Abstract
Introduction
Sepsis is currently a common condition in emergency and intensive care units, and is defined as life-threatening organ dysfunction caused by a dysregulated host response to infection. Cardiac dysfunction caused by septic myocardial injury (SMI) is associated with adverse prognosis and has significant economic and human costs. The pathophysiological mechanisms underlying SMI have long been a subject of interest. Recent studies have identified ferroptosis, a form of programmed cell death associated with iron accumulation and lipid peroxidation, as a pathological factor in the development of SMI. However, the current understanding of how ferroptosis functions and regulates in SMI remains limited, particularly in the absence of direct evidence from human heart.
Methods
We performed a sequential comprehensive bioinformatics analysis of human sepsis cardiac transcriptome data obtained through the GEO database. The lipopolysaccharide-induced mouse SMI model was used to validate the ferroptosis features and transcriptional expression of key genes.
Results
We identified widespread dysregulation of ferroptosis-related genes (FRGs) in SMI based on the human septic heart transcriptomes, deeply explored the underlying biological mechanisms and crosstalks, followed by the identification of key functional modules and hub genes through the construction of protein-protein interaction network. Eight key FRGs that regulate ferroptosis in SMI, including HIF1A, MAPK3, NOX4, PPARA, PTEN, RELA, STAT3 and TP53, were identified, as well as the ferroptosis features. All the key FRGs showed excellent diagnostic capability for SMI, part of them was associated with the prognosis of sepsis patients and the immune infiltration in the septic hearts, and potential ferroptosis-modulating drugs for SMI were predicted based on key FRGs.
Conclusion
This study provides human septic heart transcriptome-based evidence and brings new insights into the role of ferroptosis in SMI, which is significant for expanding the understanding of the pathobiological mechanisms of SMI and exploring promising diagnostic and therapeutic targets for SMI.
Introduction
Sepsis, one of the leading causes of death in critically ill patients worldwide, is a life-threatening organ dysfunction caused by a dysregulated host response to infection.Citation1,Citation2 Although the prognosis of sepsis patients has improved with the development of therapeutic measures such as intensive care and antibiotic application, it is still considered a major public health problem with significant health care and social impact due to the high morbidity and mortality.Citation3–5 The pathogenesis of sepsis is complex, and not only involves systemic inflammation but also dysfunction of multiple organs.Citation1 Cardiac dysfunction caused by septic myocardial injury (SMI) is a common and lethal manifestation of sepsis, and is associated with septic shock and increased mortality.Citation6,Citation7 Clinical and pathological research related to SMI is progressing worldwide, however, there is still a lack of characteristic biomarkers and precise clinical diagnostic and therapeutic strategies.Citation8–11 Therefore, it is crucial to elucidate the molecular basis of SMI to identify promising targets for its prevention, diagnosis and treatment.Citation12
Disruption in iron homeostasis is one of the critical pathological features of sepsis and SMI, and entails increased iron transport and uptake into cells and decreased iron export.Citation13,Citation14 There is evidence of iron homeostasis disorder in both circulating blood and organ tissues of septic patients, which has been found to be related to the clinical prognosis.Citation15–17 Since ferroportin is the only known iron exporter in vertebrate cells, cellular iron overload is susceptible to occur when iron homeostasis is disordered.Citation14 Iron is an important trace element involved in multiple biological processes such as DNA synthesis and energy production. However, the accumulation of unstable iron ions can lead to oxidative damage and cell death when cellular iron is overloaded.Citation13 Ferroptosis is a form of programmed cell death driven by iron-dependent lipid peroxidation, which has unique morphological, genetic, and biochemical characteristics.Citation17,Citation18 Recent studies have reported ferroptosis in both in vivo and in vitro SMI models, and inhibition of ferroptosis by small molecule compounds has been found to be protective in SMI models.Citation19–21 Despite increased research focus on the role of ferroptosis in SMI, the current understanding of its molecular biology is still scattered and unclear, further in-depth exploration is necessary and urgent, which may lead to promising targets for diagnosis and treatment.
Deep transcriptomic analyses based on human pathological tissues contribute to bring the closest insights to reality in exploring the molecular biological mechanisms in various diseases, many of which have translated into clinical benefits.Citation22–25 However, current studies on the mechanisms of ferroptosis in SMI still lack important information from human heart samples. Here, we performed an in-depth analysis of the human septic heart transcriptomes, which identified the variations of ferroptosis-related genes (FRGs) in SMI, and further explored their potential biological functions and pathways. A protein-protein interaction (PPI) network was constructed to identify key functional modules and hub genes. The expression of hub genes and ferroptosis features was then validated in the mouse SMI model, and the diagnostic capability and prognostic relevance of key FRGs for SMI were subsequently evaluated. The expression and distribution of key FRGs were determined through human heart single-cell transcriptome data. Furthermore, we performed immune infiltration correlation analyses of identified key FRGs in septic hearts given the tight association between SMI, ferroptosis, and immune infiltration.Citation8,Citation26–28 Finally, we predicted potential ferroptosis-modulating drugs for SMI based on the drug-target correlation of key FRGs, and performed molecular docking for further exploration.
Materials and Methods
Data Collection
As described in our previous study,Citation29 the microarray datasets GSE79962 and GSE54514 were retrieved from the GEO database (https://www.ncbi.nlm.nih.gov/geo/). The transcriptomic data of 11 control hearts (sourced from non-failed donors) and 20 septic hearts (sourced from patients who died from sepsis) were obtained from the GSE79962 dataset, while the transcriptomic data of whole blood samples (within 24 hours of admission to the intensive care unit) from 26 sepsis survivors and 9 sepsis nonsurvivors were obtained from the GSE54514 dataset. After probe merging and ID conversion, all expression data were log2 transformed and quantile normalized before further analyses.
Transcriptomic Differential Analysis and Identification of Differentially Expressed Genes (DEGs) and Differentially Expressed FRGs (DEFRGs)
The limma package (version 3.48.0) in the R software (version 4.1) was used to screen the DEGs in the septic hearts, and the Benjamini-Hochberg correction method was used to control the false-discovery rate (FDR). Referring to previous studies,Citation30,Citation31 the thresholds we set for selecting the DEGs were FDR < 0.05 and |log2FC| ≥ 0.25. The FRGs were derived from the ferroptosis-related database FerrDB (version dated 10 May 2022) (http://www.zhounan.org/ferrdb/),Citation32 and the combined FRG set included a total of 388 FRGs after deduplication of genes (Table S1). DEGs and FRGs were overlap analyzed using the VennDiagram package in R software and the overlapped genes were defined as DEFRGs.
Gene Set Enrichment Analysis (GSEA)
To evaluate the overall correlation between FRGs and septic hearts, GSEA was performed using the Sangerbox online tool (http://vip.sangerbox.com/).Citation33 Genes in the ferroptosis-associated gene set obtained as mentioned above were scored and ranked by expression value in the GSE79962 dataset. Normalized enrichment score (NES) was calculated and FDR < 0.05 was considered significant.
Gene Ontology (GO) and Kyoto Encyclopedia of Genes and Genomes (KEGG) Enrichment Analyses
As described in our previous study,Citation29 the identified DEFRGs were subjected to GO and KEGG enrichment analyses using the clusterProfiler package in the R software.Citation34 FDR < 0.05 was the criterion for significantly enriched by DEFRGs.
PPI Network and Identification of Key Modules and Hub Genes
The STRING database (https://string-db.org/) and Cytoscape software were used to establish and visualize a PPI network of DEFRGs as described in our previous studies.Citation29 Functional key modules were identified from the PPI network by the MCODE plugin using the K-means clustering algorithm (degree cutoff = 2, node score cutoff = 0.2, K-core = 2). The genes in the PPI network were assigned and ranked accordingly by Cytohubba’s built-in MCC algorithm, and the top ten genes were screened as hub genes.
Animals and Establishment of the SMI Model
Male BALB/c mice (8–10 weeks old) were adopted, provided by Charles River Laboratories. Mice were acclimatized for 1 week at 23±1°C under a 12 hours light/dark period, housed with bacteria-free water and food provided ad libitum. Twenty-one mice were used in the control group, while twenty-four mice per group were used in the experimental groups. To induce SMI, the mice were injected intraperitoneally with lipopolysaccharide (LPS, Sigma-Aldrich, USA) (10 mg/kg). The sham-operated controls received an equal volume of PBS. The LPS+Fer-1 group was pre-treated with Ferrostatin-1 (Fer-1, Sigma-Aldrich, USA) (5 mg/kg) 30 min before LPS injection. After 24 hours of LPS injection, mice received transthoracic echocardiography to identify the heart functions. The mice were then euthanized, and the blood and heart samples were collected for subsequent experiments. All animal experiments were approved by the Animal Experimentation Ethics Committee of the First Affiliated Hospital of Nanchang University (ethics number: CDYFY-IACUC-202209QR004), and all the laboratory procedures were followed the “Laboratory Animals-Guideline of welfare and ethics” of the State Standard of P.R. China for the welfare of animals.
