Abstract
Aim: To investigate the CDH1, DLEC1 and SFRP5 gene methylation panel for advanced epithelial ovarian carcinoma (EOC). Materials & methods: One hundred and seventy-seven advanced EOC specimens were evaluated by methylation-specific PCR. We also used The Cancer Genome Atlas dataset to evaluate the panel. Results: The presence of two or more methylated genes was significant in recurrence (hazard ratio [HR]: 1.91 [1.33–2.76]; p = 0.002) and death (HR: 1.96 [1.26–3.06]; p = 0.006) in our cohort. In The Cancer Genome Atlas dataset, the presence of two or three methylated genes was significant in death (HR: 1.59 [1.15–2.18]; p = 0.0047) and close to the significance level in recurrence (HR: 1.37 [0.99–1.88]; p = 0.058). Conclusion: The CDH1, DLEC1 and SFRP5 methylation panel is a potential prognostic biomarker for advanced EOC.
Graphical abstract
Epithelial ovarian cancer leads to high cancer-related mortality [Citation1–3]. Most patients are diagnosed at an advanced stage with a 5-year survival of less than 50%, due to a lack of efficient screening tools or obvious symptoms [Citation4–6]. Despite good initial response rates for primary debulking surgery and adjuvant chemotherapy, many patients suffer from tumor recurrence with a poor response to salvage treatments and eventually die of ovarian cancer [Citation4,Citation7,Citation8].
As treatments for epithelial ovarian cancer more in the direction of precision medicine, the management strategy depends on its molecular features. Antiangiogenesis and poly-ADP-ribose polymerase inhibitors are the most promising targets for ovarian cancer management. Bevacizumab in combination with chemotherapy demonstrates an improved progression-free survival, but an overall survival (OS) benefit is only observed in high-risk patients [Citation9–11]. Olaparib is approved for maintenance therapy in platinum-sensitive BRCA-mutated serous ovarian cancer patients [Citation12], and a significantly longer progression-free survival is observed in platinum-sensitive recurrent ovarian cancer patients receiving niraparib [Citation13]. However, these targeted drugs are expensive and benefit only a small subpopulation of patients. Cytotoxic chemotherapy remains the current mainstream treatment for ovarian cancer management, and it is important to identify and predict resistant patients in order to provide cost-effective treatments [Citation14,Citation15].
Epigenetic mechanisms influence gene expression without the changing the DNA coding sequence, and gene methylation is the primary mechanism for epigenetic transcriptional silencing [Citation16,Citation17]. DNA methylation is regulated by DNA methyltransferases that add methyl groups to the cytosine of the DNA chain without changing the sequence, in order to regulate the transcription of genes related to cell growth, angiogenesis and DNA repair [Citation18]. Both aberrant epigenetic and genetic alterations are viewed as crucial to carcinogenesis [Citation19,Citation20]. Gynecologic and other malignancies have varying profiles of gene methylation, which are associated with prognosis [Citation17]. Our previous study showed that CDH1, DLEC1 and SFRP5 gene methylation could be a prognostic biomarker for patients with ovarian clear cell and endometrioid carcinomas, especially in advanced-stage patients [Citation21]. The majority of epithelial ovarian cancer patients are advanced stage and serous carcinoma, therefore, we investigate the prognostic value of the gene methylation panel in advanced epithelial ovarian cancer patients and further evaluate the panel using The Cancer Genome Atlas (TCGA) dataset in the present study.
Materials & methods
Patients & specimens
The protocol was approved by the National Taiwan University Hospital Research Ethics Committee (201012111RC). Informed written consents were obtained and the methods were performed in accordance with the guidelines and regulations. We enrolled 177 women diagnosed with epithelial ovarian cancer who received debulking surgery and adjuvant chemotherapy at the National Taiwan University Hospital between January 2010 and June 2014. A portion of the cancerous tissue specimen collected during debulking surgery was immediately frozen in liquid nitrogen and stored at -70°C. The remaining tissue specimens were sent for frozen section and pathology examinations to confirm the diagnosis and ensure that tumorous tissue sufficient for the following experiments was present in the specimens. Clinical data were obtained from medical records, and included age, cancer stage, surgical findings during debulking, treatment course, recurrence and survival. The status of debulking surgery was divided to two groups as a maximal residual tumor size less than 1 cm and ≥1 cm after debulking surgery. Tumor grading was based on the International Union Against Cancer criteria, and staging was based on the International Federation of Gynecology and Obstetrics criteria [Citation22]. All patients received regular follow-ups after primary treatment, and recurrence was defined as abnormal results from imaging studies (including computerized tomography or MRI), elevated CA-125 (more than twice the upper normal limit) for two consecutive tests in 2-week intervals, or biopsy-proven disease. Patients with disease progression or recurrence ≤6 months after completing primary treatment were defined as chemoresistant, and those without recurrence or recurrence more than 6 months were defined as chemosensitive. Disease-free survival (DFS) was defined as the time from the date of primary treatment completion to the date of confirmed recurrence, disease progression or last follow-up. OS was defined as the period from surgery to the date of death associated with the disease or the date of last follow-up.
Extraction of DNA from ovarian cancer tissue
Cancer tissue samples were collected during surgery, frozen and stored at -70°C until analysis. All samples were from primary ovarian cancerous tissue, rather than peritoneal or metastatic sites. Histological examinations were performed to calculate the percentage of malignancy in the frozen tissue, and tissue containing more than 80% malignant cells were used for further analysis. Genomic DNA was isolated using a Qiagen EZ1 DNA Tissue Kit (Qiagen, CA, USA) according to the manufacturer’s instructions.
Methylation-specific PCR & capillary electrophoresis
Methylation-specific PCR (MS-PCR) of the isolated genomic DNA of the cancer tissues was performed using an EZ DNA Methylation Kit™ (Zymo Research, CA, USA), following the manufacturer’s instructions. Bisulfite-modified, Sss I (New England Biolabs, MA, USA)-treated normal lymphocyte DNA served as the methylated control, and bisulfite-treated normal lymphocyte DNA served as the unmethylated control. DNA methylation was determined after treatment by primers specific for the methylated and unmethylated alleles of each gene, and the methylation status of CDH1, DLEC1 and SFRP5 was evaluated. The primer sequences are summarized in Supplementary Table 1 [Citation21,Citation23–34].
