Abstract
Background
Primary liver cancer (PLC) is a fatal malignancy, sleep quality and gut microbiota were shown to be associated with PLC. However, the mechanism of how sleep quality affects PLC is unclear. This study aims to investigate the mediation/moderation effects of gut microbiota on sleep quality and the occurrence of PLC.
Methods
The causality of sleep quality and the occurrence of PLC was detected through the Mendelian randomization (MR) analysis based on the data including 305,359 individuals (Finland Database) and 456,348 participants (UK Biobank). The primary method used for MR analysis was inverse-variance weighted analysis. Gut microbiota’ mediation/moderation effects were uncovered in the case–control study including 254 patients with PLC and 193 people with benign liver diseases through the mediation/moderation effect analyses. People’s sleep quality was evaluated through the Pittsburgh sleep quality index (PSQI).
Results
Poor sleep quality could lead to PLC through the MR analysis (P = 0.026). The case–control study uncovered that Actinobacteria had mediation effects on the relationship between PSQI score, self-sleep quality, and the occurrence of PLC (P = 0.048, P = 0.046). Actinobacteria and Bifidobacterium could inhibit the development of PLC caused by short night sleep duration (P = 0.021, P = 0.022). Erysipelotrichales could weaken the influence of daytime dysfunction on PLC (P = 0.033). Roseburia modulated the contribution of nocturnal insomnia and poor sleep quality to PLC (P = 0.009, P = 0.017).
Conclusion
Poor sleep quality was associated with PLC. Gut microbiota’ mediation/moderation effects on poor sleep quality and the occurrence of PLC prompted an insightful idea for the prevention of PLC.
Introduction
Primary liver cancer (PLC) is one of the most common malignancies, which ranks globally sixth in incidence rate (4.7%) and third in fatality rate (8.3%).Citation1 PLC is closely bound up with human being’s life. The processes and variables influencing the incidence of PLC are complicated. Previous studies have shown that sleep disturbances have a significant impact on PLC.Citation2 The International Agency for Research on Cancer (IARC) evaluates the experimental and epidemiological evidence of the link between shift work and various cancers, concluding that circadian rhythm abnormalities can accelerate the occurrence and development of tumors.Citation3 These researches uncover sleep quality is closely related to the occurrence of PLC, but the specific relationship between them remains unclear.
Gut microbiota, a new “organ”, is regarded as closely related to human health.Citation4 Strong evidence suggests that an imbalance of intestinal microorganisms can promote the development of PLC and pancreatic carcinoma through the gut–liver axis.Citation5 Coincidentally, the microbial gut-brain axis is a critical regulatory factor in gut-brain activity.Citation6 These findings suggest that the gut-brain axis and associated microbes may be the crucial regulatory routes for brain neurodegeneration.Citation7 Similarly, people’s sleep conditions can influence intestinal bacteria via the gut-brain axis directly or indirectly.Citation8 While the effects of sleep quality and gut microbiota on the occurrence of PLC are increasingly realized, a comprehensive understanding of the relationship between sleep quality, gut microbiota and PLC is lacking.
The mediation/moderation effect analyses are regarded as the effective methods for uncovering the relationship between risk factors and the occurrence of diseases.Citation9,Citation10 These methods can detected the roles of the third party factors in the causal pathway from exposures to outcomes. Accordingly, mediation/moderation effect analyses were further performed to reveal the effects of gut microbiota on sleep quality and the occurrence of PLC. Meantime, Mendelian randomization (MR), an effective strategy for overcoming confounding variables and reverse causality in classical observational research, measures the causal link between exposure and outcome using an indicator of genetic variation.Citation11 MR analysis uses single nucleotide polymorphisms (SNPs) as instrumental variables (IVs) because they are not affected by potential environmental confounders or disease status, thus MR analysis is considered as a form of natural randomized controlled trial.Citation12 The extended nature of PLC progression challenges traditional research methodologies in effectively exploring the relationship between sleep quality and PLC development. MR analysis emerges as a powerful method, addressing confounding variables and uncovering the causal links between these factors. Additionally, there exists a significant research gap regarding the interplay between sleep quality, gut microbiota, and PLC. This underscores the critical importance of employing mediation/moderation analyses and MR analysis to facilitate pertinent studies, thereby revealing the complex interactions within these relationships. In this study, we first engaged MR analysis to reveal the causal relationship between sleep quality and PLC. Then, a case–control study was undertaken to uncover the causal mediation/moderation effects of gut microbiota on the relationship between sleep quality and the occurrence of PLC.
