Abstract
Aim: This study aimed to assess physician preferences for later lines (third to fifth) of therapy in patients with relapsed/refractory multiple myeloma (RRMM) in the USA. Materials & methods: Factors relevant to physicians’ treatment preferences for RRMM were identified from a literature search and refined in a qualitative phase. Preferences were quantitatively assessed using a discrete choice experiment. Physicians (n = 227) made choices regarding treatment scenarios for RRMM. Results: Efficacy had the highest mean relative importance, with overall survival valued as most important when making treatment decisions for patients with RRMM. Reduced incidences of keratopathy and thrombocytopenia had similar relative importance in later-line treatment. Conclusion: Greater understanding of physicians’ criteria for clinical decision-making may help inform wider adoption of new treatments.
Plain language summary
When deciding which treatment patients with relapsed or refractory multiple myeloma should receive, physicians have to weigh the benefits of each treatment against the risk of side effects. This study required physicians to complete a survey on aspects involved in their treatment decisions and identified those of highest importance. Physicians chose patient survival as the most important factor and minimization of side effects as less important considerations. Reducing patients’ risk of developing corneal conditions or low platelet (a type of blood cell) count were of equal importance to doctors. Understanding physicians’ treatment preferences and the reasons behind them will help identify gaps in education about new therapies as they become available.
Graphical abstract
Multiple myeloma (MM) is a hematological malignancy resulting in the accumulation of terminally differentiated plasma cells in the bone marrow [Citation1,Citation2]. This disease has a high symptomatic burden including hypercalcemia, renal insufficiency, anaemia, end organ damage and bone disease, with lytic lesions resulting in pain and poor health-related quality-of-life [Citation1,Citation3–5].
Introduction of new classes of therapies, including immunomodulatory drugs, proteosome inhibitors (PIs) and anti-CD38 monoclonal antibodies, has significantly improved the overall survival (OS) of patients with MM in the last two decades [Citation1,Citation2,Citation6]. Despite these therapeutic advances, MM remains an incurable disease, with all patients experiencing relapse and eventually becoming refractory to the available therapies [Citation2,Citation7–9]. Treatment of patients with MM who have previously received two or more prior lines of therapy is challenging [Citation10,Citation11]. Additionally, patients who have become refractory to these therapeutic classes have been reported to have poor survival outcomes [Citation2]. A recent study showed the median OS of patients with relapsed/refractory MM (RRMM) was only 5.6 months once patients had developed refractoriness to two PIs, two immunomodulatory agents and anti-CD38 antibodies [Citation7].
In the treatment guidelines for patients with MM, no clear treatment recommendations have been described for patients with triple-class RRMM who are frequently retreated with agents that they have previously been exposed to [Citation2,Citation12,Citation13]. Approval of new/novel agents for treatment of RRMM in recent years [Citation12,Citation14–16], along with agents currently in development [Citation11], has increased the factors that must be considered when choosing a treatment for patients with RRMM in their third line of treatment and beyond (3L+). These factors include age, comorbidities, performance status, and disease factors (nature of disease, genetic risk status, duration of response to prior therapies, relapse characteristics). Treatment factors including first line of therapy received, depth of response to previous therapies, number of prior lines of therapy, resistance to other MM therapies, adverse reactions/residual toxicities from therapy, and prior receipt of autologous stem cell transplantation are also factors in treatment decisions [Citation12–14,Citation17]. Given the range of therapeutic factors to consider, it is important to understand the drivers for treatment decision-making in RRMM in later-line settings (3L+). Patient preferences in the treatment of MM have previously been evaluated [Citation18–21], as has physicians’ understanding of/alignment with patients’ preferences [Citation22,Citation23]. To date, no studies focusing on physicians’ treatment preferences for RRMM in later-line settings have been reported.
The current study assessed treatment preferences of US-based hematology-oncology specialists (HemOnc)/oncologists (Onc) in academic and community settings for patients with RRMM in later lines of therapy (3L or 4L+).
Materials & methods
Study overview
The study consisted of a qualitative phase to define the decision factors and associated levels that physicians considered when making treatment decisions for patients with RRMM. The subsequent quantitative phase assessed the decision-making processes of physicians treating patients with RRMM using a discrete choice experiment (DCE) incorporating the factors identified in the qualitative phase. A DCE allows factors and associated levels to be tested in different scenarios where respondents make trade-offs between factors when making a treatment decision, emulating real-world situations [Citation24,Citation25].
Qualitative phase
A targeted literature review (design shown in Supplementary Figure 1) was conducted to identify relevant treatment decision factors for currently marketed treatments for RRMM and the processes and language followed by physicians when treating patients with RRMM. Findings (shown in Supplementary Results, Supplementary Table 1) were used to develop the decision factors (e.g., attributes) and associated levels incorporated in the DCE, including progression-free survival (PFS), overall response rate (ORR) and OS. Additional factors included adverse events (AEs; keratopathy, thrombocytopenia and neutropenia), prior AEs, drug administration mode (oral alone or oral and intravenous infusion) and the frequency of administration and clinic visits.