Capillary electrophoresis (CE) was performed in order to analyze the MS-PCR products using an HDA system with a GCK-5000 cartridge kit (eGene, CA, USA). The gel matrix in the cartridge was composed of a proprietary linear polymer with ethidium bromide dye. The PCR products were diluted 20-fold with deionized water and placed in the instrument’s sample chamber. The DNA samples were injected into the capillary channels and subjected to electrophoresis according to the manufacturer’s protocol. BioCalculator Graphing software was used to automatically label peak sizes.
TCGA database
The prognostic value of CDH1, DLEC1 and SFRP5 gene methylation for ovarian cancer patients was evaluated by the microarray dataset retrieved from TCGA. The methylation microarray platform used in the TCGA dataset is Illumina 27K array, which has approximate 27K probes. The CpG sites analyzed in the three interested genes are summarized in Supplementary Table 2. For each CpG site, we calculated the 75th percentile of its β-values from all analyzed patients. Subsequently, if one patient has higher β-value than the cut-off, we classified the gene in this patient as methylated. If one patient has lower β-value than the cut-off, we classified the gene in this patient as unmethylated. If a patient has at least two methylated genes, the patient is classified into high methylation group. Alternatively, if a patient has less than two methylated genes, the patient is classified into low methylation group.
The methylation profiles and clinical outcomes of ovarian cancer patients were downloaded from the TCGA dataset. The original number of the samples with available methylation profiles is 605, and 32 samples were excluded due to duplicate samples from the same patient and incomplete clinical outcomes. Therefore, a total of 573 samples remained for further analyses. For the OS analysis, five patients have no corresponding information and thus they were excluded, which resulted in 568 patients were included in the OS analysis. For the DFS analysis, we selected the patients who have clear recurrent information to perform the analysis, and thus only 290 patients remained in the DFS analysis. A Kaplan–Meier survival curve was used to evaluate the differences of OS and DFS in these two groups. A Cox hazard regression model was utilized to examine the prognostic values of the methylation groups through a univariable analysis. To test whether this methylation group is an independent predictor, a multivariable analysis adjusting age, gender and stage was also performed by using a cox hazard regression model.
Statistical analysis
The statistical analyses were performed using the Statistical Package for Social Sciences software package (SPSS for Windows, version 15.0.0, SPSS, Inc., IL, USA). The analysis for TCGA dataset was performed in R (version 3.1.2). One-way analysis of variance (ANOVA) was used to compare continuous variables and Fisher’s exact test was used for categorical variables. Survival curves were generated using the Kaplan–Meier method, and differences were calculated using the log-rank test. A multivariate Cox’s regression model was used to evaluate the prognostic factors for DFS and OS. Benjamini–Hochberg calculation was performed to correct for multiple hypothesis testing in order to reduce the chance of false-positive findings. We applied a false discovery rate (5%) in the Benjamini–Hochberg calculation. Statistical significance was set as a p-value of less than 0.05.
Results
Clinical characteristics of the patients
One hundred and seventy-seven advanced-stage epithelial ovarian carcinoma patients were enrolled, and included 121 patients with serous adenocarcinomas, 24 patients with endometrioid adenocarcinomas and 32 patients with clear cell carcinomas (). The mean age was 55.4 years old and the median CA125 value pretreatment was 1046.5 U/ml. Approximately half of the patients (51.4%, 91/177) had residual tumor size less than 1 cm after debulking surgery, and all patients received adjuvant chemotherapy with platinum and paclitaxel regimens. The patients were followed up to September 2017. The median follow-up times were 32 months (range: 1–78). The overall frequency of CDH1, DLEC1 and SFRP5 gene methylation was 31.1% (55/177), 65.5% (116/177) and 19.2% (34/177), respectively. 30.5% (54/177) of patients had two or more methylated genes.
Table 1. Characteristics of advanced-stage patients.
Correlations between gene methylation & clinic–pathologic parameters
Representative results obtained from MS-PCR by CE are shown in . The cytosine (C) in the methylated gene does not change after application of the C–T conversion agent, and these sites are detected by the primers used for methylated genes. In contrast, the cytosine bases (C) of the CpG dinucleotides in the unmethylated gene are converted to thymidine (T), which is detected by the primers used for unmethylated genes. Genes were classified as methylated when the sample simultaneously showed a positive result with primers used for methylated genes and a negative result with primers used for unmethylated genes.
Gene methylation of DLEC1 was found in patients 1, 2 and 3, while patients 4 and 5 had unmethylated DLEC1.
†Positive findings of methylation-specific PCR products.
M: Primers for the methylated gene promoter; U: Primers for the unmethylated gene promoter.
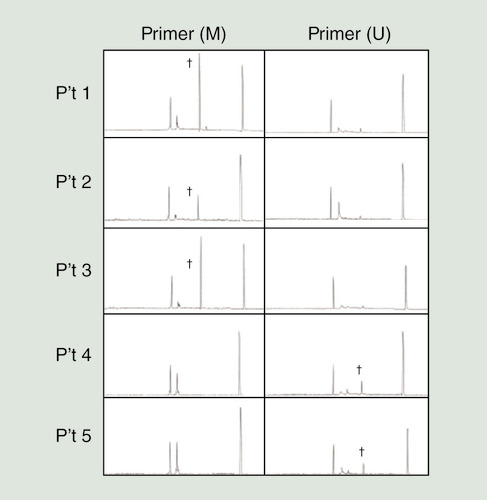
SFRP5 methylation frequency was significantly lower in patients with serous adenocarcinoma relative to those with nonserous (endometrioid and clear cell) adenocarcinoma (7.4 vs 44.6%; p = 0.004; Fisher’s exact test; ). Patients with tumor recurrence had trended toward higher frequencies of CDH1 (35.4 vs 20%) and DLEC1 methylation (70.1 vs 54.0%) than patients without tumor recurrence, although that difference did not reach statistical significance. Chemoresistant patients had trended toward higher frequencies of CDH1 (40.3 vs 25.5%), DLEC1 (71.6 vs 61.8%) and SFRP5 methylation (22.4 vs 17.3%) than chemosensitive patients, although that difference did not reach statistical significance.
