Abstract
Advantages of lipid nanoparticles for pulmonary applications are possibility of deep lung deposition with prolonged release and low toxicity. This study aimed to evaluate the effects of formulation and processing parameters on particle size of prepared SLNs. Budesonide-loaded solid lipid nanoparticles (BUD-SLNs) were prepared with different values of drug content, ultrasonication amplitude, and homogenization time and the data were modeled using artificial neural networks (ANNs). Optimal conditions for fabrication of small-sized particles of 170–200 nm were found to be low drug content with high-amplitude and high-homogenization time. In vitro aerosolization performance of BUD-SLNs was then compared to that of commercial budesonide which indicated enhancement in fine particle fraction value.
Introduction
In recent years, nanoparticles have played an important role in improving bioavailability of hydrophobic drugs. Of particular interest, solid lipid nanoparticles (SLNs) which were introduced at the beginning of the 1990s, represent an interesting alternative to other nanocarriers, such as polymeric nanoparticles and liposomes (Mansour et al. Citation2009). The solid core of SLNs consists of biodegradable lipids, different from liquid cores of liposomes and emulsions (Kukowska-Latallo et al. Citation2000). Such lipid matrices are physiologically tolerable with high biocompatibility profiles (Abbasalipurkabir et al. Citation2011). Additional advantages include improved bioavailability of poorly water soluble molecules, possibility of providing a controlled drug release system, site specific delivery of drugs (Bai et al. Citation2007), and production without the need for organic solvents (Abbasalipurkabir et al. Citation2011).
Budesonide (BUD) is a water insoluble glucocorticoid, used for management of asthma. Hydrophobic nature of the drug is an important obstacle in its clinical use that causes low solubility and absorption for the drug. To improve its solubility and absorption, solid lipid nanoparticles have been suggested (Zhang et al. Citation2011).
It has already been shown that efficacy and pharmacokinetics of nanoparticles in body mainly depends on their size, polydispersity, and surface charge (Cheow et al. Citation2010, Naikwade et al. Citation2009). Clearance of inhaled nanoparticles from the lungs depends mainly on their particle size. Small particles are cleared slower, with more translocation to interstitial sites and regional lymph nodes, compared with larger ones. Also, it is generally believed that particles smaller than 260 nm can escape from phagocytosis by macrophages (Amini et al. Citation2014, Emami et al. Citation2014). In addition, nanosized particles adhere to mucosal surfaces of the lung for a longer period of time (Amini et al. Citation2014, Chattopadhyay et al. Citation2007, Mansour et al. Citation2009). On the other hand, in pulmonary drug delivery, a certain aerodynamic diameter (∼1–5 μm) must be obtained for the preparation. It may therefore be concluded that aerosol droplet with an optimum aerodynamic diameter containing particles with nano-size could be efficiently deposit in lung tissues, and simultaneously show superior bioactivity in lung tissues (Kaur et al. Citation2012, Zhang and Zhang Citation2014).
Artificial neural networks (ANNs) are a class of learning algorithms. Employing ANNs to optimize the effective parameters in chemical processes is a useful approach for decreasing total cost with lowering the number of required experiments (Fathinia et al. Citation2010). In this process, experimental data are used for establishing relationships between independent variables and the dependent ones. Then, the model(s) are used to estimate the optimal settings of input variables (Fox et al. Citation2009). ANNs have been widely applied for modeling/optimization of nano-processes (Amani and Mohammadyani Citation2011). Determination of effects of independent (input) parameters on particle size (Sunitha et al. Citation2011) and polydispersity Index (Mezzena et al. Citation2009) of nanoparticles are examples of using ANNs. Considering the literature, some reports have worked on size of SLNs. For instance, effect of poloxamer 188 (Lv et al. Citation2009) and lecithin concentration (Lv et al. Citation2009) on size of SLNs have been reported. However, the majority of such works are one-factor-at-a-time with its limitations such as inaccurate estimate of the effects (Czitrom Citation1999).
