Abstract
The large diversity and volume of extracellular RNA (exRNA) data that will form the basis of the exRNA Atlas generated by the Extracellular RNA Communication Consortium pose a substantial data integration challenge. We here present the strategy that is being implemented by the exRNA Data Management and Resource Repository, which employs metadata, biomedical ontologies and Linked Data technologies, such as Resource Description Framework to integrate a diverse set of exRNA profiles into an exRNA Atlas and enable integrative exRNA analysis. We focus on the following three specific data integration tasks: (a) selection of samples from a virtual biorepository for exRNA profiling and for inclusion in the exRNA Atlas; (b) retrieval of a data slice from the exRNA Atlas for integrative analysis and (c) interpretation of exRNA analysis results in the context of pathways and networks. As exRNA profiling gains wide adoption in the research community, we anticipate that the strategies discussed here will increasingly be required to enable data reuse and to facilitate integrative analysis of exRNA data.
This paper is part of the Special Issue: Extracellular RNA Communication Consortium. More papers from this issue can be found at http://www.journalofextracellularvesicles.net
To catalyze the understanding of extracellular RNA (exRNA) in human health and disease, the Extracellular RNA Communication Consortium (ERC Consortium) aims to generate a large volume of highly diverse exRNA expression profiles, assimilate them into a publicly accessible exRNA Atlas and enable their integrative analysis using online accessible exRNA analysis tools. The exRNA Atlas profiles will originate from biofluid samples provided by multiple Consortium participants, will be generated using diverse experimental methods and will be made publicly accessible according to a public data release policy developed by the ERC Consortium and made accessible at www.exrna.org. The exRNA Atlas profiles will be analysed in the context of source genomes (human and non-human), subtypes of RNA species within these genomes, and specific biological pathways and networks within cell types of origin and target cells.
The informatics infrastructure developed for the exRNA Atlas will be implemented as free open-source code and will also be made available for use by the broad scientific community as a web-hosted service to enable integrative analysis data beyond that produced by the ERC Consortium members. The initial exRNA Atlas profiles will be generated by the ERC Consortium and in the future may be expanded to include data from literature, although ERC Consortium currently does not focus on systematic compilation of data from literature.
We describe strategies that will be employed by the Data Management and Resource Repository (DMRR), a component of the Consortium, to process and analyse exRNA profiles generated by Consortium members and to support integrative analysis of exRNA profiling data through the exRNA Atlas. Towards this goal, the DMRR has organized Consortium Working Groups, including the Metadata and Data Analysis Standards and Ontology Working Groups.
During the past year, the Metadata Working Group has been actively developing the data and metadata standards for submission of exRNA profiling data for inclusion in the exRNA Atlas. A process has now been established to submit sequence data to the DMRR along with metadata in standard formats. The standards cover metadata about donors, biosamples, experiments, studies and analysis steps. The metadata enable efficient selection of samples of interest (e.g. specific health condition of the donor, biofluid or cell/tissue type, library preparation method and sequencing assay) for integrative analyses. The metadata will help organize the data in the exRNA Atlas for efficient interactive access via the exRNA Portal as well as for programmatic access via REST Application Programming Interfaces (APIs) and Linked Data technologies.
Biological ontologies provide controlled vocabulary for metadata fields, thus promoting integration both within the exRNA Atlas and with important non-ERC Consortium data sets, such as ENCODE. Our metadata standard now includes biomedical ontologies available via resources, including the BioPortal (Citation1) developed by the National Center for Biomedical Ontology (NCBO), Open Biological and Biomedical Ontology (OBO) Foundry (Citation2), Ontobee (Citation3) and Ontology Lookup Service (Citation4).
In addition, ontological relationships between concepts pave the way for knowledge-based data discovery, integration and analysis. Specifically, transitive relations such as “is-a” and “part-of” can be traversed in order to group samples and experiments into more broad categories for the purpose of retrieval and integrative analyses. Also, non-hierarchical relationships (e.g. inhibit, interact and regulate) can be used to implement expressive semantic data queries.
Both metadata and ontologies fall within the broad category of approaches to data integration that also includes Linked Data technologies such as RDF (Resource Description Framework; www.w3.org/RDF/). The Consortium aims to develop an RDF knowledge base about pathways and network modules of relevance for exRNA biology that will inform interpretation of exRNA profiling data. In the following, we review a strategy to employ metadata, ontology-based reasoning and RDF to integrate and analyse exRNA profiling data, focusing on the three tasks highlighted in a.
