Abstract
Decision analysis has become an increasingly popular decision-making tool with a multitude of clinical applications. Incorporating patient and expert preferences with available literature, it allows users to apply evidence-based medicine to make informed decisions when confronted with difficult clinical scenarios. A decision tree depicts potential alternatives and outcomes involved with a given decision. Probabilities and utilities are used to quantify the various options and help determine the best course of action. Sensitivity analysis allows users to explore the uncertainty of data on expected clinical outcomes. The decision maker can thereafter establish a preferred method of treatment and explore variables which influence the final clinical outcome. The present paper reviews the technique of decision analysis with particular focus on its application to clinical decision making.
Introduction
In the face of complex scenarios, it has been observed that many decision makers have a natural tendency to make overly optimistic, uninformed decisions, often more on the basis of intuition than a rational weighing of outcomes and probabilities.Citation1,Citation2 This phenomenon is exemplified in the clinical setting, where there is frequently the need to make intricate decisions with a limited amount of time and resources. Under such circumstances, a rigorous and objective analysis of outcomes and probabilities is essential to achieve the best possible decision given a specific clinical situation. This demand has created the need for more objective decision-making techniques, such as decision analysis. Clinical decision analysis – the application of decision analysis to a clinical or patient-based setting – is a technique that incorporates literature-derived probabilities with expert and patient preferences to result in an informed clinical decision.
Decision analysis and evidence-based medicine
Evidence-based medicine (EBM) refers to the incorporation of critically appraised scientific evidence into clinical practice.Citation3,Citation4 EBM allows users to integrate both clinical expertise and the best available evidence in the literature.Citation5,Citation6 Decision analysis is a tool that allows users to apply EBM to make informed decisions when confronted with difficult scenarios. It has been applied to a number of settings including the management of ventricular septal defects,Citation3 treatment of early osteoarthritis of the wrist,Citation7 and screening for prostate cancer.Citation8 Decision analysis, when executed correctly, has become a powerful and effective technique with a variety of clinical applications. The present paper reviews the technique of decision analysis with particular focus on its application to clinical decision making.
What is decision analysis?
The decision tree
Decision analysis is a method that uses probabilities and expected values together with a decision model to replicate a problem and help determine the best course of action.Citation3,Citation9 A decision tree is a pictorial illustration of all plausible relationships, alternatives, and outcomes in a given decision. Associated with each step in the decision tree is a corresponding probability and outcome value. Incorporating both probabilities and outcome values, the decision-analysis model expresses its conclusion in terms of the average expected result, which may be interpreted as life-years, days of treatment, cost, or other variables depending on clinical context.Citation7 By using such a tree, the decision maker can accurately weigh the outcomes associated with a given decision, thus leading to more informed clinical judgement.Citation3,Citation10
Decision analysis is most usefully applied in clinical decisions where there is uncertainty regarding appropriate clinical strategy and when a meaningful trade-off of advantages and disadvantages is present in the clinical problem.Citation9 Furthermore, decision trees are adaptable and values represent a current, and not static, benchmark on which further evolution can be critically evaluated.Citation3
Probability values: Incorporating the literature
Probabilities are a quantitative estimate of the chance or likelihood that a given outcome will occur.Citation11 In clinical decision making, reliable probabilities of clinical outcomes can be attained through a systematic and rigorous analysis of available literature, preferably randomized controlled trials or other systematic reviews. If there is a deficiency of such literature, researchers must turn to alternatives such as observational studies, expert judgment, existing databases, or unpublished work.Citation11 These probabilities are then incorporated into the decision tree to assist in the decision-making process.
