Abstract
Quantitative structure activity relationship analysis was performed on a series of xanthone derivatives to establish the structural features required for α-glucosidase inhibitory activity. The computational and statistical analysis was performed with V life MDS (Molecular Design Suite) and Statistica software. The selected models show significant predictive power, stability, and reliability in terms of cross-validated correlation coefficient (Q2cv > 0.74 and Q2test > 0.5) and other validation parameters. The results show that the SaaaC count, MMFF_6 and dipole moment are mainly contributed for the activity along with the hydrophobicity descriptors. It describes that heteroatoms (oxygen atom connected with carbon atom) in the molecules are favourable for α-glucosidase inhibitory activity. The E-state count descriptor suggests that when carbon atoms connected with three aromatic bonds and hydrogen or other atoms are favourable for the activity. The SAHA and SAMH descriptors show that the hydrophilic area in the molecule is important for the activity while high hydrophilicity is unfavourable for the activity. This study concluded that hydrophilic, polar and/or electron negative groups, which are responsible for hydrogen bonding and interaction with the enzyme for favourable activity.
Introduction
Acquired immunodeficiency syndrome caused by human immunodeficiency virus (HIV) is one of the most important life threatening diseases worldwide. HIV envelope contains two glycoproteins, the surface glycoprotein (gp120) and the transmembrane glycoproteins (gp41) that are non-covalently linked in an oligomeric structure. Basically, these glycoproteins are the cleavage product of the gp160 precursor and the gp120 mediates HIV binding while gp41 mediates fusion with CD4+ cellsCitation1–3.
The glycosylation pathway (including the transfer of the glycon precursor onto the nascent protein and the subsequent glucose trimming) is needed for the processing, the folding, and the routing of the precursor gene (Env). Glucosidases catalyze the final step in the digestive process of carbohydrates i.e., the hydrolysis of a glycosidic bond in oligosaccharides. They are responsible for the catalytic cleavage of a glycosidic bond with specificity, depending on the number of monosaccharides, the position of cleavage site, and the configuration of the hydroxyl groups in the substrateCitation4,Citation5. Due to the catalytic role in digesting carbohydrate substrates, α-glucosidase has also been the object of a special interest by the pharmaceutical research community for other carbohydrate-mediated diseases such as cancer, viral infections, diabetics, and hepatitisCitation6–10.
Inhibition of these glucosidases, especially α-glucosidase (EC 3.2.1.20), has a profound effect on the glycon structure, which consequently affects the maturation, transport, secretion, and function of glycoproteins and could alter the cell–cell or the cell–virus recognition processCitation11. α-Glucosidase inhibitors such as DNJ, NB-DNJ and castanospermine are potent inhibitors of the HIV replication and HIV-mediated syncytium formation in vitro. NB-DNJ is known to impair the processing of gp120 associated N-linked oligosaccharides, resulting in predominantly neutral glucosylated precursor N-glyconCitation2,Citation12.
Quantitative structure activity relationship (QSAR) is one of the tools that link biological activity data with physiochemical descriptors of molecules. The aim of a developed QSAR model is to be able to predict the activity of previously untested structures/compounds and understanding the influence of chemical descriptors on the biological activityCitation13–15.
Related to the foregoing and continuation of our group effort in the design and development of novel anti-HIV agents, the present work represents an effort to examine the applicability of QSAR approaches to the series of xanthone derivatives in α-glucosidase inhibitory activity. Xanthones, readily isolated from some medicinal plants, have been reported to exhibit several important biological activities, such as antitumor, anti-inflammatory, anti-thrombotic, and eukaryote kinase effectsCitation16–19. In this present work, a wide number of descriptors are used to construct QSAR models to interpret the structural features of the compounds required for α-glucosidase inhibitory activity. The literature search shows that only some QSAR study has been done on this target, using simple 2D and 3D descriptorsCitation16,Citation20,Citation21. Hence, to explore the additional structural features determining the binding affinity and mechanism of inhibition, more topological descriptors (mainly electrotopological descriptors), atom count descriptors and hydrophobicity descriptors were considered in this QSAR study. These atomic level topological indices characterize the structural environment of each atom type in a molecule and offer the possibility of understanding the role of individual atomic types or groups, particularly functional groups such as -OH, -COOH, -NH2, etc., in a molecule for binding to the active site of α-glucosidase enzyme. The electrotopological state indices, based on the chemical graph theory have been found useful in several QSAR studiesCitation22–24. They have been used to develop models for many activities and properties, in both their atom level and atom type forms, and they represent the potential non-covalent intermolecular interaction between the molecules and α-glucosidase enzymeCitation25,Citation26.