Echocardiography
The mice were anesthetized with 1.5% isoflurane, and cardiac function was evaluated by two-dimensional transthoracic echocardiography using a Vevo2100 imaging system (VisualSonics, Canada). All measurements were performed by an experienced operator blinded to the study.
Biochemical Analyses
Blood samples were collected from the abdominal aorta. Serum levels of lactate dehydrogenase (LDH), creatine kinase-myoglobin binding (CK-MB), cardiac troponin T (cTnT), interleukin (IL)-6, IL-1β and tumor necrosis factor (TNF)-α were measured using specific ELISA kits (Nanjing Jiancheng Bioengineering Institute, China) according to the manufacturer’s instructions. In addition, freshly collected myocardia (with removal of atriums) were homogenized in cold, the malondialdehyde (MDA), superoxide dismutase (SOD), glutathione peroxidase (GSH-Px), and reduced (GSH)/oxidized (GSSG) glutathione (GSH/GSSG) ratios were measured using specific kits according to the manufacturer’s instructions (Nanjing Jiancheng Bioengineering Institute, China). The absorbance was measured using a Spark® multimode microplate reader (Tecan, Switzerland).
Measurement of Cardiac Iron Content
The myocardia (with removal of atriums) were weighed, homogenized and lysed. The iron content in the myocardium was measured using a kit according to the manufacturer’s instructions (Pulilai Gene Technology, China). The absorbance was measured at 550 nm using the Spark® multimode microplate reader (Tecan, Switzerland).
Histological Analysis
Isolated hearts were fixed in 10% formalin, dehydrated and paraffin-embedded, and cut into 5-μm cross-axis sections. Hematoxylin and eosin (H&E) staining was performed according to the standard protocols. For immunohistochemical staining, the sections were sequentially probed with the anti-4 hydroxynonenal antibody (4-HNE, 1:200, Bioss, China), biotin-labeled goat anti-rabbit IgG antibody (1:100, Beyotime, China) and HRP-labeled Streptavidin (1:300, Beyotime, China), then HRP was detected by DAB kit (Beyotime, China).
Detection of Radical Oxygen Species (ROS)
Fresh isolated hearts were frozen sectioned after OCT embedding. The sections were incubated with a dihydroethidium (DHE) probe (Yeasen, China) at 37°C for 60 min away from light, and imaged under a fluorescence microscope (Nikon Eclipse Ci, Japan) fitted with a digital camera (Nikon DS-U3, Japan).
Transmission Electron Microscopy (TEM) Imaging
Fresh mice left ventricular myocardium (1 mm × 1 mm × 1 mm) were rapidly harvested. After fixation, washing, dehydration, embedding, sectioning and staining. The ultrastructure of myocardial mitochondria was observed by TEM (Hitachi 7800, Japan), and the Flameng score method was used to evaluate the ultrastructural damage of mitochondria.Citation35
Western Blot Analysis
Western blotting was performed as described in our previous study.Citation29 Briefly, the myocardium (with removal of atriums) was homogenized and lysed, and the extracted proteins were separated by 10% sodium dodecyl sulfate-polyacrylamide gel electrophoresis (SDS-PAGE) and transferred to PVDF membranes. After blocking with 5% non-fat dry milk at room temperature for 2 hours, the blots were incubated overnight with primary antibodies against PTGS2 (Proteintech #12375-1-AP, China, 1:1000) and β-actin (Biosharp #BL005B, China, 1:2000) at 4°C. Subsequently, the membrane was incubated with the secondary antibody (Beyotime # A0208, China, 1:2000) for 2 hours at room temperature. The positive bands were visualized using the Ultra High Sensitivity ECL kit (Beyotime, China) and imaged using FluorChem FC3 (ProteinSimple, USA). The blots were densitometrically scanned using the ImageJ software (NIH, USA), and β-actin was selected as the internal reference according to previous studies.Citation36,Citation37
Quantitative Real-Time PCR (qRT-PCR)
The protocol for qRT-PCR has been described in our previous study.Citation29 Briefly, myocardia (with removal of atriums) were homogenized in TRIzol (Invitrogen, USA) to extract total RNA. After removal of genomic DNA by DNase treatment, total RNA was then reverse-transcribed into cDNA using RevertAid MM (ThermoFisher Scientific, USA) according to the manufacturer’s instruction. qRT-PCR was performed using the Power SYBR Green PCR Master Mix (ThermoFisher Scientific, USA) on the real-time PCR system (StepOnePlus Real-Time PCR System, Applied Biosystems, USA). According to previous studies,Citation38,Citation39 for normalization, ACTB levels were used as an internal reference and mRNA levels relative to control were calculated according to the 2−ΔΔCT method. The primers were designed using NCBI Primer-Blast and synthesized by Sangon Biotech Co. Ltd. (Shanghai, China), the sequences are listed in Table S2.
Receiver Operating Characteristic (ROC) Curve Analyses
To evaluate the diagnostic accuracy of genes, ROC curves were plotted as described in our previous study.Citation29 The area under the ROC curve (AUC) was used to quantify the classification. Genes with AUC > 0.6 were considered diagnostic, and those with AUC > 0.8 were considered excellently diagnostic.
Single-Cell Sequencing Analyses
The Single Cell Portal (https://singlecell.broadinstitute.org/single_cell) was availed to obtain single cell sequencing data for key FRGs. The single-cell sequencing data of the human fetal heart and adult heart from SCP498 and SCP1021 were used.Citation40,Citation41
Immune Infiltration Analyses
The Sangerbox platform (http://vip.sangerbox.com/) was used to perform immune infiltration analyses as previously described.Citation29 The proportion of 22 immune cell species was calculated using the CIBERSORT algorithm based on normalized gene expression data.
Potential Therapeutic Drug Prediction and Molecular Docking
The potential ferroptosis-regulating drugs for SMI were predicted by the Enrichr online enrichment analysis tool (http://amp.pharm.mssm.edu/Enrichr) based on the protein-drug interaction data from the DSigDB database (http://dsigdb.tanlab.org/).Citation42,Citation43 The corresponding FDR and the combined score were calculated as described in the study of Avi Ma’ayan et al.Citation43 FDR < 0.05 and combined score > 10,000 were set as cut-offs for valid drug candidates, while higher combined scores were considered to have a stronger interaction between the drug and target genes.
Molecular docking was used to model the combination between drug and target proteins, and to predict the extent of the interaction. The chemical structures of the predicted drugs and target proteins were obtained from the PubChem database (http://pubchem.ncbi.nlm.nih.gov),Citation44 the RCSB protein data bank database (https://www.rcsb.org),Citation45 and the AlphaFold protein structure database (https://alphafold.ebi.ac.uk/).Citation46 After collating the receptor protein and ligand small molecule compounds separately, molecular docking was performed using the CDOCK module in Discovery Studio 2019 software (BIOVIA, USA).
Statistical Analyses
All the statistical analyses not mentioned above were performed using the Sangerbox platform and the GraphPad Prism (version 8.0.2, La Jolla, CA). Comparisons between two groups in the transcriptomic analyses which not mentioned above were performed using signed-rank test. Ordinary one-way ANOVA was used for comparisons between multiple groups in the animal experiments. The Spearman correlation test was used for correlation analysis. Data are presented in mean ± SD. The Benjamini-Hochberg correction method was applied to adjust the P values for FDR, and statistical significance was considered at FDR < 0.05. P values are shown as indicated: *P < 0.05, **P < 0.01, ***P < 0.001, ****P < 0.0001, ns = not significant.
Results
Overall Study Protocol
The illustrative and detailed protocol of our study is summarized ( and ). All the raw data were normalized before further analyses, as shown in Figure S1.
Figure 1 The overall protocol of this study. The illustrative (A) and detailed (B) protocol of this study, drawn by Figdraw.
