Abstract
Aim: We examined the preferences of adults with Philadelphia chromosome-positive acute lymphoblastic leukemia (Ph+ ALL) for benefits and risks of tyrosine kinase inhibitors combined with chemotherapy for first-line treatment. Methods: In a discrete choice experiment, 201 patients chose between hypothetical treatment alternatives with varied levels of remission duration and overall survival (OS), and risks of major cardiovascular (CV) events and myelosuppression. Results: Although OS was the most important attribute to patients with Ph+ ALL, they were willing to tolerate a 2.9% increase in CV risk for 1 additional month of OS. Older patients (>59 years) and patients not in remission were less likely to tolerate increased CV risk. Conclusion: Preferences and risk tolerance varied between patients, highlighting the importance of shared decision making when selecting treatments for Ph+ ALL.
Plain language summary
Treatments differ in their potential benefits and side effects they may come with. Patients should be involved in deciding which treatments they receive. This is because patients may have different views than physicians on how the benefits and side effects of treatment would affect their quality of life. Additionally, patients may have different risk tolerances. This study shows how patients with a form of leukemia valued survival benefits and side effects of treatments, and the trade-offs that they were willing to make between these. On average, longer survival had most value to patients. They were willing to accept a higher risk of a major cardiovascular side effect (e.g., having a stroke) if the treatment would allow them to live longer. However, not all patients had the same opinion, and some groups of patients were less willing to accept risks to receive longer survival. By involving patients in treatment decisions, we can help ensure they receive treatments that match their personal preferences.
Philadelphia chromosome-positive acute lymphoblastic leukemia (Ph+ ALL), which represents around 30% of all adult ALL cases [Citation1,Citation2], previously had a very poor prognosis with a 1-year overall survival (OS) of around 10% on traditional chemotherapy [Citation3]. Clinical outcomes improved significantly following the identification of the BCR-ABL1 oncogene, along with the introduction of the tyrosine kinase inhibitors (TKIs) imatinib and later second-generation TKIs such as dasatinib and bosutinib [Citation3,Citation4]. Several TKIs are approved for treating relapsed or refractory Ph+ ALL in adults [Citation2,Citation5]. TKIs are also used off-label for first-line treatment of Ph+ ALL in combination with chemotherapy [Citation5], and imatinib plus chemotherapy is approved for this indication in Europe. Despite the effectiveness of early-generation TKIs, many treated patients relapse because of mutation-specific resistance [Citation3,Citation6]. Ponatinib, a third-generation TKI, is able to inhibit most BCR-ABL1 mutations and has achieved significantly longer event-free survival and OS than earlier TKIs when used as first-line therapy for Ph+ ALL [Citation7,Citation8].
Although TKIs have revolutionized Ph+ ALL treatment for patients, they have been associated with major cardiovascular (CV) events and myelosuppression, the rates of which differ with each TKI [Citation9–11]. CV and cerebrovascular events were observed in a phase II trial of ponatinib in Ph+ leukemias [Citation12]. The toxicities noted for ponatinib, which led to updated label warnings, also resulted in closer assessment of cardiac, vascular and metabolic adverse events for other TKIs used to treat leukemias [Citation13].
CV events have also been observed with the use of other TKIs in leukemias [Citation13–15]. For example, in an analysis of the US FDA Adverse Event Reporting System database, nilotinib treatment was associated with the highest proportion of CV events among five TKIs examined (including ponatinib), and was particularly related to ischemic heart disease and cardiac arrythmias [Citation16]. Dasatinib and bosutinib were the TKIs most associated with cardiac failure.
Although some TKIs have lower risk of CV events than others, their associated clinical responses may be less common and shorter in duration [Citation17]. Since there is a trade-off between Ph+ ALL treatments with the potential for longer remission and OS, but with higher risk of CV events and myelosuppression, it is important to understand patients’ preferences. Patients may have different perceptions from clinicians and regulatory authorities, as well as among themselves, for how treatment outcomes and adverse events affect their quality of life [Citation18–20]. Additionally, preferences differ among patients based on their individual circumstances and risk tolerance; therefore, it is important to understand which patients are willing to accept certain risks associated with a treatment. The FDA, EMA and other health technology assessment agencies increasingly promote direct patient participation in regulatory decision making and using patient preference data as part of the evidence considered [Citation19–22].