Vignettes containing the factors identified in the literature search were developed to represent the different hypothetical profiles of patients with RRMM. Physicians’ understanding of the vignettes, clinical feasibility of the vignettes, and the relative importance of factors for treatment decision-making were assessed in qualitative interviews with seven HemOnc/Onc physicians treating patients with RRMM. Vignettes used in the DCE contained factors and associated levels most relevant to physicians when making a treatment decision for patients with RRMM in 3L, 4L or 5L, which were identified in the literature search and refined during preliminary testing of the vignettes by physicians.
DCE (quantitative phase)
The literature review and feedback from the qualitative phase informed the factors considered when deciding on a treatment plan for patients with RRMM (). Each factor had different associated levels specific to that factor for participating physicians in the USA to consider. For example, in the case of OS, the associated levels to that factor were 13, 9 or 6 months (). Physicians were presented with pairwise choice sets of two alternative hypothetical treatment profiles in an online survey shown in the DCE example in the Supplementary Methods. The choice sets consisted of combinations of the decision factors and associated levels shown in . Combinations of factors and associated levels that were infeasible in real-world situations were excluded from the survey. In each choice set, physicians were presented with a hypothetical patient requiring treatment in 3L, 4L and 5L, and were asked to make choices between two alternative treatments that had variation in levels of all the factors involved. The survey was designed to identify the main effects based on 20 choice sets, with two choices in each task and an estimated sample size of 200 respondents [Citation25,Citation26].
Table 1. Decision factors and levels in the discrete choice experiment task.
Selection of choice sets for physician completion used an orthogonal main effects plan, where all main effects were unrelated to each other and were optimal measures for main effects linear models. The fractional-factorial model consisted of two different treatment profiles with variations of the levels of each factor, which were presented in the 20 choice sets. Physicians traded off combinations of factors when asked to choose which alternative they preferred from each choice set. An example of the DCE scenarios is provided in the Supplementary Methods.
Eligible HemOnc/Onc physicians (n = 227) were identified using a screener containing the inclusion criteria developed for this study. Physicians who met the inclusion criteria in the screener were then invited to complete the DCE survey, provided on a separate web link, after providing consent. Socio-demographic and clinical information were collected during the screening process. Physicians’ inclusion and exclusion criteria for the qualitative and DCE phases are shown in Supplementary Table 2. Physicians who consented to take part in the study completed the online DCE survey using the QualtricsXM database platform (Qualtrics International, WA, USA).
Physician demographics collected included primary medical specialty, typical location where physician sees and treats patients with MM, typical number of MM patients seen in the last 12 months, and number of patients the physician has seen with newly diagnosed MM versus RRMM in the last 12 months. Additionally, physicians’ age, gender, and state of current license to practice medicine were collected. The preferred choice for each of the randomly selected choice sets presented to each physician and the relative importance of the factors presented were collected for each physician.
Following the DCE choice tasks, physicians ranked in order of importance the ten factors, from 1 (most important) to 10 (least important). Difficulties in making choices between scenarios, understanding of the scenarios, or understanding (or lack thereof) of the factors described within the scenarios were also collected in the DCE survey.
Statistical analyses
Multinomial logistic regression (MNL) models were performed for the DCE survey results to assess physician preferences when selecting treatments for RRMM patients and to understand the trade-offs between factors. For the analysis, 4L and 5L were combined into a single category (4L+) as analyses of the pilot DCE, and the DCE revealed that responses to these 2 categories were comparable. Preferences in 3L and 4L+ were analyzed separately. The regression estimates from the model are interpreted as the utilities associated with each respective factor level. The dependent variable was choice, whereas independent variables were the factor levels. A reference level for each factor was set to the lowest utility estimation. The MNL model used in these analyses is shown in the Supplementary Methods. The mean relative importance of the factors was estimated using the MNL model. Relative importance of factors was estimated and normalized to ascertain the factor’s relative importance, ranging from 0 to 100 on a normalized scale. These values allowed for comparisons to assess the relative importance of the factors, from most important to least important. MNL models included clinical and socio-demographic variables to control for differences in error variance and explore consistency of treatment choices.
The data obtained from the ranking exercise were described using frequencies and related percentages. A cumulative preference score, weighted for all the rankings each factor received at any level and the number of physicians ranking it at each level, was calculated. DCE data statistics were performed in Statistical Analysis System (SAS)® version 9.2 or higher (SAS Institutes, NC, USA).
Study ethics
This study did not capture physician-identifiable data, and a waiver exemption was obtained from Advarra Institutional Review Board, (MD, USA,) as the study was considered exempt from Institutional Review Board approval. This study was compliant with all applicable laws regarding subject privacy.
Data sharing statement
GlaxoSmithKline makes available anonymized individual participant data and associated documents from interventional clinical studies that evaluate medicines, upon approval of proposals submitted to www.clinicalstudydatarequest.com. To access data for other types of GlaxoSmithKline-sponsored research, for study documents without patient/participant-level data, and for clinical studies not listed, please submit an enquiry via the website.
Results
The literature search identified key decision factors for deciding on treatments in RRMM, which are summarized in Supplementary Figure 1. The key factors and associated levels identified as important in driving physicians’ treatment decisions were used to create and refine vignettes for a DCE survey. Further details of this process are shown in the Supplementary Results.
