Abstract
Intramammary infection affects the quality and quantity of dairy goat milk. Health status (HS) and milk quality can be monitored by electrical conductivity (EC). The aim of the study was to determine the detection potential of EC when measured on-line on a daily basis and compared with readings from previous milkings. Milk yields (MYs) were investigated with the same approach. To evaluate these relative traits, a multivariate model based on fuzzy logic technology – which provided interesting results in cows – was used. Two foremilk samples from 8 healthy Saanen goats were measured daily over the course of six months. Bacteriological tests and somatic cells counts were used to define the HS. On-line EC measurements for each gland and MYs were also considered. Predicted deviations of EC and MY were calculated using a moving-average model and entered in the fuzzy logic model. The reported accuracy has a sensitivity of 81% and a specificity of 69%. Conclusions show that fuzzy logic is an interesting approach for dairy goats, since it offered better accuracy than other methods previously published. Nevertheless, specificity was lower than in dairy cows, probably due to the lack of a significant decrease of MY in diseased glands. Still, results show that the detection of the HS characteristics with EC is improved, when measured on-line, daily and compared with the readings from previous milkings.
Introduction
Intramammary infection (IMI) mainly of bacterial origin is an important factor that affects the quality and the quantity of dairy goat milk, although other factors related to lactation and management could also be of relevance (Bergonier et al., Citation2003). Intramammary infection is associated with an increase of somatic cell count (SCC) in small ruminants. Somatic cell count is a common method for the evaluation of milk quality and, indirectly, of mammary gland health status (HS) in dairy cow and ruminants (Merle et al., Citation2007; Rossi et al., Citation2009). For these reasons, Reg. CE n 1662/2006 defines a legal limit of somatic cells in raw goat milk which is equal to 1,500,000 cells/mL. The Pasteurized Milk Ordinance regulation (FDA, Citation2007), instead, allows 1,000,000 somatic cells/mL in grade A goat milk.
The consumption of milk with a high number of somatic cells may pose risks for human health, as it is more likely to contain pathogens. Furthermore, the proteolysis triggered by microflora enzymes affects cheese-making and milk coagulation (Dragoni et al., Citation2010). Therefore, the improvement of the goat milk quality can be a valid strategy to increase the quality of cheese production according to consumer’s expectations in terms of health, safety, and taste.
Although SCC and microbiological tests are valid approaches, also the support of a laboratory is required to evaluate the quality and the main hygienic characteristics of milk. For this reason, other methods of IMI early detection may be fundamental to ensure food safety, improve milk quality, and enhance the opportunity to treat successfully infected animals. In this respect, the evaluation of electrical conductivity (EC) represents an interesting approach, because inflammation modifies the concentration of anions and cations and can affect milk EC (Zaninelli and Tangorra, Citation2007). As a consequence, in dairy cows, this parameter has become one of the most studied indicators of udder HS and its use to detect mastitis has been largely adopted with successful results in terms of sensitivity and specificity (De Mol et al., Citation1999; Kamphuis et al., Citation2008, Maatje et al., Citation1992; Nielen et al., Citation1995; Tangorra et al., Citation2006). On the contrary, in dairy goats, this has not been the case, mainly because only few, and sometimes contradictory studies were published on the relationship between EC and udder HS (Argüello, Citation2011). In a study carried out with different breeds, Ying et al. (Citation2004) reported that EC increased with infection in Saanen and decreased in Alpine goats. Petzer et al. (Citation2008) identified a moderately positive correlation between EC levels and California Milk Cell Test. Tangorra et al. (Citation2010) found a significantly higher level of EC in infected glands, but only in different lactation stages (LSs) and types of EC traits. Diaz et al. (Citation2011) observed a remarkable effect with unspecific mastitis (negative bacterial culture and SCC>1,000,000 cells/mL), which they related to EC variations arising from other factors, such as farm, parity, month of lactation, and milk composition. In another study based on daily EC measurements (Díaz et al., Citation2012), a remarkable increase in EC was observed, when major pathogens were causing an infection. Romero et al. (Citation2012) reported low sensitivity and specificity of mastitis detection with EC, when different milking fractions and thresholds were considered. Furthermore, the authors highlighted that such low performance was in line with the results obtained by Díaz et al. (Citation2011) and highlighted that, in order to develop a better mastitis detection system using EC, the intrinsic variation in the animal must be considered, the use of simple thresholds have to be avoided (as in cows), and other significant factors need to be included. One of these could be MY. Ying et al. (Citation2004) reported a MY reduction by more than 40% in Saanen and Alpine goats in case of IMI. Leitner et al. (Citation2004) found a significant reduction in MY, if both glands were infected and a greater damage to the gland was caused.
The aim of the study was to determine the detection characteristics of EC when relative trends are evaluated. Electrical conductivity measurements were acquired daily on-line through specific conductimeters located in the goat clusters. The readings were then compared with those from previous milkings in order to consider the intrinsic variation of the animals. Milk yields were also taken into account and evaluated with the same approach. A multivariate model developed with fuzzy logic technology was used to evaluate these relative traits. Fuzzy logic is an easy and powerful method that translates general knowledge into a formal mathematical model suitable for computer processing (Biewer, Citation1997). Fuzzy logic was used in cows with interesting results (Cavero et al., Citation2006; de Mol and Woldt, Citation2001; Kramer et al., Citation2009), offering better results than univariate methods. Nevertheless, no examples of the application of this method to assess the HS of dairy goats are present in scientific literature.
