Abstract
Background
The implementation of eHealth systems with a trial-and-error approach is very expensive and unsuccessful. So, this study aims to examine the constructs and relationships of the modified technology acceptance model (TAM) to determine whether it can be applied to assess health professional’s behavioral intention to adopt eHealth systems in resource-limited settings or not.
Methods
The institutional-based cross-sectional study design was conducted among a total of 384 healthcare professionals in referral hospitals of Amhara regional state, Ethiopia. Self-administered questionnaire was used to collect the data, and the data were entered using Epi-info version 7 and the descriptive data were analyzed using SPSS version 25. Structural equation modeling, using AMOS 22, was also applied to describe and validate the degree of relationships between variables.
Results
The findings of the structural equation modeling (SEM) indicate that perceived usefulness has a significant influence on attitude (β =0.298, P<0.01) and intention to use eHealth (β =0.387, P<0.01). Perceived ease of use has significant influence on perceived usefulness (β=0.385, P<0.05) and attitude (β=0.347, P<0.05) and intention to use eHealth (β=0.339, P<0.01). Technical infrastructure has significant influence on attitude (β =0.412, P<0.01) and intention to use eHealth (β =0.355, P<0.01). The staffs IT experience has a significant influence on perceived usefulness (β =0.595, P<0.01) and attitude (β =0.267, P<0.05), but the effect of IT experience on the intention to use eHealth was not significant. Among all the constructs, healthcare professionals attitude towards eHealth showed the strongest effect on the intention to use eHealth systems (β = 0.52, P<0.01).
Conclusion
Overall, this model describes 56.2% of the variance in behavioral intention to use eHealth systems. Therefore, the implementers should give priority in enhancing the organizations technical infrastructure, staff’s IT skill, and their attitude towards eHealth by giving continuous support.
Introduction
eHealth, defined as use of information and communications technologies (ICT) in support of health and health-related fields, including health-care services, health surveillance, health literature, and health education knowledge and researchCitation1 has potential benefits in providing and supporting the healthcare service by facilitating information sharing and evidence-based health practice.Citation2,Citation3 Health technology applications, like hospital information system (HIS), electronic health record (EHR), mobile health (M-health), decision support system (DSS), electronic medical record system (EMR) and research/data collection systems (RCS) have a big value in different settings of the healthcare by reducing healthcare costs, advancing access for up-to-date information, enable quick access for patient records and improve communication between patient and healthcare providers.Citation4–Citation7
Over the past decade, the practical implementation and integration of eHealth systems have been scaled drastically. Many developed nations are utilizing eHealth technologies and make a real difference in improving patient care, and the provision of efficient and effective healthcare services.Citation8–Citation11 As the developed nations in the developing world even though the progress and the success rate are not satisfied there are a trial and deployment of eHealth technology in many sectors of the health arena.Citation12–Citation16 Un despised changes in documentation practice, patient alerting, teleconsultation and evidence-based practice were showed and a big emphasis from the government side in deploying systems is also increased with a growing need of identifying crucial constructs for the sustainable adoption of eHealth technologies.Citation14,Citation15
In Ethiopia, several eHealth technologies had been implemented like SmartCare, mobile ENAT messenger, maternal interactive voice record (IVR) and Health management information system (HMIS). District Health Information System (DHIS2): a tool for collection, validation, analysis, and presentation of aggregate and patient-based statistical data, is also in progress to deploy throughout the country.Citation17–Citation19 All the previously deployed systems and the systems that are in progress have been implemented using a trial-and-error approach which is very expensive and unsuccessful.Citation5–Citation7,Citation20 Due to this, the implementation and diffusion of eHealth technology in Ethiopia is still in the embryonic stage.Citation21–Citation24
Developing nations like Ethiopia, with limited resources to implement eHealth technology, needs an empirically validated model that supports to identify the main elements of the system implementation and user’s behavioral intention to use eHealth technologies that can be used by executives for prior preparation.Citation22,Citation23 Numerous empirical studies have been conducted in different domains to explore the confusion on the sustainable adoption of technologies.Citation10,Citation25–Citation31 Technology acceptance model (TAM) is one of the popular and commonly used model to study the social mechanisms of technology adoption, which has been modified from time to time.Citation25,Citation32 Much smaller researches have been conducted to assess whether TAM elements can be applied to eHealth technology adoption and user acceptance.Citation33–Citation36 Some studies showed that the main elements of TAM perceived usefulness, perceived ease of use, and attitude towards eHealth technology have a strong impact on a user’s intention to use eHealth technology.Citation9,Citation35,Citation37,Citation38 Besides the main constructs of TAM other external variables like information sharing, the staff’s IT experience, and technical infrastructure was found positively significant to the intention of eHealth technology.Citation28,Citation39 However, there is still inadequate information on how technologies are adopted and used in Healthcare by health professionals, particularly in a resource-limited context.
This study is therefore intended to fill these gaps by assessing the validity of the modified TAM model and by determining the effect of staff IT experience and technical infrastructure on the original TAM constructs among healthcare professionals in a low-resource setting. The main purpose of this study was to:
Introduce a modified theoretical model constructed based on the Technology acceptance model (TAM).
Empirically test the modified technology acceptance model for determining the key factors influencing the intention of healthcare professionals to adopt eHealth technologies in a resource-limited setting.