The objective of this study was to understand how Ph+ ALL patients trade off the benefits and risks of TKIs in combination with chemotherapy for first-line treatment of Ph+ ALL, and how this varies with their demographic and clinical characteristics.
Patients & methods
Study overview
An online survey was conducted in the USA between February and April 2020. To elicit patient preferences, the survey included a discrete choice experiment (DCE). DCEs comprise a series of questions that present the respondent with a choice between two treatments where there is a trade-off, such as a trade-off between efficacy and risk of adverse events [Citation23]. The results can be used to calculate the relative importance of treatment attributes to patients. DCEs are the most used method to generate patient stated preferences and are encouraged by the US FDA, EMA and other decision-making authorities [Citation19,Citation20,Citation24]. Qualitative interviews were conducted in parallel to the survey to aid interpretation of the results. The study was approved by Ethical and Independent Review Services (E&I, MO, USA) and was conducted in accordance with best practice guidelines on preference-based methods from International Society for Pharmacoeconomics and Outcomes Research [Citation25,Citation26]. All participants provided online consent before participating in the survey. Patient representatives were engaged in the design of the study; The Leukemia and Lymphoma Society provided input on study deliverables (including the protocol, statistical analysis plan and report) and participated in data acquisition.
Participants
US adults (aged ≥ 18 years) with Ph+ ALL were identified and recruited via healthcare practitioner referrals, databases and social media, and through the Leukemia and Lymphoma Society. Potentially eligible participants were invited to participate in the study via emails, which contained a web link to the survey. To participate in the survey, respondents had to self-report that they had Ph+ ALL; were aged ≥ 18 years; resided in the USA; could read, speak and understand English; and provide online consent. Patients were excluded if they self-reported a previous diagnosis of chronic phase, accelerated phase or blast phase chronic myeloid leukemia. Patients did not need to have received treatment with a TKI to participate in the study. Participants were remunerated for taking part in the study.
DCE attributes
The treatment choices shown to participants as part of the DCE were described using predefined attributes and levels. Although there are many potential benefits and adverse events associated with TKI treatments for Ph+ ALL, it is not possible to include all possible attributes in a DCE, as it is cognitively burdensome on respondents and the inclusion of additional attributes requires a larger sample size for precise preference estimates. Key attributes and their levels were therefore determined through a targeted literature review using Embase and MEDLINE databases, interviews with two hematologists treating patients with Ph+ ALL, and input from the Leukemia and Lymphoma Society.
No studies were identified in the literature review that specifically examined ALL patients’ preferences for aspects of treatment. The interviewed hematologists suggested that survival and remission should be included in the DCE, and that heart attack and stroke are the key CV risks that patients are concerned about. They also suggested that patients with Ph+ ALL and a short life expectancy may be less concerned by long-term risks and more concerned by their treatment working, and that myelosuppression could be a key cause of short-term adverse events. Four key benefit and risk attributes of treatments for Ph+ ALL were identified: OS, duration of remission (DoR), risk of major CV events and risk of a suppressed immune system (referred to as myelosuppression henceforth). The format, attribute levels and terminology used in the DCE were refined through feedback from a patient representative (identified by the Leukemia and Lymphoma Society). The patient representative indicated that they would be willing to tolerate CV risk and myelosuppression risk much higher than that associated with treatment. As a result, the maximum CV risk level was increased to 50% in the DCE survey and the maximum myelosuppression risk level to 100%, to ensure there were trade-offs and that survival and remission did not dominate patient choices.
The relevance, completeness, tradability and clarity of the attributes were refined through cognitive pilot interviews conducted by telephone with five participants with Ph+ ALL matching the eligibility criteria. Attributes and levels included in the final DCE are summarized in .
Table 1. Final attributes and levels included in the discrete choice experiment.
