Abstract
Background: Landscape fires in South America have considerable impacts on ecosystems, air quality and the climate system. We examined long-term trends and interannual variability of forest, savanna and agricultural fires for the continent during 2001–2012 using multiple satellite-derived fire products. Results: The annual number of active fires in tropical forests increased significantly during 2001–2005. Several satellite-derived metrics, including fire persistence, indicated that this trend was mostly driven by deforestation. Fires between 2005 and 2012 had a small decreasing trend and large year-to-year changes that were associated with climate extremes. Fires in savannas and evergreen forests increased in parallel during drought events in 2005, 2007 and 2010, suggesting similar regional climate controls on fire behavior. Deforestation fire intensity (the number of fires per unit of deforested area) increased significantly within the Brazilian Amazon in areas with small-scale deforestation. Conclusion: Fires associated with forest degradation are becoming an increasingly important component of the fire regime and associated carbon emissions.
Vegetation types were grouped into EvgF, DecF, Savn and Agri. The EvgF region was additionally divided into a nondeforestation component (L0) and eight deforestation levels (L1–L8, corresponding to cumulative deforestation fractions from 2000 to 2005 between 0.7 and 5, 5 and 10, 10 and 15, 15 and 20, 20 and 30, 30 and 40, 40 and 50, and 50 and 100%, respectively). All other land cover types are shown in white. G1–G3 mark the locations of three representative regions used for illustration of fire persistence in . The two-letter state codes of Brazil follow ISO 3166–2.
Agri: Agriculture; DecF: Deciduous forest; EvgF: Evergreen forest; G1: Deforestation region; G2: Savanna region;
G3: Agricultural region; Savn: Savanna.
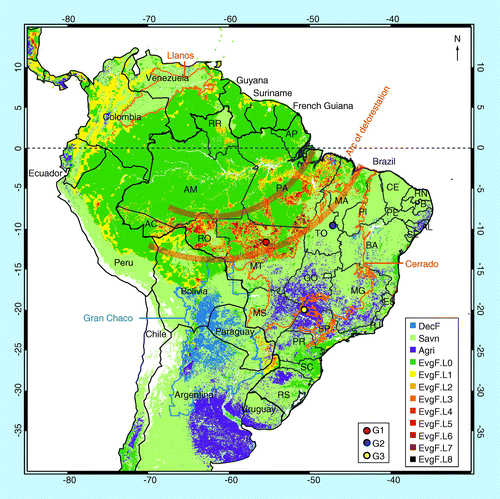
(A) Fire locations and years as measured by Terra Moderate resolution Imaging Spectroradiometer at the native resolution (approximately 1 km) of the active fire product. (B) Mean annual fire days in each 0.01° × 0.01° pixel. (C) All-year mean fire persistence: the mean annual fire days in each fire location averaged over each 0.05° × 0.05° grid cell. The locations of these three regions (G1–G3) are shown in .
G1: Deforestation region; G2: Savanna region; G3: Agricultural region.
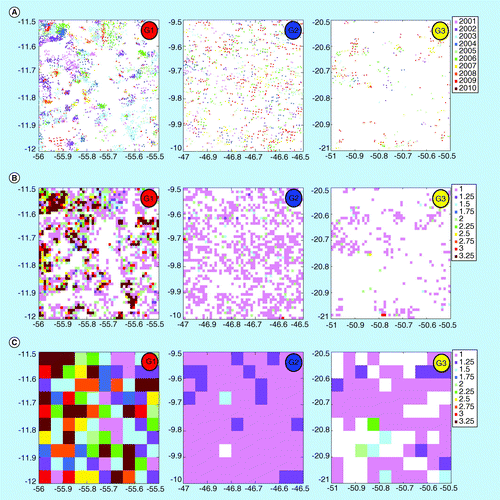
(A) Mean annual active fire density (Mha-1 yr-1) and (B) fire persistence (days yr-1). (C) Forest cover fraction in 2001 and (D) Global Fire Emissions Database version 3 annual mean fire emissions during 2001–2011 are also shown. All data are shown at a 0.05° spatial resolution with the exception of Global Fire Emissions Database version 3 emissions shown at a 0.5° resolution.