The purpose of this research was to model the effect of some independent parameters, namely, drug concentration, amplitude, and homogenization time, on particle size of prepared SLNs. Once optimized, in vitro aerodynamic behavior of bidesonide-loaded SLNs was compared with the commercially available microsuspension of budesonide.
Materials and methods
Materials
Hydrogenated palm oil (Softisan 154, S154) was a gift from Condea (Witten, Germany). Soybean lecithin was purchased from Merck chemicals (Darmstadt, Germany). Sorbitol, (2S,3R,4R,5R)-Hexane-1,2,3,4,5,6-hexol, was purchased from Merck (Darmstadt, Germany). Poloxamer 188, used as emulsifier, was a gift from BASF AG (Ludwigshafen, Germany). Budesonide (Pharm. Eur.) was purchased from Industriale Chimica s.r.l. (Italy). Double distilled water was used for all solutions and dilution.
Preparation of budesonide loaded SLNs
Budesonide (BUD) loaded SLNs (BUD-SLNs) were prepared by ultrasonication method. The main advantage of using ultrasound waves to prepare nanoparticles is producing smaller particles with reduced polydispersity and aggregation of nanoparticles (Mehnert and Mäder Citation2001). A mixture of S154 (3.5 g), Lipoid S100 (1.5 g), and BUD (0–0.5 mg/ml) was heated to 65–70 °C while being stirred with a PTFE-coated magnet until a clear-yellowish lipid matrix (LM) solution was obtained. A solution containing 1 g poloxamer 188, 4.75 g Sorbitol, and 89.25 mL water at the same temperature was added to 5 g of LM. The pre-emulsion was homogenized with an Ultra-Turrax T25 (Janke Kunkel GmbH, Germany), at 15,000 rpm for 5–35 min. The dispersions were immediately treated using an ultrasonic probe (diameter 0.5 cm, Lab Sonic®, B. Broun, Germany). A power output with different amplitudes (55–90%) was applied for 3 min to make droplet breakage by acoustic cavitations, and subsequently formation of nanoparticles.
ANNs modeling
The relations between input parameters (i.e., BUD concentration, amplitude, and homogenization time) and output parameter (i.e., size of SLNs) were predicted using ANNs commercial software (INForm version 4.02 (Billingham, UK), Intelligensys, Billingham, UK). Then, the response surfaces were illustrated as 3D graphs and used to show the changes in size of SLNs against variations of two input parameters while the third input parameter was fixed at its low, medium, or high value, as described previously (Bartos et al. Citation2014, Gershenson Citation2003, Sun et al. Citation2003).
The obtained size data from 28 experimentally prepared samples at different input values, were applied to train, test, and validate the model using the software. Of these samples, total of 21 data were used as “training data” for network learning in order to find the relationships between input parameters and output parameter. Also, two data sets were taken as “test data” (i.e., 10% of the training data as presented by the software) for prevention of overtraining process during the training procedure of network. shows the training parameters used during ANN modeling. Further details have been given previously (Kasabov Citation1996). The training and test data applied in current study are listed in .
Table 1. The training parameters used with INForm version 4.02 (Billingham, UK)
Table 2. The training and test data set used in ANNs modeling
Subsequent to training of the network, seven remaining data were used as set of “validation data” or “unseen data” to validate the generated model (). In fact, a better predictability of the model may be determined using correlation coefficient (R2) closer to one for the validation data (Berne and Pecora Citation2000, Kätzel et al. Citation2008).
Table 3. The unseen data sets utilized in ANNs modeling
Particle size measurement
Photon correlation spectroscopy (PCS) was used to obtain the mean particle sizes. Measurement was performed immediately after preparation of the samples at 25 °C (Scatteroscope, with PCS software, version 1.32, Qudix, Korea). Before measurement, all samples were diluted with 3 ml distilled water to 1 ml of sample.
SEM analysis
For evaluating the nanoparticle morphology, sample was analyzed using scanning electron microscope (XL-30, Philips, Eindhoven, The Netherlands). Samples were initially frozen at −80 °C, then, dried for 24 h in freeze dryer.