DCE design
In each choice task, participants had to choose between two treatment alternatives (chemotherapy + TKI A and chemotherapy + TKI B). The attribute levels differed between the two treatment alternatives () and were varied according to the experimental design. The experimental design was developed using the dp-optimal method in Ngene software version 1.1.2 (ChoiceMetrics, Sydney, Australia). To avoid potential dominance of the survival attribute, two variants of the DCE were run: one with OS ranging from 30 to 60 months (variant A), and the other with OS levels ranging from 60 to 90 months (variant B). The design also had constraints that each pair of treatment alternatives would have a maximum of 10 months’ difference in OS. Each variant had 24 experimental choice tasks, which were split into two blocks per variant, so each participant completed 12 choice tasks. An example choice task is shown in . In addition, participants completed two nonexperimental choice tasks to assess internal validity: the third choice task was repeated for the second-to-last choice task to assess the consistency of participants’ answers; a dominated choice task was presented as the final choice task. The dominant alternative was described with more favorable levels for all attributes, with exception of OS, which was constant between both options. At the end of the survey, participants were required to complete health literacy, numeracy, clinical and sociodemographic questions.
Statistical analysis
The statistical analysis was conducted using R version 3.6.1. Statistical tests were two-sided and used a significance level of 0.05. DCE preference data were analyzed within the framework of random utility maximization [Citation27,Citation28]. Preferences for changes in the attributes were expressed as part-worth utilities, where a higher part-worth utility for a specific attribute level indicates a greater likelihood that a treatment scenario including that attribute level will be chosen, if everything else is equal. To measure participants’ preferences for changes in the treatment attributes, an initial multinomial logit (MNL) model was estimated with a system of dummy-coded variables (0/1). The model also included an interaction term between OS and DoR, since participants’ valuation of OS may be influenced by the length of time in remission. After verifying the linearity in preferences for changes in the attributes, a separate MNL model was estimated with linear encoding for all four attributes to derive meaningful maximum acceptable risk and minimum acceptable benefit measures. Several robustness checks were conducted to establish which model to use as the reference model (see Supplemental Methods and Supplementary Table 1). To assess potential effects of sociodemographic and clinical characteristics on preference heterogeneity, an MNL model was used with interaction terms between the personal characteristics of the respondents and the attributes’ levels of the choice options. To assess willingness to make trade-offs between attributes, maximum acceptable risk of a major CV event was estimated as the percent increase in risk tolerated to improve other treatment attributes by one unit.
Qualitative interviews
Five participants meeting the eligibility criteria were interviewed using a semi-structured interview guide to gain insight into the symptoms and impacts of Ph+ ALL and provide qualitative insight to aid interpretation of the DCE results (note that these are separate from the cognitive pilot interviews which pilot test the quantitative survey). Interviews were conducted via teleconference and lasted 60 min. A coding dictionary was developed based on the interview guide and themes/concepts that emerged during the discussions. The coding dictionary was reviewed and revised prior to initiating analysis. Transcripts were analyzed using ATLAS.ti version 8.0.
Results
Participant characteristics
The DCE survey was completed by 201 participants: 60% were male, the mean age was 44.8 ± 12.9 years and 67% had a college education or higher (). Most participants (65%) were White, 9% were Black or African–American and 6% were Asian. Nearly one-fifth (19%) were Hispanic or Latinx. Two-thirds of participants (67%) reported being in remission (including those who previously relapsed), 12% had not achieved remission and 19% had relapsed after achieving remission. Nearly one-half of participants (48%) had experienced one or more relapses. Twenty-five participants (12%) were treatment naive. The most common TKI treatments being received at the time of the survey were dasatinib (n = 48, 24%) and imatinib (n = 36, 18%).
Table 2. Patient demographics and baseline characteristics in the main survey.
