Abstract
Background
Bedtime procrastination (BP) has become an important factor affecting individual well-being. This study aimed to assess the stability and changes in BP and examine risk and protective factors.
Methods
The study recruited 1423 respondents. Latent profile analysis was used to identify subgroups of BP and latent transition analysis to determine transition probabilities for each subgroup. Logistic regression examined associations between identified classes and related factors.
Results
Three subgroups of BP were identified. In terms of stability and changes, the moderate bedtime procrastination group showed the highest stability (66%), followed by the severe bedtime procrastination group (62.4%), and the mild bedtime procrastination group had a 52% probability of switching to moderate bedtime procrastination. In terms of influencing factors, more problematic phone use (PSU) (OR: 1.08; 95% CI = 1.05–1.12), more depression (OR: 1.17; 95% CI = 1.06–1.29) and anxiety (OR: 1.16; 95% CI = 1.05–1.28) are all factors that aggravate the transition from mild to moderate sleep procrastination. Similarly, PSU (OR: 1.15; 95% CI = 1.12–1.19), anxiety (OR: 1.10; 95% CI = 1.06–1.14), and depression (OR: 1.10; 95% CI = 1.06–1.14) increased the risk of severe bedtime procrastination. Self-control emerged as a protective factor against BP.
Conclusion
This study identified three subgroups of BP at two time points and the rule of transition for each subgroup. Our findings indicate that BP were relatively stable, with some changes over time. The results also highlight the important function that PSU, depression, anxiety, and self-control can play in preventing and intervening in BP.
Introduction
Adequate sleep is crucial for maintaining health and preventing diseases.Citation1 A significant number of undergraduate students report poor sleep quality,Citation2 and over 30% of adult Americans experience insufficient sleep.Citation3 Sleep insufficiency is linked to adverse health issues, including mood disorders,Citation4 cognitive impairment, weakened immunity,Citation5 and obesity.Citation6 This sleep deprivation also leads to diminished work efficiency,Citation7 and poor academic performance,Citation8 negatively impacting university students’ mental and physical well-being, as well as their academic and personal lives. Recent research identifies bedtime procrastination (BP) as a direct cause of insufficient sleep.Citation9 A study among Chinese undergraduates found a positive correlation between higher BP, reduced sleep duration, and poorer sleep quality.Citation10 BP is failing to go to bed at the intended time without external impediments.Citation11 BP is prevalent among Chinese college students, which have been linked with higher levels of depression, fatigue and sleep problem.Citation12,Citation13 However, discussion on BP’s contributing factors remains limited, and there’s a lack of longitudinal studies identifying predictors of BP. Exploring BP’s growing trend is vital for understanding insufficient sleep and its impact on university students’ lives. Studying its contributing factors will lead to effective prevention strategies.
Recent research highlights various factors contributing to BP.Citation12,Citation14–16 Understanding BP from a perspective of protective and risk factors is crucial for early risk assessments and prevention. Identified risk factors include problematic smartphone use (PSU),Citation17 negative emotions, biological rhythms,Citation18 and immersive activities before bed.Citation19 PSU, characterized by uncontrollable cravings impairing daily functioning,Citation14 is a primary factor contributing to BP. Belongingness theory suggests that humans crave lasting, meaningful relationships. Concurrently, gratifications theory highlights that smartphones initially meet these social needs due to their convenience, leading to satisfaction and happiness. However, as satisfaction diminishes with repetitive use, individuals often delay sleep to prolong smartphone use and maintain these social connections.Citation17 Negative emotions, referring to dissatisfaction, failures, and adverse life events, include symptoms of depression, anxiety, and stress.Citation20 These emotions can lead to bedtime delays, resulting in BP.Citation15,Citation16 For instance, studies have shown that sleep-related anxiety—sleep effort, including cognitive (“I must sleep”) and behavioral (“trying very hard to sleep”) —can lead to individuals taking longer to fall asleep. Despite these factors, some people still maintain regular bedtime routines, suggesting that protective factors can prevent sleep procrastination. Recent studies have found that self-control, the ability to resist external and internal temptations, may act as a protective factor that can enhance sleep quality and habits, both in the general population and in specific groups such as adults with ADHD.Citation12,Citation21
Most research on BP among Chinese undergraduates has been cross-sectional.Citation13,Citation15 However, BP is dynamic, influenced by individual environments, psychological states, and behaviors, necessitating longitudinal studies. Previous research predominantly used variable-centered approaches, which may not capture subtle changes over time.Citation22 Our longitudinal study seeks to fill the gap in understanding potential categorical transitions in sleep procrastination. We hope to contribute valuable insights into this under-explored area. Latent Transition Analysis (LTA) is a person-centered statistical method that can identify and track latent classes that change over time, and analyze the probabilities of transitioning from one latent state to another. This kind of analysis helps to understand the trends and processes of change in individuals’ behavioral patterns, psychological states, or other characteristics over a specific period.Citation23 Through LTA, researchers can explore the underlying factors that affect these transitions, providing a basis for the development of preventive and intervention measures. This study aims to explore BP patterns among first- and second-year college students and examine BP’s latent class transition patterns using LTA, investigating changes in parenting attitudes over time and identifying risk and protective factors. The following hypotheses were formulated: a) college students exhibit considerable heterogeneity in BP, which can be categorized into distinct subgroups; b) the BP subgroups would maintain a certain degree of stability and that the mild BP group would display the highest level of stability; c) participants with higher levels of PSU, depression and anxiety are more likely to have an exacerbation of BP, while self-control serves as a protective factor against BP.
