Abstract
Purpose
To explore the role of the mean apnea–hypopnea duration (MAD) and apnea–hypopnea duration per hour (HAD) in hypoxemia and evaluate whether they can effectively predict the occurrence of hypoxemia among adults with OSA.
Patients and Methods
A total of 144 participants underwent basic information gathering and polysomnography (PSG). Logistic regression models were conducted to evaluate the best index in terms of hypoxemia. To construct the prediction model for hypoxemia, we randomly divided the participants into the training set (70%) and the validation set (30%).
Results
The participants with hypoxemia tend to have higher levels of obesity, diabetes, AHI, MAD, and HAD compared with non-hypoxemia. The most relevant indicator of blood oxygen concentration is HAD (r = 0.73) among HAD, MAD, and apnea–hypopnea index (AHI). The fitness of HAD on hypoxemia showed the best. In the stage of establishing the prediction model, the area under the curve (AUC) values of both the training set and the validation set are 0.95. The increased HAD would elevate the risk of hypoxemia [odds ratio (OR): 1.30, 95% confidence interval (CI): 1.13–1.49].
Conclusion
The potential role of HAD in predicting hypoxemia underscores the significance of leveraging comprehensive measures of respiratory disturbances during sleep to enhance the clinical management and prognostication of individuals with sleep-related breathing disorders.
Introduction
Hypoxemia, characterized by an inadequate level of oxygen in the arterial blood, represents a significant physiological disturbance with far-reaching implications for individual health.Citation1 Inadequate tissue oxygenation can result in the occurrence and development of various diseases, such as cognitive impairment,Citation2 cardiac impairment,Citation3,Citation4 pulmonary hypertension,Citation5 and compromised immune function,Citation6 thereby increasing the risk of morbidity and mortality. It is noteworthy that hypoxemia is now being recognized as a potentially major factor contributing to the pathogenesis of obstructive sleep apnea (OSA)-related comorbidities.Citation7 Furthermore, the hypoxemia associated with OSA is gaining recognition as a significant factor that fosters the development and progression of cancer.Citation8 Therefore, early identification and intervention for hypoxemia is imperative in mitigating its adverse effects and preventing the occurrence of severe comorbidities, especially for patients with OSA.
The Apnea–Hypopnea Index (AHI) serves as a crucial metric in the assessment of sleep-disordered breathing, encompassing both apneas (complete cessation of airflow) and hypopneas (partial airflow reduction) during sleep. AHI has been widely utilized as a diagnostic tool to quantify the severity of OSA.Citation9 However, as a diagnostic criterion, the AHI reflects only a portion of the pathophysiological characteristics of OSA,Citation10,Citation11 it cannot reflect the degrees of hypoxemia and sleep fragmentation or the duration and distribution of respiratory events.Citation12,Citation13 Furthermore, AHI’s reliance on quantifying respiratory events without direct consideration of oxygen saturation dynamics, intermittent hypoxemia, or related physiological responses presents a notable drawback in the comprehensive assessment of sleep-related hypoxemia. This insufficiency underscores the need for complementary metrics and assessment tools to more accurately predict and evaluate the risk of hypoxemia during sleep, thereby enhancing the clinical management and prognostication of individuals with sleep-disordered breathing.
With the gradual prolongation of apnea and hypopnea event durations, oxygen reserves are gradually depleted, causing a series of effects on the body, such as sleep fragmentation, cardiac dysfunction, hypertension, endothelial impairment, atrial fibrillation, and even sudden cardiac death.Citation7,Citation14–16 These suggested that the duration of adverse respiratory events may be closely related to the occurrence of hypoxemia. Therefore, the mean apnea–hypopnea duration (MAD) and apnea–hypopnea duration per hour (HAD), which comprised the combination of apnea and hypopnea event durations according to polysomnography (PSG) data could play an important role in predicting hypoxemia. However, far too little attention has been paid to MAD and HAD in identifying hypoxemia among patients with OSA.