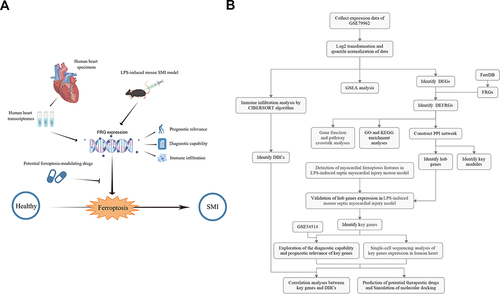
Widespread Dysregulation of FRGs in the Human Septic Heart Transcriptomes
GSEA is a commonly utilized method to evaluate the overall relevance of specific characteristic gene set in the disease transcriptome to the disease phenotype.Citation22 To evaluate whether the variation of the FRG set in the human septic heart transcriptome is significant, the GSEA of total FRG set in the GSE79962 dataset was performed. The result of GSEA showed that FRGs were dramatically differentially expressed in the two groups, and the FRG set showed significant positive correlation with the septic heart phenotype compared to the control (FDR < 0.05, NES = 1.489) (Figure S2). This result suggests that the widespread dysregulation of FRGs is present in the human septic heart transcriptomes as an essential feature, which indicates a functional correlation between ferroptosis and SMI.
Identification of DEGs and DEFRGs
There were significant transcriptomic differences between control and septic hearts, as shown in the clustered heatmap of genes in the top and last 250 of the log2FC ranking (Figure S3). There were 2316 DEGs identified from the GSE79962 dataset, as listed in Table S3, of which 1031 were upregulated and 1285 were downregulated in the septic hearts ().
Figure 2 Identification of differentially expressed ferroptosis-related genes. Volcano plot of DEGs in the GSE79962 dataset (FDR < 0.05 and |log2FC| ≥ 0.25). (B) Venn diagram showing the overlap of genes between DEGs in the GSE79962 dataset and FRGs from FerrDB. (C) Clustered heatmap of DEFRGs in the GSE79962 dataset. (D) Correlation heatmap of DEFRGs in the septic hearts from the GSE79962 dataset. Ncontrol = 11, Nseptic heart = 20.
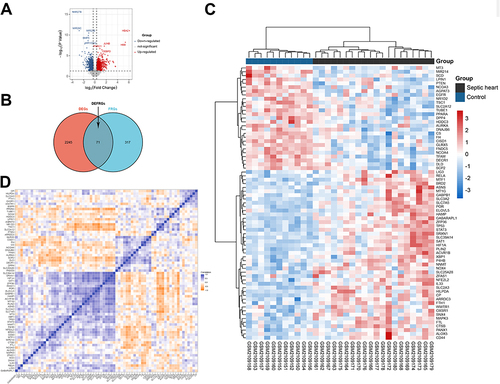
Furthermore, we identified DEFRGs in the GSE79962 dataset since the GSEA result showed a meaningful association between the FRG set and septic heart phenotype. 71 out of the 388 FRGs obtained from FerrDB overlapped with the DEGs, which were identified as DEFRGs for subsequent analyses (), as listed in Table S4. These 71 DEFRGs were significantly differentially expressed in control and septic hearts, as shown in the clustered heatmap (). Further analysis of expression correlations between DEFRGs in septic hearts revealed numerous significant correlations, as shown in the correlation heatmap (). For example, HIF1A was significantly positively correlated with SAT1 (r = 0.87), and POR was significantly positively correlated with ELOVL5 (r = 0.84) and negatively correlated with NR1D2 (r = −0.86) and TSC1 (r = −0.82). These extensive correlations between genes suggest that intricate functional networks may exist in DEFRGs.
GO and KEGG Enrichment Analyses
To explore the functions and related pathways of the DEFRGs, we performed GO and KEGG enrichment analyses. The GO enrichment analyses showed that the DEFRGs are mainly involved in the biological processes (BP) of cellular iron ion homeostasis and response to nutrient, oxygen, and chemical stress (). Furthermore, cellular components (CC) to which the DEFRGs localize include apical part, basal plasma membrane, autophagosome or autolysosome (). Among the molecular functions (MF), the DEFRGs were associated with the binding of DNA-binding transcription factor, ubiquitin protein ligase, transcription coregulator and coactivator, heat shock protein and the activities of acyltransferase and oxidoreductase (). The KEGG enrichment analysis showed that the DEFRGs are mostly involved in ferroptosis, reactive oxygen species, autophagy, and cancer-related pathways (). The cross-talks between gene functions and pathways were further analyzed, as shown in . DEFRGs have an intricate web-like relationship with different BP, CC, MF, and KEGG pathways, and the results suggested that FRGs regulate the progression of SMI may via the cross-talks of multiple gene functions and pathways rather than individually.
Figure 3 GO and KEGG enrichment analyses of differentially expressed ferroptosis-related genes. GO enrichment analyses of DEFRGs in (A) the biological process category (BP); (B) the cellular component category (CC); (C) the molecular function category (MF). (D) KEGG enrichment analysis of DEFRGs. Crosstalk analysis between DEFRGs and (E) gene functions in BP; (F) gene functions in CC; (G) gene functions in MF; (H) KEGG pathways.
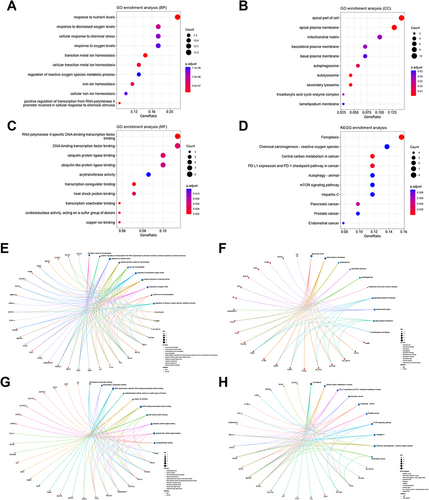
Construction of PPI Network and Identification of Key Modules and Hub Genes
Given the cross-talk of multiple genes and pathways in FRGs-mediated regulation of SMI, we subsequently constructed a PPI network of DEFRGs using the STRING database to identify functional gene clusters and individual genes among DEFRGs (). Two key modules () and ten hub genes (STAT3, NOX4, TP53, HIF1A, NFE2L2, MAPK3, RELA, PTEN, EGFR, PPARA) () were identified. Notably, the identified hub genes were exactly overlapped with one of the key modules, which further demonstrates the important pivotal role of these genes as major functional clusters in DEFRGs. and show the multiple correlations between hub genes and with other DEFRGs.
Figure 4 PPI network and identification of hub genes. (A) PPI network of all DEFRGs constructed by STRING database, the no connected dots were hidden. (B) Modules identified by the MCODE plugin using the K-means clustering algorithm (degree cutoff = 2, node score cutoff = 0.2, K-core = 2). (C) The expression variations of hub genes ranked by MCC algorithm in the septic hearts from the GSE79962 dataset. (D) Cross-talks between hub genes, the deeper color of the dot means that the rank order of the hub gene is more advanced. (E) Cross-talks between hub genes and other DEFRGs.
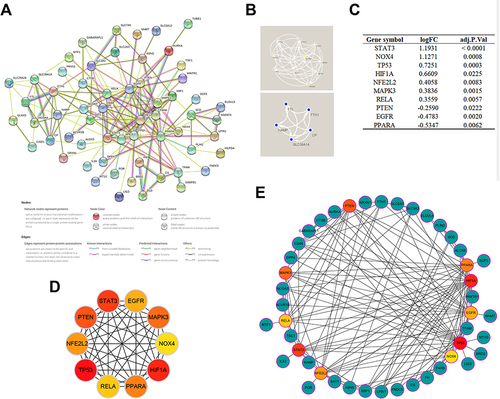
Ferroptosis Features in LPS-Induced Mouse Septic Myocardial Injury Model
The LPS-induced mouse SMI model is a commonly used experimental model for exploring SMI in vivo, which we used here to observe the ferroptosis features and hub gene expression in SMI myocardium.Citation19,Citation21,Citation47 Cardiac dysfunction in the SMI mice was manifested by reduced left ventricular ejection fraction and fractional shortening (), along with increased serum inflammatory factors (IL6, IL-1β, TNF-α) and myocardial injury markers (LDH, CK-MB, cTnT), which could be partially rescued by the ferroptosis inhibitor Fer-1 (). The ferroptosis marker PTGS2 was upregulated in the myocardium of SMI mice at both mRNA and protein levels (). Consistent with this, the inflamed myocardial tissues of SMI mice exhibited the biochemical and morphological features of ferroptosis, such as high levels of ROS and 4-HNE, and extensive mitochondrial damage characterized by atrophy and matrix loss ( and ). Furthermore, we also observed increased levels of iron and MDA, decreased levels of SOD and GSH-Px, and reduced GSH/GSSG ratio in the myocardia of SMI mice (). Fer-1 protected against most of these pathological alterations, although there was no significant decrease in cardiac iron content, most likely due to the fact that Fer-1 only chelates Fe2+ rather than expelling iron from cells.Citation48 These results suggest that ferroptosis is a crucial pathological driver of SMI, and targeting ferroptosis pathways can be a promising therapeutic strategy for SMI.