Determination of budesonide entrapment efficiency
Percentage of entrapment efficiency was measured by High-Performance Liquid Chromatography (HPLC). Chemical analysis of BUD was performed using aWaters 600 model HPLC Controller, 2487 model dual-wave length absorbance detector, 3.9 × 150 mm Waters Nova-PakC18 column, and 515 model pump equipped with a 717plusmodel Autosampler. The HPLC settings were as follows: detection wavelength 240 nm, flow rate 1.0 ml min−1, and injection volume 100 μl. A 60:40 (v/v) methanol:acetonitril solution was used as mobile phase. A calibration curve between 1 and 100 μg/ml was established. Drug content in the supernatant after centrifugation (5000 rpm for 15 min) was measured at 240 nm. The drug entrapment efficiencies were calculated from the following equation.
Stability studies
The optimum formulation was subjected to two different stability tests to evaluate its physical stability. Centrifuge test (13,000 rpm for 30 min) (Lv et al. Citation2009) and freeze–thaw cycles (three cycles of −21 °C and +25 °C with storage time at each temperature not less than 48 h) (Sunitha et al. Citation2011). Signs of phase separation, creaming, or cracking were considered as instability.
In vitro drug release study
In vitro release experiment was performed using a dialysis bag (cut-off 12,000 Da), loaded with 2 ml BUD-SLNs. Sample was then put in the glass beaker with 150 ml PBS (0.1 M, pH 7.4, 37 °C) which was stirred at 150 rpm. At scheduled time intervals of 0.5, 1, 2, 4, 8, 12, and 24 h, 1 ml of the solution was replaced with 1 ml fresh PBS and the content of budesonide in the samples was determined by HPLC. Similar approach was applied on a sample containing equal amount of budesonide to compare the result of release from BUD-SLNs with that of bulk budesonide.
In vitro aerosol characterization
To determine the percentage of fine particle fraction (FPF), in the aerosol, 2 ml of the suspension prepared from nanoparticles was placed into the jet nebulizer (Beurer-Medical Jet Nebulizer IH25, Ulm, Germany) to be nebulized at a flow rate of 60 l/min using Twin Impinger (). During nebulization, aerosol droplets containing nanoparticles deposit on two stages based on the aerodynamic size. The nebulization was ceased after 5 min. The amount of budesonide deposited on stages A and B and the remaining amount within the nebulizer were determined through washing by ethanol and analyzing the samples by HPLC. Fine particle fraction (FPF) was calculated by Eq. (Equation2(2) ). All experiments were repeated three times.
(1)
(2)
Where RD, S1, S2, and S3 represent recovered dose (total amount of drug collected from the system), amount of drug in stage A, amount of drug in stage B, and drug remained in the nebulizer.
Results
Following the modeling procedure, the predicted model showed R2 values of 0.75, 0.98, and 0.71 for the training, test, and validation data, respectively. These values show an acceptable trained model. From the generated model, the effect of input parameters on the particle size may be evaluated. To determine the relations, one may use sensitivity analysis as a classic approach (Nic et al. Citation1997). However, in this work, we used an alternative approach as reported previously (Höhne et al. Citation2003, Schick Citation2008) which employs response surfaces when one parameter is fixed at a certain value and other two parameters are investigated in terms of their effect on the output.
In this study, first, drug concentration was fixed at high, mid-range, and low values (i.e., 0.45, 0.25, and 0.05 mg/ml, respectively) and the effect of other two variables, homogenization time, and amplitude, on the SLNs size was studied. As shown in , size decreases by increasing amplitude. On the other hand, the details show that the effect of homogenization time on the size is complicated while in general increase in time makes the size smaller, at medium, or low concentrations, extreme values of homogenization time make the size larger.
Figure 2. 3D plots of SLNs size predicted by the ANNs model fixed at low, mid-range, and high values of the concentration.