Methods
Participants
Participants were invited to anonymously complete a questionnaire on the Wenjuanxing platform during each survey wave. Wenjuanxing is an online crowdsourcing platform in China. They provided voluntary informed consent prior to completing self-report online questionnaires on the Sleep Procrastination Scale, Mobile Phone Addiction Index, Depression Anxiety Stress Scales-21, and Brief Self-Control Scale. Participation was entirely voluntary, and all information provided was kept strictly confidential. The initial survey, conducted in December 2021 (T0), received 1663 responses. After removing duplicates, incomplete, or inconsistent responses (such as systematic answers or incorrect basic information), and questionnaires with a response time under 120 seconds, 1593 valid questionnaires remained, yielding an effective response rate of 95.8%. A follow-up survey in June 2022 (T1) matched participants from the two waves based on their school and student ID. After screening, 1423 valid questionnaires were collected, with an effective response rate of 89.3%. All participants were from the School of mental health, School of nursing, and School of public health and management. This study received ethical approval from the Institutional Review Board of the Wenzhou Medical University (Approval Number: 2022–028), dated January 7, 2022. The Declaration of Helsinki was followed in carrying out the study’s methods. In the initial phase of our study aimed at the general population, preliminary activities commenced prior to the formal ethical approval due to administrative delays. These preliminary tasks were deemed non-intrusive. We have implemented strict procedural checks to ensure all ethical guidelines are adhered to from the project outset.
Measures
Bedtime Procrastination
To assess collegiate sleep procrastination behaviors, we employed the Sleep Procrastination Scale, developed by KroeseCitation24 and later adapted by Xu Yanjing.Citation25 This 8-item instrument uses a 5-point Likert scale, ranging from 1 (never) to 5 (always). Higher scores indicate a greater tendency to delay bedtime. The scale’s strong psychometric properties align closely with actual sleep procrastination patterns among Chinese college students.Citation25 In this study, the Cronbach’s alpha values were 0.91 and 0.92 at T0 and T1, respectively.
Problematic Smartphone Use
The Mobile Phone Addiction Index (MPAI),Citation26 a 17-item scale rated from 1 (“never”) to 5 (“always”), was used to assess PSU among undergraduates. Scores range from 17 to 85, with higher scores indicating more severe addiction. A score above 8 items suggests a positive diagnosis. The scale’s reliability and validity are well-established among Chinese college students.Citation27 In this study, the Cronbach’s alpha values for the MPAI were 0.90 at T0.
Negative Emotions
The Depression Anxiety Stress Scales-21 (DASS-21) assesses symptoms of depression, anxiety, and stress.Citation28 It consists of 21 questions divided into three 7-item subscales. Responses are on a 4-point Likert scale, from 0 (not at all) to 3 (very much). Scores range from 0 to 63. The DASS-21 is known for its robust reliability and validity, making it apt for gauging the mental health of Chinese college students.Citation29 In this study, the Cronbach’s alpha values for the subscales were 0.92 (DASS 21-D), 0.89 (DASS 21-A), and 0.91 (DASS 21-S) at T0.
Self Control
The Brief Self-Control Scale, originally developed by Tangney and modified by Morean,Citation30 was used to measure self-control. This scale features two dimensions, Self-Discipline and Impulse Control, across 7 items. Responses are on a 5-point Likert scale from 1 (strongly disagree) to 5 (strongly agree), with higher scores indicating better self-regulatory abilities. The Chinese adaptation by Luo Tao et al shows commendable psychometric properties.Citation31 In this study, the Cronbach’s alpha values were 0.65 at T0.