Materials and methods
Animals and farm management
The experiment was conducted for six months at the Experimental Farm of Borgo Adorno, University of Milan, Italy. Eight healthy Saanen goats at second lactation, 10±5 days after delivery were randomly selected from a herd of 400 dairy goats. The animals were housed in a pre-fabricated building and fed twice a day with a commercial feed covering specific nutritional requirements during the entire experimental period. Goats were milked twice a day (7:00 a.m. and 5:00 p.m.). The milking parlour had a low-line design with self-locking gates with 2 platforms consisting of 16 milking units and 16 places per platform. Milking parameters were: 90 pulsations per minute, a vacuum level of 40 kPa and a pulsation ratio of 60%. Milk yields were measured during each milking using milk meters approved by the International Committee for Animal Recording (ICAR) (AfiFree; Afimilk Ltd., Kibbutz Afikim, Israel), installed in each milking unit, and automatically recorded in the farm management software application (AfiShepherd; Afimilk Ltd.).
Collection and analyses of milk samples
Individual milk samples were collected daily aseptically from each mammary gland during the morning milking for SCC and bacteriological evaluation. In particular, milk samples were collected after teat disinfection with chlorhexidine-moistened towels, after discarding the first milk streams.
A total of 2804 milk samples were collected for bacteriological analysis according to the International Dairy Federation standard method (FIL-IDF, Citation1981). Other 2804 samples were analysed for SCC using a Bentley SomacountTM 500 analyser (Bentley Instruments Inc., Chaska, MN, USA), following the FIL-IDF (Citation1995) recommendations.
According to the results of microbiological tests and SCC, samples were classified into three experimental cases. Case 1 (C1) included milk samples with SCC<1,000,000 cells/mL and absence of pathogenic microorganisms. Case 2 (C2) included milk samples with bacteriological tests positive for IMI. Case 3 (C3) included milk samples with SCC>1,000,000 cells/mL in 2 or more consecutive sampling days for non-physiological causes and without pathogenic microorganisms. The threshold of 1,000,000 somatic cells/mL was selected, considering both the legal requirements and the literature. Reg. CE n 1662/2006 sets at 1,500,000 cells/mL the legal limit for somatic cells in raw goat milk, whereas this limit was set at 1,000,000 cells/mL according to the Pasteurized Milk Ordinance regulation (FDA, Citation2007). In the literature, some recent studies on dairy goats (Diaz et al., Citation2011, Citation2012; Romero et al., Citation2012) reported that the persistence of 1,000,000 somatic cells/mL which is not due to physiological causes is indicative of non-healthy glands, even if bacteriological analysis are negative for IMI. In the present study, the threshold of SSC was selected being the lower level among those reported above. Samples classified in C1 were associated with healthy glands, while those in C2 and C3 with non-healthy mammary glands.
Data acquisition system and electrical conductivity measurements
Four experimental milking clusters (Tangorra et al., Citation2010) were used to measure EC. They were developed by modifying commercial units equipped with individual milking claws [Vanguard; Interpuls S.p.A., Albinea (RE), Italy]. At the basis of each individual milking claw, a pair of stainless electrodes was placed to measure the specific EC, as milk was flowing from the gland. An additional couple of electrodes was placed inside each short milk tube to detect the milk flow. Therefore, inconsistent data due to milk residues in each milking claw was avoided.
During every morning milking, EC (mS/cm) was measured on-line in milk from each gland. The hardware used for the EC data acquisition included: a portable PC with a data acquisition and analogue/digital conversion card (DAQCard AI-16E-4; National Instrument, Austin, TX, USA) and five conductivity reader boards [Milkline S.r.l., Gariga di Podenzano (PC), Italy]. A customised software application developed with LabVIEW 8.02 (National Instrument) was used to acquire the signals with a sampling rate of 1 Hz. Data was recorded in simple .txt files using the goat ID farm number, date and time to name each file. A calibration procedure of the entire acquisition system was carried out every 2 weeks using a solution of water and chlorine-based detergent for the milking machines.
A customised Matlab routine (The Mathworks, Natick, MA, USA) was used to identify the milk EC of a specific goat and day of milking by processing all the .txt files. The rules implemented in the routine were the following: i) milk EC readings less than 2 mS were considered outliers and excluded, ii) series with less than 20 valid readings were coded as missing, iii) the mean of the 20 highest readings was calculated and identified as the milk EC.
Electrical conductivity and milk yield traits
For every morning milking and each individual goat, estimated EC values (for each gland) and MY were calculated through the following moving-average model:
This model calculates a new estimated value (Yt’) from the mean of the last N values. According to the findings of Cavero et al. (2007), ten previous values were considered in the model (N=10). Subsequently, relative deviations of EC and MY between measured and estimated values were calculated.