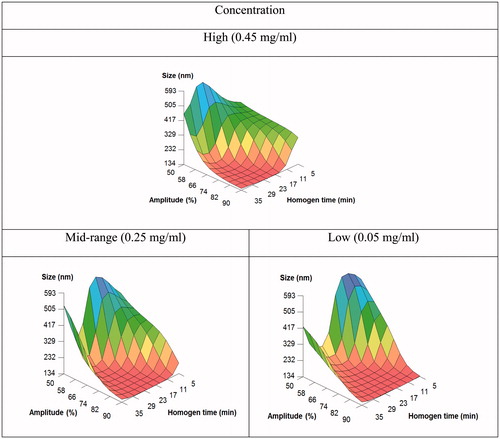
shows effect of drug concentration and homogenization time on size, when the amount of amplitude is fixed at high, mid-range, and low values (i.e., 85, 70, and 55%). Details show that when concentration is low and the size is small too. However, by increasing the concentration, above a critical value, a sharp increase in the size may be observed. This certain value is not fixed and depends on the homogenization time – when homogenization time is maximum the concentration needs to be maximized too. When the homogenization time becomes lower, the concentration value becomes lower too and minimum of both values is enough to reach the critical value.
Figure 3. 3D plots of SLNs size predicted by the ANNs model fixed at low, mid-range, and high values of the amplitude.
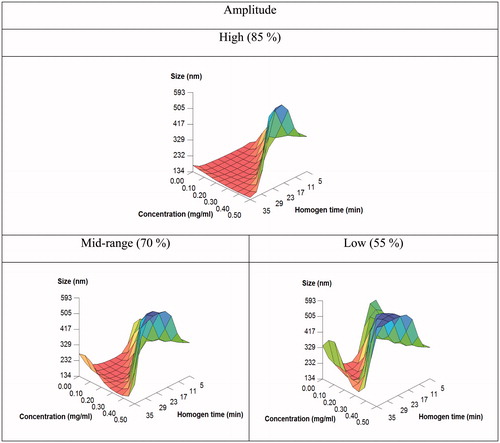
in which homogenization time is fixed at values of high, mid-range, and low (i.e., 30, 20, and 10 min, respectively), creates 3D graphs of size against amplitude and drug concentration. It is clear that by increasing the amplitude, size decreases. In fact, when homogenization time is longer, this becomes more obvious. Furthermore, increased drug concentration has an important impact on particle size too. A sharp increase in the size may be observed at a critical value of concentration.
Figure 4. 3D plots of SLNs size predicted by the ANNs model fixed at low, mid-range, and high values of the homogen time.
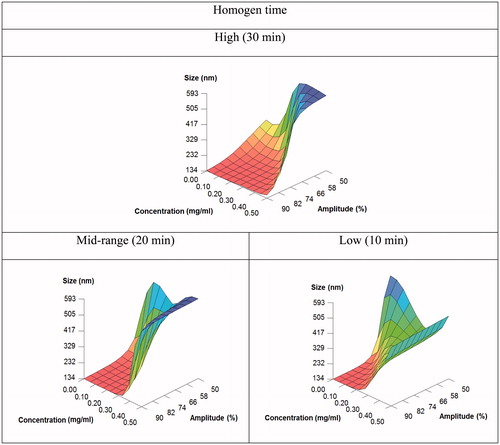
Considering findings obtained above, optimum formulation of SLNs containing budesonide with a minimum particle size may be suggested as maximum possible amplitude (90%) and possibly maximum homogenization time (35 min). The concentration of budesoinde was however fixed at 0.5 mg/ml to represent the concentration of commercially available suspension of budesonide (Pulmicort Respules®). A sample was then prepared and analyzed using SEM. The data show spherical particles with size of 176 nm (). The entrapment efficiency of budesonide in SLNs with minimum particle size was determined to be 97%.
Stability and in vitro drug release studies
The sample showed satisfactory stability profile with no sign of phase separation during the two accelerated stability tests, employed in the work. Results of release study are given in . From the details, nearly all the drug has been release from bulk budesonide during the first 24 h, while still ∼20% of the drug has remained in the nanoparticles when loaded on SLNs.