Table 2. Correlations between gene methylation and clinical parameters.
Combination of CDH1, DLEC1 & SFRP5 gene methylation as prognostic marker
We further evaluated whether combinations of gene methylation correlate with clinical outcomes for these epithelial ovarian carcinoma patients (). Patients with tumor recurrence had higher frequencies of two or more methylated genes (37.8 vs 12%; p = 0.004; Fisher’s exact test; Supplementary Figure 1A) than patients without recurrence, and chemoresistant patients also had higher frequencies of two or more methylated genes (43.3 vs 22.7%; p = 0.028; Fisher’s exact test; Supplementary Figure 1B) than chemosensitive patients. Patients that died of the disease had trended toward higher frequencies of two or more methylated genes (38.4 vs 23.1%; Supplementary Figure 1C).
As shown in , patients with CDH1 methylation (p = 0.021; log-rank test; ) and DLEC1 methylation (p = 0.026; log-rank test; ) had a significantly shorter DFS. Patients with SFRP5 methylation had a significantly shorter OS (p = 0.026; log-rank test; ). Patients with two or more methylated genes had a significantly shorter DFS (p < 0.001; log-rank test; ) and OS (p = 0.001; log-rank test; ) than those with fewer than two methylated genes. In the serous ovarian cancer patients, patients with DLEC1 methylation had shorter DFS close to the significance level (p = 0.050; log-rank test; ). Patients with two or three methylated genes had a significantly shorter DFS (p = 0.004; log-rank test; ). However, patients with two or three methylated genes did not have significant different OS (p = 0.067; log-rank test; ). In the subgroup with residual tumor size less than 1 cm after debulking surgery, patients with two or more methylated genes also had a significantly shorter DFS (p = 0.001; log-rank test; Supplementary Figure 2A) and OS (p = 0.003; log-rank test; Supplementary Figure 2C) than those with fewer than two methylated genes. In the subgroup with residual tumor size ≥1 cm after debulking surgery, patients with two or more methylated genes had a significantly shorter DFS (p = 0.029; log-rank test; Supplementary Figure 2B) and a trend toward shorter OS (p = 0.115; log-rank test; Supplementary Figure 2D) than those with fewer than two methylated genes.
(A) Patients with CDH1 methylation had a significantly shorter disease-free survival (DFS; p = 0.021; log-rank test). (B) Patients with CDH1 methylation did not have significant different overall survival (OS; p = 0.313; log-rank test). (C) Patients with DLEC1 methylation had a significantly shorter DFS (p = 0.026; log-rank test). (D) Patients with DLEC1 methylation did not have significant different OS (p = 0.183; log-rank test). (E) Patients with SFRP5 methylation did not have significant different DFS (p = 0.524; log-rank test). (F) Patients with SFRP5 methylation had a significantly shorter OS (p = 0.026; log-rank test). (G) Patients with two or three methylated genes had a significantly shorter DFS (p < 0.001; log-rank test). (H) Patients with two or three methylated genes had a significantly shorter OS (p = 0.001; log-rank test).
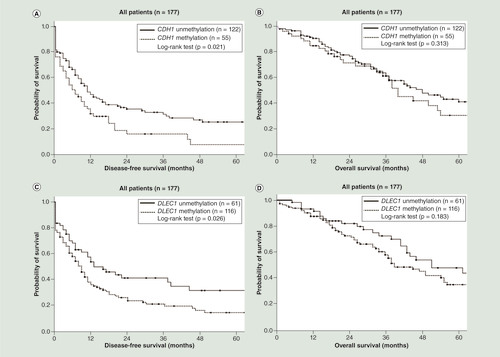
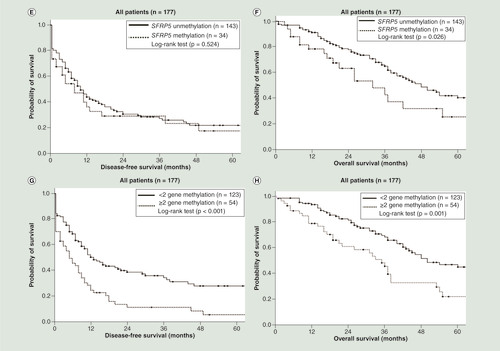
(A) Patients with CDH1 methylation did not have significant different disease-free survival (DFS; p = 0.145; log-rank test). (B) Patients with CDH1 methylation did not have significant different overall survival (OS; p = 0.758; log-rank test). (C) Patients with DLEC1 methylation had shorter DFS close to the significance level (p = 0.050; log-rank test). (D) Patients with DLEC1 methylation did not have significant different OS (p = 0.152; log-rank test). (E) Patients with SFRP5 methylation did not have significant different DFS (p = 0.781; log-rank test). (F) Patients with SFRP5 methylation did not have significant different OS (p = 0.514; log-rank test). (G) Patients with two or three methylated genes had a significantly shorter DFS (p = 0.004; log-rank test). (H) Patients with two or three methylated genes did not have significant different OS (p = 0.067; log-rank test).