Statistical Analyses
Models were estimated in M-plus Version 8.0. BP behaviour profiles at T0 and T1 and their changes from T0 to T1 were identified by using LTA. First, potential categories were identified using Latent Profile Analysis (LPA) on 1423 college students to identify homogeneous unobserved subgroups. The analysis included 8 BP variables. Models were compared to determine the optimal number of classes using criteria by Lubke and Muthén,Citation32 starting with a one-class model and incrementally adding classes. The Akaike Information Criterion (AIC) and Bayesian Information Criterion (BIC) were used, with lower values indicating better fit. An entropy value above 0.8 suggests over 90% classification accuracy.Citation33 We also considered the bootstrapped Likelihood Ratio test (BLRT) and Lo-Mendell-Rubin test (LMR). A significant BLRT or LMR at k classes (p < 0.05) signifies that the k-class model is an improvement over the k-1 class model.Citation34 Next, we assessed the stability and transition probabilities of BP subgroups from T0 to T1. Finally, multinomial logistic regression in SPSS 22.0 was used to estimate the predictive effects of depression, anxiety, stress, self-control, and PSU on BP subgroup transitions, interpreted as odds ratios (ORs). Statistical significance was set at the 5% level (two-tailed test).
Results
Descriptive Statistics
Of the 1423 respondents, 80.6% were female, and 851 (59.8%) lived in rural areas. The majority had siblings (n = 892, 62.7%). At T0 and T1, the average time to go to bed was 22:45 and 22:56 pm, the time to fall asleep was 23:40 and 23:53 pm, and the average time to wake up was 7:36 and 8:02 am, respectively. The average duration of using smartphone before sleep was 3.8 and 3.9 hours (see, ).
Table 1 Demographic Characteristics of the Sample
Latent Class and Transition Model of Bedtime Procrastination
The LPA model’s fit indices for both time points are detailed in . The AIC and BIC values consistently decreased as the number of latent classes increased at both T0 and T1. However, in the fourth and fifth categories, a subgroup consisting of less than 10% of the total sample size was present, leading to the exclusion of these categories. At T0, the third category had a slightly lower entropy than the second, but still maintained a classification accuracy greater than 90%. Considering the consistency across both time points and the model’s simplicity, a three-class solution was selected, aligning with the guideline that entropy values around 0.80 or higher indicate at least 90% correct classification.Citation30
Table 2 Fit Indices of the for the LPA Models (T0 to T1), for Increasing Number of Classes (1 to 5)
LPA revealed three distinct profiles. and illustrate the conditional item probabilities for each class for the three time solution. These probabilities provide insight into the characteristics of the three different BP profiles among college students. The LPA model results at T0 and T1 revealed consistent trends in conditional item probabilities for class 1, leading to its labeling as the mild BP group. This group had the lowest probability of high scores on all observed indicators. Conversely, class 3, labeled the severe BP group, displayed high probabilities across all indicators, followed by the moderate BP group.
Figure 1 Latent profile indicator conditional means for the three‐profile solution at T0.A line chart that shows the mean scores on 8 items of bedtime procrastination for three subgroups identified by the Latent Profile Analysis (LPA) method at baseline (T0). The third subgroup has the highest mean score and is named the High Bedtime Procrastination group, the second subgroup has the next highest score and is named the Moderate Bedtime Procrastination group, and the first subgroup has the lowest mean score, named the Low Bedtime Procrastination group.
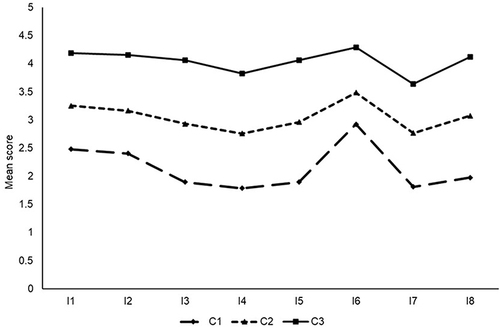
Figure 2 Latent profile indicator conditional means for the three‐profile solution at T1. A line chart that shows the mean scores on 8 items of bedtime procrastination for three subgroups identified by the Latent Profile Analysis (LPA) method at T1. The third subgroup has the highest mean score and is named the High Bedtime Procrastination group, the second subgroup has the next highest score and is named the Moderate Bedtime Procrastination group, and the first subgroup has the lowest mean score, named the Low Bedtime Procrastination group.