Materials and Methods
Mendelian Randomization Analysis
Study Design
A two-sample MR design was used to evaluate the causal effect of sleep quality on PLC. It was based on the following three core assumptions: (i) genetic Instruments Variables (IVs) must be strongly correlated with sleep quality evaluation index; (ii) the IVs selected must be irrelevant to the confounding factors; (iii) IVs can only affect PLC through the exposure.Citation13
Data Sources for Exposure and Outcome
First, we used the summary-level GWAS data correlated with the disorder of the sleep-wake schedule from the Finland Database (https://r9.finngen.fi/), a Finnish cohort database (410 cases and 371,145 controls) containing very comprehensive genetic information, as the exposure to evaluate the people’s sleep quality. Meanwhile, we drew summary statistics of GWAS associated with PLC, which was strictly defined by the International Classification of Diseases Tenth Revision (ICD-10) codes from UK Biobank (a large cohort of approximately 500,000 individuals aimed at collecting the genotype and various phenotypic data).Citation14 The outcome data includes 128 cases and 456,220 controls,Citation15 and Supplementary Table S1 displays the information of datasets utilized in this study.
Instrumental Variables Selection
To explore the causal relationship between the disorder of the sleep quality and PLC better, SNPs were used as IVs. IVs were selected via the following criteria: (i) a GWAS-correlated P-value of 1 × 10−5 and (ii) a linkage disequilibrium r2 of <0.001, and <10,000 KB from the index variantCitation16 To guarantee a high connection between IVs and exposure variables, the F-statistic of each SNP was utilized to avoid bias induced by weak IVs.Citation17 When the F value exceeded 10, it was assumed that there was no weak instrumental variable bias. Furthermore, IVs selected for participation in this study were scanned on the PhenoScanner website (http://www.phenoscanner.medschl.cam.ac.uk/) for pleiotropic effects; if any SNP was connected with the outcomes and confounding factors, they should be removed. Then, MR Pleiotropy RESidual Sum and Outlier (MR-PRESSO) was applied to avoid outliers. Supplementary Figure S1 shows the MR analysis process.
Two-Sample Mendelian Randomization Analysis
Four distinct approaches of MR (inverse-variance weighted (IVW), MR-Egger, MR robust adjusted profile score (MR-RAPS) and weighted median) were performed to make the results based on the summary-level GWAS data correlated with the disorder of the sleep-wake schedule and PLC more robust. IVW was used as the major method, and MR-RAPS was also utilized for further avoidance of bias due to weak IVs. To detect heterogeneity, the Cochran’s Q test was also performed.
Case–Control Study
Patients
Patients with PLC and normal control group were recruited from January 2020 to May 2022 at the First Affiliated Hospital of Wenzhou Medical University. PLC was clinically or pathologically diagnosed according to the National Comprehensive Cancer Network (NCCN) standards.Citation18 Patients’ inclusion criteria were as follows: (i) >18 years old; (ii) no medication history that could modify the microbiome (like antibiotics, probiotics, lactulose, rifaximin, proton pump inhibitors, etc.) in the last eight weeks; (iii) no history of hepatic encephalopathy and other malignant tumours; (iv) signing the informed consent form. Fecal samples were collected before treatment. All clinical indicators such as age, gender, body mass index (BMI), eating habits, smoking history, drinking history, hepatitis B infection, cirrhosis, and serum markers were collected.
Eating habits were categorized into three levels: meat, vegetarian and balanced. Meat eaters were people who ate a lot of meat and fish (three meals a day, every meal) and very little vegetarian food (less than one meal a day); vegetarians included people that did not eat meat and fish (less than one meal a day) and lots of vegetarian food (three meals a day, every meal); and balanced eaters for those who ate a balanced and reasonable amount of meat, fish, and vegetarian food (three meals a day, every meal).Citation19–21 According to the 11th Revision of the International Classification of Diseases (ICD-11), the history of smoking and alcohol consumption was classified as never had, currently have, and quit.Citation22 Never smokers were those who had never smoked; smokers were those who had smoked for six months or more in their lifetime and were currently smoking at the time of the survey; quitters included those who had smoked in the past but did not smoke anymore at the moment of the survey. Meanwhile, never drinkers were those who had never consumed alcohol; drinkers included those who have consumed alcohol at least once a week or more, continuously or cumulatively for more than one year; and abstainers were individuals who had previously consumed alcohol but had not consumed alcohol for one year at the time of the study. The flowchart of the case–control study is shown in .