The present study was conducted to clarify the important impact of MAD and HAD on hypoxemia and evaluate whether they can effectively predict the occurrence of hypoxemia among adults with OSA.
Materials and Methods
Sample Characteristics
We screened patients with OSA over the age of 18 admitted to the respiratory and sleep centre of the Second Affiliated Hospital of Anhui Medical University from September 2018 to January 2020. Questionnaires and anthropometric measurements were conducted on the evening of PSG, and blood pressure was measured before sleep.Citation17 As recommended by the AASM, we considered an AHI ≥5 events/h as the diagnostic criteria for OSA. The exclusion criteria were central sleep apnea accounting for >50% of apnea events, previous noninvasive ventilator treatment, <5 hours of total sleep time, and incomplete data. The Ethics Committee of the Second Affiliated Hospital of Anhui Medical University approved the study protocol, and all participating patients signed informed consent forms. The study complies with the Declaration of Helsinki.
Sleep Measures
All participants underwent full-night PSG. PSG was performed using a PSG instrument (Embla S4500, USA), and electroencephalography, bilateral electro-ophthalmology and electromyography were performed. Airflow was monitored by nasal pressure sensors and/or oronasal thermal sensors. Oxygen saturation was measured with pulse oximetry. Hypoxemia was defined as pulse oximetry (SpO2) <90%.Citation18 All records were manually reanalyzed according to the standards defined by the AASM in 2012 by an experienced PSG technologist.Citation19 Patients with a total sleep duration of less than 5 hours were excluded.
MAD(s) is defined as the mean apnea and hypopnea duration in any body position and during any stage of sleep. HAD (min) is defined as the apnea–hypopnea duration per hour and calculated as MAD×AHI/60.
Statistical Analysis
The quantitative data with normal distributions are described as means ± standard deviations. The quantitative data with skewed distributions is described by M (P25, P75). Kruskal–Wallis and Mann–Whitney U-tests were used for intergroup comparisons. Frequency was used to describe qualitative data, and Pearson’s χ2 test was used for comparison between groups. Spearman correlation analysis was used to determine the correlations between variables. We assessed the adjusted R2, Akaike’s information criteria (AIC), and Schwarz Bayesian information criteria (BIC) as indicators of goodness-of-fit of the predictive models to explore which indexes could predict hypoxemia better. The larger the R2, the smaller the ACI and BIC, and the better the model fits. All participants were randomly assigned to a training cohort (70%) or a validation cohort (30%) in the stage of constructing the prediction model. The area under the curve (AUC) was predicted based on a receiver operating characteristic (ROC) curve.
SPSS 23.0 and R software 4.1.2 were used for the statistical analyses. P < 0.05 was considered a statistically significant difference.
Results
Baseline Characteristics
The 144 participants () had a mean age of 41.46 years and a mean body mass index (BMI) of 32.86 kg/m2. The sample was 73.6% male and 57.6% obese. The OSA severity groups were distributed as follows: 19.4% mild, 11.1% moderate, and 69.4% severe. The median values of the AHI, MAD, and HAD were 52.47 events/h, 23.03 s, and 20.47 min. The participants with hypoxemia tend to have higher levels of obesity, diabetes, AHI, MAD, and HAD compared with non-hypoxemia (all P value < 0.05). There was no difference between the two groups in terms of age, sex, and risk of hypertension.
Table 1 General Characteristics of the Participants
There were moderate to strong correlations between HAD, MAD, AHI, and blood oxygen concentration ( and Figure S1). The most relevant indicator of blood oxygen concentration is HAD (r = 0.73) among HAD, MAD, and AHI.
Figure 1 Correlation between oxygen saturation and sleep indexes.