Theoretical Background
Technology acceptance model (TAM) is one of the popular models, which helps to model how peoples come to admit and utilize new technologies. The model focuses on factors determining behavioral intention to use new technologies from the end user’s perspective.Citation36,Citation40–Citation42 TAM comprises core variables of user motivation: perceived ease of use, perceived usefulness, and attitudes toward technology. Of these elements, perceived usefulness (PU) and perceived ease of use (PEU) are considered as a principal determinant that directly or indirectly explains the behavioral intention to use (“acceptance”) new technology.Citation29,Citation43–Citation48 In this study, we hypothesized that the constructs and associations described in the modified TAM model are valid to measure the behavioral intention to use eHealth technology by health professionals in low-resource settings. The proposed research variables, their relationships, the research framework, and our hypotheses are explained in .
Perceived Usefulness (PU)
Perceived usefulness refers to “the extent to which an individual believes that applying certain technology will advance job performance”.Citation44 Studies conducted on technology acceptance in different domains have suggested that PU as the main determinant factor for new technology acceptance and use.Citation30,Citation49–Citation51 Users perception on the usefulness of a technology could be affected by external factors.Citation34,Citation50,Citation52,Citation53 With respect to this context, this study tests the following premises:
H1: Perceived usefulness will have a positive effect on user’s attitude towards eHealth.
H2: Perceived usefulness will have a positive effect on intention to use eHealth.
Users Perceived Ease of Use (PEU)
Perceived ease of use is the extent to which a person believes that a particular technology will be effortless and easy to use.Citation54,Citation55 PEU is considered as one of the most important constructs of TAM that helps to predict user’s acceptance or rejection of technologies.Citation56–Citation58 Agreeing with the above findings, we would like to extend the hypotheses by testing the following hypotheses:
H3: Perceived ease of use will have a positive effect on Perceived usefulness of eHealth.
H4: Perceived ease of use will have a positive effect on user’s attitude towards eHealth.
H5: Perceived ease of use will have a positive effect on intention to use eHealth.
Attitude Towards Using eHealth (ATT)
Attitude is a predisposed state of mind regarding the benefits of a system in improving work performance, time management to conduct their work and its effect in improving the quality of the work they do.Citation59 Several studies suggest that attitudes of users are a significant factor in the acceptance and efficiency of the use of technologies in practice.Citation60–Citation63 Findings from different studies show that the Healthcare provider’s attitude and acceptance are vital in the success of eHealth system implementation in the healthcare systems since they are the primary users of the system.Citation20,Citation64–Citation66 With respect to the above findings, this study tests the following hypotheses:
H6: users’ attitude towards e-health will positively influence intention to use eHealth.
Staff IT Experience (ITE)
IT experience deals with health professional’s knowledge of information technology, understanding the basic benefits of technology and their exposure to it, or taking the training.Citation67,Citation68 Healthcare providers with a sound level of IT experience were found interactive with, medical information systems, electronic health records, telehealth solutions, and other up-to-date eHealth applications.Citation65,Citation67–Citation71 Thus, with this background this study tests the following hypotheses:
H7: staff’s IT experience will have a positive effect on user’s Perceived usefulness of eHealth.
H8: staff’s IT experience will have a positive effect on attitude towards eHealth.
H9: staff’s IT experience will have a positive effect on intention to use eHealth.
Technical Infrastructure (TI)
TI refers to a set of information technology (IT) components that are the foundation of an IT service in the organization.Citation72 The technical infrastructure of the organization like the availability of computers to use eHealth systems, the existing infrastructure of the hospital, and the current system that maintains the hospitals existing infrastructure were addressing here. Although technical infrastructure is not one of the TAM constructs, several studies consistently indicate that facilitating conditions influences users’ attitude and intention to use technology.Citation73–Citation79 In this regard, the technical infrastructure is expected as one of the main predictors for the sustainable adoption of eHealth. Therefore, this study tests the following hypotheses:
H10: technical infrastructure will positively influence on attitude towards eHealth.
H11: technical infrastructure will positively influence intention to use eHealth.
Methods
Study Design and Setting
An institutional-based quantitative cross-sectional study design was conducted from April 15 to May 10, 2018, at five referral hospitals (Debre Berhan, Debre Markos, Felege Hiwot, Dessie, and University of Gondar referral Hospitals) of Amhara region, Northern Ethiopia. The hospitals serve more than 30 million population of the region of Amhara and provide referral services for neighborhood regions. All Hospitals had an implementation history of eHealth systems like EMR and e-HMIS, though the systems are functioning in some departments of the hospitals.
Sample Size and Study Participants
All health professionals who were working in the five-referral hospitals of the Amhara region were the source population for this study. The sample size of the study was calculated using a single population proportion formula,Citation80,Citation81 and by considering all the sample size calculations used for Structural Equation Modeling (SEM) analysis.Citation82–Citation85 Then, the final sample size was calculated assuming a 95% of confidence level, 5% margin of error, and 10% of non-response rate, which resulted in a participant of 423. Staff who were on annual and sick leave were excluded from the study, and the participants were selected using a simple random sampling technique. Two data collectors were assigned to each hospital.
Instrument Development and Validation
A structured questionnaire was adapted by reviewing several works of literature conducted on the area.Citation28,Citation48,Citation50,Citation79,Citation86 The structured questionnaire has two categories, the first category encompasses sociodemographic-related questions and the second part contained elements related to TAM constructs (PU, PEU, ATT, and BI) and additional elements ITE and TI with 24 Likert scale items. The questioner was a self-administered questioner and the respondents filled it. The questioner was developed to test the hypotheses. The pretest of the questioner was conducted at another hospital which is other than the study hospitals. Based on the feedback from the respondents, the questions had modified with their wording by language experts. Reliability tests were checked using the Cronbach alpha coefficient (Cα), composite reliability (CR), and standard loading (SD) using structural equation modeling. The results of the three tests demonstrated scores for all the items over the criterion as shown in . Thus, the indicators measuring the constructs in the present study all carried sufficient item reliability, as shown in the following .