Fine particle fraction
The drug deposited on each stage of the Twin Impinger was determined and compared with that of commercial budesonide suspension. provides mean (SD) FPF data obtained for the samples.
Table 4. Mean (SD) for FPF results of BUD-SLNs and commercial budesonide suspension
Discussion
In this study, high shear mixing and ultrasonic techniques were used in production of nanoparticles. It could be argued that both approaches have contributed to lowering the size of prepared SLNs. The ultrasonic melt-emulsification method adopted in this work has a number of advantages, including simplicity, promptness, and minimum stress conditions. This method enabled production of SLNs with a low particle size ranging from 150 to 300 nm. This is in contrast with the work of other researchers claiming problems with poor polydispersity for both the ultrasonic and high shear methods (Hu and Jia Citation2010, Weber et al. Citation2014). Bhaskar et al. prepared flurbiprofen-loaded SLN by applying high shear followed by probe sonication. The prepared SLN had polydispersity values of 0.3–0.5 and a particle size in the range of 250 nm–350 nm (Patlolla et al. Citation2010). The employed high-shear generated a monodispersed dispersion with finer nanoparticles by applying a force to break the particles into smaller ones.
Results of this study show that all the parameters studied have influence on the nanoparticles size. In brief, the results show that to obtain the smallest size, amplitude, and homogenization time should be high while concentration should be low. An increase in amplitude results in increasing power of ultrasound waves. Acoustic cavitations, which are produced by ultrasound waves, exert a high shear force on the nanoparticles (Suslick and Price Citation1999). Therefore, particles are exposed to greater forces and consequently smashed to finer particles.
The effect of budesonide concentration on size may be described through the influence of lipid content on the size. It has already been reported that with increasing lipid content, the mean particle size of SLNs increases. In fact, size of lipid nanoparticles is highly dependent on lipid concentration which is explained as tendency of the lipid particles to coalesce at high lipid concentration (Emami et al. Citation2014). Sarmento et al. found that particle size tends to increase following the increment of lipid mass (Sarmento et al. Citation2007). Our finding that incorporating the budesonide into SLNs, makes the size larger, suggests that loaded budesonide is either adsorbed onto the particles surface or enters the lipid core (Abbasalipurkabir et al. Citation2011).
Also, homogenization time shows a reverse relationship with particle size. Once the dispersion is produced, agglomeration of particles is usually inescapable. Nevertheless, during the homogenization process, particles are exposed to rotation and smashing with each other, which results in destruction of agglomeration and reduction of size. When the homogenization time is at high value, this effect is more obvious than low and mid-range values.
The EE% of BUD-SLNs was quite high in our work (i.e., 97%). However, this value is expected due to hydrophobic nature of budesoinde which makes the drug molecules “escape” from aqueous preparations. The encapsulation efficiency of similar preparations has been reported as 95.7% (Emami et al. Citation2014) and 97% (Zhang et al. Citation2011) too.
In the in vitro release studies showed a constant rate during 24 h. It is thus arguable that the budesonide molecules are evenly dispersed throughout the lipid matrix, with some molecules adsorbed on the surface of SLNs (i.e., presence of a small burst effect). The burst effect was considerably lesser than that of bulk budesonide, a finding which is commonly preferred for drug delivery applications.
Furthermore, results of aerodynamic behavior studies displayed that the amount of FPF in BUD-SLNs sample 19.16%, significantly higher than the commercial microsuspension (i.e., 14.22%). This suggests a possible improvement in lung deposition of the drug. Possible explanation for the superior aerodynamic behavior is that smaller particles require less energy for being turned to aerosol and exposed to aerosol droplets. Therefore, smaller aerosol droplets are produced from the nebulizer, as we have previously shown with other nanoparticles (Amini et al. Citation2014, Amani et al. Citation2009, Citation2010).
Conclusion
In this study, we used artificial neural networks (ANNs) as powerful modeling platforms to analyze the effect of homogenization time, amplitude, and drug amount on particle size of SLNs. It is concluded that all of the three factors play a role in size determination, with reverse effect from amplitude and process time and direct impact from drug content. The significant higher FPF value for the budesonide-loaded SLNs compared to that of commercial microsuspension, suggested an improvement in the performance of the system. In addition, high stability and slow drug release from SLNs, showed its potential as an alternative to conventional preparations.