Validity assessments
Participants spent an average of 7 min, 17 s to complete the DCE part of the survey (i.e., 36 s per choice task). The internal validity assessments suggested that participants were attending to choice tasks and trading off between attributes when choosing their preferred treatment: 88% (n = 177) passed the choice dominance test (i.e., choosing the treatment option that was superior for all attributes), which exceeded the expected pass rate of 87%, and 78% (n = 156) demonstrated consistency in answering repeated choice questions in line with existing research [Citation29]. In addition, 96% (n = 193) of respondents did not display lexicographic preferences (i.e., choices always based on one attribute), and 98% (n = 196) varied their choices between TKI A + chemotherapy and TKI B + chemotherapy (i.e., did not select the same option throughout the survey).
Preferences for attributes
Patients’ choices were significantly impacted by all attributes, and preferences were in the expected direction (i.e., preference for less risk and more benefit; ). An increase in OS by 1 month had the greatest impact on participant choices (part-worth utility = 0.032 [95% CI: 0.021–0.042], p < 0.001). The utility gain for a 1-month increase in OS was valued roughly three-times more than a 1% decrease in the risk of a major CV event and 3.5-times more than a 1% decrease in myelosuppression risk. Participants’ choices were also impacted by a 1-month increase in DoR (part-worth utility = 0.017 [95% CI: 0.012–0.023], p < 0.001), a 1% reduction in CV risk (part-worth utility = 0.011 [95% CI: 0.008–0.013], p < 0.001) and a 1% reduction in myelosuppression risk (part-worth utility = 0.009 [95% CI: 0.008–0.010], p < 0.001). On average, participants were willing to tolerate a 2.9% (95% CI: 1.78–4.02) increase in risk of a major CV event for 1 additional month of OS, and a 1.59% (95% CI: 0.95%–2.24%) increase in risk of a major CV event for an additional 1 month in remission ().
Discrete choice experiment preference data collected from the main survey were analyzed using a linear multinomial logit model. Preferences for changes in the attributes are expressed as part-worth utilities. Markers indicate maximum likelihood estimates, and the bars indicate 95% CI.
CV: Cardiovascular; DOR: Duration of remission; OS: Overall survival.
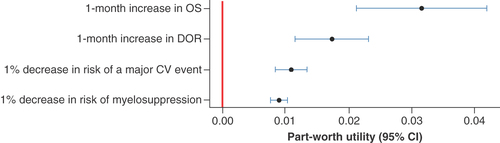
Preference heterogeneity
Preferences of patients for treatment attributes were heterogeneous, and risk tolerance varied due to personal characteristics (). The willingness to tolerate an increase in risk of a major CV event for 1 month of additional OS was higher in patients who were aged <40 years versus >59 years, in remission versus not in remission, and who had 1 or more versus no Ph+ ALL-related emergency room visit in the past year.
Maximum acceptable CV risk for 1 month additional OS by participant subgroups.
CV: Cardiovascular; ER: Emergency room; MAR: Maximum acceptable risk; OS: Overall survival; Ph+ ALL: Philadelphia chromosome-positive acute lymphoblastic leukemia; y: Year.
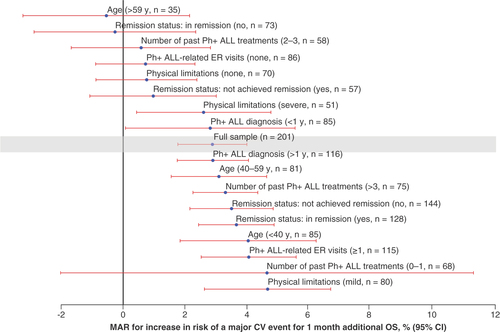
The qualitative interviews similarly revealed heterogeneity in willingness to trade off OS or quality of life for reduced risk of CV events or myelosuppression (). The sample was split on whether improving survival or avoiding the risk was most important. For example, some participants were deeply concerned by the increased risk of having a heart attack or stroke (e.g., from knowing people who had suffered such events) and believed that such an event would substantially reduce their quality of life and limit planned activities and enjoyment in their remaining time; thus, they tolerated giving up 10 months of longer survival to reduce this risk from 50 to 25%. Similarly, several participants prioritized avoiding the risk of myelosuppression due to concerns for how a suppressed immune system might reduce quality of life (e.g., fatigue, sickness and hospitalizations, staying at home to avoid community-acquired infections), whereas some interviewees believed that this could be managed by their own behavior – for example by taking vitamin C and other over-the-counter supplements, regular handwashing and wearing a mask in public.