Experimental
The data set for the present QSAR analysis was obtained from the literature published by Yan, et alCitation16,Citation27. The data set contains 43 α-glucosidase inhibitors with defined activity against Baker’s yeast and represented as concentration needed to inhibit the α-glucosidase activity by 50% (IC50) (). The reported µM inhibitory concentration was converted to molar concentration and then to−logIC50 (pIC50) to make the concentration proportional to free energy of interaction of the compounds with the receptor and to reduce the skewness of the data set.
Table 1. Structure and α-glucosidase inhibitory activity of xanthone derivatives considered for the study.
The structure of the xanthone derivatives considered for this present study was sketched on Chemdraw ultra 11.0.1 software and converted to its 3D structure in Chem 3D module of ChemBioOffice 2008 softwareCitation28. The structure and its inhibitory concentration are given in . The energy optimization of the molecules was performed by batch calculation in V life MDS (Molecular Design Suite)Citation29 software using Universal Force Field. The physicochemical descriptors include 239 physicochemical parameters, 700 alignment type parameters, and 99 atoms types count descriptors that were calculated for the energy-optimized molecules using the same software. Molecular descriptors have been routinely used for quantitative description of structural and physicochemical properties of molecules. In this study, more than 300 calculated descriptors (2D) were subjected to sequential multiple linear regression analysis, in order to establish a correlation between physicochemical parameters and α-glucosidase inhibition. The QSAR models were built using the QSAR module of V life software and the Statistica 8.0 statistical softwareCitation30. The data set was divided into training and test set in order to perform the QSAR analysis. The test set compounds (~25%) were selected by randomly in V life software. The analysis was performed on different training and test set compounds, and the best variables were selected by partial least squares. In order to reduce the chance correlation of the descriptors while developing the QSAR models, care was taken to reduce the intercorrelation between the descriptor below 0.5 and the rule of thumb was adopted for limiting the number of descriptors in the model (three to six times the number of parameters under consideration)Citation31. Since a multiple linear model with a large number of variables can be too cumbersome to use, we have used stepwise regression to refine the model by determining the relative importance of each variable and its statistical significance. Furthermore, an equation containing an excessive number of independent variables is likely to be overfitted. In this present study, the upper limit (six compounds for a descriptor) was adopted for limiting the number of descriptors in the model.
Any QSAR modeling should ultimately lead to statistically robust models capable of making accurate and reliable predictions of biological activities of new compounds. The derived models were validated to examine the self-consistency between them, which implies a quantitative assessment of the model robustness and its predictive power. The predictive ability of the model is also quantified in terms of the corresponding leave-one-out cross-validated parameters, Q2, predicted residual sum of squares (PRESS), SPRESS, and standard deviation of the error of prediction (SDEP). To obtain information on the reliability of prediction, a validation (test) data set was randomly selected (~25%) of the whole data set.
Results and discussion
Multiple regression analysis was performed to correlate the physicochemical descriptors with the α-glucosidase inhibitory activity of xanthone derivatives. The best-derived QSAR models were selected on the basis of the highest correlation coefficient (R), F statistic, t-test and the statistical relevance of the incorporated descriptors. The selected significant models are given below.
Model 1
pIC50 = 0.0125 (±0.0016) SAHA +0.3828 (±0.0338) SaaaC count (SCC) −0.1100 (±0.0263) dipole moment (DM) +3.7139 (±0.0804).