Fuzzy logic and model setup
Fuzzy logic is a multivariate method that translates general knowledge into a formal mathematical modelling suitable for computer processing (Biewer, Citation1997). A fuzzy logic system includes three steps (Klir and Yuan, Citation1995; Zimmerman, Citation1996): fuzzification, fuzzy inference and defuzzification (Zimmermann, Citation1996). During fuzzification, each real input variable is transformed into a linguistic one, where values are terms rather than numbers. Each term has a membership function, with an output range from zero to one and a piecewise linear trend, often with a triangular or trapezoidal shape. For each real value of the variable, it describes its grade of membership to the term’s fuzzy set. For example, the real variable MY could be transformed into a linguistic variable MY with the terms low, moderate, and high. For each term, a specific membership function could be defined, obtaining three fuzzy sets as reported in . After this step, for each real value of MY, the corresponding grade of membership to each fuzzy set could be determined. As an example, in case of a real yield of 1.95 (kg/d), the grade of membership to the fuzzy set low would be 0.0, to the fuzzy set moderate would be 0.3 and to the fuzzy set high, 0.7. This means that the considered yield may not certainly be low, but high and also somewhat moderate. Fuzzy inference is the step in which the real knowledge of the modelled system is expressed through the formalisation of a set of rules. These rules have the IF ... THEN base structure and are defined in terms of linguistic variables identified during the fuzzification step. This kind of rule could be for example: IF (bacteriological analysis is positive AND SSC is high) THEN the gland is probably not healthy.
Defuzzification is the final step, in which the outputs obtained from the application of the rules to the linguistic variables used in the model are transformed back into real variables. This is performed through special areas included below the membership functions of the output variable, which on the basis of different calculation techniques (i.e. the centre of gravity) translate data into a single output value.
Fuzzification
Electrical conductivity and MY relative deviations were used as input variables for the fuzzification step. Also, the maximum EC value between the udder glands was used as an input variable. The membership functions of the input variables were mainly derived from the literature (Cavero et al., Citation2006; de Mol and Woldt, Citation2001; Salehi et al., Citation2000). Details on terms and shapes used are reported in .
Fuzzy inference
Also the rules for the fuzzy inference step were mainly derived from the well (Cavero et al., Citation2006; de Mol and Woldt, Citation2001; Díaz et al., Citation2011, Citation2012; Salehi et al., Citation2000; Ying et al., Citation2004) and different combinations of these rules were evaluated. The determination of the HS of the goat with membership functions equal to very high, high, middle and low probability of disease were the outcomes of the combined input variables. All the rules applied to variables like maximum EC, deviation of EC and deviation of MY are reported in .
Defuzzification
The output values of the fuzzy inference step were transformed back into a single number by calculating the centre of gravity of the area below a specific geometric shape. This contour was obtained from the superimposed membership functions of the output variable, which was dynamically modified by the output values of the fuzzy inference. The x-axis of the calculated centre of gravity was set as the defuzzified result of the fuzzy system and used in the statistical analyses.
All of these computational evaluations were carried out using the Fuzzy Logic Toolbox of Matlab (The Mathworks).
Statistical analyses
In order to validate the data acquired during the experiment, relationships between HS and SCC, EC, MY and deviations of EC and MY were studied.
In the first step, the distribution of variables was graphically evaluated using box-plots, histograms, and normal probability plots. Values of EC (mS/cm) and SCC (×1000 cells/mL) were transformed into base 10 logarithm to normalise their distributions. The Shapiro-Wilk test was used to confirm the normal distribution of all the variables under study.
In the following step, the associations between the explanatory variables and SCC, EC and MY were evaluated using a generalised linear model (GLM) (IBM SPSS Statistics, version 21). Explanatory variables and the first-order interaction term that were investigated with SCC, EC and MY were: HS (0=healthy; 1=not healthy), LS (1=0 to 60 days in milking (DIM); 2=61 to 120 DIM; 3=>120 DIM) and the LS×HS. In the GLM model, goats were considered as random terms to account for the clustering of mammary glands within animals (Barkema et al., Citation1997). Furthermore, the relationship between deviations of EC and MY, calculated as the difference between measured and estimated values, was studied using the Spearman coefficient of correlation (SPSS Statistics, version 21.0), in accordance with the HS. In the final step, the EC detection characteristics were evaluated on the basis of the accuracy reached by the fuzzy logic model. Each value of defuzzification was compared with a specific cut-off level (0.1 to 0.9 with incremental step of 0.1). When the defuzzified value exceeded that level, an alarm was reported. Otherwise, the predicted status was considered healthy. For each goat and days of milking after the first ten observations, a comparison between the alarms and the observed status was performed and classified as: true positive (TP), if an alarm was reported by the model and the corresponding milk sample was classified in C2 or C3; false negative (FN), if no alarm was reported and the corresponding milk sample was classified in C2 or C3. Furthermore, when milk samples were classified in C1, each result was considered true negative (TN), if no alarm was reported, or false positive (FP), if an alarm was reported by the fuzzy logic model. Lastly, the accuracy was evaluated on the basis of sensitivity and specificity. In this context, sensitivity represents the percentage of alarms reported correctly in all the cases of milk samples classified in C2 or C3:
sensitivity=TP/(TP+FN)*100
The specificity indicates the percentage of healthy states predicted correctly out of all the cases of milk samples classified in C1 (i.e. healthy glands):
specificity=TN/(FP+TN)*100
The sensitivity and the specificity of a test are inversely correlated in general. In order to use the test in a practical application, a cut-off level has to be selected. On the basis of this selection, the sensitivity and the specificity of the test can be determined. Then by changing this selection, another pair of sensitivity and specificity values can be calculated. The final cut-off level selected for the test will depend on the needs and/or on the gold standards, when available, of each specific application.