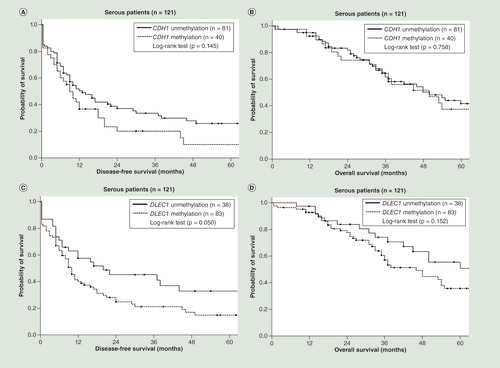
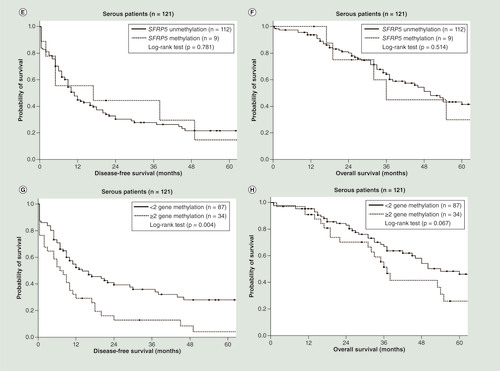
We evaluated the clinical and gene methylation factors by univariate Cox regression model and Benjamini–Hochberg calculation (). Residual tumor size less than 1 cm after debulking surgery (HR: 0.59 [0.41–0.84]; p = 0.012) and two or more methylated genes (CDH1, DLEC1 and SFRP5; HR: 1.97 [1.36–2.84]; p = 0.008) were important factors for recurrence in univariate Cox regression model analysis. Only these significant factors were included as covariates in the multivariate analysis. For recurrence, residual tumor size less than 1 cm after debulking surgery (HR: 0.60 [0.42–0.86]; p = 0.006) and two or more methylated genes (CDH1, DLEC1 and SFRP5; HR: 1.91 [1.33–2.76]; p = 0.002) were significant prognostic factors in multivariate Cox regression model.
Table 3. Cox regression model for the risk factors for recurrence and death in all patients.
In serous carcinoma (), high-grade (HR: 2.27 [1.13–4.57]; p = 0.044), residual tumor size less than 1 cm after debulking surgery (HR: 0.57 [0.37–0.87]; p = 0.030) and two or more methylated genes (CDH1, DLEC1 and SFRP5; HR: 1.85 [1.19–2.87]; p = 0.030) were important factors for recurrence in univariate Cox regression model analysis. Residual tumor size less than 1 cm after debulking surgery (HR: 0.64 [0.42–0.98]; p = 0.045) and two or more methylated genes (CDH1, DLEC1 and SFRP5; HR: 1.67 [1.06–2.60]; p = 0.023) were significant prognostic factors in multivariate Cox regression model, but the p-value was close to significant level after Benjamini–Hochberg calculation. For death, residual tumor size less than 1 cm after debulking surgery (HR: 0.37 [0.21–0.65]; p = 0.006) was the only significant factor.
Table 4. Cox regression model for the risk factors for recurrence and death in serous-type patients.
The sensitivity and specificity of gene methylation panel was calculated for outcome prediction (Supplementary Table 3). The gene methylation panel had a specificity of 88% for predicting recurrence and a specificity of 76.92% for predicting death. The regression models were performed to evaluate the predictive value of the clinical variables and the methylation panel. The methylation panel improved the coefficient of the model for recurrence and death. The methylation panel was the significant variable in the model for recurrence, and residual tumor size was the significant variable in the model for death (Supplementary Table 4).
Prognostic marker evaluation using the TCGA database
The DFS analysis was performed using 290 ovarian cancer patients with information regarding disease recurrence in the TCGA database. Fifty-one patients with two or three methylated CDH1, DLEC1 and SFRP5 genes had a significantly shorter DFS (p = 0.023; log-rank test; ) than those with fewer than two methylated genes. Data from a total of 568 ovarian cancer patients with prognosis information were retrieved for OS analysis. Eighty-eight patients with two or three methylated CDH1, DLEC1 and SFRP5 genes had a significantly shorter OS (p = 0.003; log-rank test; ) than those with fewer than two methylated genes.
(A) Patients with two or three methylated genes had a significantly shorter disease-free survival (p = 0.023; log-rank test). (B) Patients with two or three methylated genes had a significantly shorter overall survival (p = 0.003; log-rank test).
TGCA: The Cancer Genome Atlas.
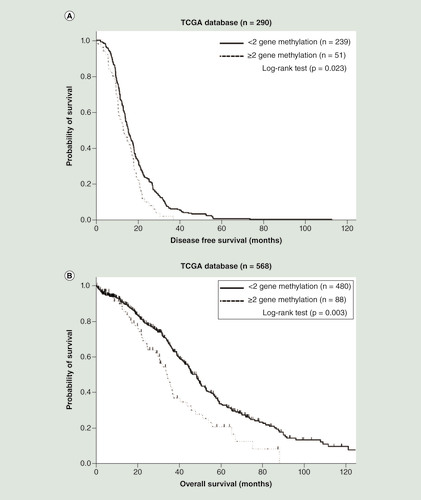
The Cox regression model was used to evaluate the performance of the panel. In a univariate Cox model, the patient with two or three methylated CDH1, DLEC1 and SFRP5 genes showed significant for both recurrence (HR: 1.42 [1.05–1.93]; p = 0.024) and death (HR: 1.60 [1.17–2.19]; p = 0.003). We put three clinical factors into the adjustment model, including age, stage and grade. In this multivariate Cox model (), the patient with two or three methylated CDH1, DLEC1 and SFRP5 genes were significant predictors in death (HR: 1.59 [1.15–2.18]; p = 0.0047) and close to the significance level in recurrence (HR: 1.37 [0.99–1.88]; p = 0.058).
Table 5. Multivariate Cox regression model for the risk factors for recurrence and death in The Cancer Genome Atlas dataset.
In the TCGA dataset, only few CpG sites in the promotor were examined by the Illumina 27K methylation array, including CDH1 (cg17655614, cg11667754), DLEC1 (cg23881725) and SFRP5 (cg05937453, cg06692050, cg25156443). We averaged the methylation level of these CpG sites and classified the patients by the same definition. However, the results from the average methylation level was not significantly associated with DFS and OS.