Discrete choice experiment phase
Physicians completed the DCE survey for either 3L (n = 83), 4L (n = 73) or 5L (n = 71) theoretical patient profiles. The socio-demographic and clinical variables of the 227 physicians completing the DCE are shown in . The variables were consistent among the physicians completing the surveys for the 3L, 4L or 5L patient profile groups, with the majority male and at least 43 years of age. Most physicians were from either the Midwest, Northeast, or Pacific regions of the USA. Overall, most physicians had a primary affiliation in either a community-based center, hospital or office (65%), compared with a university, academic or research hospital setting (35%). A similar number of 3L, 4L and 5L patients were treated in a 12-month period by the physicians who completed the DCE for the theoretical patients in 3L, 4L or 5L treatment decisions. There were no statistical differences between physician demographics (age, sex, affiliation, geographic region, specialty and the number of patients treated in a 12-month period, all p > 0.05). The numbers of patient profile groups that physicians typically treat in a 12-month period were all comparable apart from the distribution of 5L patients, which differed significantly.
Table 2. Socio-demographics and clinical variables of physicians completing the discrete choice experiment.
Efficacy
The primary objective of this study was to value the factors that determine physicians’ preferences when selecting 3L/4L+ treatments for patients with RRMM and to understand the trade-offs between these factors. In the DCE, when physicians were presented with different patient profiles, the highest valuation among all decision factors was placed on OS; p < 0.0001 for factor levels 13 months versus 6 months, and 9 months versus 6 months, for both 3L and 4L+ ( & ). For secondary and exploratory objectives, respectively, OS showed the highest relative importance in both 3L (38.1%) and 4L+ (36.5%; A), and in the physician’s explicit rankings, most physicians considered OS to be the most important factor for both 3L and 4L+ (B).
Physician preferences and trade-offs, when selecting treatments for relapsed/refractory multiple myeloma patients, were assessed using multinomial logistic regression models. The regression estimates from the model are interpreted as the utilities associated with each respected factor level. The dependent variable was choice, whereas independent variables were the factor levels. A reference level for each factor was set to the lowest utility estimation. Third line (n = 83).
i.v.: Intravenous; ref: Reference group.
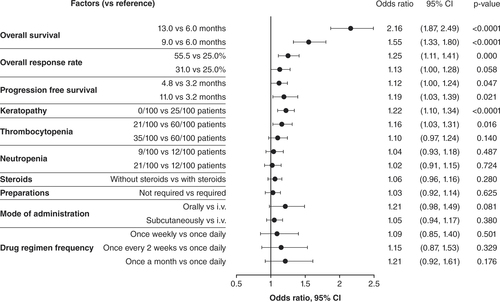
Physician preferences and trade-offs, when selecting treatments for relapsed/refractory multiple myeloma patients, were assessed using multinomial logistic regression models. The regression estimates from the model are interpreted as the utilities associated with each respected factor level. The dependent variable was choice, whereas independent variables were the factor levels. A reference level for each factor was set to the lowest utility estimation. Fourth line+ (n = 144).
i.v.: Intravenous; ref: Reference group.
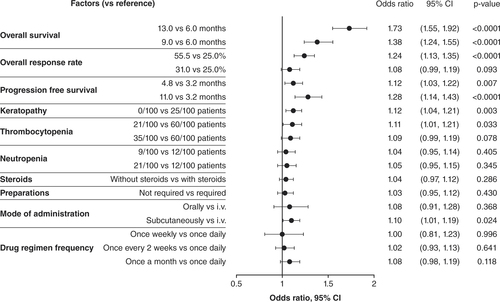
(A) Mean relative importance estimates are calculated from the ultinomial logistic regression models used for the primary analysis. Part-worth values were scaled and normalized to allow for comparisons to assess the relative importance of the factors from most important to least important. Relative importance estimates across the factors sum to 100%. (B) Physician treatment factors considered most important through explicit ranking in relapsed/refractory multiple myeloma 3L (n = 76) and 4L+ (n = 140). Explicit ranking level of importance was from 1 = most important to 10 = least important (full set shown in ).
3L: Third line; 4L: Fourth line.
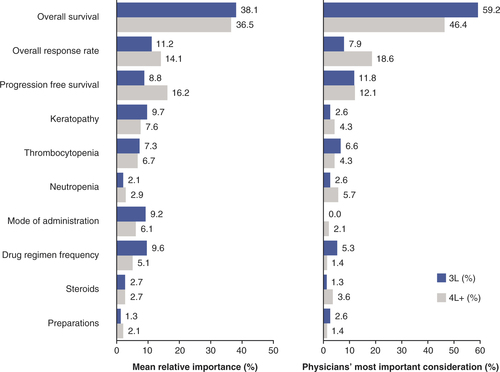
The other efficacy measures, increased ORR (55.5 vs 25.0%: 3L, p = 0.000; 4L+, p < 0.0001) and PFS (11.0 months vs 3.2 months: 3L, p = 0.021; 4L+, p < 0.0001), were also important in physician decision-making for treating RRMM ( & ). For relative importance of treatment factors, 4L+ PFS (16.2%) was the next most important efficacy measure after OS, followed by ORR (14.1%); in 3L, ORR was the second most important (11.2%), with PFS at 8.8% (A). When physicians explicitly ranked their most important treatment factor, ORR was ranked highest by over twice as many physicians in 4L+ as in 3L (B). In the cumulative preference scores considering all rankings received for each factor, PFS was more important than ORR at 3L, while ORR and PFS were equally important at 4L+ ().