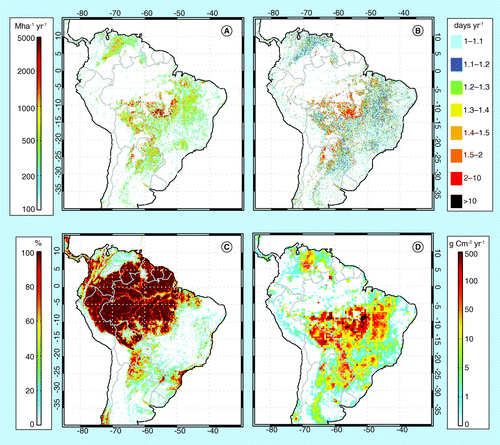
(A) Active fire density (Mha-1 yr-2) derived from Terra Moderate resolution Imaging Spectroradiometer (2001–2012); (B) burned area (2001–2011; Kha Mha-1 yr-2); and (C) fire carbon emission from Global Fire Emissions Database version 3 (2001–2011; gC m-2 yr-2).
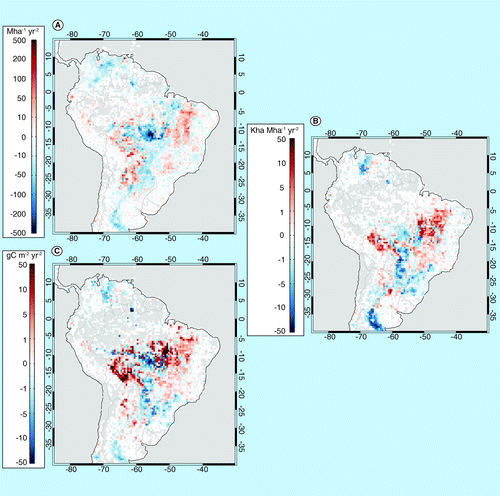
In regions stratified by: (A) biome type; (B) level of deforestation during 2000–2005; and (C) country. Data for the top six countries with the largest number of active fire counts are shown (Brazil data are scaled by 1/5). Right panel shows the annual total active FC (104 yr-1) and FP (days yr-1) values in each region.
Agr: Agriculture; DecF: Deciduous forest; EvgF: Evergreen forest; FC: Fire counts; FP: Fire persistence; NH: Northern hemisphere;
Sav: Savanna; SH: Southern hemisphere.
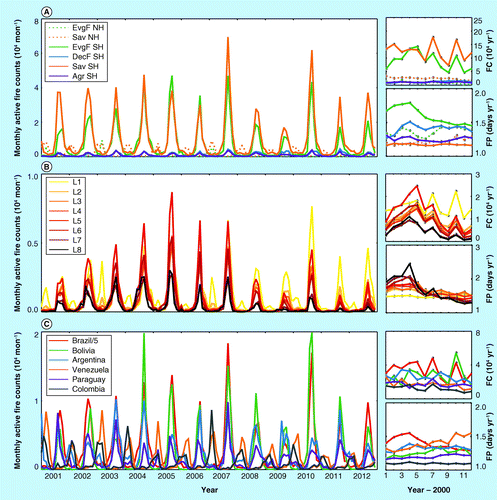
Fire persistence in each region was averaged using all-year mean active fire counts as weighting factors. GFED3 data were averaged over fire affected areas (0.01° regions that had at least one active fire observed by Terra Moderate resolution Imaging Spectroradiometer during 2001–2012). The numbers in the circles represent years after 2000.
Agr: Agriculture; DecF: Deciduous forest; EvgF: Evergreen forest; GFED3: Global Fire Emissions Database version 3; Savn: Savanna.
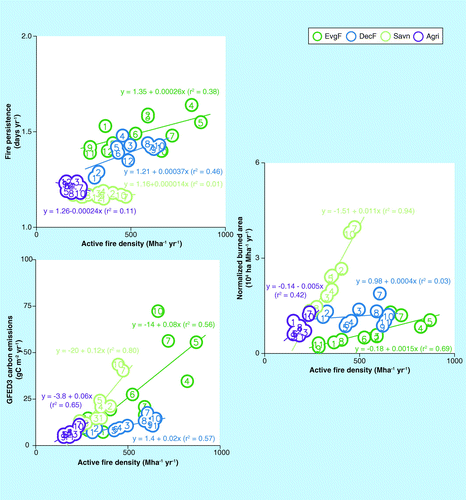
(A) FC, (B) DFA, (C) FP, (D) DFI and (E) GFED3 fire emissions. The spatial distribution of these zones changed year by year according to the annual Program for the Estimation of Deforestation in the Brazilian Amazon deforestation data. FC and FP data were derived from the Terra Moderate resolution Imaging Spectroradiometer active fire product. DFA was the total Program for the Estimation of Deforestation in the Brazilian Amazon deforestation area summed over all of the grid cells (at a 0.05° resolution) for each deforestation land cover classification. DFI was the ratio of FC to DFA.