This study was conducted in accordance with the ethical standards laid down in the 1964 Declaration of Helsinki and its later amendments. All human participants provided informed consent prior to inclusion in the study. This study was approved by the Ethics Committee in Clinical Research of the First Affiliated Hospital of Wenzhou Medical University, Zhejiang, China (Ref KY2022-101). Written informed consent from the patient was required for each patient before the research.
Sleeping Quality Evaluation
Patients’ previous sleep quality in recent three years was estimated by the Pittsburgh sleep quality index (PSQI) schedule.Citation23 The total PSQI score consisted of seven parts: self-sleep quality evaluation, the time required to enter sleep at night, night sleep time, sleep efficiency, night sleep disorders, hypnotic drug use, and daytime dysfunction (often feel sleepy or have no energy to complete the work during the day). The sleep quality was regarded as unpleasant when the PSQI score was more than five; otherwise, sleep quality was regarded as excellent.
Fecal Sample Collection, 16S rRNA Sequencing and Analysis
Fecal samples were collected before treatment, which could reflect the changes in gut microbiota over a six-month period. Each fecal sample’s genomic DNA was extracted using the EZNA Stool DNA Kit (D4015, Omega, Inc., USA). The V3-V4 region of 16S rRNA was amplified by PCR using two primers, 341F (5’-CCTACGGGNGGCWGCAG-3’) and 805R (5’-GACTACHVGGGTATCTAATCC-3’).Citation24 Divisive Amplicon Denoising Algorithm 2 in Quantitative Insights Into Microbial Ecology 2 was hired to identify and rectify the original readings. The distribution differences between the two groups of different flora were detected through principal coordinate analysis (PCoA) and non-metric multidimensional scaling (NMDS). Linear discriminant analysis effect size (LEfSe) was utilized to filter the discriminating taxa across groups with the LDA ≥3.Citation25
Propensity Score Matching Method
This study employed the propensity score matching (PSM) approach to eliminate confounding factors such as age, gender, BMI, diet, smoking history, drinking history, and the prevalence of Hepatitis B and cirrhosis. The nearest neighbor matching method (NNM) was utilized for 1:1 matching with the caliper value was 0.12.
Mediation/Moderation Effect Analyses
The mediation/moderation effect analyses were employed to explore the effects of gut microbiota on sleep quality and the occurrence of PLC. We used the occurrence of PLC as the dependent variable, intestinal microorganisms as the intermediate/regulatory variables, and indicators with different sleep statuses between both were taken as the independent variables for analysis. The intermediate impact was verified using the Bootstrap approach. The Johnson-Neyman (JN) method verified the regulation effects’ simple slopes and the range of moderation variables.
Statistical Analyses
All statistical analyses were performed with R software (V4.2.0). Continuous data were analyzed by t-test or Mann–Whitney U-test, shown as mean ± standard deviation (SD) or medians (interquartile range) according to the distribution. All MR-related analyses were based on “TwoSampleMR”, “MR-PRESSO”, and “mr.raps” R packages. PSM was performed with the “MatchIt” R package. The mediating/moderating effect analyses were accomplished with the “mediation” and “interactions” R packages. P-values <0.05 was deemed statistically significant.
Results
Mendelian Randomization Analysis
In total, 11 index SNPs were selected to genetically predict people’s sleep quality, which was measured by the disorder of the sleep-wake schedule. The results of the F statistics for these genetic instruments were all larger than 10, which indicated strong instruments (Supplementary Table S2). There were no SNPs identified to be linked with the outcome or confounding variables through the Phenoscanner website (Supplementary Table S3). The MR-PRESSO, leave-one-out plot, as well as scatter plot showed that there was no outlier (Supplementary Table S4 and Supplementary Figure S2). The IVW results revealed that disorder of the sleep-wake schedule could lead to PLC (OR = 1.30, 95% CI: 1.03–1.63, P = 0.026). There also existed this causal relationship through the MR-RAPS (OR = 1.31, 95% CI: 1.03–1.68, P = 0.030). The MR results are displayed in Supplementary Figure S3. And the P-value of Cochran’s Q test was >0.05 demonstrating that there was no heterogeneity (Supplementary Table S4).
Case–Control Study
Baseline Characteristics
First, 254 individuals with PLC and 193 normal people were registered, among which 145 normal individuals and 173 with PLC were involved according to the inclusion criteria. Then, 102 pairs of patients with PLC and normal people were eventually selected for analysis after PSM with no difference in baseline characteristics (age, gender, BMI, diet, smoking history, and alcohol drinking) (Supplementary Table S5). Clinical characteristics are shown in and Supplementary Table S6.