Declaration of interest
The authors express that they have no conflict of interest in this work. This work was financially supported by the grant No 92–02-87–23141 from Tehran University of Medical Sciences and Health Services, Tehran, Iran.
References
- Abbasalipurkabir R, Salehzadeh A, Abdullah R. 2011. Delivering tamoxifen within solid lipid nanoparticles. Pharm Technol. 35:74–79.
- Amani A, Mohammadyani D. 2011. Artificial neural networks: applications in nanotechnology. In: Hui C-L, Eds. Artificial Neural Networks – Application. Rijeka: INTECH.
- Amani A, Chrystyn H, Clark BJ, Abdelrahim ME, York P. 2009. Evaluation of supercritical fluid engineered budesonide powder for respiratory delivery using nebulisers. J Pharm Pharmacol. 61:1625–1630.
- Amani A, York P, Chrystyn H, Clark BJ. 2010. Evaluation of a nanoemulsion-based formulation for respiratory delivery of budesonide by nebulizers. AAPS PharmSciTech. 11:1147–1151.
- Amini MA, Faramarzi MA, Gilani K, Moazeni E, Esmaeilzadeh-Gharehdaghi E, Amani A. 2014. Production, characterisation, and in vitro nebulisation performance of budesonide-loaded PLA nanoparticles. J Microencapsul. 31:422–429.
- Bai S, Thomas C, Ahsan F. 2007. Dendrimers as a carrier for pulmonary delivery of enoxaparin, a low-molecular weight heparin. J Pharm Sci. 96:2090–2106.
- Bartos, C, Szabo-Revesz P, Ambrus R. 2014. Optimization of technological parameters by acoustic cavitation to achieve particle size reduction. Optimization. 62:1–34.
- Berne BJ, Pecora R. 2000. Dynamic Light Scattering: With Applications to Chemistry, Biology, and Physics. New York: Courier Dover Publications.
- Chattopadhyay P, Shekunov BY, Yim D, Cipolla D, Boyd B, Farr S. 2007. Production of solid lipid nanoparticle suspensions using supercritical fluid extraction of emulsions (SFEE) for pulmonary delivery using the AERx system. Adv Drug Deliv Rev. 59:444–453.
- Cheow WS, Li S, Hadinoto K. 2010. Spray drying formulation of hollow spherical aggregates of silica nanoparticles by experimental design. Chem Eng Res Design. 88:673–685.
- Czitrom V. 1999. One-factor-at-a-time versus designed experiments. Am Stat. 53:126–131.
- Emami HM, Hamishehkar H, Varshosaz JJ. 2014. Formulation and optimization of solid lipid nanoparticle formulation for pulmonary delivery of budesonide using Taguchi and Box-Behnken design. Res Pharm Sci. 10:17–33.
- Fathinia M, Khataee AR, Zarei M, Aber S. 2010. Comparative photocatalytic degradation of two dyes on immobilized TiO2 nanoparticles: Effect of dye molecular structure and response surface approach. J Mol Catal A Chem. 333:73–84.
- Fox RJ, Elgart D, Davis SC. 2009. Bayesian credible intervals for response surface optima. J Stat Plan Inference. 139:2498–2501.
- Gershenson C. 2003. Artificial neural networks for beginners. UK: arXiv preprint cs/0308031.
- Höhne GW, Hemminger WF, Flammersheim HJ. 2003. Differential scanning calorimetry. Berlin: Springer.
- Hu L, Jia Y. 2010. Preparation and characterization of solid lipid nanoparticles loaded with epirubicin for pulmonary delivery. Pharmazie Int J Pharm Sci. 65:585–587.
- Kasabov NK. 1996. Foundations of Neural Networks, Fuzzy Systems, and Knowledge Engineering. Rio de Janeiro: Marcelo Alencar.