The number of physicians who ranked the level of importance for each factor at each level was combined to give the overall explicit preferences considering all levels. Explicit ranking level of importance was from 1 = most important to 10 = least important. n = 216. Data were missing for 11 physicians.
3L: Third line; 4L: Fourth line.
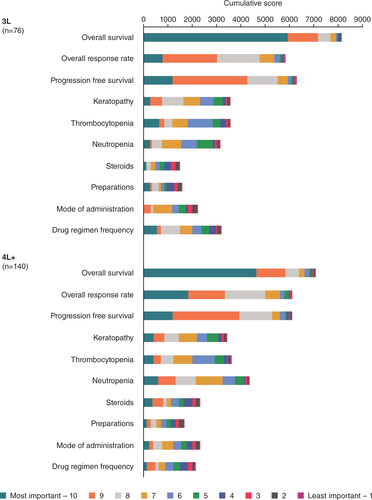
Adverse events
AEs were rated as less important than efficacy in all groups. Contrary to efficacy, where highly rated factors were preferable, in the consideration of AEs a higher rating indicated that these were the events that physicians most wanted to avoid when treating patients. Physicians preferred treatments with fewer keratopathy events (3L, p < 0.0001 []; 4L+, p = 0.003 []), and keratopathy had a mean relative importance of 9.7% in 3L and 7.6% in 4L+ (A). Thrombocytopenia risk reduction (21/100 vs 60/100 patients) was favored in treatment choices (3L, p = 0.016 []; 4L+, p = 0.033 []). The mean relative importance of preventing thrombocytopenia was 7.3% in 3L and 6.7% in 4L+ (A). Explicit physician preference rankings did not rate keratopathy as the most important of the AEs to avoid in 3L or 4L+ (B); thrombocytopenia at 3L and neutropenia at 4L+ were the most highly ranked AEs in treatment decisions (B). In the cumulative preference scores, keratopathy and thrombocytopenia were similar considerations at 3L, but neutropenia was more important at 4L+ for treatment decisions ().
Mode of administration, regimen frequency, use of steroids & preparations/other factors in decision making
The mean relative importance of the drug regimen frequency and mode of administration in 3L were 9.6 and 9.2%, respectively (A). In 4L+, mode of administration had a mean relative importance of 6.1% and regimen frequency was 5.1% (A). In the physicians’ explicit preferences, mode of administration was ranked most important more frequently than regimen frequency (B).
The mean relative importance of regimens requiring use of steroids or without use of steroids was equal in 3L and 4L+ (2.7%, A). When explicitly ranked by physicians, avoiding the use of steroids was more important in 4L+ than in 3L (B).
The requirement for preparation in treatment decisions was of lowest mean relative importance in 3L (1.3%, A) and joint lowest in 4L+ (2.1%, with neutropenia, A) and was explicitly ranked among the least important factors in 3L and 4L+ ().
Discussion
HemOnc/Onc preferences for treatment choices evaluated in the current DCE survey identified factors important to physicians in the USA when choosing treatments for patients with RRMM. Physicians viewed OS as the most important factor in decision-making, considered as having the highest relative importance and the most important factor in the physicians’ explicit rankings for both 3L and 4L+. Overall, physicians preferred treatment options with longer survival, higher ORR, longer PFS and reduced risk of keratopathy and thrombocytopenia. Lower consideration was given to the use of steroids, preparations, mode of administration and drug regimen frequency.
There are no studies to date that examine only physicians’ treatment preferences for RRMM. However, placing a high value on patient survival in RRMM is consistent with previous reports comparing physician treatment preferences to patients’ own views [Citation18,Citation27,Citation28]. Patients with MM have previously categorized OS as the most important consideration in treatment choice [Citation18,Citation27,Citation28], a finding that was consistent with a DCE examining the preferences of patients with MM, their caregivers, physicians and nurses [Citation22]. In contrast to our study, which identified the priorities of physicians, a study on patient preferences regarding PI-based combination therapy in later-setting RRMM identified the top priorities as reduced therapy regimen frequency or least invasive mode of administration, followed by time to disease progression [Citation21].