Discussion
Gene panel methylation including CDH1, DLEC1 and SFRP5 can act as a prognostic biomarker for advanced epithelial ovarian cancer. Epithelial ovarian cancer is viewed as a heterogenous group of diseases, involving various dysregulated molecular pathways including aberrant gene methylation that result in a variety of pathological features and clinical outcomes [Citation35]. Here, we show that patients with two or more of methylated CDH1, DLEC1 and SFRP5 genes have a poorer prognosis. The analysis of TCGA dataset by our definition was consistent with the findings of MS-PCR in our patients. Our gene methylation panel is a potential prognostic predictor in addition to conventional clinicopathologic factors, including histology, tumor grade and residual tumor volume after debulking surgery. Our primary cohort was Asian ancestry, and we used TCGA dataset to evaluate whether our findings would be generalizable. We found that only 19 patients out of the 568 patients in the OS analysis were Asian (3.3%) and only eight patients out of the 290 patients in the DFS analysis were Asian (2.7%). More than 85% of the patients in the TCGA dataset were from white population. Therefore, we believed the panel can be utilized in at least Asian and white populations.
In our study, panel of combined methylated genes is superior to single methylated gene in prognostic prediction of advanced epithelial ovarian cancer patients. The heterogeneity of epithelial ovarian cancer as well as differences in study design and experimental protocols could account for the variation in the methylation frequencies of individual genes found in the literature. Gene methylation panels are inarguably superior to individual gene methylation status as prognostic biomarkers [Citation36], and could improve the sensitivity and specificity of prognostic biomarkers, which would in turn help in planning personalized management [Citation37]. Methylation gene panels have also been reported for other malignancies. For example, the CDKN2a, CDH13, APC and RASSF1a methylation panel could help to identify early recurrent NSCLC patients, and the SOX17, TAC1, HOXA7, CDO1, HOXA9 and ZFP42 methylation panel improves accuracy in lung cancer diagnosis [Citation38].
Aberrant gene methylation of CDH1 in ovarian cancer has been reported previously. A meta-analysis of nine studies including 485 cancerous and 255 nonmalignant tissues reported that CDH1 methylation can be a predictor of ovarian cancer, especially in Asian populations [Citation39]. An in vitro study showed that 5-Aza demethylating treatment could lead to E-cadherin re-expression as well as the expression of the CDH1-coding protein, and inhibit invasion in SKOV3 cells [Citation40]. The epithelial-to-mesenchymal transition is important for tumor metastasis, and altered methylation of CDH1 was observed in TGF-β-induced epithelial-to-mesenchymal transition [Citation41]. Transcriptional silencing of CDH1 by gene methylation could contribute to chemoresistance, since E-cadherin renders cancer cells more sensitive to paclitaxel [Citation42]. DLEC1 methylation was associated with disease recurrence in a cohort of primary epithelial ovarian cancer patients, independent of either cancer stage or debulking operation. Loss of DLEC1 expression increased cell proliferation and led to disseminated disease, which was associated with poor prognosis [Citation43]. DLEC1 methylation was associated with its own downregulation, and a demethylating agent enhanced DLEC1 expression, which, in turn, suppressed the growth of ovarian cancer cells [Citation44]. Frequent SFRP5 methylation was also noted in epithelial ovarian cancer, and was a potential prognostic factor for ovarian clear cell carcinoma in particular [Citation23,Citation45,Citation46]. Another in vitro study showed that SFRP5 methylation activated the Wnt/β-catenin oncogenic pathway and led to ovarian cancer progression and chemoresistance [Citation47].
Epigenetic therapies currently include DNA demethylation agents, such as azacitidine and decitabine, as well as histone deacetylase inhibitors, such as vorinostat, romidepsin, panobinostat and belinostat. DNA demethylation agents inhibit DNA methylation by binding covalently to DNA methyltransferase 1 in order to express genes otherwise silenced by DNA methylation. Histone deacetylase inhibitors target the zinc ion dependency of histone deacetylase catalytic activity to allow for gene expression [Citation48]. These agents might allow for the resensitization of resistant or refractory ovarian cancer patients to platinum-based chemotherapy through the amelioration of the epigenetic repression of the genes responsible for chemoresistance [Citation49]. The restoration of maximal gene expression may require correction of both epigenetic silencing mechanisms, gene methylation and histone deacetylation [Citation50]. Treatments combining demethylating agents and histone deacetylase inhibitors in ovarian cancer cell lines or cancer tissue specimens showed synergistic effects for the upregulation of tumor-suppressor gene expression [Citation51,Citation52]. However, the toxicity associated with the demethylating agent/histone deacetylase inhibitor combination were largely intolerable. In vitro and xenograft studies showed that demethylating agents mediated the resensitization of platinum-resistant ovarian cancer cells to platinum-based treatment [Citation53]. Phase II trial of an azacytidine/carboplatin combination showed benefits for platinum-resistant ovarian cancer patients, indicating that the demethylating agent may partially reverse platinum resistance [Citation54]. In another Phase II trial of decitabine combined with carboplatin in heavily pretreated platinum-resistant ovarian cancer patients indicated that the treatment-induced decrease in methylation was associated with a reversal of platinum resistance [Citation55]. Therefore, a method to identify appropriate patients for epigenetic therapy is necessary for precision medicine in ovarian cancer, and this gene methylation panel could be a selection mechanism for candidates for demethylating agents.
There were some limitations in the current study. MS-PCR is a cost-effective method with high analytical sensitivity [Citation56–59]. In the original publication [Citation60], it was reported to detect 0.1% methylated DNA in an excess of unmethylated DNA (i.e., detection of one methylated allele in a background of 1000 unmethylated alleles). In subsequent other studies, 0.1% (50 pg of methylated DNA out of 50 ng of total DNA) or 1% (0.1 ng of methylated DNA out of 10 ng of total DNA) was also reported [Citation61]. The performance of methylation-specific PCR (MS-PCR) is influenced by quality of DNA, bisulfite treatment, primer design, annealing temperature and the PCR cycles [Citation57,Citation61]. The weakness of MS-PCR, including the qualitative method, inadequate analytic specificity, the possibility of false-positive result and limited throughput, could be improved by quantitative experimental platforms for DNA methylation [Citation57,Citation58,Citation61]. Another major limitation was that we used one single CpG site to represent the methylation level of one gene in the TCGA dataset. The main challenge of gene methylation is to determine the key regulatory CpG sites in the promoter region that controls mRNA transcription. The majority is considered to be near the open-reading frame, however, there can be a specific repetitive sequence sites or even no CpG regulatory site in the promotor region [Citation62]. However, the genome-wide methylation profiles in the TCGA dataset were investigated by using the Illumina 27K methylation microarray, which had a relatively low coverage of CpG sites due to the low probe numbers. Therefore, we could not examine all CpG sites located in the promoter region of one single gene at the same time, which may cause some biases and errors in the determination of the methylation level of one single gene. Besides, different proportions of patients positive for the methylation panel were observed in this study. The proportion was 30.5% in our cohort. However, in TCGA database, the proportions were 17.5% in the group for DFS analysis and 15.4% in the group for OS analysis. This may be mainly attributed to that two different experimental technologies, including MS-PCR (our cohort) and methylation microarray (TCGA database), were used to investigate the methylation level of those three genes. The genomic locations of the primers used in our cohort did not completely correspond to the probes of the Illumina 27K methylation microarray in the TCGA dataset. Furthermore, different sensitivity of these two methods and different cut-off values to define a patient were both possible explanations of this discrepancy in different patient cohorts.