Figure 5 Altered ferroptosis features in LPS-induced mouse septic myocardial injury model. Male BALB/c mice (8–10 weeks old) were treated with LPS (10 mg/kg) for 24 hours in the presence or absence of Fer-1 (5 mg/kg) pretreatment, while the sham-operated controls received an equal volume of PBS, followed by subsequent experiments. Cardiac function evaluated by echocardiography in each group (A), quantified as (B) left ventricular ejection fraction (LVEF), (C) left ventricular fractional shortening (LVFS%) (N = 3 per group). Serum levels of (D) LDH, (E) CK-MB, (F) cTnT, (G) IL-6, (H) IL-1β, (I) TNF-α in each group (N = 3 per group). (J) Representative Western blotting bands of PTGS2. Quantification of (K) mRNA and (L) protein expression levels of PTGS2 in the left ventricular myocardium in each group (N = 9 for mRNA and N = 3 for protein per group). (M) Representative images of HE, DHE (ROS detection), 4-HNE staining and transmission electron microscopy in the left ventricular myocardium in each group (N = 3 per group). (N) Ultrastructural damage evaluated with the Flameng score method in each group. Cardiac levels of (O) iron, (P) SOD, (Q) MDA, (R) GSH-Px and (S) GSH/GSSG ratios in each group (N = 3 per group). *P < 0.05, **P < 0.01, ***P < 0.001, ****P < 0.0001, Data are presented as the mean ± SD, n ≥ 3.
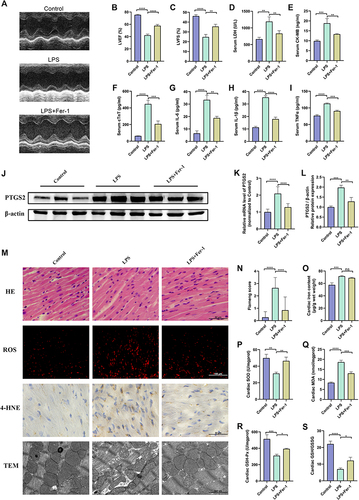
Identification of Key FRGs and Exploration of Their Diagnostic Capability and Prognostic Relevance
We sequentially validated the expression of hub genes in the myocardium of SMI mice. As shown in , most of these genes showed trends consistent with that in the human septic heart transcriptome, such as increased expression of HIF1A, MAPK3, NOX4, RELA, STAT3 and TP53, and decreased expression PPARA and PTEN. The Fer-1 treatment could partially but not completely reverse these alterations, suggesting that these genes may participate in other biological processes alongside ferroptosis. Interestingly, the EGFR expression was similar in the myocardium of SMI and control mice, while NFE2L2 even showed opposite expression trend, which may be attributed to the presence of individual differences or different biological circumstances, and we excluded them as key genes given these uncertain expression alterations.
Figure 6 Identification of key FRGs and exploration of their diagnostic capability and prognostic relevance. mRNA expression levels of (A) EGFR, (B) HIF1A, (C) MAPK3, (D) NFE2L2, (E) NOX4, (F)PPARA, (G) PTEN, (H) RELA, (I) STAT3, (J) TP53 in each group detected by qRT-PCR (N = 9 per group). ROC curves show the diagnostic capability of (K) HIF1A, (L) MAPK3, (M) NOX4, (N) PPARA, (O) PTEN, (P)RELA, (Q) STAT3, (R) TP53 for SMI in hearts from the GSE79962 dataset, Ncontrol = 11, Nseptic heart = 20. (S) Comparison of key FRG expression in whole blood from sepsis survivors and nonsurvivors in GSE54514, Nsurvivor = 26, NNon-survivor = 9. Linear regression plots presenting the significant correlation of APACHE II score-quantified severity with PPARA (T) and TP53 (U) expression in whole blood from GSE54514, N = 35. *P < 0.05, **P < 0.01, ***P < 0.001, ****P < 0.0001. Data are presented as the mean ± SD.
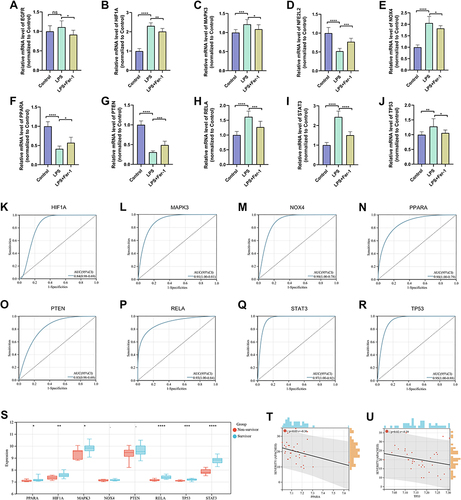
We finally identified HIF1A, MAPK3, NOX4, PPARA, PTEN, RELA, STAT3 and TP53 as key FRGs, and further ROC analyses revealed that all of them have excellent diagnostic capability for septic heart (AUC > 0.8) (). Furthermore, the expression of several key FRGs (PPARA, HIF1A, MAPK3, RELA, TP53 and STAT3) was significantly lower in the whole blood of sepsis non-survivors on the first day of ICU admission compared to survivors, indicating their relevance to the prognosis of sepsis (Figure 6S), further analysis revealed that PPARA and TP53 expression in whole blood of sepsis patients was significantly negatively correlated with APACHEII scores-quantified severity ( and , S4). Interestingly, while SMI in general usually leads to poor prognosis in sepsis, we found the variability trends of key FRGs in the septic hearts do not fully coincide with those in whole blood of sepsis non-survivors, probably due to the comprehensive pathological condition of sepsis as a complex systemic disease. To conclude, these key genes that regulating ferroptosis in SMI have the potential to serve as biomarkers for SMI.
Distribution of Key FRGs in Human Heart
To explore the distribution of key FRGs in human heart, we investigated single-cell sequencing of key FRG expression using the Single Cell Portal. All key FRGs were expressed to varying degrees in both human fetal and adult hearts ( and ). Noticeably, while key FRGs are predominantly expressed in cardiomyocytes, they are also expressed in other cell types such as immune cells.
Immune Infiltration Analyses
Immune cells play an important role in many cardiac diseases.Citation49,Citation50 The immune and inflammatory responses are critical to the pathological progression of SMI.Citation8,Citation51 Since single-cell sequencing analysis revealed that the key FRGs are also expressed in immune cells, while a close association between ferroptosis and immune cell function and activity has been reported in other biological environments, the immune infiltration analyses were further performed in our study to explore the cross-talks between ferroptosis and immune cells in SMI.Citation28,Citation52,Citation53 We estimated the relative proportions of infiltrating immune cells in each heart sample from the GSE79962 dataset using the CIBERSORT algorithm, as shown in , while the clustered heatmap of infiltrating immune cells shows the differences between control and septic hearts (). M2 macrophage was found at the highest proportion among immune cells in these heart samples, and it was lower in septic hearts (0.35 ± 0.08 vs 0.37 ± 0.08, septic hearts vs control), corresponding to a higher proportion of M1 macrophage (0.02 ± 0.04 vs 0.005 ± 0.01, septic hearts vs control) However, M1 and M2 macrophage did not show significance due to large individual differences (p > 0.05). The proportion of infiltrating neutrophils and resting NK cells was significantly increased in the septic hearts, while that of resting mast cells and CD8+ T cells were significantly decreased, which were identified as differentially infiltrating immune cells (DIICs) in the SMI myocardium ().
Figure 8 Correlation analyses between the proportion of DIICs and the expression of key FRGs. (A) The proportion of infiltrating immune cells estimated by the CIBERSORT algorithm on hearts from the GSE79962 dataset. (B) Clustered heatmap of the proportion of DIICs in the GSE79962 dataset (C) Comparison of infiltrating immune cells proportion between control and septic hearts in the GSE79962 dataset, immune cell with zero proportion was hidden. (D) Correlation heatmap of DIICs proportion with key FRGs expression in the septic hearts from the GSE79962 dataset. Ncontrol = 11, Nseptic heart = 20. *P < 0.05, ***P < 0.001.
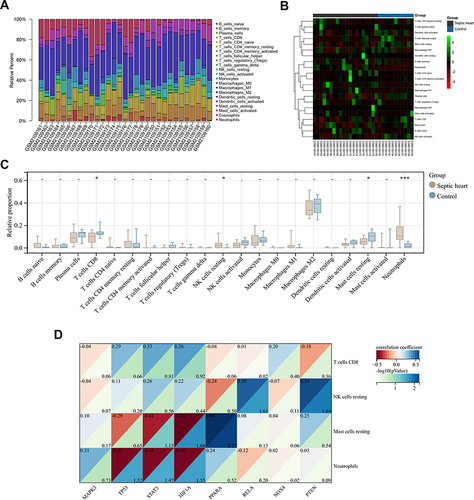
Further analyses revealed correlations between immune cells (Figure S5A) and with several key FRGs in the septic hearts (), the proportion of resting mast cells was significantly positive correlated with PPARA expression and significantly negative correlated with HIF1A expression, and the proportion of neutrophils was significantly negative correlated with TP53, HIF1A, and STAT3 expression, while that of resting NK cells was significantly positive correlated with PTEN and RELA expression (Figure S5B). In contrast, none of these correlations were significant in control hearts, suggesting that the correlation between key FRGs and immune cells may be pathological rather than physiologically present.