Most notable was avoidance of keratopathy, which had a similar relative importance to PFS in 3L but was considered ∼50% less important than PFS in 4L+. After OS, which is regarded as the most important beneficial factor in treatment decision-making at 4L+, physicians may decide on a treatment plan that accepts a higher keratopathy risk if PFS can be lengthened. This suggests that there may be a benefit:risk trade-off as treatment progresses, with the risk of high incidence of keratopathy possibly acting as a deterrent to using some treatments in earlier lines of therapy, or the preference to avoidance of neutropenia in later lines of therapy when patients may be more susceptible to infections [Citation29]. Variation in physicians’ perceptions of, or experience with, different risk levels of grade 3–4 AEs may have also influenced physicians’ preferences for treatment choice. During the qualitative phase of the study, physicians described varying experiences with, and understanding of, keratopathy. Opinions varied on the acceptability of grade 3–4 keratopathy as an AE and how this varied between different lines of treatment (Supplementary Results). Corneal events such as keratopathy have been observed with use of antibody–drug conjugates (ADCs) for the treatment of various cancers [Citation30–38]. The emergence of keratopathy as an AE due to the use of ADCs as therapeutics is relatively recent in comparison to the incidence of thrombocytopenia, a known side effect of many treatments for MM [Citation16,Citation39,Citation40] and other diseases [Citation32,Citation37]. In contrast to physicians’ varying views, collected in the qualitative phase, regarding the risk of keratopathy, their opinions regarding thrombocytopenia risk were more confident due to greater experience of managing thrombocytopenia in patients (Supplementary Results).
Real-world studies evaluating treatment patterns in MM have shown that bortezomib is commonly used in first and subsequent lines of treatment in combination with other agents [Citation41,Citation42]. Thrombocytopenia is among the most frequent AEs associated with bortezomib treatment [Citation43]. Emerging real-world studies on treatment patterns in RRMM have identified that patients are frequently retreated with agents, even if they have previously shown refractoriness to them [Citation44–46]. Given the reliance on retreating patients with treatment classes resulting in thrombocytopenia as a side effect, it is plausible that physicians have become accustomed to mitigating the effects of thrombocytopenia and will favor this as an option instead of the less familiar corneal event of keratopathy. Management of keratopathy during treatment with ADCs through dose delays, dose reductions and avoiding the use of contact lenses [Citation35,Citation36,Citation47] permits long-lasting effects with these agents [Citation48], and physicians may become more tolerant of the risk of keratopathy as these mitigation strategies become more familiar.
In this study, physicians rated the use of steroids in treatment regimens as one of the least important factors when making treatment decisions, which appears to be in contrast with patients’ views on steroid use. Patients have reported that steroid-associated side effects, including sleep and mood disturbances [Citation27], are detrimental to their quality-of- life, along with muscle atrophy and adverse effects on glucose homeostasis [Citation49]. Dexamethasone and prednisone-based regimens result in raised blood glucose levels through multiple mechanisms affecting glucose metabolism [Citation50], which can result in increased infections, diabetic eye disease, and eventual diabetic neuropathy in at-risk myeloma patients with diabetes [Citation51,Citation52]. Corticosteroids have also been linked to side effects including fluid-retention, bone loss and insomnia [Citation53]. Faced with these additional considerations, patients may prefer to avoid taking steroids, whereas physicians’ major focus is prescribing regimens that promote the best OS, PFS or ORR.
The results presented here have identified consistency in multiple factors involved in 3L and 4L+ treatment decision-making by physicians and may help inform physicians when making decisions about therapies for patients with RRMM. Limitations to this study include the fact that although self-reporting of data can lead to information bias, the study design included anonymized data collection online to reduce this and offset potential skewing of the dataset. When recruiting physicians to the study, efforts were made to ensure that the population of participating physicians was as generalizable as possible although all were in the USA. However, an additional limitation could be selection bias due to the voluntary completion of the study surveys resulting in a study population that is not truly representative of the general population of HemOnc/Onc physicians. Physician characteristics were recorded and are reported here to reflect this. It is possible that there are other considerations in physicians’ decisions on treatment, including additional non-hematologic AEs, that were not captured in this study, though this risk was minimized by basing the factors captured on a targeted literature search, feedback from the qualitative interviews, and collecting their relative importance in the study. Inclusion of inaccuracies in the data was minimized by determining that physicians had understood the factors in the DCE. The final potential limitation of this study relates to the high number of predictors in the MNL model producing a risk of multiplicity, a caveat that applies to all regression models and is not otherwise controlled.
The study reported here found that physicians placed highest importance on efficacy when treating both 3L and 4L+ patients with RRMM. Physicians preferred decreased risks for grade 3–4 keratopathy and thrombocytopenia when choosing later-line therapies for RRMM. Thrombocytopenia is a side effect that has been observed and managed by physicians for many years, while MM treatment-associated keratopathy has emerged only recently with the use of ADCs. It is noteworthy that these two AEs were given similar importance by physicians during their decision-making processes. As effective physician education strategies and networks with eye care professionals are further developed to ameliorate treatment-related keratopathy, the importance of this factor may decline. Although other non-hematological AEs or newly emerging AEs were not included in this study, the importance of dissemination of mitigation and management strategies for these as they become apparent has been highlighted by physicians.
In conclusion, the current study assessed later-line RRMM treatment preferences of US HemOnc/Onc specialists in academic and community settings and enhances our understanding of preferences that inform clinical decision-making in these patients. This information is important to inform new treatment strategies with novel agents and highlight where additional information on supportive treatment for these emerging therapies would be beneficial.