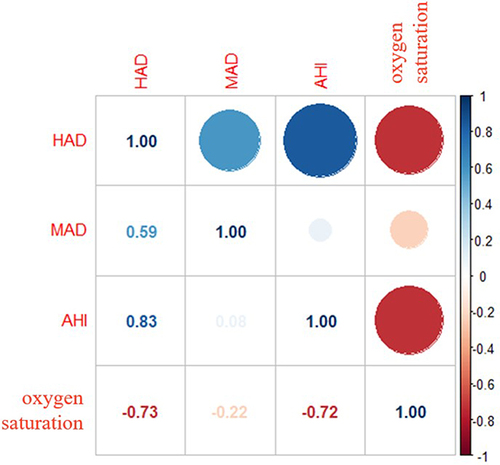
As shown in , we conducted a series of logistic regression models to assess which index could better predict a decrease in hypoxemia. The results indicated that the fitness of HAD showed the best (the highest R2, the lowest AIC, and the lowest BIC), suggesting that not only demographic data and AHI should be evaluated, but HAD should also be considered in the early warning of hypoxemia.
Table 2 Logistic Regression Models for Hypoxemia
We further used HAD, age, sex, and BMI to conduct a logistic prediction model for hypoxemia. We randomly divided all participants into the training set (70%, n = 100) and the validation set (30%, n = 44). The characteristics of the training and internal validation cohorts are summarized in detail in Table S1. All general characteristics showed no statistically significant difference between the training and validation sets (P > 0.05). The AUC values of the training set () and the validation set () are 0.95 and 0.95, respectively, indicating excellent performance. The increased HAD would elevate the risk of hypoxemia [odds ratio (OR): 1.30, 95% confidence interval (CI): 1.13–1.49] (). Further, decision curve analysis showed a good clinical application value in the training set () and the validation set ().
Table 3 Multiple Logistic Prediction Models in the Combined Set
Discussion
The present study found that, in comparison to AHI and MAD, HAD is more closely associated with oxygen saturation. When considering AHI alone, its ability to predict hypoxemia is relatively limited. However, the logistic prediction model revealed that HAD is effective in predicting the occurrence of hypoxemia (both AUC values of the training set and the validation set are 0.95), suggesting that future monitoring of sleep problems and hypoxemia should further consider HAD, in addition to AHI.
Intermittent episodes of low oxygen levels are acknowledged as a significant contributing factor to the development of comorbidities in OSA. This intermittent hypoxemia enhances oxidative stress, heightens sympathetic activation, and triggers inflammation.Citation5 Furthermore, previous studies have demonstrated that the severity of nocturnal hypoxemia might be equally or even more informative than the AHI in predicting the risk of related conditions, including hypertension, cardiac dysfunction, and endothelial impairment.Citation10 Consistent with previous literature,Citation20,Citation21 the present study found a higher level of AHI was related to the risk of hypoxemia and decreased oxygen saturation. In addition, we also found that MAD and HAD are positively associated with hypoxemia, indicating the necessity and possibility of a prediction model for hypoxemia according to respiratory disturbances during sleep.
Previous studies have demonstrated that apnea and hypopnea event durations were related to various adverse outcomes. In Saraç’s study, patients with OSA with a long average duration had more negative effects of sleep apnea than those with a short average duration, including snoring, morning tiredness, witnessed apnea and hypertension.Citation22 Another study also found that longer events were associated with lower mean oxygen saturation, and longer obstructive and total apnea events were associated with a higher oxygen desaturation index. Obstructive, mixed, and total apnea durations were positively associated with SpO2<85%.Citation23 All these authors believed that event duration could supplement the AHI in the follow-up and treatment. Although our previous study has established a percentage of total sleep time spent with apnoea and hypopnoea duration time (AHT%) and reported a negative correlation between it and oxygen saturation,Citation21 the study did not establish a prediction model of hypoxemia. The present study indicated that elevated HAD was not only positively significantly associated with the risk of hypoxemia but also could better predict the occurrence of hypoxemia among patients with OSA than AHI and MAD. The utility of HAD in predicting hypoxemia from its capacity to offer a more nuanced perspective on the cumulative duration of respiratory disturbances during sleep, aligning closely with the physiological mechanisms underlying oxygen desaturation. Previous studies have found that shorter respiratory event duration significantly predicted higher 11-year mortality compared with longer respiratory event duration.Citation12 Moreover, the average event duration may provide insights into ventilatory dynamics and airway collapsibility that are not encompassed by AHI. Consistent with the studies, we believed that HAD, the index representing a combination of apnea and hypopnea event durations, holds promise as a valuable metric for predicting and evaluating the risk of concomitant hypoxemia among individuals with sleep-related breathing disorders.