Potential Therapeutic Drug Prediction and Molecular Docking
We screened for potential drug candidates for SMI based on the key FRGs using the DSigDB database, which may treat SMI by modulating ferroptosis. Finally, 13 potential therapeutic drugs were predicted, as listed in Table S5. shows the top ten predicted potential therapeutic drugs ranked according to the combined score, and resveratrol (C14H12O3) was the most strongly drug-target correlated drug predicted for ferroptosis-related key genes (Combined Score = 2975802) (). Further molecular docking simulations showed that resveratrol formed stable complexes with all key FRGs (), especially PTEN (-CDOCKER Interaction Energy = 38.57) and RELA (-CDOCKER interaction energy = 33.56), as shown in .
Figure 9 Potential therapeutic drug prediction and molecular docking. (A) The top ten potential therapeutic drugs ranked according to combined score in the DSigDB database. (B) The chemical structures of resveratrol. (C) Molecular docking simulations showed that resveratrol formed stable complexes with all key FRGs. (D) Detailed information on molecular docking simulations of resveratrol with all key FRGs.
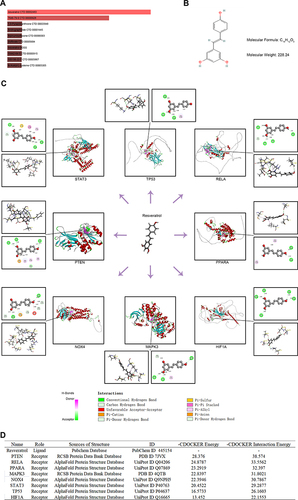
Discussion
Given the diagnostic uncertainty and serious prognostic impact of SMI, it is crucial to elucidate the underlying pathophysiological mechanisms, and identify reliable diagnostic markers and therapeutic targets for clinical practice. Ferroptosis has recently been reported as a noteworthy pathological mechanism in SMI, however, the underlying functional and regulatory mechanisms still unclear. Rapid advances in transcriptomic studies have improved our understanding of disease. In this study, we comprehensively analyzed dysregulated FRGs in the human septic heart transcriptomes, including regulatory mechanisms, functional pathways, key genes and their diagnostic capacity, immune infiltration relevance and potential ferroptosis-targeting drugs, and further validated prognostic relevance and key gene expression through an independent cohort of sepsis patients and in vivo SMI model, which provides new insights to explore the role of ferroptosis in the development of SMI.
Widespread dysregulation of ferroptosis-related genes in the septic hearts was identified by GSEA, suggesting that ferroptosis is an essential pathological mechanism of SMI, which corroborates previous studies.Citation14,Citation47,Citation54 Further GO enrichment analyses revealed that the cellular component localization, molecular functions, and biological processes of dysregulated FRGs in SMI have mostly been demonstrated or observed to be associated with ferroptosis in different biological circumstances. Ferroptosis is a form of programmed cell death caused by iron-dependent lipid peroxidation and massive accumulation of ROS, and its cellular sensitivity was demonstrated to be controlled by a combination of energy metabolism, iron homeostasis and oxidative stress responses.Citation18,Citation55 Lipid peroxidation of the plasma membrane is an essential part of the ferroptosis process, ferritinophagy is also being focused on as a regulatory process of intracellular iron metabolism and cascade response to ferroptosis.Citation18,Citation56,Citation57 Transcription factor regulation, protein ubiquitination, and regulation of oxidoreductase activity have been reported as key functions of many ferroptosis-regulating molecules that have a strong influence on the ferroptosis process.Citation58–60 Apart from ferroptosis and ROS-related pathways, the dysregulated FRGs in SMI were also enriched in some cancer-related pathways, suggesting that some key molecules and pathways that regulate ferroptosis in SMI may not yet be defined. We believe that these enrichment results and the cross-talks between them warrant further investigation to clarify the specific mechanisms that affecting and being affected by ferroptosis in SMI.
We identified eight key dysregulated FRGs in the septic hearts by constructing a PPI network, and validated them in an in vivo model of SMI. All key FRGs had convincing evidence to support their regulation of ferroptosis in different biological circumstances,Citation32 in which NOX4, PTEN and MAPK3 are positive regulators,Citation61–63 while PPARA and RELA are negative regulators,Citation64,Citation65 and HIF1A, STAT3 and TP53 were bidirectional regulators.Citation66–68 Interestingly, while septic hearts were generally in a ferroptosis-activated state, the expression of the positive regulator PTEN is significantly reduced while the expression of the negative regulator RELA is significantly increased. We consider that this may attribute to their hitherto unrecognized bidirectional regulatory effect on ferroptosis or their simultaneous involvement in biological processes independent of ferroptosis in SMI. HIF1A and TP53 were identified in a previous study as key genes that regulate autophagy in SMI, and our enrichment analysis also found that dysregulated FRGs were enriched in the autophagy pathway, suggesting that autophagy and ferroptosis may be closely associated in SMI. Some key FRGs have been identified as essential targets in the development of SMI pathology, and regulation of ferroptosis may be their unidentified molecular biological function in SMI, yet further studies are required to elucidate the specific regulatory mechanisms.Citation69–73
The relationship between immune cell infiltration and SMI is complex and poorly understood. Immune and inflammatory responses are a double-edged sword in the pathological development of SMI.Citation8,Citation74,Citation75 The correlations between ferroptosis and immune cells have been demonstrated.Citation53 While immune cells can influence cellular ferroptosis by regulating iron metabolism or releasing cytokines, ferroptosis can also regulate the proliferation, function, activation and death of immune cells. However, the correlation between ferroptosis and immune infiltration in SMI is of interest but remains unclear. M2 macrophage, as an important anti-inflammatory immune cell, was the most abundant immune cell in the heart samples we studied. Its important functionality and abundant proportion in the heart have been reported in previous studies,Citation76–78 but it did not show differences in the control and septic hearts we studied, suggesting that there may be individual differences in its role in SMI which require further research. The proportion of infiltrating neutrophils and resting NK cells were increased in the septic hearts we analyzed, while that of infiltrating resting mast cells and CD8+ T cells was decreased, which has been partially observed in previous research.Citation79–82 Several significant correlations between key FRGs and infiltrating immune cells in the septic hearts were identified, which may be pathological cross-talks between ferroptosis and abnormal immune responses in SMI as these correlations were not present in the healthy counterparts. Nevertheless, some immune cells can exhibit both proinflammatory and antiinflammatory biological functions, and hyper-inflammation may coexist with immunoparesis in SMI, immune infiltration in SMI also varies by individual and disease stage, thus further investigation of the relationship between ferroptosis and immune infiltration in SMI is required.Citation8,Citation83,Citation84
There is considerable interest in exploring effective therapies for diseases based on essential pathobiological targets.Citation3,Citation85 Here, we predicted potential ferroptosis-modulating drugs for SMI based on the key FRGs, and resveratrol in particular showed high drug-target relevance. Echoing our study, resveratrol has been shown to alleviate SMI through multiple mechanisms, such as enhancement of SERCA2a activity by promoting the phospholamban oligomerization,Citation86 activation of the PI3K/AKT/mTOR and Nrf2 pathways,Citation87,Citation88 and inhibition of the NF-κB signaling pathway and iron transport from plasma to myocardium.Citation88,Citation89 Ferroptosis has recently been identified as one of the mechanisms by which resveratrol alleviates SMI,Citation21 and our study provides the molecular basis and potential targets for further investigation of how resveratrol regulates ferroptosis in SMI.
Our study provides evidence and new insights into the dysregulation of FRGs in the human septic heart transcriptome to explore the role of ferroptosis in SMI. Nevertheless, some limitations ought to be considered. Although the occurrence of ferroptosis has been conclusively observed in various experimental models of SMI and targeting ferroptosis has shown a protective effect in alleviating SMI, most studies in human SMI hearts, including our study, have only identified the occurrence of ferroptosis in terms of biochemical indicators or biomarkers while the corresponding morphological features are lacking. The data on the immune cell proportions of heart samples were derived from the side evidence provided by the CIBERSORT algorithm, which may present bias. Several protein structures used for molecular docking were predicted based on available vestigial PDB structures, as complete experimentally observed protein structures were not yet available. In addition, the human septic heart transcriptomes we used for analyses may only be representative of terminal SMI patients or those with poor prognoses as derived from patients who died of sepsis. To identify more reliable diagnostic and therapeutic targets, and translate our findings to clinical benefit, further mechanistic and functional studies of the identified key FRGs to adequately understand their regulation of ferroptosis in SMI are needed.