Conclusion
Understanding the factors involved in physicians’ treatment choices for patients with RRMM is important given the emergence of new therapies with novel mechanisms of action. This study has highlighted the prominence of efficacy measures for physicians and how the importance of ORR and PFS change in later lines of therapy for RRMM. While physicians in the USA place lower levels of importance on AEs in later lines of therapy and more focus on efficacy measures, decisions factors across all lines of therapy include avoiding AEs for patients. The prior experience of physicians in managing AEs like thrombocytopenia, compared with lack of experience with AEs emerging with the more recently approved therapies, which include ocular symptoms for ADCs and cytokine release syndrome in CAR T-cell therapy, may be a determining factor for physicians when making treatment decisions. For emerging therapies, understanding of mitigation strategies and management of AEs alongside efficacy may be of predominant importance for adoption of new therapies in RRMM patients.
Factors involved in physician treatment decisions for therapy in patients with relapsed/refractory multiple myeloma (RRMM) were initially identified using a literature search, and treatment scenarios relevant to real-world situations were developed and reviewed by qualitative physician interviews.
Treatment scenarios were then finalized and tested using a discrete choice experiment.
Physicians valued efficacy most highly when treating patients with RRMM in later lines of therapy (3–5) in discrete choice experiments.
The value physicians placed on progression-free survival and overall response rate differed between lines of therapy.
Physicians preferred treatments with fewer adverse events.
Adverse events became less of a consideration for treatment decisions as the line of therapy increased.
In treatment decisions for patients with RRMM, reduced risk of thrombocytopenia and keratopathy had similar relative mean importance.
In the qualitative phase, physicians felt more confidence in their ability to manage adverse events they had previously treated.
Physicians placed the lowest priority on the regimen frequency, the need for steroid use, or drug preparation requirements.
Author contributions
All authors contributed to writing this manuscript. L Batchelder contributed to the conception or design of this study, acquisition of data and data analysis and interpretation. CC Chen, V Divino, AM Rodriguez, N Boytsov, EM Maiese, C Hogea and T Buckingham contributed to the conception or design of this study and data analysis and interpretation. S Philpott contributed to the acquisition of data and data analysis and interpretation.
Financial & competing interests disclosure
L Batchelder, CC Chen, V Divino, S Philpott and AM Rodriguez are employees of IQVIA Inc., which has received funding for this study from GlaxoSmithKline. N Boytsov is an employee of, and hold stocks and shares in, GlaxoSmithKline. T Buckingham, EM Maiese and C Hogea were employees and held stocks and shares in GlaxoSmithKline at the time of the study. The authors have no other relevant affiliations or financial involvement with any organization or entity with a financial interest in or financial conflict with the subject matter or materials discussed in the manuscript apart from those disclosed.
Writing assistance was provided by E Walsby of Fishawack Indica Ltd, part of Fishawack Health, and funded by GlaxoSmithKline.
Ethical conduct of research
This study did not capture physician-identifiable data and a waiver exemption was obtained from Advarra Institutional Review Board, Columbia, MD, USA, as the study was considered exempt from Institutional Review Board approval. This study was compliant with all applicable laws regarding subject privacy.
Data sharing statement
GlaxoSmithKline makes available anonymized individual participant data and associated documents from interventional clinical studies which evaluate medicines, upon approval of proposals submitted to www.clinicalstudydatarequest.com. To access data for other types of GlaxoSmithKline-sponsored research, for study documents without patient/participant-level data, and for clinical studies not listed, please submit an enquiry via the website.
supplement
Download MS Word (166.2 KB)Supplementary data
To view the supplementary data that accompany this paper please visit the journal website at: www.futuremedicine.com/doi/suppl/10.2217/fon-2022-0378
Additional information
Funding
References
- Kumar SK , RajkumarV, KyleRAet al. Multiple myeloma. Nat. Rev. Dis. Primers.3, 17046 (2017).
- Mikhael J . Treatment options for triple-class refractory multiple myeloma. Clin. Lymphoma Myeloma Leuk.20(1), 1–7 (2020).
- LeBlanc R , BergstromDJ, CoteJ, KotbR, LouzadaML, SutherlandHJ. Management of myeloma manifestations and complications: the cornerstone of supportive care: recommendation of the Canadian Myeloma Research Group (formerly Myeloma Canada Research Network) consensus guideline Consortium. Clin Lymphoma Myeloma Leuk.2021.
- Michels TC , PetersenKE. Multiple myeloma: diagnosis and treatment. Am. Fam. Physician95(6), 373–383 (2017).
- Raab MS , PodarK, BreitkreutzI, RichardsonPG, AndersonKC. Multiple myeloma. Lancet374(9686), 324–339 (2009).
- van de Donk N , UsmaniSZ. CD38 antibodies in multiple myeloma: mechanisms of action and modes of resistance. Front. Immunol.9, 2134 (2018).
- Gandhi UH , CornellRF, LakshmanAet al. Outcomes of patients with multiple myeloma refractory to CD38-targeted monoclonal antibody therapy. Leukemia.33(9), 2266–2275 (2019).