Figure 2 Clinicopathological features of individuals with and without Primary Liver Cancer. *P < 0.05; **P < 0.01; ***P ≤ 0.001.
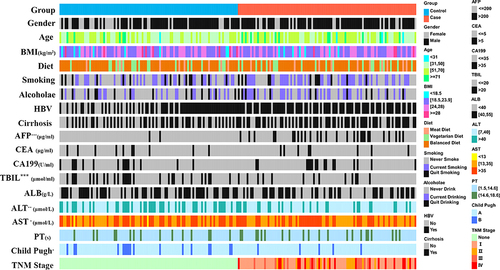
Sleep Quality Comparison
The results of PSQI scale showed that there were significant distinctions in sleep quality between the control and case groups, mainly in these aspects: Self sleep quality assessment (P = 0.001), Time required to go to sleep at night (P = 0.001), Sleep time at night (P = 0.002), Nocturnal sleep disorder (P < 0.001), Daytime dysfunction (P < 0.001), PSQI Score (P < 0.001) (Supplementary Table S7). Supplementary Figure S4 displays the distribution and differences in overall PSQI scores.
Gut Microbiota Diver Differences
Based on the observed species index, Chao1 index, and goods_Coverage index, we found that alpha diversity in patients with PLC was different from those without PLC (). Simpson index showed no statistical difference (). PCoA and NMDS illustrated the beta diversity of intestinal flora ( and ). The differences in intestinal flora between the two groups were found through the Spearman correlation analysis (Supplementary Figure S5). The LEfSe identified specific microbiota biomarkers, which was used as mediating/moderating variables (). Biomarkers exhibiting statistical differences between the two groups were discovered from phylum to species ().
Figure 3 Analysis and description of differences in alpha diversity and beta diversity of gut microbiota between the individuals with and without Primary Liver Cancer. (A) Observed_otus; (B) Goods_coverage; (C) Chao Index; (D) Simpson Index; (E) PCoA of weighted Unifrac distance matrix; (F) NMDS Analysis; *P < 0.05; **P < 0.01; ***P < 0.001.
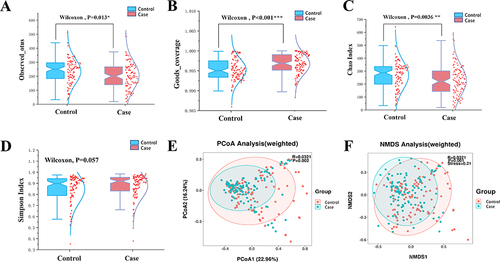
Mediation Effect Analysis
There was a connection between the PSQI Score, self-sleep quality assessment and PLC (). Actinobacteria was employed as an intermediary factor between PSQI score, self-sleep quality assessment and PLC (95% CI: 0.00003–0.01, P = 0.048; 95% CI: 0.00044–0.05, P = 0.046). Likewise, the direct and total impacts were significant, showing partial intermediary effects existed (6.09%, 10.88%).
Table 1 The Mediation Effects of Various Levels of Gut Microbiota on the Relationship Between Sleep Quality and Primary Liver Cancer
Moderation Effect Analysis
represents the moderation effects of the intestinal flora between the two groups. Firstly, the Actinobacteria’s regulatory effect was found between the score of night sleep time and PLC (P = 0.021). The regression coefficient of the main effect implied that less night sleep time might increase the probability of PLC. However, the negative regression coefficient of the regulatory effect indicated that Actinobacteria had a negative regulatory function. Then, we found that Actinobacteria’s negative regulation existed when its relative abundance was less than 12.42% ( and Supplementary Figure S6A). Secondly, the moderating effect also existed when Erysipelotrichales was applied as the regulatory variable, while the independent variable was the daytime dysfunction score (P = 0.033). Moreover, the positive main effect revealed that severer daytime dysfunction (higher daytime dysfunction score) was positively related to PLC. The positive regression coefficient of the regulatory impact demonstrated that Erysipelotrichales performed a beneficial regulatory function. Regulation occurred when the relative abundance of Erysipelotrichales was over 0.97% ( and Supplementary Figure S6B).