N = 43, R = 0.9056, R2 = 0.8202, AdjR2 = 0.8063, F(3,39,0.01) = 59.2920 (4.3130), Q2 = 0.7782, standard error of estimation (SEE) = 0.2032, t(39,0.0005) = 46.1840 (3.2905), p = 0.0000, r2se = 0.2032, q2se = 0.2256, β-value for DM = −0.3100, SCC = 0.7700, and SAHA = 0.5660.
Model 2
pIC50 = 0.4065 (±0.0665) MMFF_6 −13.9314 (±4.2734) SAMH +0.3863 (±0.0411) SCC +0.0674 (±0.0280) Z-CompDipole (ZCD) +1.3507 (±0.4874).
N = 32, R = 0.9135, R2 = 0.8344, AdjR2 = 0.8099, F(4,27,0.01) = 34.0140 (4.1060), Q2 = 0.7357, SEE = 0.2130, t(27,0.0005) = 2.7713 (3.6896), p = 0.0100, r2 se = 0.2129, q2se = 0.2691, pred_r2 = 0.6650, pred_r2se = 0.2296, β-value for ZCD = 0.1970, SCC = 0.7630, SAMH = −0.2600, and MMFF_6 = 0.4930.
In the analysis, we have also tried to develop significant models with additional descriptors and different training set to explain the inhibitory activity of the compounds. Unfortunately, other developed models possessed poor validation parameters; hence, we rejected those models for further analysis and discussion (see Supplementary material).
In the models, N is number of compounds contributed to build the respective model. The figures within the parenthesis following the regression coefficient terms are the standard error of the regression terms and the constants. R is the correlation coefficient and R2 is the squared correlation coefficient, describing the relative measure of the quality of fit by the regression equation. They explain the variation in the observed data (experimental) and their value varies from −1 to +1. The closer the R or R2-value to 1, the better the goodness of fit of the regression equation.
F is the Fischer ratio and it represents the ratio between the variance of calculated and observed activities. The value within parentheses that follows the calculated F-value is the tabulated value for 99% significance. The F-value indicates that the regression relations are not a chance fit but are a significant occurrence.
The adjusted R2 is interpreted similarly to R2 but the adjusted R2 takes into consideration the number of degrees of freedom (Equation 1).
where residual SS stands for error sum of squares and total SS for total sum of squares. It is the most widely used measure of the ability of a QSAR model to reproduce the data (goodness of fit). t Is the Student t-test and the value in the parenthesis after the calculated value, is the tabulated t-value at 0.0005 confidence level. The F statistics and the t-value of the selected models (1 and 2) have a large margin of difference from tabulated values at 0.01 (99%) and 0.0005 (99.95%), respectively, which shows that the models are significant for further study.
The β-coefficient value is the standard regression coefficients that would have been obtained by adjusting the variable values to a mean of 0 and a standard deviation of 1. It also allows us to compare the relative contribution of each independent variable in the prediction of the dependent variablesCitation30.
Q2 is the cross-validated correlation coefficient, which provides the statistical significance and predictability of the model. Q2 is used as a criterion of both robustness and predictive ability of the model. A high Q2 in the models is suggesting that the models will be useful for meaningful predictionsCitation32–34. Leave-one-out cross-validation techniques were used to find out the predictive power of the model. It may be considered that a high Q2 (for instance Q2 > 0.5) is an indicator, or even the ultimate proof, that the model is highly predictiveCitation32–35. Cross-validated correlation coefficients of the derived models are greater than 0.74 for training set and complete data set (). This shows that the selected models have sufficient predictive power and self-consistency. The relationship between the predicted and observed activity values are represented graphically in and ). The low SPRESS and SDEP value for the models developed with the training set and the complete data set reveals the models are statistically significant for the activity prediction (). It is supported by the PRESS and SDEP value obtained from the test set compounds is <2 confirm that the above-mentioned statement for the predictive ability of the models ()Citation36. The models also have been used to predict the activity of the test compounds and are given in . The cross-validated correlation coefficient of the test set compounds is >0.5 shows the selected model (model 2) is significant for activity prediction. PRESS and SDEP values for the test compounds also <1 shows the model is significant. The high pred_r2 and low pred_r2se were show high predictive ability of the model. The low standard error of pred_r2se, q2_se and r2_se shows absolute quality of fitness of the model. The scatter plot between the observed and the predicted activity of the test set compounds are represented in .