Probabilities, however, are always associated with uncertainty. As such, along with the average probability for each outcome attained from the literature, defined as baseline probability, reasonable probability ranges must also be specified.Citation11,Citation12 These ranges can then be used in a sensitivity analysis to assess how different estimates can affect the final decision, as discussed later.Citation12
Outcome values: Incorporating patient and expert preferences
Perhaps the strength of decision analysis comes in its incorporation of available literature with outcome values, which are summary measurements of a particular outcome.Citation11 Depending on the clinical scenario, they are most commonly expressed as life-years, quality-adjusted life years (QALYs), costs, or utilities.Citation11 A utility is a measure of the relative preference or desirability for a given outcome, and is generally expressed as a value between 0 and 1, where 0 is the worst outcome and 1 is the best in a given clinical scenario. Utility values can be estimated by 1) arbitrary assignment of values based on expert judgment; 2) published values in the literature; or 3) patient preferences.Citation11 In the incorporation of patient preferences, it is important to note that utility values vary from patient to patient. For example, a young and active patient with a hip fracture may elect to have internal fixation, whereas a similar patient who is elderly and inactive may opt for prosthetic replacement. This difference in preference stems from a difference in utility values. Whereas the elderly patient may value pain relief and the avoidance of implant failure, the athlete values range of motion. Furthermore, as with probability values, the uncertainty of utilities can be accounted for by including a range of reasonable values and thereafter performing a scrupulous sensitivity analysis to determine the range of values for which a given outcome is preferred.Citation12
Calculating the decision tree
Once baseline probabilities and outcome values are attained from the literature, expert opinion, and/or patient preferences, the tree is ready to be calculated. Decision trees are calculated by mathematically incorporating probabilities and outcome values. Outcome values are multiplied by their respective probabilities and summed across nodes within a particular branch. In this way, the model expresses its conclusion in terms of the average expected result, which may be interpreted as life-years, days of treatment, cost, or other variables depending on clinical context.Citation7 These final values represent baseline values that can then undergo further analysis in the decision trees.
shows a decision tree for a simple hypothetical example of the hip fracture scenario described earlier, focusing on adverse events. Prosthetic replacement of the femoral head is compared with internal fixation for an elderly patient with hip fracture. Each option is associated with a number of advantages, disadvantages, costs, and preference values. For example, in comparison with internal fixation, prosthetic replacement may reduce the risk of reoperation. This however comes at the cost of greater infection rates, blood loss, operative time, and possibly mortality.Citation13 The probability of a major complication – defined as an adverse event that may be life threatening, may require major intervention, or may be associated with important long-term sequelaeCitation3 – is ideally derived from a systematic review of the literature. Utilities, or relative preferences for each outcome, are represented as variables in the right most end-nodes. The tree is calculated by multiplying each utility with its associated probability and adding across nodes.
Figure 1 Hypothetical decision tree showing two possible treatment options for an elderly patient with a hip fracture. Each treatment option is associated with either an unfavorable (major complications) or favorable (no major complications) outcome. The probability of that outcome, derived from the literature, is shown as a variable under the branch it represents. Utilities of each outcome, represented as variables, are shown at the right-most nodes. The decision tree is calculated by multiplying each utility with its associated probability and adding across nodes.
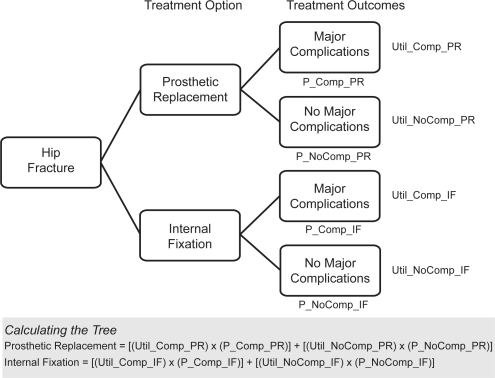
Sensitivity analysis: Accounting for error
There are three possible outcomes of a decision analysis: 1) one option is clearly better; 2) the expected values of the options are very close; or 3) no clear outcome.Citation12 Although the baseline probabilities and outcome values may show one method to be preferred over another, the difference between the options may be quite small. As such, a feature of decision analysis, called sensitivity analysis, allows users to perform decision analysis while varying probabilities and outcome values. Sensitivity analysis is the process of repeatedly rolling back the tree with different probability and outcome values, thus allowing users to explore the uncertainty of data and to examine what the effects of variability on probabilities and outcome values in the decision tree have on expected clinical outcomes.Citation12 One-way sensitivity analysis enables the decision maker to test the sensitivity of a proposed decision to simultaneous changes in the values of any independent variable in the tree, whereas multi-way analysis allows for changes in more than one independent variable. In this way, the user can then systematically manipulate baseline values to determine which factors are most important in influencing the final outcome of a given decision.Citation3,Citation7,Citation12
Limitations of decision analysis
Like any other statistical tool, decision analysis does not guarantee a correct decision; its validity and application depends entirely on the specific clinical scenario, the availability of data, and the strength and inclusion criteria of the selected literature. There are several practical impediments to the application of decision analysis.Citation13 Firstly, accurate estimates with regards to the likelihood of the outcomes of treatment are required. In areas of medicine where therapeutic options, risks and benefits of options and the frequency of common adverse events have been well studied, data can more likely be considered valid. Additionally, the measurement of accurate utility values also poses another potential limitation as internal and external biases may affect data.Citation13 In order to ensure the validity of utility estimates, a fairly rigorous methodology of deriving utilities must be in place. It should be kept in mind that the conclusions drawn from a decision analysis can be no more valid than the data used. Finally, it should be noted that clinical decision making is a complex process in which the decision maker has to take into consideration several dimensions of a given scenario. This may include treatment options, costs, side effects, social implications, patient preferences, and others. In such situations, the decision maker must prioritize the major issues that need to be considered for a given situation. In advanced cases, multiple decision trees or complex segmented trees can be constructed to allow for more rigorous decision making.