Table 1 Evaluation of Reliability Measurement
Data Management and Analysis
Data from respondents were entered using Epi-info version 7 and exported into SPSS version 25 for descriptive data analysis. The extent of the relationship between variables was clarified using AMOS 22 structural equation modeling (SEM). For this reason, SEM was used to test the hypotheses since it is a dominant statistical method that measures and clarifies the degree of relationships between variables. The model’s overall goodness of fit was also measured and assessed based on standards from previous studiesCitation87,Citation88 using Chi-square ratio (<3), normal fit index (NFI > 0.9), the goodness of fit index (GFI >0.9), adjusted goodness of fit index (AGFI > 0.8), and root mean square of standardized residual (RMSR <0.08).
Ethics Approval and Consent to Participate
This study was conducted in accordance with the Declaration of Helsinki. Ethical clearance was obtained from the Ethical review board of Institute of Public Health, University of Gondar. Supporting letter was also obtained from Amhara regional state Public Health Research Institute and respective referral hospitals. The objectives and the purpose of the study were presented for the participants at the front page of the questioner. An informed written consent was accepted for this particular study from the study participants. Each study participant had the right of withdrawal from the study at any time during data collection. Due attention was given to not personalize any of the response of the respondents during data presentation, analysis and interpretation.
Result
A total of 423 study subjects were included in the study, 384 (response rate 91%) of them gave their consent, and responded to the questions. From the total of (n=384) respondents, 244 (63.5%) of them were males, and more than half of the respondents 212 (55.2%) had less than or equal to 5 years of work experience, and more than half of the 214 (55.7%) had taken basic computer courses. In addition, the mean age of the respondents was 29.9 years with a standard deviation of ±6.17 years as shown in .
Table 2 Sociodemographic Characteristics of Healthcare Providers in Amhara Regional State Referral Hospitals, Ethiopia (n=384)
Validation of the Revised TAM Constructs
The constructs were tested by examining the structural models and are summarized in , in which the t-statistics and standardized path coefficients (β) are presented. The relationships between the dependent and independent variables are also shown. All hypotheses proposed in this study were found significant, except for H9.
Table 3 Results of Structural Equation Modeling in AMOS with the Path Coefficients for All of the Nine Hypotheses
The analysis showed perceived usefulness has a significant effect on the intention to use eHealth (β =0.387, P<0.01) and attitude towards eHealth (β =0.298, P<0.01); the organization’s technical infrastructure has a significant effect on healthcare providers intention to use eHealth (β =0.355, P<0.01) and their attitude towards eHealth (β =0.412, P<0.01); healthcare providers experience on IT has a strong effect on perceived usefulness (β =0.595, P<0.01) and their attitude towards eHealth (β =0.267, P<0.05); the user’s perceived ease of use about the system has a significant effect on perceived usefulness (β =0.385, P<0.05), attitude towards eHealth (β =0.347, P <0.01) and behavioral intention to use eHealth (β =0.339, P<0.05), attitude towards eHealth is also found with a strong effect on the intention to use eHealth (β =0.526, P<0.01).
Perceived usefulness and technical infrastructure exhibited a stronger effect than perceived ease of use on user’s attitude and behavioral intention to use eHealth, which implies Perceived Usefulness and Technical Infrastructure are a crucial factor in developing nations’ eHealth systems adoption. And Attitude takes the lead in determining people’s behavioral intention to use eHealth systems. In terms of goodness of fit indicators, Perceived usefulness, Perceived ease of use, IT experience, and Technical infrastructure accounted for 53.3% and 46.7% of the variance in attitude and BI to use eHealth, respectively, with the exception of IT experience for the second scenario. An Individual’s attitude to use eHealth accounted for 44.3% of the variance in Behavioral intention to use eHealth. Generally, the structural model results have elucidated that 56.2% of the variance in behavioral intention to use eHealth systems was explained by these measures.
Discussion
This study was conducted to empirically validate the generalizability of the modified technology acceptance model (TAM) by assessing the constructs of the model in the context of Resource-limited setting hospitals. Additionally, insights were provided into future eHealth implementation success research by assessing the effect of additional factors (staff’s IT experience and technical infrastructure of the organization) on the relationship between intention to use and attitude towards eHealth systems. For sustainable adoption of eHealth systems in resource-limited settings, the healthcare providers IT experience and the organizations technical infrastructure were found crucial.
All the hypotheses were checked ether they had a relationship with the outcome variable or not. All constructs, except the effect of the staff’s IT experience with the intention to use eHealth (), which tests the relationship between the independent variables and the outcome variable were found with a strong positive association. Relationships of perceived usefulness, organization’s technical infrastructure, perceived ease of use, user attitude towards eHealth, and intention to use eHealth were proven to possess adequate psychometric properties and thus can be used as effective measures of eHealth adoption in resource-limited settings. Separate relationships and their implication for behavioral intention to use eHealth are explained below.
Figure 2 Results of the structural model after a considerable modification of the original TAM model. Notes: *p < 0.05, **P < 0.01.