Table 2. Summary of the validation parameters.
Table 3. Observed and predicted activity of the models obtained from training set and complete data set.
Table 4. Observed and predicted activity of the test set.
Figure 1. Graphs represent the plot between observed and predicted activity of the selected models. (A) Observed vs predicted activity of model 1 (R2 = 0.8202). (B) Observed vs predicted activity of model 2 (R2 = 0.8344).
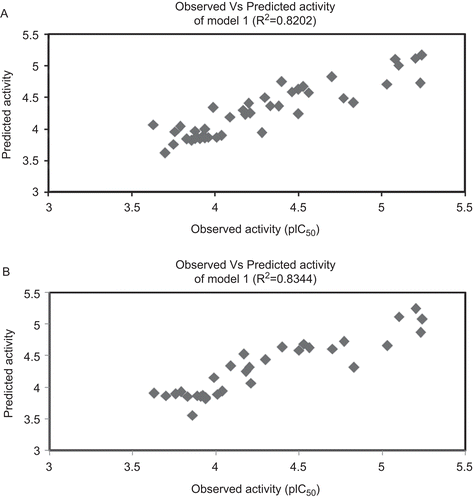
Figure 2. Observed vs predicted activity of test compounds. Graphs represents the plot between observed and predicted activity of test compounds.
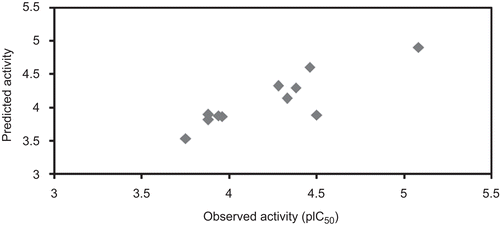
The stability of the models depends upon the multicollinearity and serial correlation of the descriptors. To confirm the absence of multicollinearity, the variable inflation factor (VIF) was calculated for each parameter in the regression. VIF denotes the fact that the variance of the standardized regression coefficients can be computed as the product of the residual variance (for the correlation transformed model) and it can be calculated as Equation 2Citation14,Citation15.
where R2 is the multiple correlation coefficient of one parameter’s effect regressed on the remaining parameters. VIF values less than 10 are statistically significant. In this present analysis we have chosen very stringent criteria in terms of VIF value (<4) while performing the QSAR analysis. The descriptors used in the selected significant models have VIF values less than 1.1691, which show that the models are free from multicollinearity ()Citation14.
Table 5. Redundancy and Durbin–Watson values of the descriptors used in the significant models.
Durbin–Watson (DW) test was employed to check the serial correlation of residuals (correlation of adjacent residuals). The DW statistics is useful for evaluating the presence or absence of a serial correlation of residuals (i.e., whether or not residual for adjacent cases are correlated, indicating that the observations or cases in the data file are not independent)Citation37,Citation38. A DW value of the model 1 is 1.1103, and the model 2 is 1.6876, which is significant at 1 and 5% respectively with the tabulated values. This shows that there is probably no autocorrelation in the residuals ().
Distance based approaches are also a way for validation of the models. They represent the distance from each point to a particular point. Mahalanobis distances methods (D2) identify the interpolation region by assuming that the data have a normal distribution.
where ∑−1 is the inverse of the covariance matrixCitation25,Citation39,Citation40.
Mahalanobis distances improve the prediction accuracy and speed up a solution for QSAR. The higher the Mahalanobis distances for a case (molecule), the more the independent variable diverges from the average value. The Mahalanobis distances of most of the cases in the selected models are less than or equal to the case value. This shows that the models have significant predictive power. Cook’s distance indicates the distance between the computed regression coefficient values and the values one would have obtained had the respective case has been excluded (leave-one-out). All distances should be of about equal magnitude otherwise there is reason to believe that the respective case (s) have biased the estimation of the regression coefficientsCitation41–43.