Furthermore, the results of a decision analysis must be interpreted carefully. Clinicians must define their target population and look at how closely their particular clinical situation resembles that of the analysis, the strength and reliability of the probabilities and utilities that were attained, as well as the results of the sensitivity analysis. Such information is then used by the decision maker to form an informed, objective decision regarding the specific clinical scenario. Finally, it should be noted that constructing a decision tree, along with its corresponding probabilities and utilities, may be time-consuming and require a great deal of information and rigorous analyses. However, when it is well executed, incorporating probabilities and outcome values based on accepted data and expert opinion, decision analysis is a powerful tool that has been shown to generate highly credible results.Citation3,Citation8,Citation9,Citation11,Citation12
Summary
Decision analysis is an objective, explicit method that uses models to represent specific decision problems. A decision tree, together with probabilities and outcome values, is used to determine the best course of action. Outcome probabilities are derived from a systematic and rigorous analysis of available literature. Outcome values, most often incorporating patient and expert preferences, are summary measurements of a particular outcome and are expressed in life-years, QALYs, costs, or utilities. Sensitivity analysis then allows users to explore the effects of variation on important probabilities and outcome values, and its impact on final clinical outcomes. By combining the evidence with patient and expert preferences, the decision maker can thereafter establish a preferred method of treatment and explore variables which influence the final decision. Decision analysis has thus become a powerful and effective evidence-based technique with numerous clinical applications.
Disclosure
Dr Bhandari was funded in part by a Canada Research Chair, McMaster University, Hamilton, Ontario, Canada. The authors report no conflicts of interest in this work.
References
- BonabeauEDon’t trust your gutHarv Bus Rev20038111612313012747167
- LovalloDKahnemanDDelusions of success. How optimism undermines executives’ decisionsHarv Bus Rev200381566311712858711
- AleemISKaramlouTBensonLNMcCrindleBWTranscatheter device versus surgical closure of ventricular septal defects: a clinical decision analysisCatheter Cardiovasc Interv20066763063616548005
- RosenbergWMSackettDLOn the need for evidence-based medicineTherapie1996512122178881108
- MykhalovskiyEWeirLThe problem of evidence-based medicine: directions for social scienceSoc Sci Med2004591059106915186905
- SackettDLEvidence-based medicineSpine199823108510869615357
- GrahamBDetskyASThe application of decision analysis to the surgical treatment of early osteoarthritis of the wristJ Bone Joint Surg Br20018365065411476298
- KrahnMDMahoneyJEEckmanMHTrachtenbergJPaukerSGDetskyASScreening for prostate cancer. A decision analytic viewJAMA19942727737807521400
- DetskyASNaglieGKrahnMDNaimarkDRedelmeierDAPrimer on medical decision analysis: Part 1 – Getting startedMed Decis Making1997171231259107606
- DetskyASNaglieGKrahnMDRedelmeierDANaimarkDPrimer on medical decision analysis: Part 2 – Building a treeMed Decis Making1997171261359107607
- NaglieGKrahnMDNaimarkDRedelmeierDADetskyASPrimer on medical decision analysis: Part 3 – Estimating probabilities and utilitiesMed Decis Making1997171361419107608
- KrahnMDNaglieGNaimarkDRedelmeierDADetskyASPrimer on medical decision analysis: Part 4 – Analyzing the model and interpreting the resultsMed Decis Making1997171421519107609
- BhandariMDevereauxPJSwiontkowskiMFInternal fixation compared with arthroplasty for displaced fractures of the femoral neck. A meta-analysisJ Bone Joint Surg Am200385-A91673168112954824