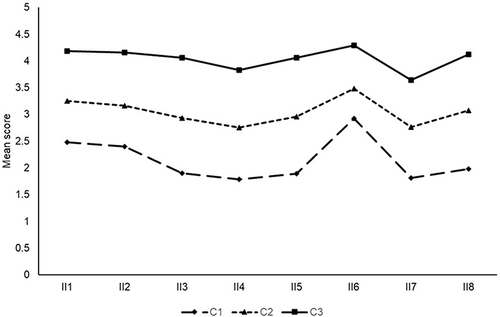
The transition matrix’s diagonal represents the probability of subjects maintaining their original latent state at T0 and T1 (see, ). The moderate BP group exhibited the highest stability, with a 66% probability of subjects remaining in the same group. For the mild and severe BP groups, these probabilities were 39.2% and 62.4%, respectively. Over time, participants in the mild and moderate groups tended to progress to the moderate and severe groups, with shift probabilities of 52% and 27.1%, respectively. Notably, the severe BP group showed a 33.9% probability of reduced symptoms over time.
Table 3 Latent Transition Probabilities Examining Continuity and Change from T0 to T1
Predictors of Bedtime Procrastination Subgroups Transition
The study also examined PSU, depression, anxiety, self-control, and stress as predictors of transitions in BP subgroups (see, ). The reference category was the group that maintained its original latent profile, with gender as a controlled factor. The results indicated that higher levels of PSU (OR: 1.08; 95% CI = 1.05–1.12) were associated with an increased likelihood of transitioning from mild to moderate BP. Higher levels of depression (OR: 1.17; 95% CI = 1.06–1.29) and anxiety (OR: 1.16; 95% CI = 1.05–1.28) also raised the risk of this transition. Similarly, PSU (OR: 1.15; 95% CI = 1.12–1.19), anxiety (OR: 1.10; 95% CI = 1.06–1.14), and depression (OR: 1.10; 95% CI = 1.06–1.14) increased the risk of individuals with mild and moderate BP progressing to severe BP. Interestingly, self-control emerged as a protective factor against BP, suggesting that greater self-control reduced the likelihood of severe BP.
Table 4 Results of Multinomial Logistic Regressions on Transition Probabilities
Discussion
In this study, we explored the various dimensions of BP from an individual-centered perspective, and classifying potential categories among college students. Additionally, we examined the trend of BP over time and the predictive effects of PSU, depression, anxiety, stress, and self-control on shifts in BP categories.
Subgroups of Bedtime Procrastination
Using a person-centered approach, LPA, we identified three latent classes of BP: mild, moderate, and severe. This classification underscores the hierarchical nature of BP among college students and highlights differences in procrastination types, which is consistent with our first hypothesis. The severe group, characterized by high endorsement of procrastination items, likely represents the most serious procrastinators with potential issues in self-regulation and resistance to temptation.Citation24 These students might struggle with procrastination across various domains, exhibit lower conscientiousness,Citation35 and have difficulties in managing time effectively. Specifically, individuals in the severe group often struggle to meet deadlines for various tasks resulting in significant delays in both their daily activities and academic obligations.Citation36,Citation37 The moderate group, characterized by lower endorsement of procrastination items, may benefit from positive reinforcement to transition towards the lowest procrastination group. Targeted interventions could help prevent their procrastination tendencies from escalating. In addition, targeted interventions are required to prevent escalation. Lastly, the mild group demonstrates the lowest endorsement of procrastination items. Individuals in this group exhibit minimal procrastination tendencies in their daily activities and appear to excel in time management and decision-making.