The average Cook’s distance value of the models is <0.77 which is <1 (squared Cook’s distances)Citation41,Citation42 and Cook’s distance of all the compounds has almost equal magnitude (<1), showing that the equation has significant predictive ability for α-glucosidase inhibitory activity.
Model 1 is a triparametric equation constructed with the complete data set. This models consist of SCC, DM, and SAHA, where SCC is an electrotopological descriptor (E-state) related to the total number of carbon atoms connected to a carbon atom which makes three aromatic bonds (SaaaC count). The magnitude of this descriptor depends on the nature of the substituents. It is not a mere count of atoms but varies with the bonding environment of each atom. The positive contribution of these electrotopological descriptors shows that the introduction of a substitution in which a carbon atom binds to aromatic carbons and has less or non-electronegative groups tends to increase the E-state value, obviously increasing the α-glucosidase inhibitory activityCitation44. DM is calculated from the partial charges (spatial separation of positive and negative charges) of the molecule. The DM descriptor is an electronic property that indicates the response of a molecule to an electrostatic field. The dipole of the molecule has been correlated to long-range ligand receptor recognition and subsequent bindingCitation45. It also promotes binding affinity of molecules to the polar active sites. The negative sign in the coefficient of the descriptors reveals that the DM of the molecules is detrimental for the binding to the α-glucosidase enzyme. SAHA (SA hydrophilic area, SlogP A) is a hydrophobicity descriptor related to the vdW surface with hydrophilic surface area (By Audry method using SlogP). The positive contribution of these descriptors suggests that the enzyme may contain some hydrophilic area for binding. The significant correlation coefficient and cross-validated correlation coefficient shows that this model has sufficient predictive power and that the descriptors have a significant contribution to the α-glucosidase inhibitory activity.
Model 2 was constructed with electrotopological (SCC), Z-CompDipole (ZCD), hydrophobicity (SAMH), and atom type count descriptors (MMFF_6). The MMFF_6 descriptor corresponds to the number of carbon atoms carrying oxygen atoms connected by single or double bonds as substituentsCitation24. It is evidenced by the compounds in the series have oxygen substituted carbon atoms leads to a greater α-glucosidase inhibitory activity. SAMostHydrophilic (SAMH) signifies most hydrophilic value on the vdW surface (By Audry method using SlogP). The negative sign of these descriptors implies that the lower electrostatic potential in the vdW surface area of the molecule and the optimum hydrophilic value on the surface of the molecule are important for favourable α-glucosidase inhibitory activity.
ZCD is the DM descriptor signifies the z component of the DM. It calculated from the partial charges (spatial separation of positive and negative charges) of the molecule in z-axis. This is an electrostatic descriptor explain the ligand receptor recognition and binding. In contrast to the DM in models 1, this descriptor positively correlated with the activity reveals that the DM in z-axis is important for the binding than in the whole compounds.
In this present QSAR study, different types of descriptors and training sets were used to build the significant models. The selected models show that the electrotopological and atom count descriptors along with electronic and hydrophobicity descriptors, resulted in models with very good statistical parameters. Topological structure descriptors and atom count descriptors are a representation of molecular structure that arise from the chemical identity of each atom, including valence state and the nature of the set of connections in the molecular skeleton, the chemical bonding pattern. The electrotopological state (E-state) index is shown to contain information reflecting intermolecular accessibility of atoms and groups in a molecule, especially electroaccessibility.
SaaaC count is atom type E-state indices that reflect the structural information for an individual atom (or atom type) but encoded from all atoms in the moleculeCitation46–48. The atomic count descriptors MMFF_6 is positively contributed for the activity, which means that heteroatoms like all oxygen atoms bound to the carbon skeleton of the molecule (MMFF_6) is favourable for the α-glucosidase inhibitory activity.