Fig. 1. Data slicing and pathway enrichment analysis. This illustration is based on a hypothetical example of sequencing-based exRNA profiling of cerebrospinal fluid (CSF) from a brain tumour patient. Based on metadata about the selected samples, (a) “data slice” is extracted for further downstream analysis using pathway/network modules to detect activation of a metastatic brain tumour pathway. Panel b details selection of samples for profiling and inclusion in the exRNA Atlas using sample (CSF) and disease (CNS neoplasm) ontology traversals. Panel c details sequencing assay selection process using assay and experiment ontology traversals. The highlighted ontologies “CNS neoplasm” and “sequencing assay” are examples of terms that occur within an “ontology slim.” Ontology traversal in panel d identifies RNA species of interest. (a) “Data slice” defined by selections (b–d) is analysed to obtain a set of exRNA genes that show a pattern of coordinated changes. The metastatic brain tumour pathway (www.wikipathways.org/index.php/Pathway:WP2249) in panel e shows enrichment for the exRNA genes overexpressed in this hypothetical case.
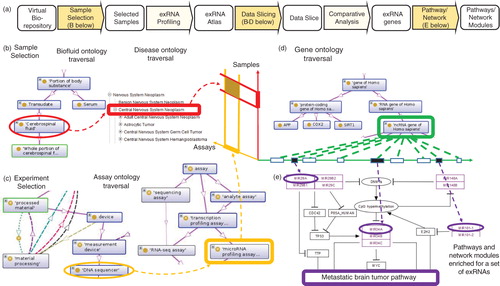
Selection of samples from a virtual biorepository
As part of an overall exRNA profiling project illustrated in a, the Resource Sharing Working Group within the Consortium is developing a virtual biorepository that will provide access to exRNA profiles derived from clinically relevant biofluid samples. Clinical information about donors, and appropriately curated information about the biosamples and their derivatives (e.g. exosomes, RNA, DNA or protein extracts), is critical for large translational science projects such as the ERC Consortium. It is important to record the information about the methods used to obtain, process and store biosamples and also to link this information to both clinical and laboratory information. Ideally, a virtual biorepository will allow secure, de-identified storage of key biological sample data in a way that can be easily linked to assay results. The biorepository will grant different levels of data entry, editing and superuser/supervisor privileges and have the ability to interface with data entry applications running on iOS, Android and Windows Phone platforms. Sample curation will allow recording of sample location, temperature histories and use/depletion.
The first use case focuses on selecting biorepository samples for the purpose of exRNA profiling. b describes a use case based on exRNA profiling done on cerebrospinal fluid (CSF) and serum samples from patients with brain tumours (Citation5). The virtual biorepository would potentially store information on donors, biospecimens, sample collection procedures, disease at the time of sample collection, available quantity of biospecimen, sample request and other relevant sample metadata, thereby facilitating the sample selection process.
To link our metadata model to ontologies, we started with the ontologies adopted by the ENCODE Data Coordination Center or DCC (Citation6, Citation7) as both the ERC Consortium and ENCODE include RNA-seq data. These ontologies include Uber Anatomy Ontology (UBERON) (Citation8) for tissues and Foundation Model of Anatomy (FMA) (Citation9) for biofluids (including controlled values for exRNA sources such as serum, plasma, CSF, urine, saliva and other body fluids), Cell Ontology (CL) (Citation10) for primary cell types and Experimental Factor Ontology (EFO) (Citation11) for immortalized cell lines. While this initial set of ontologies serves as a good starting point, additional ontologies will need to be included since the ERC Consortium is more clinically focused than ENCODE. Because many exRNA experiments will include samples from subjects affected by disease (e.g. cancer, Alzheimer's disease, etc.), additional ontologies such as the Disease Ontology (DOID) (Citation12) will be required to capture the disease terms of interest.
Ideally, the metadata describe samples and disease conditions in highly specific terms that are most informative but not suitable for retrieval. A set of general terms that covers all samples – referred to as an “ontology slim” – is generally useful to group samples at the top level. For example, the general term “central nervous system neoplasm” may provide a useful grouping of samples, including those that are annotated by highly specific terms such as “glioblastoma” or “metastatic CNS neoplasm.” These general terms are inferred by traversing ontologies from more specific terms up the hierarchy until the “slim” terms are encountered, as illustrated in b. We note that the general terms are useful for grouping and retrieval, while the most specific terms are still available for drilling-down and sub-selection.