DFA: Deforestation area; DFI: Deforestation fire intensity; FC: Fire counts; FP: Fire persistence;
GFED3: Global Fire Emissions Database version 3; HiDef: High mean annual deforestation rates;
LoDef: Low mean annual deforestation rates; MedDef: Medium mean annual deforestation rates.
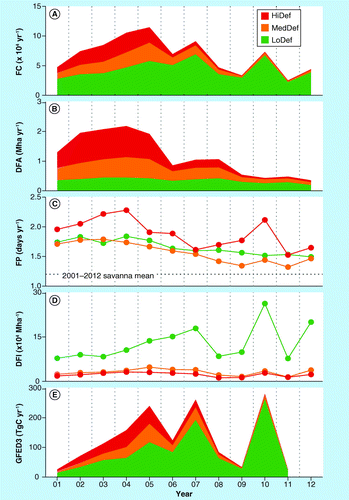
FSS is the sum of Terra Moderate resolution Imaging Spectroradiometer active fire counts during the period from 3 months before the PFM to 4 months after PFM. The early wet season, late wet season and dry season were defined as the 3-month periods centered at 8, 5 and 2 months before PFM, respectively. The axes labels denote latitude (degrees north) and longitude (degrees east). The color bar denotes the magnitude and sign of the correlation coefficient (r).
FSS: Fire season severity; PFM: Peak fire month; PPT: Precipitation; TWS: Terrestrial water storage.
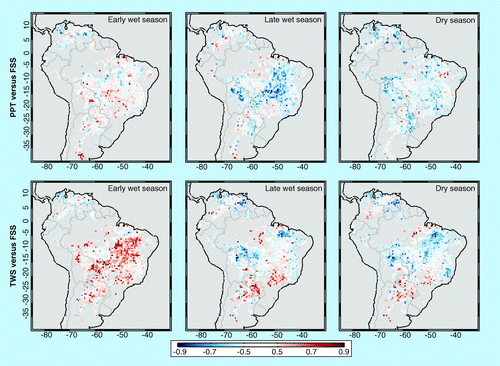
The regressions were performed separately for three Brazilian Amazon zones with different deforestation characteristics: high (HiDef), medium (MedDef) and low (LoDef). Significance levels (p < 0.05) for FSS–PPT and FSS–TWS correlations are 0.60 and 0.67, respectively.
FSS: Fire season severity; PPT: Precipitation; TWS: Terrestrial water storage.
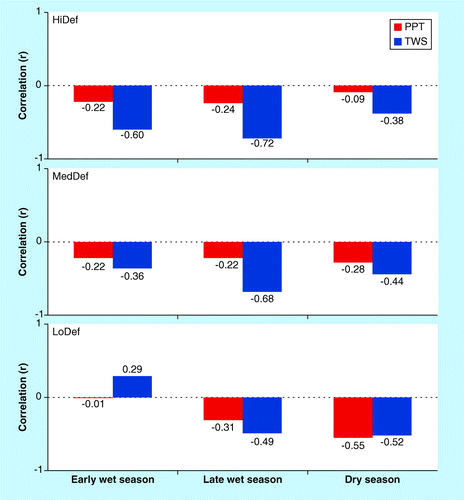
Fires in South America occur in multiple biomes, and are associated with many different natural drivers and human land-use activities. Vegetation fires have been influential in maintaining seasonally dry woodlands and forests Citation[1], as well as savannas in both the southern hemisphere (e.g., the Cerrado in Brazil Citation[2]) and northern hemisphere (e.g., the Llanos in Colombia and Venezuela Citation[3]). In recent decades, fires have been used extensively for land cover conversion, as pasture and croplands expanded into dry and humid forest areas Citation[4,5]. These deforestation activities are concentrated in the ‘arc of deforestation’ along the southern and eastern edges of the Amazon in Brazil and Bolivia Citation[6]. Forest fragmentation caused by land cover change may dry nearby undamaged forests Citation[7], and increase the likelihood that fires may escape from farms and ranches at the deforestation frontier into standing forests during drought periods Citation[8,9].