Conclusion
From the study, it is concluded that the QSAR analysis of xanthone derivatives as α-glucosidase inhibitors was performed using different types of descriptors calculated from V life software. The cross-validation correlation coefficients of the selected models are larger than 0.74, which demonstrates that all the final models are statistically significant and reliable. In the selected significant models, the topological (electrotopological) and atom count descriptors have contributed mainly to build the models. The results suggest that the presence of heteroatoms (number of oxygen atom connected with carbon atom) in the molecules is favourable for α-glucosidase inhibitory activity. The E-state count descriptor (SaaaC count) suggests that the carbon atoms connected with three aromatic bonds and hydrogen or other atoms are favourable for the α-glucosidase inhibitory activity. The hydrophobicity descriptors contributed in the models also suggest that the optimum hydrophilicity on the surface of the molecule is favourable for the inhibitory activity.
As per the earlier homology modeled structure of α-glucosidase enzymeCitation1,Citation4,Citation6,Citation11 shows that the active site has aspartic acid, histidine, and glutamic acid residues, hence it is possible the electronegative groups and other polar groups in the molecule can interact with the polar active site. These obtained results are agreement with earlier our QSAR results on this target such that the compounds with optimum partition coefficient and polar surface volume in the van der Waals surface are favourable to α-glucosidase inhibitory activity. The α-glucosidase inhibitory activity increases with increases in the distance between the hydrophobic and the hydrophilic regions of the molecules and the electronegativity of the molecules. While the molecules posses DM groups and high hydrophobic groups are detrimental for α-glucosidase inhibitory activityCitation20,Citation21. The present study on this target, in conjunction with earlier results by our laboratory with different parent molecules will be helpful for further research in the direction of the ligand based or structure based design of novel α-glucosidase inhibitors.
Acknowledgement
The authors are thankful to V Life Technologies, Pune, India for providing the softwares used in the study.
Declaration of interest
N.S.H.N.M. greatfully acknowledges the Foundation of Science and Technology (FCT), Portugal for Postdoctoral Grant (SFRH/BPD/44469/2008). The authors greatfully acknowledge FCT for providing financial support for the project PTDC/QUI/68302/2006.
References
- Silva CH, Taft CA. Computer-aided molecular design of novel glucosidase inhibitors for AIDS treatment. J Biomol Struct Dyn 2004;22:59–63.
- Fischer PB, Collin M, Karlsson GB, James W, Butters TD, Davis SJ et al. The alpha-glucosidase inhibitor N-butyldeoxynojirimycin inhibits human immunodeficiency virus entry at the level of post-CD4 binding. J Virol 1995;69:5791–5797.
- Taylor DL, Kang MS, Brennan TM, Bridges CG, Sunkara PS, Tyms AS. Inhibition of alpha-glucosidase I of the glycoprotein-processing enzymes by 6-O-butanoyl castanospermine (MDL 28,574) and its consequences in human immunodeficiency virus-infected T cells. Antimicrob Agents Chemother 1994;38:1780–1787.
- Jung-Hum P, Sungmin K, Hwangseo P. Toward the virtual screening of α-glucosidase inhibitors with the homology-modelled protein structure. Bull Korean Chem Soc 2008;29:921–927.
- Wang S, Yan J, Wang X, Yang Z, Lin F, Zhang T. Synthesis and evaluation of the alpha-glucosidase inhibitory activity of 3-[4-(phenylsulfonamido)benzoyl]-2H-1-benzopyran-2-one derivatives. Eur J Med Chem 2010;45:1250–1255.
- Hwangseo P, Kyo YH, Kyung HO, Young HK, Jae YL, Keun K. Discovery of novel α-glucosidase inhibitors based on the virtual screening with the homology-modelled protein structure. Bioorg Med Chem 2008;16:284–292.
- Humphries MJ, Matsumoto K, White SL, Olden K. Inhibition of experimental metastasis by castanospermine in mice: blockage of two distinct stages of tumor colonization by oligosaccharide processing inhibitors. Cancer Res 1986;46:5215–5222.