Table 2 The Moderation Effects of Various Levels of Gut Microbiota on the Relationship Between Sleep Quality and Primary Liver Cancer
We also discovered a significant relationship between the overall PSQI score and PLC via Roseburia (P = 0.017). Meanwhile, Roseburia’s moderation effect of the duration necessary to enter sleep at night and PLC existed (P = 0.009). A higher PSQI score or a long time to go to sleep was taken as a higher risk of PLC. Uniformly, Roseburia’s active regulatory impact was equally demonstrated in this research. The JN test revealed Roseburia’s regulatory effect on the overall PSQI score and PLC when it was present ( and Supplementary Figure S6C). Besides, we found that when the relative abundance of Roseburia was more prominent than 0.34%, its regulatory effect of the time required to enter sleep at night and PLC existed ( and Supplementary Figure S6D). We also uncovered that Bifidobacterium could be used as a regulatory variable of night sleep duration score and PLC (P = 0.022). Meanwhile, the central effect regression coefficient indicated that less sleep time at night was associated with PLC. Nonetheless, Bifidobacterium performing a passive regulatory function was proclaimed by the negative regulatory impact regression coefficient, while the Bifidobacterium’s relative abundance was less than 12.35% ( and Supplementary Figure S6E).
Association Between Specific Sleep Disorders, Gut Microbiota, and PLC
Moreover, we executed 1000 mediation and moderation analyses (5 specific sleep characteristics × 100 different gut microbiota × 2 mediation/moderation analyses) to delve into the roles of gut microbiota as mediators and moderators. These analyses covered 100 species, distributed evenly across every taxonomic level from phylum to genus, each exhibiting significant disparities between PLC patients and healthy controls, as detailed in Supplementary Figure S5. Our investigation focused on the intricate relationships between five specific sleep characteristics (each markedly different between PLC patients and healthy controls including self-sleep quality assessment, time required to go to sleep at night, sleep time at night, nocturnal sleep disorder, and daytime dysfunction as detailed in Supplementary Table S7) and PLC. Utilately, we found the mediation effect of Micrococcales and Micrococcaceae in relationship between sleep time at night, daytime dysfunction, and PLC (). Meantime, we also discovered the mediation effect of Elusimicrobia and Enterococcaceae (). Furthermore, some specific gut microbiota’s moderation effects were dected (). The results of 1000 mediation and moderation analyses were detailed in Supplementary Tables S8–S12.
Table 3 The Mediation Effects of Various Levels of Gut Microbiota on the Relationship Between Specific Sleep Disorder and Primary Liver Cancer
Table 4 The Moderation Effects of Various Levels of Gut Microbiota on the Relationship Between Specific Sleep Disorder and Primary Liver Cancer
Discussion
This study used a two-sample MR method to explore the causal relationship between sleep quality and the occurrence of PLC. The MR results showed that this causal connection existed. Furthermore, we revealed the relationship between sleep quality and PLC via the gut microbiota through a case–control study. Encouragingly, we found that some gut microbiota could mediate/moderate this relationship.
However, gut microbiota were pivotal in the onset and progression of PLC. Significant alterations had occurred in the intestinal tracts of individuals with chronic liver diseases and cirrhosis, as evidenced by correlational researches.Citation26,Citation27 Relevant research had demonstrated that people’s sleep quality could impact their gut microbiota via the gut-brain axis.Citation28 The gut microbiota also plays a significant role in determining the quality of sleep in humans.Citation8 Sleep deprivation had also been found in studies that could produce intestinal issues, inflammatory responses, and cognitive impairment among humans. Therefore, we used intestinal microorganisms as third-party variables to detect the relationship between sleep quality and PLC.
We found that when Erysipelotrichales’s relative abundance was more significant than 0.97%, it could regulate the development of daytime dysfunction in PLC. Relevant studies had shown that when its relative abundance increased in the human body, and it could lead to non-alcoholic steatohepatitis (NASH), Alzheimer’s disease (AD), and sleep diseases.Citation29–31 These studies provided a strong theoretical basis for our findings that daytime dysfunction could promote the occurrence of PLC through Erysipelotrichales. Roseburia also played a positive moderating function between poor sleep quality and PLC when its relative abundance was more significant than 0.34%. Relevant study elaborated that faecal microbiota composition in patients with ulcerative colitis and rapid-eye-movement sleep behavior disorder differed considerably from that in healthy persons; Roseburia hominis, a butyrate-producing species, was distinctly decreased, resulting in an ecological imbalance.Citation32,Citation33 However, when patients who require this probiotic medication have agrypnia or sleep difficulties, this treatment may not be all that straightforward. Although probiotic therapy, such as replenishing Roseburia, holds a bright future, it is not applicable all the time and needs to be closely linked to the patient’s specific situation for precise treatment, according to our research findings.