Integrative analysis of exRNA profiling data using the exRNA toolset in the Genboree Workbench and selection of “data slices” from the exRNA Atlas
As illustrated in a, the selected samples are profiled for their exRNA content. The profiling includes both experimental assays and computational steps. The initial focus of the ERC Consortium is on RNA sequencing and qPCR, the two most commonly used assays for profiling exRNAs. Currently, both the small and long RNA-seq pipelines accept data in FASTQ format. Data submission is accompanied by relevant metadata in JSON (JavaScript Object Notation; www.json.org/) or predefined tabbed value formats. Experimental metadata fields utilize the Ontology for Biomedical Investigations (OBI) (Citation13) for experimental assays and Chemical Entities of Biological Interest (ChEBI) (Citation14) for chemical treatments. The metadata are validated against ontologies dynamically using the BioPortal (Citation15) web service. An exRNA Metadata Tracking System provides a user interface for browsing, managing, querying, viewing, uploading and downloading exRNA metadata documents.
The exceRpt small RNA-seq pipeline, accessible via the exRNA toolset in the Genboree Workbench (www.genboree.org/), profiles sequencing data using various small RNA databases including miRNAs from miRBase (Citation16), tRNAs from gtRNAdb (Citation17), piRNAs from RNAdb (Citation18) and annotations from Gencode (Citation19). Abundance estimates for each of the genes within these RNA species are computed, as are a variety of quality control metrics such as read-length distributions, summaries of reads mapped to each library and detailed mapping information for each read mapped to each library.
The RNA species and genes are annotated using Sequence Ontology (SO) (Citation20), thus facilitating retrieval of abundance data for genes within different RNA species. Genomic coordinates of RNA genes within each species will define a “data slice.” Such a “data slice” uniquely identifies data based on specific sample and disease ontologies, sequencing assay and experiment ontologies and the RNA species of interest. Abundancy estimates will be pre-computed in the exRNA Atlas, thus making it possible to deliver the “data slices” very fast. While the standardized processing will make the profiles maximally comparable, incompatibilities will naturally exist between different technologies such as qPCR and RNA-seq. This issue will be addressed in part by providing experimental metadata that is sufficient to identify comparable profiles and selection tools in the exRNA Atlas for integrative analysis. While the immediate focus will be on integrating exRNA profiles from human biofluids, the longer term goal includes enabling cross-species analyses.
The data within the exRNA Atlas may be conceptually organized along the following three dimensions illustrated in d: (a) donors and biosamples; (b) assays and experiments and (c) genomic coordinates of RNA genes. A query of the exRNA Atlas should provide a “data slice” in this three-dimensional space that is relevant for downstream analysis. As discussed above, each of the three dimensions is covered by ontologies. Similar to ontology “slims” for the biosample dimension (discussed in the previous section), appropriate “slims” will be defined for experimental and genomic dimensions. Ontology traversal will infer general terms along all the three dimensions (illustrated in b–d), thus facilitating retrieval of “data slices” for downstream analysis.
Contextual interpretation of the results of integrative analyses
As illustrated in a, “data slices” are used for downstream integrative analysis. Such analyses produce sets of exRNA genes with relevant profiles. For example, comparative analysis may identify exRNA species that are highly abundant in plasma samples from patients that have a particular type of disease. As another example, an unsupervised clustering may identify a group of exRNA species that show highly correlated abundance levels across a variety of samples. In both examples, the result of analysis is a set of genes, possibly ranked by a significance score.
After identifying a list of candidate exRNAs, the next step often involves relating these candidates to existing knowledge of mechanism and function. The ERC Consortium is pursuing two avenues towards this goal. First, knowledge of exRNA functions is often scattered in unstructured or semi-structured form across many online databases. These databases describe key information like expression patterns, vesicle and body fluid localization, and literature references. We will aggregate and unify these resources within BioGPS under an interface specifically targeted towards exRNAs.
Second, we will integrate external knowledge in the form of pathways and networks into the analysis (illustrated in e). Such knowledge is naturally represented in a machine-readable form using the network-based RDF formalism, as evidenced by databases such as WikiPathways (Citation21) and BioPAX (Citation22) that are now available in RDF form. To facilitate contextual interpretation of exRNA gene sets, the ERC Consortium, therefore, aims to develop a knowledge base of pathways and network modules that include exRNA genes. We have already begun to manually curate pathway information based on Consortium publications into the exRNA collection at WikiPathways (www.wikipathways.org/index.php/Portal:ExRNA). Furthermore, programmatic access to “data slices” will allow third-party tools to query and import exRNA gene sets resulting from comparative analysis and clustering. Towards this end, we plan to develop a Cytoscape app (Citation23) to perform exRNA data overlays onto relevant networks and pathways. Within Cytoscape, researchers will also be able to generate novel protein networks based on exRNA gene sets by leveraging existing RNA–protein and protein–protein interaction database information. These network and pathway views of exRNA “data slices” will facilitate interpretation and hypothesis generation by providing independent biological context.