Conclusion
We identified the widespread dysregulation of FRGs in the human septic heart transcriptomes, and further bioinformatic analysis showed that dysregulated FRGs are mainly localized to the apical part, basal plasma membrane, and autophagosome/autolysosome, and regulate iron ion homeostasis and response to nutrient, oxygen, and chemical stress by affecting the binding of DNA-binding transcription factors, ubiquitin protein ligase, transcription coregulator and coactivator, heat shock protein and the activities of acyltransferase and oxidoreductase. The ferroptosis features and expression of key FRGs (HIF1A, MAPK3, NOX4, PPARA, PTEN, RELA, STAT3 and TP53) were validated in an in vivo SMI model, and key FRGs showed excellent diagnostic capability, along with significant correlation with the prognosis and immune infiltration. Furthermore, we predicted resveratrol as a potential therapeutic drug by regulating ferroptosis in SMI. Our study provides human septic heart transcriptome-based evidence and new insights into the role of ferroptosis in SMI, which is significant for deepening the understanding of the pathobiological mechanisms of SMI and exploring diagnostic and therapeutic targets for SMI.
Data Sharing Statement
Publicly available datasets were utilized in this study. The data used to support the results of this study are available from the online website mentioned above, and further inquiries can be directed to the corresponding author.
Ethics Statement
All animal experiments were approved by the Animal Experimentation Ethics Committee of the First Affiliated Hospital of Nanchang University (ethics number: CDYFY-IACUC-202209QR004). GEO database is a publicly accessible database, and includes patient data that have obtained ethical approval. There are no other required ethical statements.
Author Contributions
All authors made a significant contribution to the work reported, whether that is in the conception, study design, execution, acquisition of data, analysis and interpretation, or in all these areas; took part in drafting, revising or critically reviewing the article; gave final approval of the version to be published; have agreed on the journal to which the article has been submitted; and agree to be accountable for all aspects of the work.
Disclosure
The authors report no conflicts of interest in this work.
Additional information
Funding
References
- Gotts JE, Matthay MA. Sepsis: pathophysiology and clinical management. BMJ. 2016;353:i1585. PMID: 27217054. doi:10.1136/bmj.i1585
- Singer M, Deutschman CS, Seymour CW, et al. The third international consensus definitions for sepsis and septic shock (Sepsis-3). JAMA. 2016;315(8):801–810. PMID: 26903338. doi:10.1001/jama.2016.0287
- Huang M, Cai S, Su J. The pathogenesis of sepsis and potential therapeutic targets. Int J Mol Sci. 2019;20(21). PMID: 31671729. doi:10.3390/ijms20215376
- Perner A, Gordon AC, De Backer D, et al. Sepsis: frontiers in diagnosis, resuscitation and antibiotic therapy. Intensive Care Med. 2016;42(12):1958–1969. PMID: 27695884. doi:10.1007/s00134-016-4577-z
- Prescott HC, Angus DC. Enhancing Recovery From Sepsis: a Review. JAMA. 2018;319(1):62–75. PMID: 29297082. doi:10.1001/jama.2017.17687
- Martin L, Derwall M, Al Zoubi S, et al. The septic heart: current understanding of molecular mechanisms and clinical implications. Chest. 2019;155(2):427–437. PMID: 30171861. doi:10.1016/j.chest.2018.08.1037
- Walley KR. Sepsis-induced myocardial dysfunction. Curr Opin Crit Care. 2018;24(4):292–299. PMID: 29846206. doi:10.1097/mcc.0000000000000507
- Hollenberg SM, Singer M. Pathophysiology of sepsis-induced cardiomyopathy. Nat Rev Cardiol. 2021;18(6):424–434. PMID: 33473203. doi:10.1038/s41569-020-00492-2
- Lin Y, Xu Y, Zhang Z. Sepsis-induced myocardial dysfunction (SIMD): the pathophysiological mechanisms and therapeutic strategies targeting mitochondria. Inflammation. 2020;43(4):1184–1200. PMID: 32333359. doi:10.1007/s10753-020-01233-w
- Stanzani G, Duchen MR, Singer M. The role of mitochondria in sepsis-induced cardiomyopathy. Biochim Biophys Acta Mol Basis Dis. 2019;1865(4):759–773. PMID: 30342158. doi:10.1016/j.bbadis.2018.10.011
- Pierrakos C, Velissaris D, Bisdorff M, Marshall JC, Vincent JL. Biomarkers of sepsis: time for a reappraisal. Critical Care. 2020;24(1):287. PMID: 32503670. doi:10.1186/s13054-020-02993-5
- Cecconi M, Evans L, Levy M, Rhodes A. Sepsis and septic shock. Lancet. 2018;392(10141):75–87. PMID: 29937192. doi:10.1016/s0140-6736(18)30696-2
- Liu Q, Wu J, Zhang X, Wu X, Zhao Y, Ren J. Iron homeostasis and disorders revisited in the sepsis. Free Radic Biol Med. 2021;165:1–13. PMID: 33486088. doi:10.1016/j.freeradbiomed.2021.01.025
- Fang X, Ardehali H, Min J, Wang F. The molecular and metabolic landscape of iron and ferroptosis in cardiovascular disease. Nat Rev Cardiol. 2022:1–17. PMID: 35788564; PubMed Central PMCID: PMCPMC9252571. doi:10.1038/s41569-022-00735-4
- Brandtner A, Tymoszuk P, Nairz M, et al. Linkage of alterations in systemic iron homeostasis to patients’ outcome in sepsis: a prospective study. J Intensive Care. 2020;8:76. PMID: 33014378. doi:10.1186/s40560-020-00495-8
- Akkaş İ, Ince N, Sungur MA. Serum trace element and heavy metal levels in patients with sepsis. Aging Male. 2020;23(3):222–226. PMID: 32183594. doi:10.1080/13685538.2020.1740200
- Xl L, Gy Z. Ferroptosis in sepsis: the mechanism, the role and the therapeutic potential. Front Immunol. 2022;13:956361. PMID: 35990689. doi:10.3389/fimmu.2022.956361
- Stockwell BR. Ferroptosis turns 10: emerging mechanisms, physiological functions, and therapeutic applications. Cell. 2022;185(14):2401–2421. PMID: 35803244. doi:10.1016/j.cell.2022.06.003
- Kong C, Ni X, Wang Y, et al. ICA69 aggravates ferroptosis causing septic cardiac dysfunction via STING trafficking. Cell Death Discov. 2022;8(1):187. PMID: 35397620. doi:10.1038/s41420-022-00957-y
- Xiao Z, Kong B, Fang J, et al. Ferrostatin-1 alleviates lipopolysaccharide-induced cardiac dysfunction. Bioengineered. 2021;12(2):9367–9376. PMID: 34787054. doi:10.1080/21655979.2021.2001913
- Wang X, Simayi A, Fu J, Zhao X, Xu G. Resveratrol mediates the miR-149/HMGB1 axis and regulates the ferroptosis pathway to protect myocardium in endotoxemia mice. Am J Physiol Endocrinol Metab. 2022;323(1):E21–e32. PMID: 35532075. doi:10.1152/ajpendo.00227.2021