Our study has the following limitations. First, this study included 49 patients undergoing metabolic surgery for weight loss, and obese patients have a more than 10-fold probability of developing OSA;Citation24 therefore, selection bias may exist. Second, the cross-sectional could not draw a causal inference. Future longitudinal studies are warranted to replicate our results. Third, there has been no research on the relationship between HAD and long-term adverse prognoses, such as mortality and cardiovascular events. Moreover, our sample size was relatively small, which impedes the reliability of our results. Future studies should enlarge the sample size and replicate the findings. Lastly, the mechanisms of the relationship between HAD and hypoxemia were not clear, and there is a need to conduct more laboratory studies to clarify the potential mechanisms.
In conclusion, by complementing existing metrics such as AHI with a more detailed assessment of the cumulative duration of apneas and hypopneas, HAD has the potential to contribute to a more holistic understanding of the relationship between sleep-disordered breathing and hypoxemia, thereby informing targeted interventions and improving health outcomes for affected individuals.
Abbreviations
AASM, the American Academy of Sleep Medicine; AHI, apnea–hypopnea index; Avg SpO2, average oxygen saturation; BMI, body mass index; DBP, diastolic blood pressure; HAD, apnea–hypopnea duration per hour; LAD, longest apnea duration; LHD, longest hypopnea duration; MAD, mean apnea–hypopnea duration; Min SpO2, minimum oxygen saturation; OSA, obstructive sleep apnea; PSG, polysomnography; SBP, systolic blood pressure.
Disclosure
The authors report no conflicts of interest in this work.
Acknowledgments
Changxiu Ma is the first author of this study. This work was supported by the Natural Science Research Project of Anhui Educational Committee (2022AH050694).
References
- West JB. Causes of and compensations for hypoxemia and hypercapnia. Compr Physiol. 2011;1(3):1541–1553. doi:10.1002/cphy.c091007
- Shobatake R, Ota H, Takahashi N, Ueno S, Sugie K, Takasawa S. The impact of intermittent hypoxia on metabolism and cognition. Int J Mol Sci. 2022;23(21):12957. doi:10.3390/ijms232112957
- Liu Y. Suppression of myocardial hypoxia-inducible factor-1α compromises metabolic adaptation and impairs cardiac function in patients with cyanotic congenital heart disease during puberty (vol 143, pg 2254, 2021). Circulation. 2021;143(23):E1030–E1030. doi:10.1161/Cir.0000000000000991
- Johnson J. Systemic hypoxemia induces cardiomyocyte hypertrophy and right ventricular specific induction of proliferation. Circul Res. 2023;132(6):723–740. doi:10.1161/Circresaha.122.321604
- Huang ZH, Duan A, Hu M, et al. Implication of prolonged nocturnal hypoxemia and obstructive sleep apnea for pulmonary hemodynamics in patients being evaluated for pulmonary hypertension: a retrospective study. J Clin Sleep Med. 2023;19(2):213–223. doi:10.5664/jcsm.10286
- Taylor CT, Colgan SP. Regulation of immunity and inflammation by hypoxia in immunological niches. Nat Rev Immunol. 2017;17(12):774–785. doi:10.1038/nri.2017.103
- Dewan NA, Nieto FJ, Somers VK. Intermittent hypoxemia and OSA implications for comorbidities. Chest. 2015;147(1):266–274. doi:10.1378/chest.14-0500