Conclusion
Our CDH1, DLEC1 and SFRP5 methylation panel is a potential prognostic biomarker for advanced epithelial ovarian cancer. It could help in selecting susceptible candidates for demethylation therapy and incorporating in trial design for epigenetic therapy. Additional studies are needed to prove the utility of the methylation panel for the management of epithelial ovarian cancer patients.
Cytotoxic chemotherapy remains the current mainstream treatment for ovarian cancer management, and it is important to identify and predict resistant patients to provide cost-effective treatments.
We investigate the prognostic value of the CDH1, DLEC1 and SFRP5 gene methylation panel in advanced epithelial ovarian cancer patients and evaluate the panel using The Cancer Genome Atlas dataset.
Patients with tumor recurrence had higher frequencies of two or more methylated genes relative to patients without tumor recurrence.
Chemoresistant patients had higher frequencies of two or more methylated genes relative to chemosensitive patients.
Women with two or more methylated genes had a significantly shorter disease-free survival and overall survival compared with women with fewer that two methylated genes.
The presence of two or more methylated genes was an independent factor for recurrence and death in multivariate Cox regression model of our cohort.
In The Cancer Genome Atlas dataset, the presence of two or three methylated genes was significant in death and close to the significance level in recurrence.
The CDH1, DLEC1 and SFRP5 methylation panel is a potential prognostic biomarker for advanced epithelial ovarian carcinoma.
Author’s contributions
Conception and design were proposed by YC Chiang and WF Cheng. Development of methodology and acquisition of data were finished by HW Lin, CF Fu, MC Chang, TP Lu and HP Lin. Analysis and interpretation of data were completed by HW Lin, CF Fu, YC Chiang, CA Chen and WF Cheng. HW Lin, CF Fu and YC Chiang were engrossed the writing and revision of the manuscript. All the authors read and approved the final manuscript.
Ethical conduct of research
The protocol was approved by the National Taiwan University Hospital (NTUH) Research Ethics Committee (201012111RC). Informed written consents were obtained and the methods were performed in accordance with the guidelines and regulations.
Supplemental figure 1
Download TIFF Image (281.1 KB)Supplemental figure 2
Download TIFF Image (659.3 KB)Supplemental application 3
Download MS Word (75.5 KB)Acknowledgements
This work was supported by the 7th core laboratory facility of the Department of Medical Research of National Taiwan University Hospital.
Supplementary data
To view the supplementary data that accompany this paper please visit the journal website at: https://www.tandfonline.com/doi/suppl/10.2217/epi-2018-0035
Financial & competing interests disclosure
This work was supported by grant 103-EDN03, 105-EDN09 from E-da Hospital Kaohsiung, Taiwan-National Taiwan University Hospital Joint Research Program and grant MOST 105-2314-B-002-151 from the Ministry of Science and Technology, Taipei, Taiwan. The authors have no other relevant affiliations or financial involvement with any organization or entity with a financial interest in or financial conflict with the subject matter or materials discussed in the manuscript apart from those disclosed.
No writing assistance was utilized in the production of this manuscript.
Additional information
Funding
References
- Siegel RL , MillerKD , JemalA . Cancer statistics, 2016 . CA Cancer J. Clin.66 ( 1 ), 7 – 30 ( 2016 ).
- Siegel RL , MillerKD , JemalA . Cancer statistics, 2017 . CA Cancer J. Clin.67 ( 1 ), 7 – 30 ( 2017 ).
- Chiang YC , ChenCA , ChiangCJet al. Trends in incidence and survival outcome of epithelial ovarian cancer: 30-year national population-based registry in Taiwan . J. Gynecol. Oncol.24 ( 4 ), 342 – 351 ( 2013 ).
- Jayson GC , KohnEC , KitchenerHC , LedermannJA . Ovarian cancer . Lancet384 ( 9951 ), 1376 – 1388 ( 2014 ).
- Banerjee S , KayeSB . New strategies in the treatment of ovarian cancer: current clinical perspectives and future potential . Clin. Cancer Res.19 ( 5 ), 961 – 968 ( 2013 ).
- Vaughan S , CowardJI , BastRCJret al. Rethinking ovarian cancer: recommendations for improving outcomes . Nat. Rev. Cancer.11 ( 10 ), 719 – 725 ( 2011 ).
- Coleman RL , MonkBJ , SoodAK , HerzogTJ . Latest research and treatment of advanced-stage epithelial ovarian cancer . Nat. Rev. Clin. Oncol.10 ( 4 ), 211 – 224 ( 2013 ).
- Hiss D . Optimizing molecular-targeted therapies in ovarian cancer: the renewed surge of interest in ovarian cancer biomarkers and cell signaling pathways . J. Oncol. 2012 , 737981 ( 2012 ).
- Perren TJ , SwartAM , PfistererJet al. A Phase 3 trial of bevacizumab in ovarian cancer . N. Engl. J. Med.365 ( 26 ), 2484 – 2496 ( 2011 ).
- Oza AM , CookAD , PfistererJet al. Standard chemotherapy with or without bevacizumab for women with newly diagnosed ovarian cancer (ICON7): overall survival results of a Phase 3 randomised trial . Lancet Oncol.16 ( 8 ), 928 – 936 ( 2015 ).