- Verelst SGR , BlommesteinHM, DeGroot Set al. Long-term outcomes in patients with multiple myeloma: a retrospective analysis of the Dutch Population-based HAematological Registry for Observational Studies (PHAROS). Hemasphere.2(4), e45 (2018).
- Yong K , DelforgeM, DriessenCet al. Multiple myeloma: patient outcomes in real-world practice. Br. J. Haematol.175(2), 252–264 (2016).
- Dimopoulos MA , MoreauP, TerposEet al. Multiple myeloma: EHA-ESMO Clinical Practice Guidelines for diagnosis, treatment and follow-up(dagger). Ann. Oncol.32(3), 309–322 (2021).
- Moreau P , KumarSK, SanMiguel Jet al. Treatment of relapsed and refractory multiple myeloma: recommendations from the International Myeloma Working Group. Lancet Oncol.22(3), e105–e118 (2021).
- Durer C , DurerS, LeeSet al. Treatment of relapsed multiple myeloma: evidence-based recommendations. Blood Rev.39, 100616 (2020).
- Sonneveld P , BroijlA. Treatment of relapsed and refractory multiple myeloma. Haematologica101(4), 396–406 (2016).
- Chim CS , KumarSK, OrlowskiRZet al. Management of relapsed and refractory multiple myeloma: novel agents, antibodies, immunotherapies and beyond. Leukemia32(2), 252–262 (2018).
- Dingli D , AilawadhiS, BergsagelPLet al. Therapy for relapsed multiple myeloma: Guidelines From the Mayo Stratification for Myeloma and Risk-Adapted Therapy. Mayo Clin. Proc.92(4), 578–598 (2017).
- Lonial S , LeeHC, BadrosAet al. Belantamab mafodotin for relapsed or refractory multiple myeloma (DREAMM-2): a two-arm, randomised, open-label, phase 2 study. Lancet Oncol.21(2), 207–221 (2020).
- Rajkumar SV , KumarS. Multiple myeloma current treatment algorithms. Blood Cancer J.10(9), 94 (2020).
- Fifer S , GalinskyJ, RichardS. Myeloma patient value mapping: a discrete choice experiment on myeloma treatment preferences in the UK. Patient Prefer Adherence.14, 1283–1293 (2020).
- Muhlbacher AC , LinckeHJ, NublingM. Evaluating patients’ preferences for multiple myeloma therapy, a Discrete-Choice-Experiment. Psychosoc. Med.5, Doc10 (2008).
- Tariman JD , DoorenbosA, ScheppKG, SinghalS, BerryDL. Older adults newly diagnosed with symptomatic myeloma and treatment decision making. Oncol. Nurs. Forum.41(4), 411–419 (2014).
- Wilke T , MuellerS, BauerSet al. Treatment of relapsed refractory multiple myeloma: which new PI-based combination treatments do patients prefer? Patient Prefer Adherence. 12, 2387–2396 (2018).
- Fifer SJ , HoKA, LybrandS, AxfordLJ, RoachS. Alignment of preferences in the treatment of multiple myeloma - a discrete choice experiment of patient, carer, physician, and nurse preferences. BMC Cancer.20(1), 546 (2020).
- Muhlbacher AC , NublingM. Analysis of physicians’ perspectives versus patients’ preferences: direct assessment and discrete choice experiments in the therapy of multiple myeloma. Eur. J. Health Econ.12(3), 193–203 (2011).
- Bridges JF , HauberAB, MarshallDet al. Conjoint analysis applications in health–a checklist: a report of the ISPOR Good Research Practices for Conjoint Analysis Task Force. Value Health.14(4), 403–413 (2011).
- Reed Johnson F , LancsarE, MarshallDet al. Constructing experimental designs for discrete-choice experiments: report of the ISPOR Conjoint Analysis Experimental Design Good Research Practices Task Force. Value Health.16(1), 3–13 (2013).
- de Bekker-Grob EW , DonkersB, JonkerMF, StolkEA. Sample size requirements for discrete-choice experiments in healthcare: a practical guide. Patient8(5), 373–384 (2015).
- Parsons JA , GreenspanNR, BakerNA, McKillopC, HicksLK, ChanO. Treatment preferences of patients with relapsed and refractory multiple myeloma: a qualitative study. BMC Cancer.19(1), 264 (2019).
- Postmus D , RichardS, BereNet al. Individual trade-offs between possible benefits and risks of cancer treatments: results from a stated preference study with patients with multiple myeloma. Oncologist23(1), 44–51 (2018).
- Balmaceda N , AzizM, ChandrasekarVTet al. Infection risks in multiple myeloma: a systematic review and meta-analysis of randomized trials from 2015 to 2019. BMC Cancer.21(1), 730 (2021).
- Deklerck E , DenysH, KrepsEO. Corneal features in trastuzumab emtansine treatment: not a rare occurrence. Breast Cancer Res. Treat.175(2), 525–530 (2019).
- Thompson JA , MotzerRJ, MolinaAMet al. Phase I Trials of Anti-ENPP3 Antibody-Drug Conjugates in Advanced Refractory Renal Cell Carcinomas. Clin. Cancer Res.24(18), 4399–4406 (2018).