- Rudd PM, Storr, SJ, Royle L, Chapman CJ, Hamid UM, Robertson JF et al. The O-linked glycosylation of secretory/shed MUC1 from an advanced breast cancer patient’s serum. Glycobiol 2008;18:456–462.
- Karpas A, Fleet GW, Dwek RA, Petursson S, Namgoong SK, Ramsden NG et al. Aminosugar derivatives as potential anti-human immunodeficiency virus agents. Proc Natl Acad Sci USA 1988;85:9229–9233.
- Zitzmann N, Mehta AS, Carrouée S, Butters TD, Platt FM, McCauley J et al. Imino sugars inhibit the formation and secretion of bovine viral diarrhea virus, a pestivirus model of hepatitis C virus: implications for the development of broad spectrum anti-hepatitis virus agents. Proc Natl Acad Sci USA 1999;96:11878–11882.
- Kavitha B, Nagakumar B, Ki HP, Keun WL. Binding mode analyses and pharmacophore model development for sulphonamide chalcone derivatives, a new class of α-glucosidase inhibitors. J Mol Graph Model 2008;26:1202–1212.
- Rawling AJ, Lomas H, Adam WP, Marvin JRL, Dominic SA, Shane JSR et al. Synthesis and biological characterization of novel N-alkyl deoxynojirimycin α-glucosidase inhibitors. ChemBioChem 2009;10:1101–1105.
- Joseph R, Florent B, Catia T, Froncois M. 2D and 3D QSAR studies of diarylpyrimidine HIV-1 reverse transcriptase inhibitors. J Comput Aided Mol Des 2008;22:831–841.
- Karthikeyan C, Moorthy NSHN, Piyush T. QSAR study of substituted 2-pyridinyl quanidine as selective urokinase-type plasminogen activator (uPA) inhibitors. J Enz Inhibit Med Chem 2009;24:6–13.
- Cho DH, Lee SK, Kim BT, No KT. Quantitative structure activity relationship (QSAR) study of new fluorovinyloxyacetamides. Bull Korean Chem Soc 2001;22:388–394.
- Yan L, Zhuofeng K, Jianfang C, Wen-Hua C, Lin M, Bo W. Synthesis, inhibitory activities and QSAR study of xanthone derivatives as α-glucosidase inhibitors. Bioorg Med Chem 2008;16:7185–7192.
- Eun JS, Maecus JCL, Byong WL, Hoi YK, Young BR, Tae SJ, Woo SL, Ki HP. Xanthones from Cudrania tricuspidata displaying potent α-glucosidase inhibition. Bioorg Med Chem Lett 2007;17:6421–6424.
- Park H, Hwang KY, Kim YH, Oh KH, Lee JY, Kim K. Discovery and biological evaluation of novel alpha-glucosidase inhibitors with in vivo antidiabetic effect. Bioorg Med Chem Lett 2008;18:3711–3715.
- Raymond AD, Terry DB, Frances MP, Nicole Z. Targeting glycosylation as a therapeutic approach. Nat Rev Drug Dis 2002;1:65–75.
- Moorthy NSHN, Ramos MJ, Fernandes PA. Prediction of relationship between structural features of andrographolide derivatives and α-glucosidase inhibitory activity: a QSAR study. J Enz Inhibit Med Chem 2011;8:14–25..
- Moorthy NSHN, Ramos MJ, Fernandes PA. QSAR analysis of isosteviol derivatives as α-glucosidase inhibitors with element count and other descriptors. Lett Drug Des Dis (In Press).
- Bonchev D. Novel indices for the topological complexity of molecules. SAR/QSAR Environ Res 1997;7:23–43.
- Gálvez J, García-Domenech R, Julian-Ortiz J V de, Soler R. Topological approach to drug design. J Chem Inf Comput Sci 1995;35:272–284
- Schultz HP, Schultz EB, Schultz TP. Topological organic chemistry. 9. Graph theory and molecular topological indices of stereoisomeric organic compounds. J Chem Inf Comput Sci 1995;35:864–870.