Stability and Change Patterns of Bedtime Procrastination Subgroups
The moderate and severe BP groups remained relatively stable over time, which is inconsistent with the second hypothesis. Due to the distinctive class characteristics and labels, the transition patterns primarily indicated stability rather than significant changes. More than 60% of participants in these groups retained their initial classification after 6 months. Notably, a substantial portion of college students (52%) transitioned from mild to moderate BP, suggesting a worsening of symptoms. This suggests that most BP does not gradually decrease over time. This pattern aligns with the temporal self-regulation theory, suggesting that environmental cues and past behaviors contribute significantly to sleep procrastination when individual sleep pressure is insufficient.Citation38 For example, postponing daily activities that have to be completed before bedtime (eg, brushing teeth, washing face, removing makeup, etc.) or the immersive behavior of being fully focused on an electronic device can serve as behavioral cues that interfere with healthy sleep habits. The repeated occurrence of these bad habits will slowly turn into an ongoing automated behavior, which eventually evolves into BP with behavioral advantages. It has been suggested that immersive use of cell phones at bedtime and BP may be causally related to each other and create a vicious cycle, as smartphones have a powerful accessibility that allows for immediate gratification of an individual’s needs, thus prolonging BP.Citation17 In addition, long-term BP behavioral habits will also cause changes in biotemporal patterns, thus further reinforcing the occurrence of sleep procrastination behavior.Citation39
Multiple Risk and Protective Factors of Sleep Procrastination
Among individual characteristics, early students who exhibited higher levels of PSU were more prone to moderate or severe BP. Increased PSU predicted transitions from low to moderate and moderate to severe procrastination, while decreasing the likelihood of transitioning from severe to moderate levels. These results suggested that PSU predict subsequent BP, consistent with other studies,Citation10,Citation14 and with the conceptual model theory of procrastination which highlights the impact of temptation on procrastination.Citation40 This suggests a direct relationship between PSU and BP and reducing PSU would reduce BP.Citation17 The reason for these findings could rest on three possible explanations. First, mobile phone addiction can affect self-control which plays an important role in procrastination.Citation41 Second, the substitution hypothesis theory of the internet points that a person spends more time and energy on his mobile phone, the less energy and time he can have to put on other activities (eg sleep).Citation42 Third, blue light from mobile phones would affect the secretion of melatonin, causing our brains to be in a state of arousal, which in turn further strengthens the degree of BP.Citation43
Among individual characteristics, this study found that students with high self-control were less likely to engage in moderate or severe BP. A systematic review and meta-analysis revealed that BP had a moderate negative association with self-control.Citation12 In contrast, we found that the increase in self-control predicted a transition from heavy to moderate or low BP. These results suggested that self-control predict subsequent BP, consistent with temporal motivation theory.Citation44 Previous findings suggest that late-night entertainment is often one of people’s short-term goals, ie, people are faced with the decision problem of late-night entertainment versus going to bed, and individuals with low self-control favour short-term goals when making choices.Citation45
With respect to state factors, early people who experienced higher levels of anxiety and depression were more likely to be moderate and heavy BP. In other words, high levels of anxiety and depression increases the probability of a transition from low to moderate BP and moderate to heavy BP; while it significantly reduced the probability of a transition from heavy to moderate BP. These results suggested that anxiety and depression predict subsequent BP, consistent with other studiesCitation19 and with the conceptual model theory of procrastination which suggests that people in negative moods are more likely to delay.Citation40 In accordance with the internalization of conflicts model, it is proposed that negative emotions trigger heightened emotional arousal, subsequently leading to physiological hyper-arousal, which, in turn, hinders the ability to initiate sleep.Citation46 BP as a sleep-related issue, has been observed to be linked with negative emotional states.Citation47 Procrastination behaviors are a form of misregulation to alleviate emotions. The theory of short-term emotional repair suggests that in order to repair a bad experience in the moment, an individual will get pleasure in the form of recreational ways, which produces procrastination.Citation48 Avoidance behavior is a direct way of reducing the experience of anxiety when faced with an event that makes the individual feel challenged, threatened and distressed.Citation49 Previous research highlights the emotional‐related outcome of procrastination.Citation26 However, our studies, along with some studies, found that elevated depression and anxiety symptoms, in turn, were related to subsequent increases in BP.Citation50
Intervention Implications for Bedtime Procrastination
The associations between depression, anxiety, PSU, and self-control with BP underscore the need for targeted interventions. First, addressing mood disorders such as depressive and anxious in young people is crucial, as they are linked with higher rates of BP. Second, promoting awareness about the harms of PSU and encouraging good sleep habits are essential as the cross-lag analysis revealed a significant bidirectional relationship between problematic cell phone use and sleep procrastination.Citation14 University educators should publicize the potential harms of PSUs and sleep delays, such as brochures, presentations, and other related practices to reduce the amount of time spent on smartphone use each day, and organize dormitory sleep support groups to help students reduce their smartphone use in the evening and develop good habits of going to bed on time. Last but not least, enhancing self-control through methods such as cognitive-behavioral therapy could be beneficial in mitigating compulsive behaviors, including excessive internet use.Citation51
Limitations
This study presents several limitations, with a key concern being the gender imbalance within our sample. It is important to acknowledge that our study employed a convenience sampling method rather than random sampling, which may have exacerbated the pronounced gender disparity. This could introduce selection bias and limit the generalizability of our findings. Therefore, it is imperative to exercise caution when interpreting the results and deriving recommendations. Future research should prioritize the use of random sampling methods to enhance the external validity of our findings. In addition, the current study design consisted of only two assessments over a 6-month interval. And the measured time point is close to the final exam, which may have an impact on the sleep time of undergraduates. To address these limitations, future investigations should employ a more comprehensive, data-rich approach, including longer-term, multi-wave assessments and a broader time frame. Additionally, conducting in-depth interviews may provide deeper insights into the developmental trajectories of BP concerning PSU, negative mood, and self-control.