- Burger RA , BradyMF , BookmanMAet al. Incorporation of bevacizumab in the primary treatment of ovarian cancer . N. Engl. J. Med.365 ( 26 ), 2473 – 2483 ( 2011 ).
- Ledermann J , HarterP , GourleyCet al. Olaparib maintenance therapy in platinum-sensitive relapsed ovarian cancer . N. Engl. J. Med.366 ( 15 ), 1382 – 1392 ( 2012 ).
- Mirza MR , MonkBJ , HerrstedtJet al. Niraparib maintenance therapy in platinum-sensitive, recurrent ovarian cancer . N. Engl. J. Med.375 ( 22 ), 2154 – 2164 ( 2016 ).
- Marchetti C , LedermannJA , Benedetti PaniciP . An overview of early investigational therapies for chemoresistant ovarian cancer . Expert Opin. Investig. Drugs24 ( 9 ), 1163 – 1183 ( 2015 ).
- Kampan NC , MadondoMT , McNallyOM , QuinnM , PlebanskiM . Paclitaxel and its evolving role in the management of ovarian cancer . BioMed. Res. Int. 2015 , 413076 ( 2015 ).
- Egger G , LiangG , AparicioA , JonesPA . Epigenetics in human disease and prospects for epigenetic therapy . Nature429 ( 6990 ), 457 – 463 ( 2004 ).
- Huang YW , KuoCT , StonerK , HuangTH , WangLS . An overview of epigenetics and chemoprevention . FEBS Lett.585 ( 13 ), 2129 – 2136 ( 2011 ).
- Palmirotta R , SilvestrisE , D’OronzoS , CardasciaA , SilvestrisF . Ovarian cancer: novel molecular aspects for clinical assessment . Crit. Rev. Oncol. Hematol.117 , 12 – 29 ( 2017 ).
- Yoo CB , JonesPA . Epigenetic therapy of cancer: past, present and future . Nat. Rev. Drug Discov.5 ( 1 ), 37 – 50 ( 2006 ).
- Herman JG , BaylinSB . Gene silencing in cancer in association with promoter hypermethylation . N. Engl. J. Med.349 ( 21 ), 2042 – 2054 ( 2003 ).
- Chen CA , ChiangYC , ChangMCet al. Gene methylation profiles as prognostic markers in ovarian clear cell and endometrioid adenocarcinomas . Am. J. Transl. Res.7 ( 1 ), 139 – 152 ( 2015 ).
- Prat J . Staging classification for cancer of the ovary, fallopian tube, and peritoneum . Int. J. Gynaecol. Obstet.124 ( 1 ), 1 – 5 ( 2014 ).
- Fandy TE , HermanJG , KernsPet al. Early epigenetic changes and DNA damage do not predict clinical response in an overlapping schedule of 5-azacytidine and entinostat in patients with myeloid malignancies . Blood114 ( 13 ), 2764 – 2773 ( 2009 ).
- Moon DC , ChoiCH , LeeSMet al. Nuclear translocation of Acinetobacter baumannii transposase induces DNA methylation of CpG regions in the promoters of E-cadherin gene . PLoS ONE7 ( 6 ), e38974 ( 2012 ).
- Xiao Q , ZhouD , RuckiAAet al. Cancer-associated fibroblasts in pancreatic cancer are reprogrammed by tumor-induced alterations in genomic DNA methylation . Cancer Res.76 ( 18 ), 5395 – 5404 ( 2016 ).
- Ayadi W , Karray-HakimH , KhabirAet al. Aberrant methylation of p16, DLEC1, BLU and E-cadherin gene promoters in nasopharyngeal carcinoma biopsies from Tunisian patients . Anticancer Res.28 ( 4b ), 2161 – 2167 ( 2008 ).
- Hong Q , LiY , ChenXet al. CDKN2B, SLC19A3 and DLEC1 promoter methylation alterations in the bone marrow of patients with acute myeloid leukemia during chemotherapy . Exp. Ther. Med.11 ( 5 ), 1901 – 1907 ( 2016 ).
- Pastuszak-Lewandoska D , KordiakJ , AntczakAet al. Expression level and methylation status of three tumor suppressor genes, DLEC1, ITGA9 and MLH1, in non-small cell lung cancer . Med. Oncol.33 ( 7 ), 75 ( 2016 ).
- Tian F , YipSP , KwongDL , LinZ , YangZ , WuVW . Promoter hypermethylation of tumor suppressor genes in serum as potential biomarker for the diagnosis of nasopharyngeal carcinoma . Cancer Epidemiol.37 ( 5 ), 708 – 713 ( 2013 ).
- Corn PG , SmithBD , RuckdeschelES , DouglasD , BaylinSB , HermanJG . E-cadherin expression is silenced by 5′ CpG island methylation in acute leukemia . Clin. Cancer Res.6 ( 11 ), 4243 – 4248 ( 2000 ).
- Cheng YY , YuJ , WongYPet al. Frequent epigenetic inactivation of secreted frizzled-related protein 2 (SFRP2) by promoter methylation in human gastric cancer . Br. J. Cancer97 ( 7 ), 895 – 901 ( 2007 ).
- Chung MT , SytwuHK , YanMDet al. Promoter methylation of SFRPs gene family in cervical cancer . Gynecol. Oncol.112 ( 2 ), 301 – 306 ( 2009 ).
- Sogabe Y , SuzukiH , ToyotaMet al. Epigenetic inactivation of SFRP genes in oral squamous cell carcinoma . Int. J. Oncol.32 ( 6 ), 1253 – 1261 ( 2008 ).
- Zou H , MolinaJR , HarringtonJJet al. Aberrant methylation of secreted frizzled-related protein genes in esophageal adenocarcinoma and Barrett’s esophagus . Int. J. Cancer116 ( 4 ), 584 – 591 ( 2005 ).
- Colombo PE , FabbroM , TheilletC , BibeauF , RouanetP , Ray-CoquardI . Sensitivity and resistance to treatment in the primary management of epithelial ovarian cancer . Crit. Rev. Oncol. Hematol.89 ( 2 ), 207 – 216 ( 2014 ).