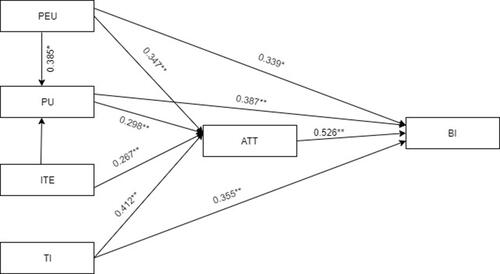
Perceived usefulness directly affects attitude (β = 0.298, P = 0.00) and intention (β = 0.387, P = 0.00) to use eHealth (H1 and H2), these shows that an increase in health professionals’ perceived usefulness leads to an increase in attitude and intention to use eHealth. The probable reason for this could be hence perceived usefulness incorporates effectiveness, ease of use, productivity and time efficiency on the systems, the care provider’s intention and attitude towards eHealth will be increased. Therefore, a net positive effect from perceived usefulness will result in a positive effect in attitude towards eHealth and intention to use eHealth. This result is consistent with other studies.Citation14,Citation43,Citation44,Citation49
Perceived ease of use also endorses a positive impact on perceived usefulness (β = 0.385, P = 0.02), attitude (β = 0.347, P = 0.00), and intention to use eHealth (β = 0.339, P = 0.02) (H3, H4, and H5). When a person believes using eHealth system would be free of effort: their attitude and intention to use eHealth will be enhanced.Citation48,Citation76,Citation89 Perceived ease of use had both a direct and indirect effect on the intention to use eHealth next to the perceived usefulness construct. This finding is consistent with other studies conducted in different countries.Citation43,Citation90 This might be due to individual’s attitude, perceived usefulness and intention to use eHealth systems is highly influenced by the effort used to manipulate the system. If the system is expected with a less effort to manipulate people’s intention to use eHealth systems will be enhanced. Therefore, while implementing eHealth technologies, the system should be easy to understand and operate by healthcare providers for the sustainable adoption of the systems in the future.
Staff’s attitude towards eHealth technologies positively affects their intention to use eHealth systems (β = 0.526, P = 0.00), (H9). As healthcare professionals thought eHealth technologies, as a tool to enhance their work performance and the quality of the work they do, their intention to use eHealth also increase. The result is found consistent with other studies.Citation20,Citation91 The possible reason for this could be the healthcare providers with positive settled way of thinking or feeling about eHealth systems will be highly angered to new eHealth systems. Therefore, activities that boost attitude like computer availability at the workplace, continuous training and support, and knowledge sharing on eHealth technologies should be given a big emphasis.
This study also shows that healthcare professionals’ IT experience strongly influences Perceived usefulness (β = 0.595, P = 0.00), and their attitude towards eHealth (β = 0.267, P = 0.02), technologies (H7 and H8). As the staffs have IT experience, having IT knowledge, and getting expertise in hospitals to train them on IT systems their perception of the usefulness of new systems and their attitude towards eHealth will be increased. The result is also found consistent with other studies.Citation67–Citation70,Citation92 However, the result shows an insignificant association between IT experience and intention to use eHealth (β = 0.062, P = 0.23) (H9). The possible reason for this could be staff with previous IT experience may know the challenges to use eHealth technologies in low-resource settings with interrupted power supply, limited computer access, and a high burden of care providers due to high patient flow. Therefore, before and after the implementation of eHealth systems capacity building of staff in IT is crucial for the sustainable adoption of eHealth technologies in the future. Additionally, it is necessary to provide more computers within the wards to practice and teach themselves without having to wait free computers.
The finding of this study also indicates that the organization’s technical infrastructure strongly influences the staff’s attitude (β = 0.412, P = 0.00) and intention to use eHealth technologies (β = 0.355, P = 0.00) (H10 and H11). The result of this study is also found consistent with other similar studies.Citation14,Citation44,Citation73,Citation93 The probable reason for this could be as the existing infrastructure, the system in place to maintain the hospitals’ existing infrastructure increases the staff’s attitude, and intention to use eHealth technologies will increase in the same direction. Therefore, ensuring sustainable power supply, availability of computers, allocating budgets are important for attitude and intention to use eHealth technologies in resource-limited setting hospitals.
Conclusion
This study revealed that the advanced technology acceptance model (TAM) to be applicable to assess the behavioral intention to use eHealth for the sustainable adoption of eHealth technologies. Attitude towards eHealth was found to be the strongest determinant factor for the intention to use eHealth. Perceived usefulness and Perceived ease of use were also found an important determinant factor for attitude towards eHealth and intention to use eHealth. Additionally, Technical Infrastructure was also found to be a predicting factor in attitude and intention to use eHealth in Resource-limited settings. Consequently, eHealth implementers and managers in those settings should give priority in improving the hospital’s technical infrastructure by providing continuous basic ICT training to health professionals; and give attention to the system they want to implement; hence, those actions will enhance the perceived usefulness and attitude of the staffs indirectly.
Strength and Limitation of the Study
This study will have a significant contribution for the future adoption of eHealth systems in low resource settings. Moreover, the discussed findings were obtained from multi-center study from different eHealth systems in the country that could be generalized to other populations and newly emerged platforms. As a cross-sectional survey, there would be a social desirability bias that is inevitable in a cross-sectional study.
Abbreviations
ATT, attitude; BI, behavioral intention; DHIS, District Health Information System; DSS, decision support system; EMR, electronic medical record; HIS, hospital information system; HMIS, Health Management Information System; ICT, information and communications technologies; ITE, information technology experience; IVR, interactive voice record; PEU, perceived ease of use; PU, perceived usefulness; SEM, structural equation modelling; TAM, technology acceptance model; TI, technical infrastructure.