Conclusion
The present study is pioneering an investigation into the transition and stability of the three BP classes within a substantial cohort of undergraduates in China. The moderate and severe BP remained relatively stable over time. It was confirmed that increased PSU and higher levels of anxiety and depression has temporal linkages with unfavorable transitions in BP over half year. Notably, individuals with high levels of self-control exhibited better outcomes, particularly among those classified within the moderate or severe BP groups. Consequently, it is imperative to accurately assess PSU, negative affective states, and self-control as pivotal factors in the psychosocial development of undergraduates’ BP. These results put forward future directions on targeted interventions for young adults who are troubled by BP.
Ethics Approval and Consent to Participate
This study was conducted in accordance with the ethical principles outlined in the Declaration of Helsinki and was approved by the Institutional Review Board of the Wenzhou Medical University (Approval Number: 2022-028), dated January 7, 2022, which adheres to international ethical standards. In the initial phase of our study aimed at the general population, preliminary activities commenced prior to the formal ethical approval due to administrative delays. These preliminary tasks were deemed non-intrusive. We have implemented strict procedural checks to ensure all ethical guidelines are adhered to from the project outset. All data in this study were kept confidential.
Author Contributions
Lan Hong and Huihui Xu have contributed equally to this work and share first authorship. All authors made a significant contribution to the work reported, whether that is in the conception, study design, execution, acquisition of data, analysis and interpretation, or in all these areas; took part in drafting, revising or critically reviewing the article; gave final approval of the version to be published; have agreed on the journal to which the article has been submitted; and agree to be accountable for all aspects of the work.
Disclosure
The authors have no competing interests to declare.
Acknowledgment
We would like to extend our thanks to the undergraduates who participated in this research.
Additional information
Funding
References
- Goel N, Rao H, Durmer JS, Dinges DF. Neurocognitive consequences of sleep deprivation. Semin Neurol. 2009;29(4):320–339. doi:10.1055/s-0029-1237117
- Seun-Fadipe CT, Mosaku KS. Sleep quality and psychological distress among undergraduate students of a Nigerian university. Sleep Health. 2017;3(3):190–194. doi:10.1016/j.sleh.2017.02.004
- Loft MH, Cameron LD. Using mental imagery to deliver self-regulation techniques to improve sleep behaviors. Annal behav med. 2013;46(3):260–272. doi:10.1007/s12160-013-9503-9
- Da Costa D, Dritsa M, Verreault N, Balaa C, Kudzman J, Khalifé S. Sleep problems and depressed mood negatively impact health-related quality of life during pregnancy. Arch Women’s Mental Health. 2010;13(3):249–257. doi:10.1007/s00737-009-0104-3
- Irwin MR. Why sleep is important for health: a psychoneuroimmunology perspective. Annual Revi Psych. 2015;66:143–172. doi:10.1146/annurev-psych-010213-115205
- Owens JA, Weiss MR. Insufficient sleep in adolescents: causes and consequences. Minerva Pediatrica. 2017;69(4):326–336. doi:10.23736/S0026-4946.17.04914-3
- Kessler RC, Berglund PA, Coulouvrat C, et al. Insomnia and the performance of US workers: results from the America insomnia survey. Sleep. 2011;34(9):1161–1171. doi:10.5665/SLEEP.1230
- Jiang F, VanDyke RD, Zhang J, Li F, Gozal D, Shen X. Effect of chronic sleep restriction on sleepiness and working memory in adolescents and young adults. J Clin Exper Neuropsych. 2011;33(8):892–900. doi:10.1080/13803395.2011.570252
- Ma X, Meng D, Zhu L, et al. Bedtime procrastination predicts the prevalence and severity of poor sleep quality of Chinese undergraduate students. J Am Coll Health. 2022;70(4):1104–1111. doi:10.1080/07448481.2020.1785474
- Zhang MX, Wu AMS. Effects of smartphone addiction on sleep quality among Chinese university students: the mediating role of self-regulation and bedtime procrastination. Addict Behav. 2020;111:106552. doi:10.1016/j.addbeh.2020.106552
- Kroese FM, Evers C, Adriaanse MA, de Ridder DTD. Bedtime procrastination: a self-regulation perspective on sleep insufficiency in the general population. J Health Psychol. 2016;21(5):853–862. doi:10.1177/1359105314540014
- Hill VM, Rebar AL, Ferguson SA, Shriane AE, Vincent GE. Go to bed! A systematic review and meta-analysis of bedtime procrastination correlates and sleep outcomes. Sleep Medicine. 2022;66:101697. doi:10.1016/j.smrv.2022.101697
- Cui GH, Yin YT, Li SJ, et al. Longitudinal relationships among problematic mobile phone use, bedtime procrastination, sleep quality and depressive symptoms in Chinese college students: a cross-lagged panel analysis. BMC Psych. 2021;21(1):449. doi:10.1186/s12888-021-03451-4
- Busch PA, McCarthy S. Antecedents and consequences of problematic smartphone use: a systematic literature review of an emerging research area. Comp Human Behav. 2021;114:106414.