In addition, we uncovered that Actinobacteria’s partial mediating effects of PSQI score, self-reported sleep quality and PLC (6.09%, 10.88%). When the relative abundance of Actinobacteria was less than 12.42%, it could adversely control the pro-liver cancer impact of shorter night sleep duration. Jia et al and Nishida et al had disclosed that the abundance of Actinobacteria was substantially greater in individuals with intrahepatic cholangiocarcinoma and sleep disturbance than in healthy people.Citation34,Citation35 This is consistent with the observations, indicating the vital function of Actinobacteria in the human body. Likewise, we identified that if its abundance was too low or too high, the negative regulation of sleep quality and the development of PLC might be employed as a new study avenue. Bifidobacterium, another well prophylactic, could inhibit the advancement of PLC resulting from a lack of nocturnal sleep duration. In addition, passive legislation arose whenever its relative abundance was less than 12.35%. A similar result was also obtained that oral administration of Bifidobacterium breve (B. breve) lw01 could inhibit tumour growth and promote tumour cell apoptosis.Citation36 Concurrently, an animal study has shown that administering probiotics such as Bifidobacterium can mitigate insulin resistance induced by chronic sleep deprivation in rhesus monkeys.Citation37 Based on the results of our study, supplementing these probiotic microorganisms may weaken the effect of lack of sleep time at night on PLC.
Conversely, although having satisfactory internal consistency, reliability and validity, the PSQI scale falls short of further defining people’s sleep quality.Citation23 Consequently, it should be adopted with appropriate wearable devices to obtain more detailed data on multiple sleep quality indices. Numerous confounding factors existed since this was a single-center cross-sectional study. Therefore, a large multi-center cohort study is further needed. Moreover, although we have identified the mediation/moderation effects of the gut microbiota on the relationship between sleep quality and PLC, the precise mechanisms upstream and downstream remain uncharted. Thus, there is a compelling need for further prospective clinical and foundational experiments to elucidate these pathways.
In conclusion, this research uncovered the relationship between sleep quality and PLC. Moreover, we utilized gut microbiota as the mediate/moderate variables to detect the association between sleep quality and PLC. Several particular gut microbiota could alter the impact of sleep quality on PLC, providing an alternative approach to probiotic therapy.
Abbreviations
PLC, primary liver cancer; MR, Mendelian randomization; GWASs, genome-wide association studies; IVW, Inverse-variance weighted; MR-RAPS, MR robust adjusted profile score; IVs, Instruments Variables; PSQI, Pittsburgh sleep quality index; PSM, Propensity score matching method; NCCN, National Comprehensive Cancer Network; ICD-10, the International Classification of Diseases Tenth Revision; ICD-11, the 11th Revision of the International Classification of Diseases; JN method, Johnson Neyman method; BMI, Body Mass Index; HBV, Hepatitis B virus; AFP, Alpha Fetoprotein; CEA, Carcinoembryonic Antigen; CA199, Carbohydrate Antigen199; TBIL, Total Bilirubin; ALB, Albumin; ALT, Alanine aminotransferase; AST, Aspartate Transaminase; PT, Prothrombin Time; PcoA, principal coordinates analysis; NMDS, non-metric multidimensional scaling.
Ethics Approval and Consent to Participate
The publication of any potentially identifiable images or data contained in this study requires the written informed consent of individuals. On enrolling, all subjects provided written informed consent. This study was approved by the Ethics Committee in Clinical Research of the First Affiliated Hospital of Wenzhou Medical University, Zhejiang, China (Ref KY2022-101).
Author Contributions
All authors made a significant contribution to the work reported, whether that is in the conception, study design, execution, acquisition of data, analysis and interpretation, or in all these areas; took part in drafting, revising or critically reviewing the article; gave final approval of the version to be published; have agreed on the journal to which the article has been submitted; and agree to be accountable for all aspects of the work.
Disclosure
All authors declare no conflicts of interest in this work.
Acknowledgments
We thank the funding supported by the National Natural Science Foundation of China (No-82072685). We thank the Finland Database and the UK Biobank for providing GWAS data. Thanks to our colleagues and nurses for their assistance in the collection of samples. Thanks to our colleagues for their contribution to this study.
Data Sharing Statement
All GWAS summary statistics analysed in this study are publicly available for download by qualified researchers. The GWAS for the disorder of the sleep-wake schedule was provided by the Finland Database (https://r9.finngen.fi/pheno/F5_SLEEPWAKE). The GWAS for PLC was provided by the UK Biobank (https://github.com/Nealelab/UK_Biobank_GWAS). All data used to support the conclusions of the case–control study are accessible upon reasonable request from the principal investigator.