An average sensitivity of 80% has been reported in the literature (Hillerton, Citation2000), as the gold standard of human observation, although it can be affected by variables such as the skills of the milker and the severity of the case. Accordingly, a specific cut-off level among those evaluated was chosen in order to reach a sensitivity of at least 80%. The resulting pair of sensitivity and specificity was considered the level of accuracy reached by the fuzzy logic model developed and used to evaluate its reliability.
Results and discussion
Individual milk samples submitted to main microbiological tests were 2804 in total. The prevalence of positive samples was 56.1% (n=1574; ). The most prevalent mastitis agent was coagulase-negative Staphylococcus (CNS) (90.1% of the bacteriological positive results; ). The prevalence of glands without pathogenic microorganisms with SCC>1,000,000, was 2.6%. The overall prevalence of non-healthy samples was about 58.7% for glands and 69.5% for goats. However, no cases of clinical mastitis were observed during the experimental period.
Relationships between HS and SCC, EC and MY were also investigated. Means of SCC increased significantly (P<0.001), as lactation progressed (5.26±0.02 log SCC in 0 to 60 DIM, 5.40±0.02 log SCC in 61 to 120 DIM, and 5.51±0.03 log SCC in >120 DIM; ). Higher significant mean value (P<0.001) of SCC was observed in NH glands (5.33±0.02 vs 5.45±0.02 log SCC), but the interaction between LS and HS was not significant. Also EC means (mS/cm) increased significantly (P<0.001) during all LSs (11.14±0.07 in 0 to 60 DIM, 14.08±0.08 in 61 to 120 DIM, and 14.40±0.10 in >120 DIM; ). The mean value showed to be significantly higher in NH glands (13.10±0.08 vs 13.31±0.06), even if the significance obtained (P<0.05) was lower than for log SCC. Interaction between LS and HS was not significant as for SCC. Milk yield means presented a low increase during lactation (1.07±0.02 to 1.12±0.03 kg/milking; ), as well as a small difference between HSs (1.09±0.02 vs 1.08±0.01 kg/milking). However, explanatory variables included in the statistical model and their interaction did not show to be statistically significant.
Correlations between deviations of EC and MY were studied as well. A significant correlation between these trends was identified when all data was considered (Spearman coefficient=0.55; P<0.01). When correlations between deviations of EC and MY were evaluated according to HS, a significant value was found for non-healthy cases (Spearman coefficient=0.18; P<0.001), while no significant correlation was observed in healthy cases. However, the deviation of EC showed to have a significant correlation with HS, when deviations of MY were not considered (Spearman coefficient=0.78; P<0.001).
Lastly, the accuracy reached by the fuzzy logic model was investigated. The model showed a sensitivity and a specificity that varied for each cut-off level, as expected. In particular, specificity increased and sensitivity decreased with increasing cut-off levels. Following this trend, for each cut-off the levels of sensitivity and specificity were obtained in pairs (). In order to have a model sensitivity of at least 80%, the cut-off level of 0.7 was selected among those evaluated. Consequently, a pair of sensitivity and specificity was obtained (81 and 69%, respectively) and considered to be the accuracy level reached by the fuzzy logic model.
Experimental data evaluation
In order to evaluate the data acquired during the experiment, the prevalence of bacteriological results, the relationships between HS and SCC, EC, MY, the deviations of EC and MY in some parameters according to LS were analysed.
The prevalence of bacteriological positive samples was high (56.1%). This result was probably influenced by different factors related to environmental conditions and chronic intramammary infections. The persistence of subclinical IMI during lactation varied depending on the causative pathogen, but it was generally high, since the Staphylococcus was the most frequent. In this study, the most prevalent mastitis agents were CNS (n. 1418). Even though CNS organisms are minor pathogens in dairy cattle, they are frequently associated with subclinical mastitis in small ruminants and are the most prevalent pathogens of the mammary gland among sheep and goats. Sources of CNS include milker’s hands and the skin of the animals, including the teat surface. In addition, CNS can potentially trigger a chronic infection (McDougall et al., Citation2002). The remaining samples classified as non-healthy glands showed a low prevalence (2.6%). They were characterised by negative bacteriological culture and high levels of SCC in the milk. These results may have been due to undiagnosed organisms, such as viruses causing viral infections.