Table 3. Patient preferences from the qualitative interviews (n = 5).
Discussion
TKIs have improved clinical outcomes in patients with Ph+ ALL, but some have been associated with major CV events and myelosuppression [Citation4,Citation9–11,Citation30]. The manner in which patients with Ph+ ALL trade off these benefits and risks may influence the choice of TKI to include in their treatment plan. This DCE survey examined the relative importance of four key benefits and risks of TKI + chemotherapy combinations in patients with Ph+ ALL: DoR, OS, risk of major CV events and risk of myelosuppression.
Survival attributes are commonly ranked as most important by patients participating in DCEs relating to cancer treatments [Citation31]. The results from this study suggest patients with Ph+ ALL place most value on longer OS and remission times associated with a TKI + chemotherapy regimen and are willing to tolerate some adverse events for improvements in these attributes. Patients’ willingness to accept risks varied – patients were less likely to tolerate the increased CV risk if they were older (>59 years), not in remission or had not had a Ph+ ALL-related emergency room visit in the past year. Besides the observed relation between preferences and sociodemographic and clinical characteristics, some of the preference heterogeneity might reflect the different experiences of participants and their individual perceived ability to manage risks. For example, some were aware of debilities caused by a stroke or heart attack in people they knew, and some believed that the impact of a suppressed immune system could be managed by their own behavior, such as wearing a mask or taking dietary supplements (note that the study was conducted before the COVID-19 pandemic).
Given the different preferences of individual patients, it is important that clinicians engage with patients in shared decision making so that they understand the trade-offs patients are making as they choose treatment. This will help clinicians understand patients’ preferences and identify the most suitable treatment for them based on their individual preferences [Citation18,Citation32]. The results of this study can also help inform patient-focused drug development by enabling the incorporation of patient preferences in the design, approval and reimbursement of TKI-based treatment regimens.
A major strength of this study was the involvement of a patient advocacy organization and a patient representative in the DCE’s development, coupled with interviews with hematologists. Involvement of all stakeholders is strongly encouraged by the FDA, EMA and other decision makers [Citation19–22]. Partly informed by the stakeholder engagement, various innovations in the DCE design were adopted to ensure that robust and meaningful data were obtained from patients. Attribute confounding is a general risk within DCEs if attributes overlap or if the preferences of one attribute could depend upon levels of other attributes [Citation33]. This is the case in oncology, with overlap and potential preference dependence between remission and survival attributes. Indeed, work with stakeholders in this study, and previous research [Citation31], have identified a risk that the survival attribute might dominate patients’ choices. Two strategies were adopted to account for this. First, the experimental design anticipated a potential interaction effect between OS and DoR, although no interaction effect was found. Second, constraints were included in the experimental design so that participants would only compare treatments with a maximum of 10 months’ difference in OS. In addition, the presentation of DoR and OS attributes was designed and tested to ensure that the relationship between these attributes was clear to participants. Other strengths were the study’s large size, particularly considering how rare Ph+ ALL is, and the data from parallel qualitative interviews, both of which supported the analysis and interpretation of preference heterogeneity.
All stated preference methods, including DCEs, may be subject to hypothetical bias (i.e., the hypothetical choices made by respondents in the DCE differ from what they would choose in reality). To minimize hypothetical bias, the DCE was designed to be as credible and relevant to participants as possible by developing it based on input from a patient representative, a patient advocacy organization and cognitive pilot interviews. Although the DCE is a simplification of the treatment scenario – with fewer attributes than in reality and not accounting for comorbidities that might influence treatment decisions – it still sheds important light on trade-offs patients are willing to make between key attributes, and the need for shared decision making. Other potential limitations include the high education level of the study sample (two-thirds had a college education or higher, which is unlikely to be representative of the general patient population), and that their Ph+ ALL diagnosis, used to determine eligibility, was self-reported in the DCE. Small subgroup sample sizes might have limited some analyses, including tests for differences in preferences associated with sociodemographic and clinical characteristics. Similarly, the study might have benefited from further qualitative research in a larger group of participants. Finally, it is important to note that the CV risk levels included in the DCE were much greater than the actual rates of serious CV events associated with some doses of TKIs, to ensure that survival attributes did not dominate preferences and that patients made trade-offs between benefits and risks. No information on willingness to trade off is collected when an attribute dominates preferences. Existing data suggest that serious CV events occur in 5% of patients receiving higher-dose ponatinib (45 mg/day) [Citation11].