Data Sharing Statement
Data will be available upon request from the correspondence author.
Author Contributions
All authors contributed to study design, data analysis, drafting and revising the article, gave final approval of the version to be published, and have agreed on the journal to which the article has been submitted; and agree to be accountable for all aspects of the work.
Acknowledgment
We would like to acknowledge the data collectors and the study participants.
Disclosure
The authors report no conflicts of interest for this work.
References
- Shaw T, McGregor D, Brunner M, Keep M, Janssen A, Barnet S. What is eHealth (6)? Development of a conceptual model for ehealth: qualitative study with key informants. J Med Internet Res. 2017;19(10):e324. doi:10.2196/jmir.8106
- Rinaldi G, Editor. New Perspectives in Medical Records: Meeting the Needs of Patients and Practitioners. Springer; 2017; 129–140. doi:10.1007/978-3-319-28661-7
- Anwar S, Prasad R. Framework for future telemedicine planning and infrastructure using 5G technology. Wirel Pers Commun. 2018;100(1):193–208. doi:10.1007/s11277-018-5622-8
- Awol SM, Birhanu AY, Mekonnen ZA, et al. Health professionals’ readiness and its associated factors to implement electronic medical record system in four selected primary hospitals in Ethiopia. Adv Med Educ Pract. 2020;11:147–154. doi:10.2147/amep.s233368
- Mekonnen ZA, Tilahun B, Alemu K, Were M. Effect of mobile phone text message reminders on improving completeness and timeliness of routine childhood vaccinations in North-West, Ethiopia: a study protocol for randomised controlled trial. BMJ Open. 2019;9(11):1–7. doi:10.1136/bmjopen-2019-031254
- Gashu KD, Gelaye KA, Mekonnen ZA, Lester R, Tilahun B. Does phone messaging improves tuberculosis treatment success? A systematic review and meta-analysis. BMC Infect Dis. 2020;20(1):42. doi:10.1186/s12879-020-4765-x
- Abiy R, Gashu K, Asemaw T, et al. A comparison of electronic records to paper records in antiretroviral therapy clinic in Ethiopia: what is affecting the quality of the data? Online J Public Health Inform. 2018;10(2). doi:10.5210/ojphi.v10i2.8309
- Melchiorre MG, Papa R, Rijken M, van Ginneken E, Hujala A, Barbabella F. eHealth in integrated care programs for people with multimorbidity in Europe: insights from the ICARE4EU project. Health Policy (New York). 2018;122(1):53–63. doi:10.1016/j.healthpol.2017.08.006
- Faber S, Geenhuizen MV, Reuver MD. Adoption factors in medical hospitals: a focus on the Netherlands. Int J Med Inform. 2017;100:77–89. doi:10.1016/j.ijmedinf.2017.01.009
- Torrent-Sellens J, Díaz-Chao Á, Soler-Ramos I, Saigí-Rubió F. Modelling and predicting eHealth usage in Europe: a multidimensional approach from an online survey of 13,000 European Union internet users. J Med Internet Res. 2016;18(7):1–19. doi:10.2196/jmir.5605
- Kazi DS. From Innovation to Implementation. J Am Coll Cardiol. 2014;64(24):2616–2618. doi:10.1016/j.jacc.2014.10.008
- Rjdoh H, Hrq D, Udvpxv R, et al. Hospitals’ readiness to implement sustainable smartcare systems in Addis Ababa, Ethiopia. Technol Manag World Intell Syst. 2019.
- Shiferaw W, Kifle M, Okuboyejo S, Mbarika V, Data Exchange A. Interoperability framework for ehealth applications in Ethiopia. Asian J Comput Inf Syst. 2018;06(02):8–13.
- Tao D, Wang TT, Wang TT, et al. A mobile health model supporting Ethiopia’s eHealth strategy. Digit Med. 2016;1(1):76–85. doi:10.4103/digm.digm
- Barkman C, Weinehall L. Policymakers and mHealth: roles and expectations, with observations from Ethiopia, Ghana and Sweden. Glob Health Action. 2017;10(3):1337356. doi:10.1080/16549716.2017.1337356
- Fanta GB, Pretorius L, Erasmus L. Organizational dynamics of sustainable eHealth implementation: a case study of eHMIS. PICMET 2017 - Portl Int Conf Manag Eng Technol Technol Manag Interconnected World, Proc. Portland, OR; 2017:1–9. doi:10.23919/PICMET.2017.8125472
- Kuyo RO, Muiruri L, Njuguna S. Organizational factors influencing the adoption of the district health information system 2 in Uasin Gishu County, Kenya. Int J Med Res Health Sci. 2018.
- Etamesor S, Ottih C, Salihu IN, Okpani AI. Data for decision making: using a dashboard to strengthen routine immunisation in Nigeria. BMJ Glob Health. 2018;3(5):e000807. doi:10.1136/bmjgh-2018-000807
- Open Health News. DHIS2 (District Health Information System 2). Open Health News. 2018.
- Tilahun B, Fritz F. Modeling antecedents of electronic medical record system implementation success in low-resource setting hospitals. BMC Med Inform Decis Mak. 2015;1–9. doi:10.1186/s12911-015-0192-0
- Perez-Chavolla LJ, Thouvenot VI, Schimpf D, Moritz A. Adopting digital technology in midwifery practice – experiences and perspectives from six projects in eight countries (2014 – 2016). J Int Soc Telemed eHealth. 2019;7:1–8. doi:10.29086/jisfteh.7.e2
- Syzdykova A, Zolfo M, Malta A, Diro E, Oliveira JL. Customization of openMRS for Leishmaniasis Research and Treatment Center in Ethiopia. J Int Soc Telemed eHealth. 2017;5:65.