Additional information
Funding
References
- Sung H, Ferlay J, Siegel RL, et al. Global cancer statistics 2020: GLOBOCAN estimates of incidence and mortality worldwide for 36 cancers in 185 countries. CA Cancer J Clin. 2021;71(3):209–249. doi:10.3322/caac.21660
- Mogavero MP, DelRosso LM, Fanfulla F, Bruni O, Ferri R. Sleep disorders and cancer: state of the art and future perspectives. Sleep Med Rev. 2021;56:101409. doi:10.1016/j.smrv.2020.101409
- Straif K, Baan R, Grosse Y, et al. Carcinogenicity of shift-work, painting, and fire-fighting. Lancet Oncol. 2007;8(12):1065–1066. doi:10.1016/S1470-2045(07)70373-X
- Heintz-Buschart A, Wilmes P. Human gut microbiome: function matters. Trends Microbiol. 2018;26(7):563–574. doi:10.1016/j.tim.2017.11.002
- Mima K, Nakagawa S, Sawayama H, et al. The microbiome and hepatobiliary-pancreatic cancers. Cancer Lett. 2017;402:9–15.
- Cryan JF, O’Riordan KJ, Cowan CSM, et al. The microbiota-gut-brain axis. Physiol Rev. 2019;99(4):1877–2013. doi:10.1152/physrev.00018.2018
- Dinan TG, Cryan JF. Gut instincts: microbiota as a key regulator of brain development, ageing and neurodegeneration. J Physiol. 2017;595(2):489–503. doi:10.1113/JP273106
- Sen P, Molinero-Perez A, O’Riordan KJ, McCafferty CP, O’Halloran KD, Cryan JF. Microbiota and sleep: awakening the gut feeling. Trends Mol Med. 2021;27(10):935–945. doi:10.1016/j.molmed.2021.07.004
- Holden S, Matthews M, Rathleff MS, Kasza J, Vicenzino B. How do hip exercises improve pain in individuals with patellofemoral pain? Secondary mediation analysis of strength and psychological factors as mechanisms. J Orthop Sports Phys Ther. 2021;51(12):602–610. doi:10.2519/jospt.2021.10674
- Liu H, Yuan KH. New measures of effect size in moderation analysis. Psychological Methods. 2021;26(6):680–700. doi:10.1037/met0000371
- Morrison J, Knoblauch N, Marcus JH, Stephens M, He X. Mendelian randomization accounting for correlated and uncorrelated pleiotropic effects using genome-wide summary statistics. Nature Genet. 2020;52(7):740–747. doi:10.1038/s41588-020-0631-4
- Yao S, Zhang M, Dong SS, et al. Bidirectional two-sample Mendelian randomization analysis identifies causal associations between relative carbohydrate intake and depression. Nat Human Behav. 2022;6(11):1569–1576. doi:10.1038/s41562-022-01412-9
- Sekula P, Del Greco MF, Pattaro C, Köttgen A. Mendelian randomization as an approach to assess causality using observational data. J Am Soc Nephrol. 2016;27(11):3253–3265. doi:10.1681/ASN.2016010098
- Lesseur C, Diergaarde B, Olshan AF, et al. Genome-wide association analyses identify new susceptibility loci for oral cavity and pharyngeal cancer. Nature Genet. 2016;48(12):1544–1550. doi:10.1038/ng.3685
- Jiang L, Zheng Z, Fang H, Yang J. A generalized linear mixed model association tool for biobank-scale data. Nature Genet. 2021;53(11):1616–1621. doi:10.1038/s41588-021-00954-4
- Xie J, Huang H, Liu Z, et al. The associations between modifiable risk factors and nonalcoholic fatty liver disease: a comprehensive Mendelian randomization study. Hepatology. 2022;77(3):949–964.