Discussion and Conclusions
We presented a strategy that employs metadata and biomedical ontologies to integrate a diverse set of exRNA profiles into an exRNA Atlas and enable integrative analysis of exRNA profiles. We have also discussed exRNA analysis in the context of pathways and networks using RDF technology to represent pathways and network modules that include exRNA genes. As exRNA profiling gains wide adoption in the research community and the knowledge base of exRNA pathways increases, we anticipate that the strategies discussed here will increasingly be required to facilitate data access and integrative analyses of exRNA profiling data.
It is important to emphasize that ontologies are by definition “works in progress” as biological knowledge is extended. They continually evolve by acquiring terms, concepts and relationships to meet the needs of the scientific community. For example, at the outset of our project we could not find a widely used ontology that would provide sufficient coverage of the different types of exRNA-containing entities that will be profiled by the ERC Consortium. To address this gap, we have established a joint project with the Gene Ontology (GO) (Citation24) Consortium to add new exRNA-related terms to GO. We have also been engaging with several other scientific consortia and societies, including the External RNA Controls Consortium, International Society for Extracellular Vesicles and the American Society for Exosomes and Microvesicles, to ensure a broad consensus around the proposed ontology concepts.
Another consideration is frequent overlap between ontologies. If the same concept or term occurs in multiple ontologies, rules need to be defined that would decide which ontology to use. We have provisionally adopted the following two rules: (a) prioritize published ontologies that have been the most highly cited (e.g. GO has been published and widely cited in the bioinformatics field); (b) prioritize ontologies that cover more terms required for a particular metadata field. More formal metrics may need to be developed to assess the quality and coverage of ontologies in the exRNA domain. Also, new tools may be needed to compare different ontologies and provide intuitive visualization of the comparison results to aid in the assessment.
While ontologies have been developed independently in different biomedical domains, upper ontologies can be built to connect or integrate domain-specific ontologies at a higher level. For example, we can create an upper ontology defining a general “biological entity” class (parent) that may have subclasses (children) such as “anatomical entity” (drawn from FMA), “cell” (drawn from CL) and “cellular component” (drawn from GO) so that interconnections can be made across anatomical, cellular and cell type levels.
Finally, we note that the data to be integrated need not reside in the same physical location. One reason for this may be the need for research groups and organizations to maintain physical custody over personal health information and identifiable information about human subjects. Another reason is the increasing trend towards local data processing and data decentralization due to the increasing bandwidth of sequencing machines. To address this issue, we anticipate employing Linked Data technologies including REST APIs, JSON-LD, RDF and the emerging Linked Data Platform 1.0 (LDP 1.0) standard. For example, the LDP 1.0 features support for integration of physically distributed donor information across a virtual biorepository, thus enabling controlled sharing of personal identifiable information. Such sharing will be essential for projects with strong translational components such as the ERC Consortium.
Conflict of interest and funding
The authors declared no conflict of interest. This research was supported by the NIH Common Fund, through the Office of Strategic Coordination and the Office of the NIH Director (U54DA036134).
Acknowledgements
The biofluid, assay, experiment and RNA species ontology traversals in were performed using the Protégé resource, which is supported by grant GM10331601 from the National Institute of General Medical Sciences of the United States National Institutes of Health. The disease ontology traversal in was performed using the NCI Thesaurus. The pathway shown in was retrieved from WikiPathways (Citation21).
Notes
This paper is part of the Special Issue: Extracellular RNA Communication Consortium. More papers from this issue can be found at http://www.journalofextracellularvesicles.net
References
- Noy NF, Shah NH, Whetzel PL, Dai B, Dorf M, Griffith N et al. BioPortal: ontologies and integrated data resources at the click of a mouse. Nucleic Acids Res. 2009; 37: W170–3.
- Smith B, Ashburner M, Rosse C, Bard J, Bug W, Ceusters W et al. The OBO Foundry: coordinated evolution of ontologies to support biomedical data integration. Nat Biotechnol. 2007; 25: 1251–5.