Coagulase-negative staphylococci significantly increased during lactation (5.26 to 5.51 log SCC) and were significantly higher in milk samples from non-healthy glands (5.33 vs 5.45 log SCC). The same trends were recently reported by other studies (Díaz et al., Citation2011, Citation2012; Paape et al., Citation2007; Romero et al., Citation2012; Tangorra et al., Citation2010). Romero et al. (Citation2012), in a study on 84 Murciano-Granadina goats at the fourth mouth of lactation, analysed three different milking fractions and found that the highest SCC levels were observed in infected glands associated with all the considered fractions. Furthermore, SCC in infected glands was approximately twice as high as in healthy glands (from 5.03 for the first fraction of milking to 5.31 log SCC for the third fraction of milking in healthy glands, compared with 5.47 for the first milking fraction and 5.66 log SCC for the third fraction of milking in cases of infected glands). Díaz et al. (Citation2012), in a study on 18 Murciano-Granadina goats at the third month of lactation, found a significant increase of SCC after infection of a gland, which was not observed in collateral healthy glands or in glands from goats free from infection. In another study conducted by Diaz et al. (Citation2011) over 7 months of lactation on 105 Murciano-Granadina goats from 3 different farms reported a significant increase in the average value of log SCC during lactation (from 5.83 for the first month of lactation to 6.03 for the seventh month of lactation). Also, a significant increase was observed in mastitis levels. Tangorra et al. (Citation2010) in a study on 8 Saanen goats over 225 days of lactation reported that in healthy glands log SCC increased from the beginning to the end of the lactation cycle (from 5.77 to 6.39) and that the mean level of SCC for the whole lactation period was significantly different in milk from healthy vs infected glands (6.18 vs 6.86 log SCC). Paape et al. (Citation2007), after analysing data from 16,041 goats of different breeds, concluded that a progressive increase in SCC is observed in non-infected goat glands, as lactation progresses. Intramammary infection proved to be the major cause for an increase of milk SCC, even if other non-infectious factors, such as oestrus, season and MY, may play a role.
The EC means (mS/cm) increased significantly during all lactation (from 11.14 to 14.40) and mean value showed to be significantly higher in glands classified in C2 and C3 groups (13.10 vs 13.31). Results obtained do not match with the findings of Romero et al. (Citation2012), who found a lack of significant effect of HS on EC. However, in this study a higher prevalence of bilateral infections was observed (47.9 vs 32.3%). Díaz et al. (Citation2012) found a significant increase of EC only in cases of bilateral infection. Higher prevalence of bilateral infections observed in this study could therefore explain the different significance that data showed in terms of the relationship between EC and HS. As to the EC trend in different LSs, Diaz et al. (Citation2011) found comparable results, such as an increase of EC with progression of lactation in multiparous goats. Tangorra et al. (Citation2010), instead, found the same results in relation to the effects of lactation and HS on EC. The mean values obtained for the parameter X20 (equal to the 20 highest EC values within a milking) at incremental LSs were: 11.23, 13.87 and 14.61 and 13.03 vs 13.45 for healthy and infected glands, respectively.
Milk yield means presented a low increment during LSs evaluation. These results are in contrast with the general knowledge about milk production curves during lactation. However, León et al. (Citation2012) in a recent study on lactation curves of Murciano-Granadina goats found that factors such as region, lactation number, and seasonal aspects affect both the scale and the shape of lactation curves. In particular, they found that goats in their first lactation have a lower initial production level, as well as a lower peak yield later on. Furthermore, a scaling effect was found in the second lactation. Also, the goats of this study were at their second lactation. In addition, the limited number of animals and the type of sampling of LSs (0 to 60, 61 to 120, >120 DIM) may have affected the data observed. As for the relationship with HS, results showed a limited difference of MY between healthy and NH glands (but a lack of significance was found). These results are in contrast with the findings of Ying et al. (Citation2004), which in a study on Saanen and Alpine goats reported a reduction of more than 40% of MY in cases with IMI. However, Diaz et al. (Citation2012) also found that MY did not decrease significantly after the initial development of the infection. They supposed that this was probably attributable to the subclinical infection that most animals developed in agreement with the results of Leitner et al. (Citation2004), who found a significant reduction in yield, when both glands were infected and severely damaged. Among the goats included in the experiment, the number of clinical cases of mastitis was not remarkable. The most frequent microorganism found in bacteriological tests performed was CNS. Koop et al. (Citation2010), in a study aimed to estimate MY losses associated with IMI in dairy goats, reported no significant MY losses in case of infection with CNS.
The correlation between deviations of EC and MY was significant, when all data was considered and also in the case of non-healthy glands when HS was taken into account. Diaz et al. (Citation2011) found a significant correlation between EC and MY in infected glands, when data was analysed according to the HS, but no significant correlation was obtained with healthy glands or when all data was considered. Although changes in absolute values of EC and MY may affect the trends of the corresponding relative deviations, these pairs of parameters have a different meaning. Consequently, results obtained by Diaz et al. (Citation2011) are not directly comparable and no other investigations on these parameters about dairy goats are reported in the scientific literature. However, the deviation of EC showed a significant correlation with HS, when the deviation of MY was not considered, as well as found for the absolute value of EC. These results suggest that the deviation of EC could be a useful parameter to improve dairy goat HS detection.
Fuzzy logic evaluation
Fuzzy logic is a technique that translates general knowledge into a formal mathematical model. Linguistic variables are the keys of this translation procedure. They use words rather than numbers and can describe a system in a way that is closer to human intuition. With their membership functions and rules, they are able to manage uncertain data about some parts of the system. Moreover, through specific logical and arithmetical calculations, they make it possible to process the model electronically.
The application of this technique, in comparison with other univariate or multivariate models built with mathematical or statistical approaches, offers the advantage of being easy to interpret, modify and adapt. In our experience, the translation of general knowledge into membership functions and rules applied to the selected linguistic variables was quite easy. Moreover, different membership functions, in terms of shapes and outputs, and different rules were considered in order to obtain a better set-up of the model, with no significant problems.