- Zou HX, Qiu BQ, Lai SQ, et al. Role of ferroptosis-related genes in Stanford type a aortic dissection and identification of key genes: new insights from bioinformatic analysis. Bioengineered. 2021;12(2):9976–9990. PMID: 34652258. doi:10.1080/21655979.2021.1988840
- Banchereau R, Cepika AM, Banchereau J, Pascual V. Understanding human autoimmunity and autoinflammation through transcriptomics. Annu Rev Immunol. 2017;35:337–370. PMID: 28142321. doi:10.1146/annurev-immunol-051116-052225
- Ren X, Wen W, Fan X, et al. COVID-19 immune features revealed by a large-scale single-cell transcriptome atlas. Cell. 2021;184(7):1895–913.e19. PMID: 33657410. doi:10.1016/j.cell.2021.01.053
- O’Donoghue ML, Rosenson RS, Gencer B, et al. Small Interfering RNA to Reduce Lipoprotein(a) in Cardiovascular Disease. N Engl J Med. 2022. PMID: 36342163. doi:10.1056/NEJMoa2211023
- Wang W, Xu H, Lin H, Molnar M, Ren H. The role of the cholinergic anti-inflammatory pathway in septic cardiomyopathy. Int Immunopharmacol. 2021;90:107160. PMID: 33243604. doi:10.1016/j.intimp.2020.107160
- Dou J, Liu X, Yang L, Huang D, Tan X. Ferroptosis interaction with inflammatory microenvironments: mechanism, biology, and treatment. Biomed Pharmacother. 2022;155:113711. PMID: 36126457. doi:10.1016/j.biopha.2022.113711
- Ni S, Yuan Y, Song S, Li X. A double-edged sword with a therapeutic target: iron and ferroptosis in immune regulation. Nutr Rev. 2022. PMID: 36130411. doi:10.1093/nutrit/nuac071
- Zou HX, Qiu BQ, Zhang ZY, et al. Dysregulated autophagy-related genes in septic cardiomyopathy: comprehensive bioinformatics analysis based on the human transcriptomes and experimental validation. Front Cardiovasc Med. 2022;9:923066. PMID: 35983185. doi:10.3389/fcvm.2022.923066
- He Y, Yuan H, Wu C, Xie Z. DISC: a highly scalable and accurate inference of gene expression and structure for single-cell transcriptomes using semi-supervised deep learning. Genome Biol. 2020;21(1):170. PMID: 32650816. doi:10.1186/s13059-020-02083-3
- Zou HX, Qiu BQ, Lai SQ, et al. Iron metabolism and idiopathic pulmonary arterial hypertension: new insights from bioinformatic analysis. Biomed Res Int. 2021;2021:5669412. PMID: 34722766. doi:10.1155/2021/5669412
- Zhou N, Bao J. FerrDb: a manually curated resource for regulators and markers of ferroptosis and ferroptosis-disease associations. Database. 2020;2020. PMID: 32219413. doi:10.1093/database/baaa021
- Shen W, Song Z, Zhong X, et al. Sangerbox: a comprehensive, interaction-friendly clinical bioinformatics analysis platform. iMeta. 2022;1(3):e36. doi:10.1002/imt2.36
- Yu G, Wang LG, Han Y, He QY. ClusterProfiler: an R package for comparing biological themes among gene clusters. Omics. 2012;16(5):284–287. PMID: 22455463. doi:10.1089/omi.2011.0118
- Zhou K, Wu G, Li Y, et al. Protective effects of indomethacin and dexamethasone in a goat model with intrauterine balloon aortic valvuloplasty. J Biomed Sci. 2012;19(1):74. doi:10.1186/1423-0127-19-74
- Wu B, Song H, Fan M, et al. Luteolin attenuates sepsis‑induced myocardial injury by enhancing autophagy in mice. Int J Mol Med. 2020;45(5):1477–1487. PMID: 32323750. doi:10.3892/ijmm.2020.4536
- Boyd JH, Kan B, Roberts H, Wang Y, Walley KR. S100A8 and S100A9 mediate endotoxin-induced cardiomyocyte dysfunction via the receptor for advanced glycation end products. Circ Res. 2008;102(10):1239–1246. PMID: 18403730. doi:10.1161/circresaha.107.167544
- Anwar-mohamed A, Zordoky BN, Aboutabl ME, El-Kadi AO. Alteration of cardiac cytochrome P450-mediated arachidonic acid metabolism in response to lipopolysaccharide-induced acute systemic inflammation. Pharmacol Res. 2010;61(5):410–418. PMID: 20045729. doi:10.1016/j.phrs.2009.12.015
- Shi G, Xing L, Wu D, et al. A rare mutation of β(1)-adrenergic receptor affects sleep/wake behaviors. Neuron. 2019;103(6):1044–55.e7. PMID: 31473062. doi:10.1016/j.neuron.2019.07.026
- Tucker NR, Chaffin M, Fleming SJ, et al. Transcriptional and cellular diversity of the human heart. Circulation. 2020;142(5):466–482. PMID: 32403949. doi:10.1161/circulationaha.119.045401
- Miao Y, Tian L, Martin M, et al. Abstract 12937: single-cell transcriptomic analysis reveals developmentally impaired endocardial population in hypoplastic left heart syndrome. Circulation. 2020;142(Suppl_3):A12937–A. doi:10.1161/circ.142.suppl_3.12937
- Yoo M, Shin J, Kim J, et al. DSigDB: drug signatures database for gene set analysis. Bioinformatics. 2015;31(18):3069–3071. PMID: 25990557. doi:10.1093/bioinformatics/btv313
- Chen EY, Tan CM, Kou Y, et al. Enrichr: interactive and collaborative HTML5 gene list enrichment analysis tool. BMC Bioinform. 2013;14:128. PMID: 23586463. doi:10.1186/1471-2105-14-128
- Kim S, Chen J, Cheng T, et al. PubChem in 2021: new data content and improved web interfaces. Nucleic Acids Res. 2021;49(D1):D1388–d95. PMID: 33151290. doi:10.1093/nar/gkaa971
- Burley SK, Bhikadiya C, Bi C, et al. RCSB Protein Data Bank: powerful new tools for exploring 3D structures of biological macromolecules for basic and applied research and education in fundamental biology, biomedicine, biotechnology, bioengineering and energy sciences. Nucleic Acids Res. 2020;49(D1):D437–D51. doi:10.1093/nar/gkaa1038
- Jumper J, Evans R, Pritzel A, et al. Highly accurate protein structure prediction with AlphaFold. Nature. 2021;596(7873):583–589. PMID: 34265844. doi:10.1038/s41586-021-03819-2
- Li N, Wang W, Zhou H, et al. Ferritinophagy-mediated ferroptosis is involved in sepsis-induced cardiac injury. Free Radic Biol Med. 2020;160:303–318. PMID: 32846217. doi:10.1016/j.freeradbiomed.2020.08.009
- Miotto G, Rossetto M, Di Paolo ML, et al. Insight into the mechanism of ferroptosis inhibition by ferrostatin-1. Redox Biol. 2020;28:101328. PMID: 31574461. doi:10.1016/j.redox.2019.101328
- Rosenzweig R, Kumar V, Gupta S, et al. Estrogen receptor-β agonists modulate t-lymphocyte activation and ameliorate left ventricular remodeling during chronic heart failure. Circ Heart Fail. 2022;15(7):e008997. PMID: 35730443. doi:10.1161/circheartfailure.121.008997
- Kumar V, Prabhu SD, Bansal SS. CD4(+) T-lymphocytes exhibit biphasic kinetics post-myocardial infarction. Front Cardiovasc Med. 2022;9:992653. PMID: 36093172. doi:10.3389/fcvm.2022.992653
- Liu YC, Yu MM, Shou ST, Chai YF. Sepsis-induced cardiomyopathy: mechanisms and treatments. Front Immunol. 2017;8:1021. PMID: 28970829. doi:10.3389/fimmu.2017.01021
- Xu S, Min J, Wang F. Ferroptosis: an emerging player in immune cells (News&Views). Sci Bull. 2021;2021:2731–2744.