- Xiang Z, Mungall C, Ruttenberg A, He Y. Ontobee: a linked data server and browser for ontology terms. 2011; 279–81. Proceedings of the 2nd International Conference on Biomedical Ontologies (ICBO), Buffalo, NY.
- Côté RG, Jones P, Apweiler R, Hermjakob H. The Ontology Lookup Service, a lightweight cross-platform tool for controlled vocabulary queries. BMC Bioinformatics. 2006; 7: 97.
- Redzic JS, Balaj L, van der Vos KE, Breakefield XO. Extracellular RNA mediates and marks cancer progression. Semin Cancer Biol. 2014; 28: 14–23.
- ENCODE Project Consortium. A user's guide to the encyclopedia of DNA elements (ENCODE). PLoS Biol. 2011; 9: e1001046. [PubMed CentralFull Text].
- ENCODE Project. Available from: https://www.encodeproject.org/help/getting-started/.
- Mungall CJ, Torniai C, Gkoutos GV, Lewis SE, Haendel MA. Uberon, an integrative multi-species anatomy ontology. Genome Biol. 2012; 13: R5.
- Rosse C, Mejino JL. A reference ontology for biomedical informatics: the foundational model of anatomy. J Biomed Inform. 2003; 36: 478–500. [PubMed Abstract].
- Masci AM, Arighi CN, Diehl AD, Lieberman AE, Mungall C, Scheuermann RH et al. An improved ontological representation of dendritic cells as a paradigm for all cell types. BMC Bioinformatics. 2009; 10: 70.
- Malone J, Holloway E, Adamusiak T, Kapushesky M, Zheng J, Kolesnikov N et al. Modeling sample variables with an experimental factor ontology. Bioinformatics. 2010; 26: 1112–18.
- Schriml LM, Arze C, Nadendla S, Chang YW, Mazaitis M, Felix V et al. Disease Ontology: a backbone for disease semantic integration. Nucleic Acids Res. 2012; 40: D940–6.
- Brinkman RR, Courtot M, Derom D, Fostel JM, He Y, Lord P et al. Modeling biomedical experimental processes with OBI. J Biomed Semantics. 2010; 1(Suppl 1): S7.
- Degtyarenko K, de Matos P, Ennis M, Hastings J, Zbinden M, McNaught A et al. ChEBI: a database and ontology for chemical entities of biological interest. Nucleic Acids Res. 2008; 36: D344–50.
- Whetzel PL, Noy NF, Shah NH, Alexander PR, Nyulas C, Tudorache T et al. BioPortal: enhanced functionality via new web services from the National Center for Biomedical Ontology to access and use ontologies in software applications. Nucleic Acids Res. 2011; 39: W541–5.
- Griffiths-Jones S, Grocock RJ, van Dongen S, Bateman A, Enright AJ. miRBase: microRNA sequences, targets and gene nomenclature. Nucleic Acids Res. 2006; 34: D140–4. [PubMed Abstract] [PubMed CentralFull Text].
- Chan PP, Lowe TM. GtRNAdb: a database of transfer RNA genes detected in genomic sequence. Nucleic Acids Res. 2009; 37: D93–7.
- Pang KC, Stephen S, Dinger ME, Engström PG, Lenhard B, Mattick JS. RNAdb 2.0 – an expanded database of mammalian non-coding RNAs. Nucleic Acids Res. 2007; 35: D178–82.
- Harrow J, Frankish A, Gonzalez JM, Tapanari E, Diekhans M, Kokocinski F et al. GENCODE: the reference human genome annotation for The ENCODE Project. Genome Res. 2012; 22: 1760–74.
- Eilbeck K, Lewis S, Mungall CJ, Yandell M, Stein L, Durbin R et al. The sequence ontology: a tool for the unification of genome annotations. Genome Biol. 2005; 6: R44.
- Kelder T, van Iersel MP, Hanspers K, Kutmon M, Conklin BR, Evelo CT et al. WikiPathways: building research communities on biological pathways. Nucleic Acids Res. 2012; 40: D1301–7.
- Demir E, Cary MP, Paley S, Fukuda K, Lemer C, Vastrik I et al. The BioPAX community standard for pathway data sharing. Nat Biotechnol. 2010; 28: 935–42.
- Lotia S, Montojo J, Dong Y, Bader GD, Pico AR. Cytoscape app store. Bioinformatics. 2013; 29: 1350–1.
- Ashburner M, Ball CA, Blake JA, Botstein D, Butler H, Cherry JM et al. Gene ontology: tool for the unification of biology. The Gene Ontology Consortium. Nat Genet. 2000; 25: 25–9.