Fuzzification and fuzzy inference are the most important steps in the fuzzy logic technique, since they affect the accuracy of the model. Unfortunately, no standard methods are available to perform these steps in order to reach optimal results, starting from the human knowledge and experience of a specific application. Only two different strategies can be followed: a trial-and-error and a mathematical procedure that can reduce classification errors of the model. The former has the disadvantage that it is a time-consuming process and does not always allow to set-up the model in order to reach the best accuracy. The latter strategy, instead, needs to be based on the experimental data collected (at least a third). Furthermore, the procedure works to find out the best accuracy on the shapes and outputs of the membership functions of the model. In some cases, at the end of this optimisation, the shapes and the outputs of the membership functions can be so different that ease of interpretation could be partially lost in the resulting fuzzy logic model. In addition, the final set-up of the fuzzy model is directly related to the data-set used for the optimisation procedure. If this set is not significant for the application studied, then the accuracy reached by the model in a real application will not be suitable.
In this study, the number of membership functions was low. Therefore, it was not difficult to reach a good set-up of the model, even if a trial-and-error approach was followed. Nevertheless, the simplicity and the potential of this technique would be best suited to broader application range. With an increased basic knowledge of dairy goat HS, new parameters could be available to be used as input linguistic variables. In that case, however, the use of a trial-and-error approach could be unsuitable to reach a good set-up of the model because of the higher number of membership functions. Therefore, it could be necessary to rely on a mathematical procedure to optimise the model, which should be considered during the development of the experimental design in order to have enough experimental data to perform correctly both the optimisation and the evaluation of the model.
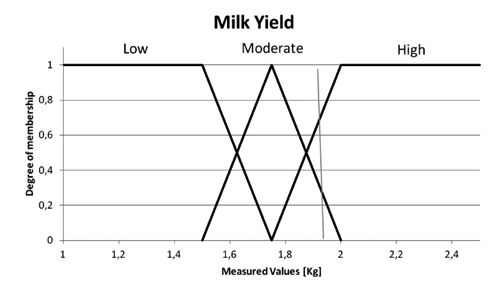
Table 1. Membership functions for the traits considered in the study.
Table 2. Rules of the fuzzy inference step about the traits considered in the study. As shown when deviation in milk yield changes from middle to high or very high, values in the table increase in order to highlight an higher probability of disease. On the contrary, values decrease when the deviation in milk yield becomes low or very low. Similar trends can be observed considering the other traits reported in the table.
Table 3. Distribution of mammary glands by health status in different samples groups.
Table 4. Distribution of pathogenic microorganisms found in infected mammary glands.
Table 5. Overall means and standard errors of somatic cell count of milk samples according to goats’ health status and lactation stages.
Table 6. Overall means and standard errors of electrical conductivity of milk samples according to goats’ health status and lactation stages.
Table 7. Overall means and standard errors of milk yield according to goats’ health status and lactation stages.
Table 8. Accuracy reached by the fuzzy logic model in terms of sensitivity and specificity at different cut-off levels.
Detection characteristics of electrical conductivity with fuzzy logic
In dairy goat research, no studies on the detection of HS characteristics with EC based on fuzzy logic models are present. However, other types of models or indexes were analysed. Romero et al. (Citation2012) studied the effect of the milking fraction on EC and found that the EC mastitis detection readings were similar in the three milking fractions analysed, and that the best performance obtainable with a defined threshold of 5.20 mS/cm had a sensitivity of 70% and a specificity of 50%. Diaz et al. (Citation2011) analysed data from 3 different farms and reported [with a threshold of 5.20 mS/cm as in Romero et al. (Citation2012)] sensitivities that ranged between 60 and 93% and specificities between 22 and 47% (depending on the farm considered).
In this study, the sensitivity and specificity reached by the fuzzy logic model were 81 and 69% respectively. Compared with the above reported results, the value of specificity found was more than what was found by Romero et al. (Citation2012) (69 vs 50%), and Diaz et al. (Citation2011), if different cut-off levels with the sensitivity set at at least 80% are selected (69 vs 40%, 5.0 mS/cm, farm 1 to 69 vs 27%, 5.1 mS/cm, farm 2 to 69 vs 35%, 5.4 mS/cm, farm 3). Therefore, the fuzzy logic model proved to be an interesting approach to detect HS in dairy goats, since the accuracy offered by the model was better than those obtained with univariate evaluations of different EC traits. Furthermore, the results showed that the use of on-line daily measurements and deviations of EC between the predicted and actual values can lead to the development of more accurate on-line health detection systems also in dairy goats, as argued by Romero et al. (Citation2012) and Diaz et al. (Citation2011).
Conversely, many methods to detect udder HS in cattle based on EC and fuzzy logic technology have already been developed and tested. Kramer et al. (Citation2009), in a research on the control of lameness and mastitis in cows based fuzzy logic mode, reported mastitis detection specificities that ranged between 88.3 and 77.4%, depending on the different definitions of mastitis (e.g. udder treatment and udder treatment or SCC over 400,000/mL). Cavero et al. (Citation2006) aimed to develop a fuzzy logic method to classify mastitis status in cows milked with an automatic milking system, reported sensitivities that ranged between 92.9 and 83.9%, and specificities between 93.9 and 75.8%, depending on the different definitions for mastitis (e.g. udder treatment performed based on SCC, with SCC>100,000 cells/mL, or with SCC>400,000 cells/mL). De Mol and Woldt (Citation2001), in a research designed to reduce the number of FP cases produced by a previously developed detection model (de Mol and Ouweltjes, Citation2000), tested a fuzzy logic method and reported a sensitivity of 100% and a specificity >99.5%. All clinical cases without measurement errors were classified correctly, and the resulting number of FP alerts from a subset of 25 cows that did not show any signs of mastitis was reduced from 1266 to 64 by applying the fuzzy logic method.