- Gloss BS , SamimiG . Epigenetic biomarkers in epithelial ovarian cancer . Cancer Lett.342 ( 2 ), 257 – 263 ( 2014 ).
- Earp MA , CunninghamJM . DNA methylation changes in epithelial ovarian cancer histotypes . Genomics106 ( 6 ), 311 – 321 ( 2015 ).
- Ronnekleiv-Kelly SM , SharmaA , AhujaN . Epigenetic therapy and chemosensitization in solid malignancy . Cancer Treat. Rev.55 , 200 – 208 ( 2017 ).
- Wang Q , WangB , ZhangYM , WangW . The association between CDH1 promoter methylation and patients with ovarian cancer: a systematic meta-analysis . J. Ovarian Res.9 , 23 ( 2016 ).
- Yuecheng Y , HongmeiL , XiaoyanX . Clinical evaluation of E-cadherin expression and its regulation mechanism in epithelial ovarian cancer . Clin. Exp. Metastasis23 ( 1 ), 65 – 74 ( 2006 ).
- Cardenas H , ViethE , LeeJet al. TGF-beta induces global changes in DNA methylation during the epithelial-to-mesenchymal transition in ovarian cancer cells . Epigenetics9 ( 11 ), 1461 – 1472 ( 2014 ).
- Ferreira P , OliveiraMJ , BeraldiEet al. Loss of functional E-cadherin renders cells more resistant to the apoptotic agent taxol in vitro . Exp. Cell Res.310 ( 1 ), 99 – 104 ( 2005 ).
- Montavon C , GlossBS , WartonKet al. Prognostic and diagnostic significance of DNA methylation patterns in high grade serous ovarian cancer . Gynecol. Oncol.124 ( 3 ), 582 – 588 ( 2012 ).
- Kwong J , LeeJY , WongKKet al. Candidate tumor-suppressor gene DLEC1 is frequently downregulated by promoter hypermethylation and histone hypoacetylation in human epithelial ovarian cancer . Neoplasia8 ( 4 ), 268 – 278 ( 2006 ).
- Niskakoski A , KaurS , StaffSet al. Epigenetic analysis of sporadic and Lynch-associated ovarian cancers reveals histology-specific patterns of DNA methylation . Epigenetics9 ( 12 ), 1577 – 1587 ( 2014 ).
- Ho CM , LaiHC , HuangSH , ChienTY , LinMC , ChangSF . Promoter methylation of sFRP5 in patients with ovarian clear cell adenocarcinoma . Eur. J. Clin. Invest.40 ( 4 ), 310 – 318 ( 2010 ).
- Su HY , LaiHC , LinYWet al. Epigenetic silencing of SFRP5 is related to malignant phenotype and chemoresistance of ovarian cancer through Wnt signaling pathway . Int. J. Cancer127 ( 3 ), 555 – 567 ( 2010 ).
- Natanzon Y , GoodeEL , CunninghamJM . Epigenetics in ovarian cancer . Semin. Cancer Biol.51 , 160 – 169 ( 2018 ).
- Oronsky B , RayCM , SpiraAI , TrepelJB , CarterCA , CottrillHM . A brief review of the management of platinum-resistant-platinum-refractory ovarian cancer . Med. Oncol.34 ( 6 ), 103 ( 2017 ).
- Jones PA , BaylinSB . The epigenomics of cancer . Cell128 ( 4 ), 683 – 692 ( 2007 ).
- Terasawa K , SagaeS , ToyotaMet al. Epigenetic inactivation of TMS1/ASC in ovarian cancer . Clin. Cancer Res.10 ( 6 ), 2000 – 2006 ( 2004 ).
- Shi H , WeiSH , LeuYWet al. Triple analysis of the cancer epigenome: an integrated microarray system for assessing gene expression, DNA methylation, and histone acetylation . Cancer Res.63 ( 9 ), 2164 – 2171 ( 2003 ).
- Seeber LM , Van DiestPJ . Epigenetics in ovarian cancer . Methods Mol. Biol.863 , 253 – 269 ( 2012 ).
- Fu S , HuW , IyerRet al. Phase 1b–2a study to reverse platinum resistance through use of a hypomethylating agent, azacitidine, in patients with platinum-resistant or platinum-refractory epithelial ovarian cancer . Cancer117 ( 8 ), 1661 – 1669 ( 2011 ).
- Matei D , FangF , ShenCet al. Epigenetic resensitization to platinum in ovarian cancer . Cancer Res.72 ( 9 ), 2197 – 2205 ( 2012 ).
- Derks S , LentjesMH , HellebrekersDM , De BruineAP , HermanJG , Van EngelandM . Methylation-specific PCR unraveled . Cell Oncol.26 ( 5–6 ), 291 – 299 ( 2004 ).
- Kristensen LS , HansenLL . PCR-based methods for detecting single-locus DNA methylation biomarkers in cancer diagnostics, prognostics, and response to treatment . Clin. Chem.55 ( 8 ), 1471 – 1483 ( 2009 ).
- Cottrell SE , LairdPW . Sensitive detection of DNA methylation . Ann. NY Acad. Sci.983 , 120 – 130 ( 2003 ).
- Laird PW . The power and the promise of DNA methylation markers . Nat. Rev. Cancer3 ( 4 ), 253 – 266 ( 2003 ).
- Herman JG , GraffJR , MyohanenS , NelkinBD , BaylinSB . Methylation-specific PCR: a novel PCR assay for methylation status of CpG islands . Proc. Natl Acad. Sci. USA93 ( 18 ), 9821 – 9826 ( 1996 ).
- Hernandez HG , TseMY , PangSC , ArboledaH , ForeroDA . Optimizing methodologies for PCR-based DNA methylation analysis . BioTechniques55 ( 4 ), 181 – 197 ( 2013 ).
- Worsham MJ , StephenJK , ChenKMet al. Delineating an epigenetic continuum in head and neck cancer . Cancer Lett.342 ( 2 ), 178 – 184 ( 2014 ).