- Campbell RL, Bridges AJ. Bedtime procrastination mediates the relation between anxiety and sleep problems. J Clinl Psych. 2023;79(3):803–817. doi:10.1002/jclp.23440
- Deng YH, Ye BJ, Yang Q. COVID-19 related emotional stress and bedtime procrastination among college students in China: A moderated mediation model. Nature and Science of Sleep. 2022;14:1437–1447. doi:10.2147/NSS.S371292
- Geng YG, Gu JJ, Wang J, Zhang RP. Smartphone addiction and depression, anxiety: the role of bedtime procrastination and self-control. J Affective Dis. 2021;293:415–421. doi:10.1016/j.jad.2021.06.062
- Kühnel J, Syrek CJ, Dreher A. Why don’t you go to bed on time? A daily diary study on the relationships between chronotype, self-control resources and the phenomenon of bedtime procrastination. Front Psych. 2018;9(77). doi:10.3389/fpsyg.2018.00077
- Chung SJ, An H, Suh S. What do people do before going to bed? A study of bedtime procrastination using time use surveys. Sleep. 2020;43(4). doi:10.1093/sleep/zsz267
- Parkitny L, McAuley J. The Depression Anxiety Stress Scale (DASS). J Physiother. 2010;56(3):204. doi:10.1016/S1836-9553(10)70030-8
- Öf U, Bahar A. The RELATIONSHIP BETWEEN ATTENTION DEFICIT HYPERACTIVITY DISORDER SYMPTOMS AND BEDTIME PROCRAstination. J Contemp Med. 2023;13(2):241–246. doi:10.16899/jcm.1242778
- Bergman LR, Trost K. The person-oriented versus the variable-oriented approach: are they complementary, opposites, or exploring different worlds? Merrill Quarter-J Develop Psych. 2006;52(3):601–632. doi:10.1353/mpq.2006.0023
- Lanza ST, Patrick ME, Maggs JL. Latent transition analysis: Benefits of a latent variable approach to modeling transitions in substance use. J Drug Issues. 2010;40(1):93–120. doi:10.1177/002204261004000106
- Kroese FM, De Ridder DTD, Evers C, Adriaanse MA. Bedtime procrastination: introducing a new area of procrastination. Front Psych. 2014;5. doi:10.3389/fpsyg.2014.00611
- Yanjing X Study on the concept and mechanism of sleep time delay behavior in college students; 2017.
- Leung L. LINKING PSYCHOLOGICAL ATTRIBUTES TO ADDICTION AND IMPROPER USE OF THE MOBILE PHONE AMONG ADOLESCENTS IN HONG KONG. J Children & Media. 2008;2(2):93–113. doi:10.1080/17482790802078565
- Li WF, Zhang XT, Chu MH, Li GY. The impact of adverse childhood experiences on mobile phone addiction in Chinese college students: A serial multiple mediator model. Front Psych. 2020;11:11. doi:10.3389/fpsyg.2020.00011
- Lovibond SH, Lovibond FP. Manual for the depression anxiety stress scales. Sydney. 1995;112–118.
- Lu S, Hu SQ, Guan YH, et al. Measurement invariance of the depression anxiety stress scales-21 across gender in a sample of Chinese university students. Front Psych. 2018;9(2064). doi:10.3389/fpsyg.2018.02064
- Morean ME, DeMartini KS, Leeman RF, et al. Psychometrically improved, abbreviated versions of three classic measures of impulsivity and self-control. Psych Assess. 2014;26(3):1003–1020. doi:10.1037/pas0000003
- Luo T, Cheng L, Qin L, Xiao S. Reliability and validity test of the Chinese version of the simplified self-control Scale. Chin J Clin Psychol. 2021;29(01):83–86.