In our study, results obtained with the fuzzy logic model were generally fewer, if compared with results reported in dairy cows. This is probably due to the lack of a significant decrease of MY in non-healthy glands, also worsened by an insignificant correlation between deviations of MY and HS, which affected the accuracy of the model. These results seem to confirm that MY is not a useful parameter to detect HS in dairy goats, as found by Díaz et al. (Citation2012). However, in dairy cows the decrease of MY is generally more significant in cows with mastitis. This is particularly true when clinical mastitis occurs (Hertl et al., Citation2014; Schukken et al., Citation2009), as in some of the above studies, where good detection performance was reached. In our study, no clinical cases were reported. This could explain the lower results we obtained. If different experimental data were to be collected and evaluated with the fuzzy logic model, the results obtained could be closer to those reported in dairy studies. Furthermore, the use of more informative parameters obtained with a more extensive knowledge of dairy goat HS, an improvement of sensor technology, and the implementation of more explanatory traits could also lead to better results in detecting HS in dairy goats.
Conclusions
The present study demonstrates that fuzzy logic is an interesting approach to detect HS also when applied to dairy goats. The multivariable model proved to reach better accuracy than the models based on univariate approaches to different traits which were previously published. Nevertheless, the specificity obtained was lower than those in dairy cows. This result seems to confirm that MY is not a useful parameter to detect HS in dairy goats. However, the results obtained showed that EC detection of HS characteristics can be improved, if EC is measured on-line, evaluated daily without specific thresholds and compared with the readings from previous milkings in order to consider the intrinsic variation of the animals.
References
- ArgüelloA., 2011. Trends in goat research, a review. J. Appl. Anim. Res. 39:429-434.
- BarkemaH.W. SchukkenY.H. LamT.J. GalliganD.T. BeiboerM.L. BrandA., 1997. Estimation of interdependence among quarters of the bovine udder with subclinical mastitis and implications for analysis. J. Dairy Sci. 80:1592-1599.
- BergonierD. de CrémouxR. RuppR. LagriffoulG. BerthelotX., 2003. Mastitis of dairy small ruminants. Vet. Res. 34:689-716.
- BiewerB., 1997. Fuzzy-methoden: praxisrelevante rechenmodelle und fuzzy-programmiersprachen. Springer Verlag, Berlin, Germany.
- CaveroD. TölleK.H. BuxadéC. KrieterJ., 2006. Mastitis detection in dairy cows by application of fuzzy logic. Livest. Sci. 105:207-213.
- CaveroD. TölleK.H. RaveG. BuxadéC. KrieterJ., 2007. Analysing serial data for mastitis detection by means of local regression. Livest. Sci. 110:101-110.
- De MolR.M. KeenA. KroezeG.H. AchtenJ.M.F.H., 1999. Description of a detection model for oestrus and diseases in dairy cattle based on time series analysis combined with a Kalman filter. Comput. Electron. Agr. 22:171-185.
- De MolR.M. OuweltjesW., 2000. Detection model for estrus and mastitis in cows milked in an automatic milking system. pp 97-107 in Proc. Int. Symp. Robotic Milking, Lelystad, The Netherlands.
- De MolR.M. WoldtW.E., 2001. Application of fuzzy logic in automated cow status monitoring. J. Dairy Sci. 84:400-410.
- DíazJ.R. RomeroG. MuelasR. AlejandroM. PerisC., 2012. Effect of intramammary infection on milk electrical conductivity in murciano-granadina goats. J. Dairy Sci. 95:718-726.
- DiazJ.R. RomeroG. MuelasR. SendraE. PantojaJ.C.F. ParedesC., 2011. Analysis of the influence of variation factors on electrical conductivity of milk in Murciano-Granadina goats. J. Dairy Sci. 94:3385-3394.
- DragoniI. BalzarettiC. RossiniS. RossiL. Dell’OrtoV. BaldiA., 2010. Detection of hen lysozyme on proteic profiles of Grana Padano cheese through SELDI-TOF MS high-throughput technology during the ripening process. Food Anal.l Method. 4:233-239.
- FDA, 2007. Grade “A” pasteurized milk ordinance. Public Health Service, Food and Drug Administration ed., Washington, DC, USA. Available from: http://www.michigan.gov/documents/mda/MDA_DP_07PMOFinal_251324_7.pdf
- FIL-IDF, 1981. Laboratory methods for use in mastitis work. Fédération Internationale de Laiterie – International Dairy Federation ed., Brussels, Belgium.
- FIL-IDF, 1995. Enumeration of somatic cells. Fédération Internationale de Laiterie – International Dairy Federation ed., Brussels, Belgium.