- Donaghy H . Effects of antibody, drug and linker on the preclinical and clinical toxicities of antibody-drug conjugates. MAbs.8(4), 659–671 (2016).
- Eaton JS , MillerPE, MannisMJ, MurphyCJ. Ocular adverse events associated with antibody-drug conjugates in human clinical trials. J. Ocul. Pharmacol. Ther.31(10), 589–604 (2015).
- Farooq AV , DegliEsposti S, PopatRet al. Correction to: Corneal Epithelial Findings in Patients with Multiple Myeloma Treated with Antibody-Drug Conjugate Belantamab Mafodotin in the Pivotal, Randomized, DREAMM-2 Study. Ophthalmol. Ther.9(4), 913–915 (2020).
- Gan HK , ReardonDA, LassmanABet al. Safety, pharmacokinetics, and antitumor response of depatuxizumab mafodotin as monotherapy or in combination with temozolomide in patients with glioblastoma. Neuro. Oncol.20(6), 838–847 (2018).
- Goss GD , VokesEE, GordonMSet al. Efficacy and safety results of depatuxizumab mafodotin (ABT-414) in patients with advanced solid tumors likely to overexpress epidermal growth factor receptor. Cancer124(10), 2174–2183 (2018).
- Lassman AB , vanden Bent MJ, GanHKet al. Safety and efficacy of depatuxizumab mafodotin + temozolomide in patients with EGFR-amplified, recurrent glioblastoma: results from an international phase I multicenter trial. Neuro. Oncol.21(1), 106–114 (2019).
- Masters JC , NickensDJ, XuanD, ShazerRL, AmanteaM. Clinical toxicity of antibody drug conjugates: a meta-analysis of payloads. Invest. New Drugs36(1), 121–135 (2018).
- Lonial S , WallerEK, RichardsonPGet al. Risk factors and kinetics of thrombocytopenia associated with bortezomib for relapsed, refractory multiple myeloma. Blood106(12), 3777–3784 (2005).
- Palumbo A , FalcoP, FalconeAet al. Melphalan, prednisone, and lenalidomide for newly diagnosed myeloma: kinetics of neutropenia and thrombocytopenia and time-to-event results. Clin. Lymphoma Myeloma.9(2), 145–150 (2009).
- Mohty M , TerposE, MateosMVet al. Multiple myeloma treatment in real-world clinical practice: results of a prospective, multinational, noninterventional study. Clin. Lymphoma Myeloma Leuk.18(10), e401–e419 (2018).
- Weil C , GelersteinS, SharmanMoser Set al. Real-world epidemiology, treatment patterns and survival of multiple myeloma patients in a large nationwide health plan. Leuk. Res.85, 106219 (2019).
- Cengiz Seval G , BeksacM. The safety of bortezomib for the treatment of multiple myeloma. Expert Opin. Drug Saf.17(9), 953–962 (2018).
- Ribbands A , GorshB, BaileyAet al. Current clinical practice and decision-making in multiple myeloma treatment in the United States of America: a real-world survey. Blood138(Suppl. 1), 3001–3001 (2021).
- Wang F , GorshB, DerSarkissianMet al. Treatment Patterns and Outcomes of Patients with Double-Class Refractory or Triple-Class Refractory Multiple Myeloma: A Retrospective US Electronic Health Record Database Study. Blood138(Suppl. 1), 2705–2705 (2021).
- Wang PF , SansburyL, DerSarkissianMet al. MM-123: Real-World Study of Treatment Patterns in Heavily Pretreated Relapsed/Refractory Multiple Myeloma (RRMM) in the US. Clinical Lymphoma, Myeloma and Leukemia.21, S423 (2021).
- Lonial S , NookaAK, ThulasiPet al. Management of belantamab mafodotin-associated corneal events in patients with relapsed or refractory multiple myeloma (RRMM). Blood Cancer J.11(5), 103 (2021).
- Lonial S , LeeHC, BadrosAet al. Longer term outcomes with single-agent belantamab mafodotin in patients with relapsed or refractory multiple myeloma: 13-month follow-up from the pivotal DREAMM-2 study. Cancer127(22), 4198–4212 (2021).
- Burwick N , SharmaS. Glucocorticoids in multiple myeloma: past, present, and future. Ann. Hematol.98(1), 19–28 (2019).
- Issa ZA , ZantoutMS, AzarST. Multiple myeloma and diabetes. ISRN Endocrinol.2011, 815013 (2011).
- Ahmed YA , EltayebA. Clinical challenges: myeloma and concomitant type 2 diabetes. Int. J. Hematol. Oncol. Stem Cell Res.7(1), 34–41 (2013).
- Snowden JA , GreenfieldDM, BirdJMet al. Guidelines for screening and management of late and long-term consequences of myeloma and its treatment. Br. J. Haematol.176(6), 888–907 (2017).
- Terpos E , MikhaelJ, HajekRet al. Management of patients with multiple myeloma beyond the clinical-trial setting: understanding the balance between efficacy, safety and tolerability, and quality of life. Blood Cancer J.11(2), 40 (2021).