Conclusion
This study suggested that patients with Ph+ ALL place most value on treatment regimens that have longer OS and remission times, and that on average patients are willing to tolerate an increased risk of major CV events for improvements in these attributes. However, variation in the way patients made these trade-offs indicates that this is not always the case. These results highlight the importance for clinicians to identify patients’ perspectives and tolerance of risks before prescribing TKI regimens. This shared decision making can help ensure that patients get access to treatments that align with their preferences and risk tolerance.
Tyrosine kinase inhibitors (TKIs) have improved outcomes in patients with Philadelphia chromosome-positive acute lymphoblastic leukemia (Ph+ ALL), but treatment-associated benefits and risks vary with each TKI.
This study examined the preferences of adults with Ph+ ALL with respect to key benefit and risk attributes of TKIs in combination with chemotherapy.
Preferences were elicited in an online discrete choice experiment that included four attributes: overall survival (OS), duration of remission, risk of major cardiovascular (CV) events and risk of myelosuppression.
The study included 201 participants with Ph+ ALL (60% male; 44.8 ± 12.9 years; 67% in remission; 12% treatment naive).
An increase in OS by 1 month had the greatest impact on participants’ choices (relative to 1-month additional remission, 1% reduction in the risk of a major CV event or a 1% reduction in risk of myelosuppression). Participants were willing to tolerate a 2.9% (95% CI: 1.78–4.02) increase in risk of a major CV event for 1 additional month of OS, and a 1.59% (95% CI: 0.95%–2.24%) increase for 1 additional month in remission.
Patients were less likely to tolerate increased CV risk if they were older (>59 years) or were not in remission.
Overall, these results suggest that most patients with Ph+ ALL were willing to tolerate an increased risk of CV events in exchange for longer survival and time in remission, although preferences were heterogeneous.
Author contributions
A Ashaye, C Thomas, M Dalal, M Sae-Hau, E Weiss, S Campbell and K Marsh contributed to conception and design of the study. C Thomas, M Sae-Hau and E Weiss contributed to acquisition of the data. N Krucien contributed to analysis of the data. C Thomas, N Krucien and K Marsh contributed to writing the first draft of the manuscript. All authors contributed to interpretation of the data and to critical revision of the manuscript.
Financial & competing interests disclosure
This study was funded by Takeda Development Center Americas Inc. A Ashaye, M Dalal and S Campbell are employees of and own stock options in Takeda Development Center Americas Inc. C Thomas, N Krucien, and K Marsh are employees of Evidera, which was paid by Takeda Development Center Americas Inc. to conduct this study. V Kota is employed by the Section of Hematology and Oncology, Georgia Cancer Center at Augusta University, Augusta, GA, USA and was paid by Takeda Development Center Americas Inc. for work related to this study. M Sae-Hau and E Weiss are employees of the Leukemia & Lymphoma Society, Rye Brook, NY, USA. The Leukemia & Lymphoma Society received funding from Evidera, who conducted the study sponsored by Takeda Development Center Americas Inc. The authors have no other relevant affiliations or financial involvement with any organization or entity with a financial interest in or financial conflict with the subject matter or materials discussed in the manuscript apart from those disclosed.
Medical writing was provided by J Pitt (Evidera, Paris, France) and funded by Takeda Development Center Americas Inc.
Ethical conduct of research
The study was approved by Ethical and Independent Review Services (E&I, MO, USA) and was conducted in accordance with best practice guidelines on preference-based methods from the International Society for Pharmacoeconomics and Outcomes Research [Citation25,Citation26]. All participants provided online consent before participating in the survey.