- HertlJ.A. SchukkenY.H. WelcomeF.L. TauerL.W. GröhnY.T., 2014. Pathogen-specific effects on milk yield in repeated clinical mastitis episodes in Holstein dairy cows. J. Dairy Sci. 97:1465-1480.
- HillertonJ.E., 2000. Detecting mastitis cowsided. pp 48-53 in Proc. 39th Annu. Natl. Mastitis Meet., Atlanta, GA, USA.
- KamphuisC. PietersmaD., van der TolR. WiedemannM. HogeveenH., 2008. Using sensor data patterns from an automatic milking system to develop predictive variables for classifying clinical mastitis and abnormal milk. Comput. Electron. Agr. 62:169-181.
- KlirG.J. YuanB., 1995. Fuzzy sets and fuzzy logic: theory and applications. Prentice Hall, Upper Saddle River, NJ, USA.
- KoopG. van WervenT. SchuilingH.J. NielenM., 2010. The effect of subclinical mastitis on milk yield in dairy goats. J. Dairy Sci. 93:5809-5817.
- KramerE. CaveroD. StamerE. KrieterJ., 2009. Mastitis and lameness detection in dairy cows by application of fuzzy logic. Livest. Sci. 125:92-96.
- LeitnerG. MerinU. GlickmanA. WeisblitL. KrifucksO., 2004. Factors influencing milk quantity and quality in Assaf sheep and goat crossbreds. S. Afr. J. Anim. Sci. 34:2002-2004.
- LeónJ.M. MacciottaN.P.P. GamaL.T. BarbaC. DelgadoJ.V., 2012. Characterization of the lactation curve in Murciano-Granadina dairy goats. Small Ruminant Res. 107:76-84.
- MaatjeK. HuijsmansP.J.M., RosingW. HogewerfP.H., 1992. The efficacy of in-line measurement of quarter milk electrical conductivity, milk yield and milk temperature for the detection of clinical and subclinical mastitis. Livest. Prod. Sci. 30:239-249.
- McDougallS. PankeyW. DelaneyC. BarlowJ. MurdoughP.A. ScrutonD., 2002. Prevalence and incidence of subclinical mastitis in goats and dairy ewes in Vermont, USA. Small Ruminant Res. 46:115-121.
- MerleR. SchröderA. HamannJ., 2007. Cell function in the bovine mammary gland: a preliminary study on interdependence of healthy and infected udder quarters. J. Dairy Res. 74:174-179.
- NielenM. SchukkenY.H. BrandA. DeluykerH.A. MaatjeK., 1995. Detection of subclinical mastitis from on-line milking parlor data. J. Dairy Sci. 78:1039-1049.
- PaapeM.J. WiggansG.R. BannermanD.D. ThomasD.L. SandersA.H. ContrerasA. MoroniP. MillerR.H., 2007. Monitoring goat and sheep milk somatic cell counts. Small Ruminant Res. 68:114-125.
- PetzerI.M. DonkinE.F. Du PreezE. KarzisJ. Van der SchansT.J. WatermeyerJ.C. Van ReenenR., 2008. Value of tests for evaluating udder health in dairy goats: somatic cell counts, California milk cell test and electrical conductivity. Onderstepoort J. Vet. 75:279-287.
- RomeroG. PantojaJ.C.F., SendraE. PerisC. DíazJ.R., 2012. Analysis of the electrical conductivity in milking fractions as a mean for detecting and characterizing mastitis in goats. Small Ruminant Res. 107:157-163.
- RossiL. GalanteF. FusiE. LuiniM. Dell’OrtoV. BaldiA., 2009. Evaluation the of PL-PG-PA System in Relation to Quality of Bovine Milk. Vet. Res. Commun. 33:293-295.
- SalehiF. LacroixR. WadeK.M., 2000. Development of neuro-fuzzifiers for qualitative analyses of milk yield. Comput. Electron. Agr. 28:171-186.
- SchukkenY.H. HertlJ. BarD. BennettG.J. GonzálezR.N. RauchB.J. SantistebanC. SchulteH.F. TauerL. WelcomeF.L. GröhnY.T., 2009. Effects of repeated gram-positive and gram-negative clinical mastitis episodes on milk yield loss in Holstein dairy cows. J. Dairy Sci. 92:3091-3105.
- TangorraF.M. ZaninelliM. CostaA. AgazziA. SavoiniG., 2010. Milk electrical conductivity and mastitis status in dairy goats: results from a pilot study. Small Ruminant Res. 90:109-113.
- TangorraF.M. ZaninelliM. De SantisC., 2006. Development of HW and SW solutions for milk traceability. In: ZazuetaF. ( ed.) Computers in agriculture and natural resources. American Society of Agricultural and Biological Engineers ed., Orlando, FL, USA, pp 475-480.
- YingC.H. YangC.H.-B., HsuJ.-T., 2004. Relationship of somatic cell count, physical, chemical and enzymatic properties to the bacterial standard plate count in different breeds of dairy goats. Asian Austral. J. Anim. 17:554-559.
- ZaninelliM. TangorraF.M., 2007. Development and testing of a ‘free-flow’ conductimetric milk meter. Comput. Electron. Agr. 57:166-176.
- ZimmermannH.-J., 1996. Fuzzy set theory and its applications. Kluwer Academic Publ., Boston, MA, USA.