- Yuan S, Mason AM, Carter P, et al. Selenium and cancer risk: wide-angled Mendelian randomization analysis. Int J Cancer. 2022;150(7):1134–1140. doi:10.1002/ijc.33902
- Benson AB, D’Angelica MI, Abbott DE, et al. NCCN guidelines insights: hepatobiliary cancers, version 1.2017. J Natl Compr Canc Netw. 2017;15(5):563–573. doi:10.6004/jnccn.2017.0059
- Kahleova H, Levin S, Barnard ND. Vegetarian dietary patterns and cardiovascular disease. Prog Cardiovasc Dis. 2018;61(1):54–61. doi:10.1016/j.pcad.2018.05.002
- Smethers AD, Rolls BJ. Dietary management of obesity: cornerstones of healthy eating patterns. Med Clin North Am. 2018;102(1):107–124. doi:10.1016/j.mcna.2017.08.009
- Sanches Machado d’Almeida K, Ronchi SS, Zuchinali P, Corrêa Souza G. Mediterranean diet and other dietary patterns in primary prevention of heart failure and changes in cardiac function markers: a systematic review. Nutrients. 2018;10(1):58. doi:10.3390/nu10010058
- Stein DJ, Szatmari P, Gaebel W, et al. Mental, behavioral and neurodevelopmental disorders in the ICD-11: an international perspective on key changes and controversies. BMC Med. 2020;18(1):21. doi:10.1186/s12916-020-1495-2
- Mollayeva T, Thurairajah P, Burton K, Mollayeva S, Shapiro CM, Colantonio A. The Pittsburgh Sleep Quality Index as a screening tool for sleep dysfunction in clinical and non-clinical samples: a systematic review and meta-analysis. Sleep Med Rev. 2016;25:52–73. doi:10.1016/j.smrv.2015.01.009
- Logue JB, Stedmon CA, Kellerman AM, et al. Experimental insights into the importance of aquatic bacterial community composition to the degradation of dissolved organic matter. ISME J. 2016;10(3):533–545. doi:10.1038/ismej.2015.131
- Segata N, Izard J, Waldron L, et al. Metagenomic biomarker discovery and explanation. Genome Biol. 2011;12(6):R60. doi:10.1186/gb-2011-12-6-r60
- Schnabl B, Brenner DA. Interactions between the intestinal microbiome and liver diseases. Gastroenterology. 2014;146(6):1513–1524. doi:10.1053/j.gastro.2014.01.020
- Qin N, Yang F, Li A, et al. Alterations of the human gut microbiome in liver cirrhosis. Nature. 2014;513(7516):59–64. doi:10.1038/nature13568
- Matenchuk BA, Mandhane PJ, Kozyrskyj AL. Sleep, circadian rhythm, and gut microbiota. Sleep Med Rev. 2020;53:101340. doi:10.1016/j.smrv.2020.101340
- Carter JK, Bhattacharya D, Borgerding JN, Fiel MI, Faith JJ, Friedman SL. Modeling dysbiosis of human NASH in mice: loss of gut microbiome diversity and overgrowth of Erysipelotrichales. PLoS One. 2021;16(1):e0244763. doi:10.1371/journal.pone.0244763
- Kim DS, Ko B-S, Ryuk JA, Park S. Protected against memory dysfunction by elevating hippocampal amyloid-β deposition through potentiating insulin signaling and altering gut microbiome composition. Int J Mol Sci. 2020;21(8):2900.
- Mohammadi Z, Bishehsari F, Masoudi S, et al. Association between sleeping patterns and mealtime with gut microbiome: a pilot study. Arch Iran med. 2022;25(5):279–284. doi:10.34172/aim.2022.46
- Machiels K, Joossens M, Sabino J, et al. A decrease of the butyrate-producing species Roseburia hominis and Faecalibacterium prausnitzii defines dysbiosis in patients with ulcerative colitis. Gut. 2014;63(8):1275–1283. doi:10.1136/gutjnl-2013-304833
- Nishiwaki H, Hamaguchi T, Ito M, et al. Short-Chain fatty acid-producing gut microbiota is decreased in parkinson’s disease but not in rapid-eye-movement sleep behavior disorder. mSystems. 2020;5(6). doi:10.1128/mSystems.00797-20
- Jia X, Lu S, Zeng Z, et al. Characterization of gut microbiota, bile acid metabolism, and cytokines in intrahepatic cholangiocarcinoma. Hepatology. 2020;71(3):893–906. doi:10.1002/hep.30852
- Nishida K, Sawada D, Kuwano Y, Tanaka H, Rokutan K. Health benefits of Lactobacillus gasseri CP2305 tablets in young adults exposed to chronic stress: a randomized, double-blind, placebo-controlled study. Nutrients. 2019;11(8):1859. doi:10.3390/nu11081859
- Li Q, Li Y, Wang Y, et al. Oral administration of promotes antitumor efficacy via dendritic cells-derived interleukin 12. Oncoimmunology. 2021;10(1):1868122. doi:10.1080/2162402X.2020.1868122
- Zhao Y, Shu Y, Zhao N, et al. Insulin resistance induced by long-term sleep deprivation in rhesus macaques can be attenuated by Bifidobacterium. Am J Physiol Endocrinol Metab. 2022;322(2):E165–e172. doi:10.1152/ajpendo.00329.2021