Fires influence the climate by means of several different pathways, including by emitting GHGs (e.g., CO2 and CH4), aerosols and ozone precursors (NOx and CO) Citation[10]. Amazonian forests alone have a carbon stock that is equivalent to approximately a decade of global contemporary fossil fuel emissions Citation[11]. Averaged over 1997–2009, fire emissions stemming from deforestation activities in South America accounted for approximately 40% of global deforestation fire emissions Citation[12]. Fires also release large amounts of trace gases and aerosols Citation[13,14] that play important roles in modifying regional atmospheric chemistry and climate Citation[15–18]. These interactions have the potential to cause feedbacks that intensify or inhibit fire activity. Zhang et al. showed, for example, that fire-emitted light-absorptive aerosols could work against the seasonal monsoon circulation transition, thus reinforcing the dry season rainfall pattern and increasing the fire potential in southern Amazonia Citation[19].
Forest and savanna fires in South America, particularly those located near the arc of deforestation, vary considerably from year to year Citation[5,20,21]. Part of the interannual variability is associated with climate and the climate–fire feedbacks described above. Climate variables, including temperature, precipitation, wind, moisture and so on, are critical for the ignition, spread and termination of fires. Observations and model studies have shown that Amazon fires are vulnerable to drying in response to circulation changes caused by global warming Citation[22,23]. More fires, in turn, may lead to a dieback of tropical rainforest with potential acceleration of global warming Citation[24], thus increasing the risk of future fires Citation[25]. Land managers may also use periods of unusual drought as a means to more efficiently convert forests to pasture and agricultural fields Citation[26]. Economic factors, such as a growing international demand for beef and soya Citation[27,28], and policy efforts to reduce burning Citation[20], also influence the interannual variability of fires in South America.
To understand the impacts of fires on the carbon cycle and ecosystems, quantitative information on the long-term trends and interannual variability of vegetation fires is needed. Remote sensing provides a unique opportunity to study the fire dynamics and their relationship to climate Citation[29]. By measuring outgoing radiances within mid-infrared channels, satellite radiometers are able to detect active fire locations and quantify fire radiative power with a relatively high spatial and temporal resolution Citation[30–34]. The timing and location information from active fire observations is useful for quantifying fire dynamics in different regions, including areas under human management and small fires Citation[35]. In addition to active fire products, satellite remote sensing observations have also been used to generate global-scale burned area (BA) products by detecting changes in surface reflectance following fire, providing estimates of the extent and severity of fire damages Citation[36–38]. Active fire and BA products represent fundamentally different constraints on fire emissions Citation[39]. In recent years, global fire emission inventories have been developed by combining BA and active fire data with model-based estimates of fuel load Citation[12,40,41], or by using satellite-measured fire radiative power Citation[42–45]. These three types of spatially and temporally explicit data sets (active fires, BA and emissions estimates) provide complementary information for evaluating the interannual variability of fires.
In this study, we used a satellite-derived time series of active fire counts (FC), BA and fire carbon emissions to assess the long-term trends and interannual variability of forest, savanna and agricultural fires in South America between 2001 and 2012. We focused on five sets of inter-related scientific questions:
▪ How have the frequency, locations and characteristics of vegetation fires changed in different biomes and regions of South America during the past 12 years? | |||||
▪ Were there any long-term trends in satellite-based estimates of fire activity and how do these trends vary geographically? | |||||
▪ How was the interannual variability of fires related to the amount of deforestation observed along the arc of deforestation in the Brazilian Amazon? | |||||
▪ What was the role of climate in regulating the interannual variability of vegetation fires? | |||||
▪ How did climate controls on fire activity evolve across space and time? |
To answer these questions, we examined satellite-observed fire patterns by spatially sampling fire observations over the continent in three different ways: by biome type; by the amount of regional deforestation; and by political region. We also used two derived variables to examine the relationship between fire and deforestation/degradation: fire persistence (FP), a measure of repeated burning in the same 1 km areas of the landscape each year, and deforestation fire intensity (DFI), defined as the number of active fires per unit of deforestation area each year. We specifically explored the evolution of annual fire activity within the Brazilian Amazon for regions with low, medium and high annual deforestation rates. Finally, we investigated how the interannual variability and trends of active fires were correlated with satellite-derived time series of precipitation rate and terrestrial water storage during different seasons. Our analysis provides a comprehensive assessment of recent trends in fire activity in South America, including interacting management and climatic drivers of fire activity.