- Lubke G, Muthén BO. Performance of factor mixture models as a function of model size, covariate effects, and class-specific parameters. Structural Eq Model Multidiscip J. 2007;14(1):26–47. doi:10.1080/10705510709336735
- Carragher N, Adamson G, Bunting B, McCann S. Subtypes of depression in a nationally representative sample. J Affective Dis. 2009;113(1–2):88–99. doi:10.1016/j.jad.2008.05.015
- Muthén L, Muthén B. Mplus user’s guide (version 7.2). Los Angeles, CA. 2012.
- Singh S, Bala R. Mediating role of self-efficacy on the relationship between conscientiousness and procrastination. Int J Work Organ Emotion. 2020;11(1):41–61. doi:10.1504/IJWOE.2020.109422
- Sirois FM. Out of Sight, Out of Time? A Meta‐analytic Investigation of Procrastination and Time Perspective. European J Personal. 2014;28(5):511–520. doi:10.1002/per.1947
- Asaoka S, Komada Y, Fukuda K, et al. Exploring the daily activities associated with delayed bedtime of Japanese university students. Tohoku J Exp Med. 2010;221(3):245–249. doi:10.1620/tjem.221.245
- Hall PA, Fong GT. Temporal self-regulation theory: a model for individual health behavior. Health Psychology Rev. 2007;1(1):6–52. doi:10.1080/17437190701492437
- Fu Y, Ma X, Mu L. The Physiological and Psychological Mechanisms of Bedtime Procrastination. J Psychl Sci. 2020;43(5):1190–1196.
- Maier SU, Makwana AB, Hare TA. Acute stress impairs self-control in goal-directed choice by altering multiple functional connections within the brain’s decision circuits. Neuron. 2015;87(3):621–631. doi:10.1016/j.neuron.2015.07.005
- Mao B, Chen S, Wei MC, Luo YL, Liu YL. Future time perspective and bedtime procrastination: the mediating role of dual-mode self-control and problematic smartphone use. Int J Environ Res Public Health. 2022;19(16):10334. doi:10.3390/ijerph191610334
- Lee PSN, Leung L. Assessing the displacement effects of the Internet. Telematic Informatic. 2008;25(3):145–155. doi:10.1016/j.tele.2006.08.002
- Selmaoui B, Touitou Y. Association between mobile phone radiation exposure and the secretion of melatonin and cortisol, two markers of the circadian system: A review. Bioelectromagnetics. 2021;42(1):5–17. doi:10.1002/bem.22310
- Steel P, Svartdal F, Thundiyil T, Brothen T. Examining procrastination across multiple goal stages: A longitudinal study of temporal motivation theory. Front Psych. 2018;9. doi:10.3389/fpsyg.2018.00327
- Exelmans L, Van den Bulck J. Self-control depletion and sleep duration: the mediating role of television viewing. Psychol Health. 2018;33(10):1251–1268. doi:10.1080/08870446.2018.1489048
- Baglioni C, Spiegelhalder K, Lombardo C, Riemann D. Sleep and emotions: a focus on insomnia. Sleep Med Rev. 2010;14(4):227–238. doi:10.1016/j.smrv.2009.10.007
- Sirois FM, Nauts S, Molnar DS. Self-compassion and bedtime procrastination: An emotion regulation perspective. Mindful. 2019;10(3):434–445. doi:10.1007/s12671-018-0983-3
- Sirois F, Pychyl T. Procrastination and the priority of short-term mood regulation: consequences for future self. Soc Personal Psychol Compass. 2013;7(2):115–127. doi:10.3389/fpsyg.2020.00011
- Balkis M, Duru E. Procrastination, self-regulation failure, academic life satisfaction, and affective well-being: underregulation or misregulation form. Eur J Psychol Educ. 2016;31(3):439–459. doi:10.1007/s10212-015-0266-5
- Krispenz A, Gort C, Schültke L, Dickhäuser O. How to reduce test anxiety and academic procrastination through inquiry of cognitive appraisals: A pilot study investigating the role of academic self-efficacy. Front Psychol. 2019;10:1917. doi:10.3389/fpsyg.2019.01917
- Zeidi IM, Divsalar S, Morshedi H, Alizadeh H. The effectiveness of group cognitive-behavioral therapy on general self-efficacy, self-control, and internet addiction prevalence among medical university students. Social Health Behav. 2020;3(3):93–102. doi:10.4103/SHB.SHB_20_20