, in vitro, and in vivo analysis identifies endometrial circadian clock genes in recurrent implantation failure. J Clin Endocrinol Metab. 2021;106(7):2077–2091. doi:10.1210/clinem/dgab119
- Hu S, Yao G, Wang Y, et al. Transcriptomic changes during the pre-receptive to receptive transition in human endometrium detected by RNA-Seq. J Clin Endocrinol Metab. 2014;99(12):E2744–2753. doi:10.1210/jc.2014-2155
- Moskalev AA, Smit-McBride Z, Shaposhnikov MV, et al. Gadd45 proteins: relevance to aging, longevity and age-related pathologies. Ageing Res Rev. 2012;11(1):51–66. doi:10.1016/j.arr.2011.09.003
- Barabasi AL, Gulbahce N, Loscalzo J. Network medicine: a network-based approach to human disease. Nat Rev Genet. 2011;12(1):56–68. doi:10.1038/nrg2918
- Ma CZ, Brent MR, Pier Luigi M. Inferring TF activities and activity regulators from gene expression data with constraints from TF perturbation data. Bioinformatics. 2021;37(9):1234–1245. doi:10.1093/bioinformatics/btaa947
- Zhang J, Xiao C, Feng Z, et al. SOX4 promotes the growth and metastasis of breast cancer. Cancer Cell Int. 2020;20(1):468. doi:10.1186/s12935-020-01568-2
- Brooimans RA, Van der Ark AA, Tomita M, Daha MR, Daha MR. CD59 expressed by human endothelial cells functions as a protective molecule against complement-mediated lysis. Eur J Immunol. 1992;22(3):791–797. doi:10.1002/eji.1830220324
- Stubbs MC, Hall DJ. The amino-terminus of the E2F-1 transcription factor inhibits DNA replication of autonomously replicating plasmids in mammalian cells. Oncogene. 2002;21(23):3715–3726. doi:10.1038/sj.onc.1205473
- Lund K, Adams PD, Copland M. EZH2 in normal and malignant hematopoiesis. Leukemia. 2014;28(1):44–49. doi:10.1038/leu.2013.288
- Li J, Gong L, Zhang R, et al. Fibroblast growth factor 21 inhibited inflammation and fibrosis after myocardial infarction via EGR1. Eur J Pharmacol. 2021;910:174470. doi:10.1016/j.ejphar.2021.174470
- Wang E, Lee MJ, Pandey S. Control of fibroblast senescence and activation of programmed cell death. J Cell Biochem. 1994;54(4):432–439. doi:10.1002/jcb.240540410
- Calvo F, Sahai E. Cell communication networks in cancer invasion. Curr Opin Cell Biol. 2011;23(5):621–629. doi:10.1016/j.ceb.2011.04.010
- Mahdian S, Aflatoonian R, Yazdi RS, et al. Macrophage migration inhibitory factor as a potential biomarker of endometriosis. Fertil Steril. 2015;103(1):153–159e153. doi:10.1016/j.fertnstert.2014.09.031
- Kats R, Al-Akoum M, Guay S, Metz C, Akoum A. Cycle-dependent expression of macrophage migration inhibitory factor in the human endometrium. Hum Reprod. 2005;20(12):3518–3525. doi:10.1093/humrep/dei234
- Yamada M, Ikeuchi T, Hatanaka H. The neurotrophic action and signalling of epidermal growth factor. Prog Neurobiol. 1997;51(1):19–37. doi:10.1016/S0301-0082(96)00046-9
- Inoue H, Hattori T, Zhou X, et al. Dysfunctional ErbB2, an EGF receptor family member, hinders repair of airway epithelial cells from asthmatic patients. J Allergy Clin Immunol. 2019;143(6):2075–2085e2010. doi:10.1016/j.jaci.2018.11.046
- Yoo JY, Kim TH, Shin JH, et al. Loss of MIG-6 results in endometrial progesterone resistance via ERBB2. Nat Commun. 2022;13(1):1101. doi:10.1038/s41467-022-28608-x
- Yan M, Parker BA, Schwab R, Kurzrock R. HER2 aberrations in cancer: implications for therapy. Cancer Treat Rev. 2014;40(6):770–780. doi:10.1016/j.ctrv.2014.02.008
- Xie M, Sun M, Ji X, et al. Overexpression of BACH1 mediated by IGF2 facilitates hepatocellular carcinoma growth and metastasis via IGF1R and PTK2. Theranostics. 2022;12(3):1097–1116. doi:10.7150/thno.65775
- Wang W, Vilella F, Alama P, et al. Single-cell transcriptomic atlas of the human endometrium during the menstrual cycle. Nat Med. 2020;26(10):1644–1653. doi:10.1038/s41591-020-1040-z
- Turco MY, Gardner L, Hughes J, et al. Long-term, hormone-responsive organoid cultures of human endometrium in a chemically defined medium. Nat Cell Biol. 2017;19(5):568–577. doi:10.1038/ncb3516
- Bonser LR, Schroeder BW, Ostrin LA, et al. The endoplasmic reticulum resident protein AGR3. Required for regulation of ciliary beat frequency in the airway. Am J Respir Cell Mol Biol. 2015;53(4):536–543. doi:10.1165/rcmb.2014-0318OC
- Lala PK, Nandi P. Mechanisms of trophoblast migration, endometrial angiogenesis in preeclampsia: the role of decorin. Cell Adh Migr. 2016;10(1–2):111–125. doi:10.1080/19336918.2015.1106669
- Goldoni S, Seidler DG, Heath J, et al. An antimetastatic role for decorin in breast cancer. Am J Pathol. 2008;173(3):844–855. doi:10.2353/ajpath.2008.080275
- Kim M, Park J, Bouhaddou M, et al. A protein interaction landscape of breast cancer. Science. 2021;374(6563):eabf3066. doi:10.1126/science.abf3066
- Zhou H, Zou X, Li H, Li T, Chen L, Cheng X. Decreased secretoglobin family 2A member 1expression is associated with poor outcomes in endometrial cancer. Oncol Lett. 2020;20(4):24. doi:10.3892/ol.2020.11885