- Gebre-Mariam M. Governance lessons from an interorganizational health information system implementation in Ethiopia. Electron J Inf Syst Dev Countries. 2018;84(5):1–16. doi:10.1002/isd2.12045
- Cunningham PM, Cunningham M, Van Greunen D, et al. Implications of baseline study findings from rural and deep rural clinics in Ethiopia, Kenya, Malawi and South Africa for the co-design of mHealth4Afrika. GHTC 2016 - IEEE Glob Humanit Technol Conf Technol Benefit Humanit Conf Proc. Seattle, WA, USA; 2016:666–674. doi:10.1109/GHTC.2016.7857350
- Lai P. The literature review of technology adoption models and theories for the novelty technology. J Inf Syst Technol Manag. 2017;14(1):21–38. doi:10.4301/s1807-17752017000100002
- Al-Emran M, Mezhuyev V, Kamaludin A. Technology acceptance model in M-learning context: a systematic review. Comput Educ. 2018;125:389–412. doi:10.1016/j.compedu.2018.06.008
- Gharaibeh MK, Arshad MRM, Gharaibh NK. Using the UTAUT2 model to determine factors affecting adoption of mobile banking services: a qualitative approach. Int J Interact Mob Technol. 2018;12(4):123–134. doi:10.3991/ijim.v12i4.8525
- Idoga PE, Toycan M, Nadiri H, Çelebi E. Factors affecting the successful adoption of e-Health cloud based health system from healthcare consumers’ perspective. IEEE Access. 2018;6(c):71216–71228. doi:10.1109/ACCESS.2018.2881489
- Scherer R, Siddiq F, Tondeur J. The technology acceptance model (TAM): a meta-analytic structural equation modeling approach to explaining teachers’ adoption of digital technology in education. Comput Educ. 2019;128:13–35. doi:10.1016/j.compedu.2018.09.009
- Ching-Ter C, Hajiyev J, Su CR. Examining the students’ behavioral intention to use e-learning in Azerbaijan? The general extended technology acceptance model for E-learning approach. Comput Educ. 2017;111:128–143. doi:10.1016/j.compedu.2017.04.010
- Mensah IK. Impact of government capacity and E-government performance on the adoption of E-government services. Int J Public Adm. 2020;43(4):303–311. doi:10.1080/01900692.2019.1628059
- Taherdoost H. A review of technology acceptance and adoption models and theories. Procedia Manuf. 2018;22:960–967. doi:10.1016/j.promfg.2018.03.137
- Suresh V, Prabhakar K, Santhanalakshmi K, Maran K. Applying technology acceptance (TAM) model to determine the factors of acceptance in out-patient information system in private hospital sectors in Chennai city. J Pharm Sci Res. 2016;8(12):1373–1377.
- Nasir S, Yurder Y. Consumers’ and physicians’ perceptions about high tech wearable health products. Procedia - Soc Behav Sci. 2015;195:1261–1267. doi:10.1016/j.sbspro.2015.06.279
- Melas CD, Zampetakis LA, Dimopoulou A, Moustakis V. Modeling the acceptance of clinical information systems among hospital medical staff: an extended TAM model. J Biomed Inform. 2011;44(4):553–564. doi:10.1016/j.jbi.2011.01.009
- Becker D. Acceptance of mobile mental health treatment applications. Procedia Comput Sci. 2016;58(Icth):220–227. doi:10.1016/j.procs.2016.09.036
- Khan I, Xitong G, Ahmad Z, Shahzad F. Investigating factors impelling the adoption of e-Health: a perspective of African expats in China. SAGE Open. 2019;9(3):215824401986580. doi:10.1177/2158244019865803
- Briz-Ponce L, García-Peñalvo FJ. An empirical assessment of a technology acceptance model for apps in medical education. J Med Syst. 2015;39(11). doi:10.1007/s10916-015-0352-x
- Zayyad MA, Toycan M. Factors affecting sustainable adoption of e-health technology in developing countries: an exploratory survey of Nigerian hospitals from the perspective of healthcare professionals. PeerJ – J Life Environ Sci. 2018. doi:10.7717/peerj.4436
- Wu B, Zhang C. Empirical study on continuance intentions towards E-Learning 2.0 systems. Behav Inf Technol. 2014;33(10):1027–1038. doi:10.1080/0144929X.2014.934291
- Tarhini A, Hone K, Liu X. The effects of individual differences on e-learning users’ behaviour in developing countries: a structural equation model. Comput Human Behav. 2014;41:153–163. doi:10.1016/j.chb.2014.09.020
- Hsieh TC, Chen SL, Hung MC. Longitudinal test of ePortfolio continuous use: an empirical study on the change of students beliefs. Behav Inf Technol. 2015;34(8):838–853. doi:10.1080/0144929X.2014.907344
- Abdullah F, Ward R, Ahmed E. Investigating the influence of the most commonly used external variables of TAM on students’ Perceived Ease of Use (PEOU) and Perceived Usefulness (PU) of e-portfolios. Comput Human Behav. 2016;63:75–90. doi:10.1016/j.chb.2016.05.014
- Marangunić N, Granić A. Technology acceptance model: a literature review from 1986 to 2013. Univ Access Inf Soc. 2015;14(1):81–95. doi:10.1007/s10209-014-0348-1
- Durodolu OO. Technology acceptance model as a predictor of using information system’ to acquire information literacy skills. Libr Philos Pract. 2016.