Supplemental materials for “Patient preferences for frontline therapies for Ph-positive acute lymphoblastic leukemia: a discrete choice experiment”
Download MS Word (22.5 KB)Acknowledgments
The authors thank M Barnhart, who passed away, for her valuable contributions throughout this study.
Supplementary data
To view the supplementary data that accompany this paper please visit the journal website at: www.futuremedicine.com/doi/suppl/10.2217/fon-2022-0082
Additional information
Funding
References
- Park HS . Current treatment strategies for Philadelphia chromosome-positive adult acute lymphoblastic leukemia. Blood Res.55(Suppl. 1), 32–36 (2020).
- Soverini S , BassanR, LionT. Treatment and monitoring of Philadelphia chromosome-positive leukemia patients: recent advances and remaining challenges. J. Hematol. Oncol.12(1), 39 (2019).
- Terwilliger T , Abdul-HayM. Acute lymphoblastic leukemia: a comprehensive review and 2017 update. Blood Cancer J.7(6), e577 (2017).
- Chalandon Y , ThomasX, HayetteSet al. Randomized study of reduced-intensity chemotherapy combined with imatinib in adults with Ph-positive acute lymphoblastic leukemia. Blood125(24), 3711–3719 (2015).
- Brown PA , ShahB, AdvaniAet al. Acute Lymphoblastic Leukemia, Version 2.2021, NCCN Clinical Practice Guidelines in Oncology. J. Natl Compr. Canc. Netw.19(9), 1079–1109 (2021).
- Ravandi F , O’BrienSM, CortesJEet al. Long-term follow-up of a phase 2 study of chemotherapy plus dasatinib for the initial treatment of patients with Philadelphia chromosome-positive acute lymphoblastic leukemia. Cancer121(23), 4158–4164 (2015).
- Jabbour E , DerSarkissianM, DuhMSet al. Efficacy of ponatinib versus earlier generation tyrosine kinase inhibitors for front-line treatment of newly diagnosed Philadelphia-positive acute lymphoblastic leukemia. Clin. Lymphoma Myeloma Leuk.18(4), 257–265 (2018).
- Sasaki K , JabbourEJ, RavandiFet al. Hyper-CVAD plus ponatinib versus hyper-CVAD plus dasatinib as frontline therapy for patients with Philadelphia chromosome-positive acute lymphoblastic leukemia: a propensity score analysis. Cancer122(23), 3650–3656 (2016).
- Jabbour E , DeiningerM, HochhausA. Management of adverse events associated with tyrosine kinase inhibitors in the treatment of chronic myeloid leukemia. Leukemia25(2), 201–210 (2011).
- Valent P , HadzijusufovicE, SchernthanerGH, WolfD, ReaD, le CoutreP. Vascular safety issues in CML patients treated with BCR/ABL1 kinase inhibitors. Blood125(6), 901–906 (2015).
- Jabbour E , ShortNJ, RavandiFet al. Combination of hyper-CVAD with ponatinib as first-line therapy for patients with Philadelphia chromosome-positive acute lymphoblastic leukaemia: long-term follow-up of a single-centre, phase 2 study. Lancet Haematol.5(12), e618–e627 (2018).
- Cortes JE , KimDW, Pinilla-IbarzJet al. A phase 2 trial of ponatinib in Philadelphia chromosome-positive leukemias. N. Engl. J. Med.369(19), 1783–1796 (2013).
- Manouchehri A , KanuE, MauroMJ, AdayAW, LindnerJR, MoslehiJ. Tyrosine kinase inhibitors in leukemia and cardiovascular events: from mechanism to patient care. Arterioscler. Thromb. Vasc. Biol.40(2), 301–308 (2020).
- Dahlén T , EdgrenG, LambeMet al. Cardiovascular events associated with use of tyrosine kinase inhibitors in chronic myeloid leukemia: a population-based cohort study. Ann. Intern. Med.165(3), 161–166 (2016).