- Biye R. Atomic level based AI topological descriptors for structure property correlations. J Chem Inf Comput Sci 2003;43:161–169.
- Jarmo H. QSAR modeling with the electrotopological state: TIBO derivatives. J Chem Inf Comput Sci 2001;41:425–429.
- Yan L, Lan Z, Lin M, Wen HC, Bo W, Zun LX. Synthesis and pharmacological activities of xanthone derivatives as α-glucosidase inhibitors. Bioorg Med Chem 2006;14:5683–5690.
- Chem BioOffice 2008, CambridgeSoft Corp, Cambridge, UK.
- V-Life Technologies, molecular design suite ™ 3.5, 2008, Pune, India.
- Statistica 8.0 statistical software, StatSoft, Inc. 2006, OK, USA
- Balaji S, Karthikeyan C, Moorthy NSHN, Trivedi P. QSAR modelling of HIV-1 reverse transcriptase inhibition by benzoxazinones using a combination of P_VSA and pharmacophore feature descriptors. Bioorg Med Chem Lett 2004;14:6089–6094.
- Gramatica P. Principle of QSAR model validation: internal and external. QSAR Comb Sci 2007;26:694–701.
- Tropsha A, Gramatica P, Gombar VK. The importance of earnest, validation is the absolute essential application and interpretation of QSPR models. QSAR Comb Sci 2003;22:69–77.
- Erikkson L, Jaworsha J, Worth AP, Mark TDC, Robert MM, Gramatica P. Methods for reliability and uncertainty assessment and for applicability evaluation of classification and regression based QSARs. Envirn Health Perspect 2003;111:1361–1375.
- Alexander G, Alexander T. Beware of Q2. J Mol Graphs Model 2002;20:269–276.
- Tuppurainen K, Viisas M, Laatikainen R, Peräkylä M. Evaluation of a novel electronic eigenvalue (EEVA) molecular descriptor for QSAR/QSPR studies: validation using a benchmark steroid data set. J Chem Inf Comput Sci 2002;42:607–613.
- Durbin J, Watson GS. Testing for serial correlation in least squares regression. II. Biometrika 1951;38:159–178.
- Prasanna S, Manivannan E, Chaturvedi SC. QSAR analysis of some fused pyrazoles as selective cyclooxygenase-2 inhibitors: a Hansch approach. Arch Pharm (Weinheim) 2004;337:440–444.
- Gnanadesikan R, Kettenring JR. Robust estimate, residuals and outlier detection with multipurpose data. Biometrics 1972;28:81–124.
- Santiago V, Humberto GD, Lourdes S, Eugenio U. QSAR model for alignment-free prediction of human breast cancer biomarkers based on electrostatic potentials of protein pseudofolding HP-lattice networks. J Comput Chem 2008;29:2613–2622.
- Cook RD. Influential observation in linear regression. J Am Stat Assoc 1979;74:169–174.
- Rotriquez G. Chapter 2, Linear models for continuous data, 1993–2000:49–58. http://data.princeton.edu/wws509/notes
- Dogra Shailay, K. Script for computing linear regression diagnostics from QSAR world-free online resources for QSAR modelling. http://www.qsarworld.com/virtualworkshop.php
- Gough JD, Hall LH. Modeling antileukemic activity of carboquinones with electrotopological state and chi indices. J Chem Inf Comput Sci 1999;39:356–361.
- Andersson PM, Michael SM, Svante W, Torbjo Rn, L. Comparison between physicochemical and calculated molecular descriptors. J Chemometrics 2000;14:629–642.
- Hliang HM, Hall LA. E-state modeling of corticosteroids binding affinity validation of model for small data set. J Chem Inf Comput Sci 2001;41:1248–1254.
- Hall LM, Hall LH, Kier LB. Modeling drug albumin binding affinity with e-state topological structure representation. J Chem Inf Comput Sci 2003;43:2120–2128.
- Maw HH, Hall LH. E-state modeling of HIV-1 protease inhibitor binding independent of 3D information. J Chem Inf Comput Sci 2002;42:290–298.