- Kendzerska T, Kapur VK. OSA-Related Hypoxemia and Cancer Risk. Chest. 2020;158(6):2264–2265. doi:10.1016/j.chest.2020.08.2046
- Benjafield AV. Estimation of the global prevalence and burden of obstructive sleep apnoea: a literature-based analysis. Lancet Respir Med. 2019;7(8):687–698. doi:10.1016/S2213-2600(19)30198-5
- Suen C, Ryan CM, Mubashir T, et al. Sleep study and oximetry parameters for predicting postoperative complications in patients with OSA. Chest. 2019;155(4):855–867. doi:10.1016/j.chest.2018.09.030
- Mazzotti DR, Keenan BT, Lim DC, et al. Symptom subtypes of obstructive sleep apnea predict incidence of cardiovascular outcomes. Am J Respir Crit Care Med. 2019;200(4):493–506. doi:10.1164/rccm.201808-1509OC
- Butler MP. Apnea-hypopnea event duration predicts mortality in men and women in the sleep heart health study. Am J Respir Crit Care Med. 2019;199(7):903–912. doi:10.1164/rccm.201804-0758OC
- Punjabi NM. Is the apnea-hypopnea index the best way to quantify the severity of sleep-disordered breathing? No Chest. 2016;149(1):16–19. doi:10.1378/chest.14-2261
- Zinchuk AV. Polysomnographic phenotypes and their cardiovascular implications in obstructive sleep apnoea. Thorax. 2018;73(5):472–480. doi:10.1136/thoraxjnl-2017-210431
- Xie J, Sert Kuniyoshi FH, Covassin N, et al. Nocturnal hypoxemia due to obstructive sleep apnea is an independent predictor of poor prognosis after myocardial infarction. J Am Heart Assoc. 2016;5(8):e003162. doi:10.1161/JAHA.115.003162
- Kainulainen S, Töyräs J, Oksenberg A, et al. Severity of desaturations reflects OSA-related daytime sleepiness better than AHI. J Clin Sleep Med. 2019;15(8):1135–1142. doi:10.5664/jcsm.7806
- Huang JF, Chen LD, Lin QC, et al. The relationship between excessive daytime sleepiness and metabolic syndrome in severe obstructive sleep apnea syndrome. Clin Respir J. 2016;10(6):714–721. doi:10.1111/crj.12276
- Vukovic AA, Hanson HR, Murphy SL, et al. Apneic oxygenation reduces hypoxemia during endotracheal intubation in the pediatric emergency department. Am J Emerg Med. 2019;37(1):27–32. doi:10.1016/j.ajem.2018.04.039
- Duce B, Milosavljevic J, Hukins C. The 2012 AASM respiratory event criteria increase the incidence of hypopneas in an adult sleep center population. J Clin Sleep Med. 2015;11(12):1425–1431. doi:10.5664/jcsm.5280
- Fan ZT. Association of dyslipidemia and hypoxemia in patients with obstructive sleep apnea hypopnea syndrome: a cross-sectional study. Am J Transl Res. 2022;14(11):8263–8270.
- Ma CX, et al. A novel parameter is better than the AHI to assess nocturnal hypoxaemia and excessive daytime sleepiness in obstructive sleep apnoea. Sci Rep. 2021;11(1):4702. doi:10.1038/s41598-021-84239-0
- Sarac S, Afsar GC. Effect of mean apnea-hypopnea duration in patients with obstructive sleep apnea on clinical and polysomnography parameter. Sleep Breathing. 2020;24(1):77–81. doi:10.1007/s11325-019-01870-y
- Durmaz DY, Günes A. Which is more important: the number or duration of respiratory events to determine the severity of obstructive sleep apnea? Aging Male. 2020;23(2):119–124. doi:10.1080/13685538.2019.1630062
- Piliar G, Shehadeh N. Abdominal Fat and Sleep Apnea The chicken or the egg? Diabetes Care. 2008;31:S303–S309. doi:10.2337/dc08-s272