Data & methods
▪ Study area
The South American study area (85°–30°W, 40°S–15°N) was analyzed according to administrative and biome boundaries and levels of recent deforestation . The classification of biome type was based on the Moderate resolution Imaging Spectroradiometer (MODIS) 500 m land cover product (MCD12Q1, collection 5), which represents vegetation cover during the beginning year of our study period (2001) Citation[46]. The accuracy of the land cover product was estimated to be 74.8% globally by quantitative analysis using cross-validation of a training site database Citation[46]. The MODIS International Geosphere-Biosphere Programme (IGBP) vegetation classes were grouped into evergreen forest (EvgF), deciduous forest (DecF), savanna (Savn), agricultural land (Agri) and other vegetation types using the dominant vegetation class within each 0.05° cell. The Savn category used in this study includes five vegetation types in the original MODIS-IGBP data set: ‘savanna’, ‘woody savanna’, ‘open shrubs’, ‘closed shrubs’ and ‘grassland’. The Agri category includes ‘croplands’ and ‘cropland/natural vegetation mosaic’ in the MODIS-IGBP data set. The EvgF region was additionally divided into a nondeforestation zone (L0) and eight deforestation zones (L1–L8) according to the total deforestation fraction from 2000 to 2005 (see section titled ‘Deforestation data’ for more information). Given differences in the timing of fire seasons and remote ocean teleconnections in the northern and southern hemisphere Citation[47], we divided each vegetation type into different classes north and south of the equator.
Within Brazilian Amazon forests (the EvgF region in Brazil, as defined by 2001 MODIS-IGBP data set), we also divided the area into three zones each year (see section titled ‘Deforestation data’ for more information) according to the annual deforestation fraction in each 0.05° cell. This separation accounted for the year-to-year changes in the location of deforestation areas and allowed us to assess if fire use at the deforestation frontier had changed over time within Brazil.
▪ Active fire & carbon emissions data sets
The primary set of active fire observations used in this study was the MODIS collection 5 global monthly fire location product (MCD14ML; ), which has been extensively validated using higher resolution satellite imagery Citation[48,49]. We aggregated all of the individual 1 km active fire observations detected by the Terra MODIS sensor (hereafter, MOD) that had confidence levels greater than 30% (representing confidence classes of nominal to high in the MODIS fire product) within 0.05° grid cells each month. Considering that optically thick clouds may impair the ability of MODIS to detect fires, we corrected the number of active fires each month using monthly cloud fractions from the MODIS climate-model-grid fire product (MOD14CMH; Citation[50]). Although this approach may reduce active fire omission errors, it implicitly assumes that fires occur with the same frequency in cloud-covered and cloud-free areas Citation[51]. Persistent hotspots from MODIS observations Citation[32] and gas flares Citation[52] were excluded from the analysis, because the burning in these pixels was mainly associated with petroleum production or industrial activity rather than vegetation fires. Specifically, we neglected all thermal anomaly observations in 0.05° grid cells that had one or more 1-km persistent (50 or more unique calendar days per year) detections during 2001–2012, or gas flare detections during 1994–2008. Note the exclusion method used in this study was relatively conservative in order to minimize the influence of nonvegetation thermal hotspots.
In addition to the 1 km MOD active fire data set described above, we also analyzed three other active fire products that had coarser spatial resolutions (0.5°; ). The monthly MYD14CMH product includes active fire observations from MODIS sensor on board the Aqua satellite (hereafter, MYD). We only used MYD data during 2003–2012, since Aqua was launched in May 2002. Tropical Rainfall Measuring Mission (TRMM) Visible and Infrared Scanner (VIRS) observations have been used to derive a monthly 0.5° active fire data set that covers latitudes from 40°S to 40°N Citation[31]. The drifting of the TRMM local overpass time allows VIRS to sample over a full diurnal cycle of fire activity Citation[53]. The European Space Agency Advanced Along Track Scanning Radiometer (ATSR) nighttime observations were used to produce global active FC products by two algorithms Citation[54]. We constructed a time series of monthly 0.5° resolution ATSR active FC from algorithm 1. Limited by data availability, we only analyzed VIRS and ATSR active fire observations through the end of 2010 and 2011, respectively. The number of active fire detections can vary significantly from different satellite sensors, owing to differences in sensor characteristics (e.g., spatial resolution and thermal channel saturation levels), local overpass time of the observations, the fire detection algorithm and so on. In this study, we used the four independent sets of observations described above to quantitatively evaluate the robustness of long-term trends and interannual variability of fire characteristics.
The Global Fire Emissions Database (GFED) consists of BA and gas and aerosol emissions from deforestation, savanna, forest, agriculture waste and peat fires. Here we used BA Citation[38] and carbon emissions Citation[12] from GFED version 3 (GFED3) to study the trends in South America. The most recent year available for GFED3 data was 2011 Citation[101].