- Kätzel U, Vorbau M, Stintz M, Gottschalk-Gaudig T, Barthel H. 2008. Dynamic light scattering for the characterization of polydisperse fractal systems: II. Relation between structure and DLS results. Part Part Syst Charact. 25:19–30.
- Kaur G, Narang RK, Rath G, Goyal AK. 2012. Advances in pulmonary delivery of nanoparticles. Artif Cells Blood Substit Immobil Biotechnol. 40:75–96.
- Kukowska-Latallo JF, Raczka E, Quintana A, Chen C, Rymaszewski M, Baker JR Jr. 2000. Intravascular and endobronchial DNA delivery to murine lung tissue using a novel, nonviral vector. Hum Gene Ther. 11:1385–1395.
- Lv Q, Yu A, Xi Y, Li H, Song Z, Cui J, Cao F, Zhai G. 2009. Development and evaluation of penciclovir-loaded solid lipid nanoparticles for topical delivery. Int J Pharm. 372:191–198.
- Mansour HM, Rhee YS, Wu X. 2009. Nanomedicine in pulmonary delivery. Int J Nanomedicine. 4:299–319.
- Mehnert W, Mäder K. 2001. Solid lipid nanoparticles: production, characterization and applications. Adv Drug Deliv Rev. 47:165–196.
- Mezzena M, Scalia S, Young PM, Traini D. 2009. Solid lipid budesonide microparticles for controlled release inhalation therapy. AAPS J. 11:771–778.
- Naikwade SR, Bajaj AN, Gurav P, Gatne MM, Singh Soni P.2009. Development of budesonide microparticles using spray-drying technology for pulmonary administration: design, characterization, in vitro evaluation, and in vivo efficacy study. AAPS PharmSciTech. 10:993–1012.
- Nic M, Jirat J, Kosata B, Jenkins A. 1997. Definition of electro kinetic potential in IUPAC. In: McNaught AD, Wilkinson A, Eds. Compendium of Chemical Terminology (the “Gold Book”). Oxford: Blackwell Scientific Publications.
- Patlolla RR, Chougule M, Patel AR, Jackson T, Tata PN, Singh M. 2010. Formulation, characterization and pulmonary deposition of nebulized celecoxib encapsulated nanostructured lipid carriers. J Control Release. 144:233–241.
- Sarmento B, Ribeiro A, Veiga F, Ferreira D, Neufeld R. 2007. Oral bioavailability of insulin contained in polysaccharide nanoparticles. Biomacromolecules. 8:3054–3060.
- Schick C. 2008. Differential Scanning Calorimeter (DSC) with Temperature Controlled Furnace. US Patent 7,448,796.
- Sun Y, Peng Y, Chen Y, Shukla AJ. 2003. Application of artificial neural networks in the design of controlled release drug delivery systems. Adv Drug Deliv Rev. 55:1201–1215.
- Sunitha R, Sireesha DS, Aparna M. 2011. Novel self-emulsifying drug delivery system-an approach to enhance bioavailability of poorly water soluble drugs. Int J Res Pharm Chem. 1:828–838.
- Suslick KS, Price GJ. 1999. Applications of ultrasound to materials chemistry. Annu Rev Mater Sci. 29:295–326.
- Weber S, Zimmer A, Pardeike J. 2014. Solid lipid nanoparticles (SLN) and nanostructured lipid carriers (NLC) for pulmonary application: a review of the state of the art. Eur J Pharm Biopharm. 86:7–22.
- Zhang P, Tu Y, Wang S, Wang Y, Xie Y, Li M, Jin Y. 2011. Preparation and characterization of budesonide-loaded solid lipid nanoparticles for pulmonary delivery. J Chin Pharm Sci. 20:390–396.
- Zhang Y, Zhang J. 2014. Preparation of budesonide nanosuspensions for pulmonary delivery: characterization, in vitro release and in vivo lung distribution studies. Artif CellsNanomed Biotechnol. [Epub ahead of print]. DOI: 10.3109/21691401.2014.944645.