- Wang P, Lu YQ. Ferroptosis: a critical moderator in the life cycle of immune cells. Front Immunol. 2022;13:877634. PMID: 35619718. doi:10.3389/fimmu.2022.877634
- Cao G, Zeng Y, Zhao Y, et al. H2S regulation of ferroptosis attenuates sepsis‑induced cardiomyopathy. Mol Med Rep. 2022;26(5). PMID: 36102305. doi:10.3892/mmr.2022.12851
- Jiang X, Stockwell BR, Conrad M. Ferroptosis: mechanisms, biology and role in disease. Nat Rev Mol Cell Biol. 2021;22(4):266–282. PMID: 33495651. doi:10.1038/s41580-020-00324-8
- Ajoolabady A, Aslkhodapasandhokmabad H, Libby P, et al. Ferritinophagy and ferroptosis in the management of metabolic diseases. Trends Endocrinol Metab. 2021;32(7):444–462. PMID: 34006412. doi:10.1016/j.tem.2021.04.010
- Qin X, Zhang J, Wang B, et al. Ferritinophagy is involved in the zinc oxide nanoparticles-induced ferroptosis of vascular endothelial cells. Autophagy. 2021;17(12):4266–4285. PMID: 33843441. doi:10.1080/15548627.2021.1911016
- Zhang Z, Guo M, Li Y, et al. RNA-binding protein ZFP36/TTP protects against ferroptosis by regulating autophagy signaling pathway in hepatic stellate cells. Autophagy. 2020;16(8):1482–1505. PMID: 31679460. doi:10.1080/15548627.2019.1687985
- Zhang X, Du L, Qiao Y, et al. Ferroptosis is governed by differential regulation of transcription in liver cancer. Redox Biol. 2019;24:101211. PMID: 31108460. doi:10.1016/j.redox.2019.101211
- Yang WS, Stockwell BR. Ferroptosis: death by Lipid Peroxidation. Trends Cell Biol. 2016;26(3):165–176. PMID: 26653790. doi:10.1016/j.tcb.2015.10.014
- Park MW, Cha HW, Kim J, et al. NOX4 promotes ferroptosis of astrocytes by oxidative stress-induced lipid peroxidation via the impairment of mitochondrial metabolism in Alzheimer’s diseases. Redox Biol. 2021;41:101947. PMID: 33774476. doi:10.1016/j.redox.2021.101947
- Liu N, Liang Y, Wei T, et al. The role of ferroptosis mediated by NRF2/ERK-regulated ferritinophagy in CdTe QDs-induced inflammation in macrophage. J Hazard Mater. 2022;436:129043. PMID: 35525219. doi:10.1016/j.jhazmat.2022.129043
- Yi J, Zhu J, Wu J, Thompson CB, Jiang X. Oncogenic activation of PI3K-AKT-mTOR signaling suppresses ferroptosis via SREBP-mediated lipogenesis. Proc Natl Acad Sci U S A. 2020;117(49):31189–31197. PMID: 33229547. doi:10.1073/pnas.2017152117
- Xing G, Meng L, Cao S, et al. PPARα alleviates iron overload-induced ferroptosis in mouse liver. EMBO Rep. 2022;23(8):e52280. PMID: 35703725. doi:10.15252/embr.202052280
- Xu M, Tao J, Yang Y, et al. Ferroptosis involves in intestinal epithelial cell death in ulcerative colitis. Cell Death Dis. 2020;11(2):86. PMID: 32015337. doi:10.1038/s41419-020-2299-1
- Li Y, Cao Y, Xiao J, et al. Inhibitor of apoptosis-stimulating protein of p53 inhibits ferroptosis and alleviates intestinal ischemia/reperfusion-induced acute lung injury. Cell Death Differ. 2020;27(9):2635–2650. PMID: 32203170. doi:10.1038/s41418-020-0528-x
- Yuan S, Wei C, Liu G, et al. Sorafenib attenuates liver fibrosis by triggering hepatic stellate cell ferroptosis via HIF-1α/SLC7A11 pathway. Cell Prolif. 2022;55(1):e13158. PMID: 34811833. doi:10.1111/cpr.13158
- Zhou B, Liu J, Kang R, Klionsky DJ, Kroemer G, Tang D. Ferroptosis is a type of autophagy-dependent cell death. Semin Cancer Biol. 2020;66:89–100. PMID: 30880243. doi:10.1016/j.semcancer.2019.03.002
- Ma H, Wang X, Ha T, et al. MicroRNA-125b prevents cardiac dysfunction in polymicrobial sepsis by targeting TRAF6-mediated nuclear factor κB Activation and p53-mediated apoptotic signaling. J Infect Dis. 2016;214(11):1773–1783. PMID: 27683819. doi:10.1093/infdis/jiw449
- Yu Y, Ou-Yang WX, Zhang H, et al. MiR-125b enhances autophagic flux to improve septic cardiomyopathy via targeting STAT3/HMGB1. Exp Cell Res. 2021;409(2):112842. PMID: 34563514. doi:10.1016/j.yexcr.2021.112842
- Zhao H, Zhang M, Zhou F, et al. Cinnamaldehyde ameliorates LPS-induced cardiac dysfunction via TLR4-NOX4 pathway: the regulation of autophagy and ROS production. J Mol Cell Cardiol. 2016;101:11–24. PMID: 27838370. doi:10.1016/j.yjmcc.2016.10.017
- Xia C, Dong R, Chen C, Wang H, Wang DW. Cardiomyocyte specific expression of Acyl-coA thioesterase 1 attenuates sepsis induced cardiac dysfunction and mortality. Biochem Biophys Res Commun. 2015;468(4):533–540. PMID: 26518651. doi:10.1016/j.bbrc.2015.10.078
- Yao Y, Sun F, Lei M. miR-25 inhibits sepsis-induced cardiomyocyte apoptosis by targetting PTEN. Biosci Rep. 2018;38(2). PMID: 29440462. doi:10.1042/bsr20171511
- Ehrman RR, Sullivan AN, Favot MJ, et al. Pathophysiology, echocardiographic evaluation, biomarker findings, and prognostic implications of septic cardiomyopathy: a review of the literature. Critical Care. 2018;22(1):112. PMID: 29724231. doi:10.1186/s13054-018-2043-8
- Lv X, Wang H. Pathophysiology of sepsis-induced myocardial dysfunction. Mil Med Res. 2016;3:30. PMID: 27708836. doi:10.1186/s40779-016-0099-9
- Pinto AR, Paolicelli R, Salimova E, et al. An abundant tissue macrophage population in the adult murine heart with a distinct alternatively-activated macrophage profile. PLoS One. 2012;7(5):e36814. PMID: 22590615. doi:10.1371/journal.pone.0036814
- Nicolás-ávila JA, Pena-Couso L, Muñoz-Cánoves P, Hidalgo A. Macrophages, metabolism and heterophagy in the heart. Circ Res. 2022;130(3):418–431. PMID: 35113662. doi:10.1161/circresaha.121.319812
- Wang L, Li Y, Wang X, et al. GDF3 protects mice against sepsis-induced cardiac dysfunction and mortality by suppression of macrophage pro-inflammatory phenotype. Cells. 2020;9(1). PMID: 31947892. doi:10.3390/cells9010120
- Zhang J, Wang M, Ye J, et al. The Anti-inflammatory mediator resolvin e1 protects mice against lipopolysaccharide-induced heart injury. Front Pharmacol. 2020;11:203. PMID: 32256344. doi:10.3389/fphar.2020.00203
- Guo L, Shen S, Rowley JW, et al. Platelet MHC class I mediates CD8+ T-cell suppression during sepsis. Blood. 2021;138(5):401–416. PMID: 33895821. doi:10.1182/blood.2020008958
- Guo L, Meng M, Wei Y, et al. Protective Effects of Live Combined B. subtilis and E. faecium in polymicrobial sepsis through modulating activation and transformation of macrophages and mast cells. Front Pharmacol. 2018;9:1506. PMID: 30719003. doi:10.3389/fphar.2018.01506
- Ver Elst KM, Spapen HD, Nguyen DN, Garbar C, Huyghens LP, Gorus FK. Cardiac troponins I and T are biological markers of left ventricular dysfunction in septic shock. Clin Chem. 2000;46(5):650–657. PMID: 10794747. doi:10.1093/clinchem/46.5.650
- Nedeva C. Inflammation and cell death of the innate and adaptive immune system during sepsis. Biomolecules. 2021;11(7). PMID: 34356636. doi:10.3390/biom11071011
- Sun K, Li YY, Jin J. A double-edged sword of immuno-microenvironment in cardiac homeostasis and injury repair. Curr Signal Transduct Ther. 2021;6(1):79. PMID: 33612829. doi:10.1038/s41392-020-00455-6
- van der Poll T, van de Veerdonk FL, Scicluna BP, Netea MG. The immunopathology of sepsis and potential therapeutic targets. Nat Rev Immunol. 2017;17(7):407–420. PMID: 28436424. doi:10.1038/nri.2017.36
- Bai T, Hu X, Zheng Y, Wang S, Kong J, Cai L. Resveratrol protects against lipopolysaccharide-induced cardiac dysfunction by enhancing SERCA2a activity through promoting the phospholamban oligomerization. Am J Physiol Heart Circ Physiol. 2016;311(4):H1051–h62. PMID: 27591219. doi:10.1152/ajpheart.00296.2016
- Hao E, Lang F, Chen Y, et al. Resveratrol alleviates endotoxin-induced myocardial toxicity via the Nrf2 transcription factor. PLoS One. 2013;8(7):e69452. PMID: 23894482. doi:10.1371/journal.pone.0069452
- Shang X, Lin K, Yu R, et al. Resveratrol protects the myocardium in sepsis by activating the phosphatidylinositol 3-kinases (PI3K)/AKT/mammalian target of rapamycin (mtor) pathway and inhibiting the nuclear factor-κB (NF-κB) signaling pathway. Med Sci Monit. 2019;25:9290–9298. PMID: 31806860. doi:10.12659/msm.918369
- Sebai H, Sani M, Aouani E, Ghanem-Boughanmi N. Cardioprotective effect of resveratrol on lipopolysaccharide-induced oxidative stress in rat. Drug Chem Toxicol. 2011;34(2):146–150. PMID: 21314464. doi:10.3109/01480545.2010.494666