- Kaipio J, Lääveri T, Hyppönen H, et al. Usability Problems Do Not Heal by Themselves: National Survey on Physicians’ Experiences with EHRs in Finland. Elsevier Ireland Ltd; 2017.
- Li J, Seale H, Ray P, et al. E-Health preparedness assessment in the context of an influenza pandemic: a qualitative study in China. BMJ Open. 2013;3(3):1–9. doi:10.1136/bmjopen-2012-002293
- Tubaishat A. Perceived usefulness and perceived ease of use of electronic health records among nurses: application of technology acceptance model. Informatics Health Soc Care. 2018;43(4):379–389. doi:10.1080/17538157.2017.1363761
- Khatun F, Heywood AE, Ray PK, Hanifi SMA, Bhuiya A, Liaw ST. Determinants of readiness to adopt mHealth in a rural community of Bangladesh. Int J Med Inform. 2015;84(10):847–856. doi:10.1016/j.ijmedinf.2015.06.008
- Rahimi B, Nadri H, Afshar HL, Timpka T. A systematic review of the technology acceptance model in health informatics. Appl Clin Inform. 2018;9(3):604–634. doi:10.1055/s-0038-1668091
- Mortenson MJ, Vidgen R. A computational literature review of the technology acceptance model. Int J Inf Manage. 2016;36(6):1248–1259. doi:10.1016/j.ijinfomgt.2016.07.007
- Ortega Egea JM, Román González MV. Explaining physicians’ acceptance of EHCR systems: an extension of TAM with trust and risk factors. Comput Human Behav. 2011;27(1):319–332. doi:10.1016/j.chb.2010.08.010
- Yarbrough AK, Smith TB. Technology acceptance among physicians. Med Care Res Rev. 2007;64(6):650–672. doi:10.1177/1077558707305942
- Davis FD. Perceived usefulness, perceived ease of use, and user acceptance of information technology. MIS Q Manag Inf Syst. 1989;13(3):319. doi:10.2307/249008
- Davis F. Perceived usefulness, perceived ease of use, and user acceptance of information technology. MIS Q. 1989;13(3):319. doi:10.2307/249008
- Hess TJ, McNab AL, Basoglu KA. Reliability generalization of perceived ease of use, perceived usefulness, and behavioral intentions. MIS Q Manag Inf Syst. 2014;38(1):1–28. doi:10.25300/MISQ/2014/38.1.01
- Schnall R, Higgins T, Brown W, Carballo-Dieguez A, Bakken S. Trust, perceived risk, perceived ease of use and perceived usefulness as factors related to mhealth technology use. Stud Health Technol Inform. 2015. doi:10.3233/978-1-61499-564-7-467
- Sánchez RA, Hueros AD. Motivational factors that influence the acceptance of Moodle using TAM. Comput Human Behav. 2010;26(6):1632–1640. doi:10.1016/j.chb.2010.06.011
- Petty RE. Attitude change. In Encyclopedia of Human Behavior. Second. The Ohio State University; 2012; doi:10.1016/B978-0-12-375000-6.00040-9
- Ward R, Stevens C, Brentnall P, Briddon J. The attitudes of health care staff to information technology: a comprehensive review of the research literature. Health Info Libr J. 2008;25(2):81–97. doi:10.1111/j.1471-1842.2008.00777.x
- Nazi KM. The personal health record paradox: health care professionals’ perspectives and the information ecology of personal health record systems in organizational and clinical settings. J Med Internet Res. 2013;15(4):e70. doi:10.2196/jmir.2443
- Simpao AF, Ahumada LM, Gálvez JA, Rehman MA. A review of analytics and clinical informatics in health care. J Med Syst. 2014;38(4). doi:10.1007/s10916-014-0045-x
- Angst CM, Agarwal R. Adoption of electronic health records in the presence of privacy concerns: the elaboration likelihood model and individual persuasion. MIS Q Manag Inf Syst. 2009;33(2):339. doi:10.2307/20650295
- Biruk S, Yilma T, Andualem M, Tilahun B. Health professionals’ readiness to implement electronic medical record system at three hospitals in Ethiopia: a cross sectional study. BMC Med Inform Decis Mak. 2014;14(1). doi:10.1186/s12911-014-0115-5
- Yehualashet G, Andualem M, Tilahun B. The attitude towards and use of electronic medical record system by health professionals at a referral hospital in Northern Ethiopia: cross-sectional study. J Heal Inf Afr. 2015. doi:10.12856/JHIA-2015-v3-i1-124
- Abebe F. Assessment of knowledge and utilization of the partograph among health professionals in Amhara Region, Ethiopia. Sci J Clin Med. 2013;2(2):26. doi:10.11648/j.sjcm.20130202.11
- Gürdaş Topkaya S, Kaya N. Nurses’ computer literacy and attitudes towards the use of computers in health care. Int J Nurs Pract. 2015;21:141–149. doi:10.1111/ijn.12350
- Asah F. Computer usage among nurses in rural health-care facilities in South Africa: obstacles and challenges. J Nurs Manag. 2013;21(3):499–510. doi:10.1111/j.1365-2834.2011.01315.x
- Sukums F, Mensah N, Mpembeni R, Kaltschmidt J, Haefeli WE, Blank A. Health workers’ knowledge of and attitudes towards computer applications in rural African health facilities. Glob Health Action. 2014;7(1):24534. doi:10.3402/gha.v7.24534
- Alquraini H, Alhashem AM, Shah MA, Chowdhury RI. Factors influencing nurses’ attitudes towards the use of computerized health information systems in Kuwaiti hospitals. J Adv Nurs. 2007;57(4):375–381. doi:10.1111/j.1365-2648.2007.04113.x
- Koivunen M, Hätönen H, Välimäki M. Barriers and facilitators influencing the implementation of an interactive Internet-portal application for patient education in psychiatric hospitals. Patient Educ Couns. 2008;70(3):412–419. doi:10.1016/j.pec.2007.11.002
- Venkatesh V, Morris M, Davis G, Davis F. Technology acceptance model - research. MIS Q. 2003.