- Larson RA , HochhausA, HughesTPet al. Nilotinib vs imatinib in patients with newly diagnosed Philadelphia chromosome-positive chronic myeloid leukemia in chronic phase: ENESTnd 3-year follow-up. Leukemia26(10), 2197–2203 (2012).
- Cirmi S , ElAbd A, LetinierL, NavarraM, SalvoF. Cardiovascular toxicity of tyrosine kinase inhibitors used in chronic myeloid leukemia: an analysis of the FDA Adverse Event Reporting System Database (FAERS). Cancers (Basel)12(4), 826 (2020).
- Levy MY , McGarryLJ, HuangH, LustgartenS, ChiroliS, IannazzoS. Benefits and risks of ponatinib versus bosutinib following treatment failure of two prior tyrosine kinase inhibitors in patients with chronic phase chronic myeloid leukemia: a matching-adjusted indirect comparison. Curr. Med. Res. Opin.35(3), 479–487 (2019).
- de Bekker-Grob EW , BerlinC, LevitanBet al. Giving patients’preferences a voice in medical treatment life cycle: The PREFER public-private project. Patient10(3), 263–266 (2017).
- Mühlbacher AC , JuhnkeC, BeyerAR, GarnerS. Patient-focused benefit-risk analysis to inform regulatory decisions: the European Union perspective. Value Health19(6), 734–740 (2016).
- Reed Johnson F , ZhouM. Patient preferences in regulatory benefit-risk assessments: a US perspective. Value Health19(6), 741–745 (2016).
- European Medicines Agency . EMA Regulatory Science to 2025 (2020). www.ema.europa.eu/en/documents/regulatory-procedural-guideline/ema-regulatory-science-2025-strategic-reflection_en.pdf
- Marsh K , van TilJA, Molsen-DavidEet al. Health preference research in Europe: a review of its use in marketing authorization, reimbursement, and pricing decisions – report of the ISPOR Stated Preference Research Special Interest Group. Value Health23(7), 831–841 (2020).
- Soekhai V , de Bekker-GrobEW, EllisAR, VassCM. Discrete choice experiments in health economics: past, present and future. Pharmacoeconomics37(2), 201–226 (2019).
- US FDA . Patient Preference Information Voluntary Submission, Review in Premarket Approval Applications, Humanitarian Device Exemption Applications and de novo Requests, and Inclusion in Decision Summaries and Device Labeling: Guidance for Industry, Food and Drug Administration Staff, and Other Stakeholders (2016). www.fda.gov/media/92593/download
- Bridges JF , HauberAB, MarshallDet al. Conjoint analysis applications in health – a checklist: a report of the ISPOR Good Research Practices for Conjoint Analysis Task Force. Value Health14(4), 403–413 (2011).
- Reed Johnson F , LancsarE, MarshallDet al. Constructing experimental designs for discrete-choice experiments: report of the ISPOR Conjoint Analysis Experimental Design Good Research Practices Task Force. Value Health16(1), 3–13 (2013).
- Manski CF . The structure of random utility models. Theory Decision8, 229–254 (1977).
- Marschak J . Binary choice constraints and random utility indicators (1960). In: Economic Information, Decision, and Prediction: Selected Essays: Volume I Part I Economics of Decision.Springer Netherlands, Dordrecht, The Netherlands,218–239 (1974).
- Sandorf ED . Did you miss something? Inattentive respondents in discrete choice experiments. Environ. Resour. Econ.73(4), 1197–1235 (2018).
- O’Hare T , ShakespeareWC, ZhuXet al. AP24534, a pan-BCR-ABL inhibitor for chronic myeloid leukemia, potently inhibits the T315I mutant and overcomes mutation-based resistance. Cancer Cell.16(5), 401–412 (2009).
- Collacott H , SoekhaiV, ThomasCet al. A systematic review of discrete choice experiments in oncology treatments. Patient14(6), 775–790 (2021).
- Yu PP . Challenges in measuring cost and value in oncology: making it personal. Value Health19(5), 520–524 (2016).
- Mandeville KL , LagardeM, HansonK. The use of discrete choice experiments to inform health workforce policy: a systematic review. BMC Health Serv. Res.14, 367 (2014).