- Wang Y, Xiao Q, Sun L, Wu Y. Chinese nurses’ acceptance of PDA: a cross-sectional survey using a technology acceptance model. Stud Health Technol Inform. 2016;225:889–890..
- Nguyen TN Institutional perspectives on implementing health information systems in developing countries: the case of electronic medical records (EMR) for children health in Vietnam. In: 16th Americas Conference on Information Systems 2010, AMCIS 2010; Lima, Peru 410; 2010. Available from: https://aisel.aisnet.org/amcis2010/410.
- Sharifian R, Askarian F, Nematolahi M, Farhadi P. Factors influencing nurses’ acceptance of hospital information systems in Iran: application of the unified theory of acceptance and use of technology. Heal Inf Manag J. 2014. doi:10.1177/183335831404300303
- Kamalzadeh Takhti H, Abdul Rahman AB, Abedini S. Factors determining nurses hospital information system usage. Int J Manag Inf Technol. 2013. doi:10.24297/ijmit.v3i3.1721
- Bhattacharya I, Ramachandran A. A path analysis study of retention of healthcare professionals in urban India using health information technology. Hum Resour Health. 2015;13(1). doi:10.1186/s12960-015-0055-x
- Tilahun B, Fritz F. Comprehensive evaluation of electronic medical record system use and user satisfaction at five low-resource setting hospitals in Ethiopia. JMIR Med Inform. 2015;3(2):e22. doi:10.2196/medinform.4106
- Idoga PE, Toycan M, Nadiri H, Çelebi E. Assessing factors militating against the acceptance and successful implementation of a cloud based health center from the healthcare professionals’ perspective: a survey of hospitals in Benue state, northcentral Nigeria. BMC Med Inform Decis Mak. 2019;19(1):1–18. doi:10.1186/s12911-019-0751-x
- Ross SM. Introductory Statistics. Academic Press; 2017. doi:10.1016/b978-0-12-804317-2.00031-x
- Wilson Van Voorhis CR, Morgan BL. Understanding power and rules of thumb for determining sample sizes. Tutor Quant Methods Psychol. 2007;3(2):43–50. doi:10.20982/tqmp.03.2.p043
- Wolf EJ, Harrington KM, Clark SL, Miller MW. Sample size requirements for structural equation models. Educ Psychol Meas. 2013;73(6):913–934. doi:10.1177/0013164413495237
- Hox JJ, Maas CJM, Brinkhuis MJS. The effect of estimation method and sample size in multilevel structural equation modeling. Stat Neerl. 2010;64(2):157–170. doi:10.1111/j.1467-9574.2009.00445.x
- Fan X, Thompson B, Wang L. Effects of sample size, estimation methods, and model specification on structural equation modeling fit indexes. Struct Equ Model. 1999;6(1):56–83. doi:10.1080/10705519909540119
- Wang J, Wang X. Structural Equation Modeling: Applications Using Mplus. John Wiley & Sons; 2012. doi:10.1002/9781118356258
- Villalba-Mora E, Casas I, Lupiañez-Villanueva F, Maghiros I. Adoption of health information technologies by physicians for clinical practice: the Andalusian case. Int J Med Inform. 2015;84(7):477–485. doi:10.1016/j.ijmedinf.2015.03.002
- Urbach N, Müller B. The Updated DeLone and McLean Model of Information Systems Success. New York, NY: Springer; 2012. doi:10.1007/978-1-4419-6108-2_1
- Fox J. Chapter 3: partial least squares path analysis. Struct Equ Model. 2002;(January):1–20. doi:10.1002/env.1000
- Hoque MR, Bao Y, Sorwar G. Investigating factors influencing the adoption of e-Health in developing countries: a patient’s perspective. Informatics Health Soc Care. 2017;42(1):1–17. doi:10.3109/17538157.2015.1075541
- Wai ISH, Ng SSY, Chiu DKW, Ho KKW, Lo P. Exploring undergraduate students’ usage pattern of mobile apps for education. J Librariansh Inf Sci. 2018;50(1):34–47. doi:10.1177/0961000616662699
- Kipturgo MK, Kivuti-Bitok LW, Karani AK, Muiva MM. Attitudes of nursing staff towards computerisation: a case of two hospitals in Nairobi, Kenya. BMC Med Inform Decis Mak. 2014;14. doi:10.1186/1472-6947-14-35.
- Huryk LA. Factors influencing nurses’ attitudes towards healthcare information technology. J Nurs Manag. 2010;18(5):606–612. doi:10.1111/j.1365-2834.2010.01084.x
- Sharifian R, Askarian F, Nematolahi M, Farhadi P. Factors influencing nurses’ acceptance of hospital information systems in Iran: application of the unified theory of acceptance and use of technology. Heal Inf Manag J. 2014. doi:10.12826/18333575.2014.0001.Sharifian