▪ Deforestation data
Deforested area is a fundamental parameter for estimating carbon fluxes of land-use change and related impacts on global climate. However, due to the spatial heterogeneity and interannual variability in satellite remote sensing products of surface reflectance, an annual estimation of the deforestation area is often difficult. Hansen et al. combined low and high spatial resolution satellite data sets to quantify humid tropical forest clearing during the 2000–2005 interval Citation[55]. Their estimation of forest clearing during this 5-year interval had a similar level of precision to that obtained from higher resolution satellite observations used at a regional scale, but with lower pan tropical spatial resolution (20 km) and reduced costs Citation[55]. The Hansen et al. data set resolves the net change between 2000 and 2005, but does not resolve the interannual variability within this interval Citation[55]. In contrast, the Brazil National Institute for Space Research’s Program for the Estimation of Deforestation in the Brazilian Amazon (PRODES) program provides annual deforestation estimates from 1988 to the present using more than 220 high-resolution Landsat™ images each year Citation[102]. PRODES, which has a sensitivity of 6.5 ha, is one of the most comprehensive deforestation tracking systems in tropical forests, but its data are available only for the Brazilian Amazon.
In this study, the evergreen forest in South America was stratified based on the cumulative deforestation rate during the 5-year period (from August 2000 to July 2005) derived from PRODES data for Brazil and from the Hansen et al. product for other South American countries Citation[55]. We converted the PRODES high-resolution deforestation polygons (GIS shape files) to annual deforestation fraction maps at a 0.05° resolution. We used this relatively coarse spatial resolution to allow for comparisons with active fire observations in a way that minimized the sensitivity of our results to temporal gaps in the satellite coverage of active fires and geolocation uncertainties that occur at high MODIS scan angles. The 2000–2005 cumulative deforestation levels (percent of forest area lost) for L1–L8 zones (shown in ) were between: 0.7–5, 5–10, 10–15, 15–20, 20–30, 30–40, 40–50 and 50–100%.
Within the Brazilian Amazon, we also defined three zones based on annual deforestation fraction from PRODES. Zones HiDef (high levels of annual deforestation), MedDef (medium levels) and LoDef (low levels) included 0.05° grid cells with annual deforestation fractions greater than 8%, between 3 and 8%, and smaller than 3%, respectively. These spatial masks varied from year to year as the deforestation frontier moved into different regions. Fires within these three zones had different characteristics. Many of the fires in the HiDef zone were likely associated with the large-scale deforestation activities that convert forests to croplands and pasturelands Citation[56]. Fires in the MedDef and LoDef zones may include a larger variety of fires, including small-scale deforestation fires, maintenance fires in postdeforestation areas, and escaped fires from adjacent crop fields and pastures.
▪ FP & DFI
Based on the active fire and deforestation data described above, we derived two additional variables to better characterize changes of fire and deforestation patterns in South America. FP has been used in previous studies to measure the repeated burning associated with the deforestation process or slow-moving fires in ecosystems with high fuel loads Citation[12,50,56]. In this study, FP was calculated at a resolution similar to the native satellite resolution of the MODIS active fire product, and is defined as the number of different days each year for which a thermal anomaly was detected at the same location. To quantify annual FP in each 0.05° grid cell, we calculated the total number of fire-detected days divided by the total number of fire-detected pixels (each pixel had a 0.01° × 0.01° resolution, which is close to the nadir resolution of the MODIS fire observations) in a year . By this approach, FP is a measure of the spatial and temporal clustering of active fires across the landscape at a coarser resolution. We defined the units for this quantity as the mean number of fire-detected days per year (or days per year in short form). The spatial distribution of active fires in forest, savanna and agricultural regions illustrates the different patterns of FP in these cover types .
DFI, a measure of the number of active fires per unit of forest clearing, was calculated as the ratio of MOD active FC to the PRODES deforestation area in the Brazilian Amazon for each year. PRODES deforestation estimates represent annual clearing activity between August of the previous year and July of the current year. Since Morton et al. estimated that the majority of deforestation fires in Mato Grosso were observed during the year of deforestation detection Citation[56], we compared active fire observations and PRODES deforestation estimates from the same calendar year. DFI represents the amount of active fire detections that could be associated with the deforestation process at a regional scale (0.05°), either as intentionally set fires associated with forest clearing, or accidental fires that burn into nearby areas. DFI in this study differs from the conventional definition of fire intensity, which is a measure of energy released during various phases of a fire Citation[57]. We note that in this calculation, the spatial location of the deforestation area evolved over time as the deforestation frontier moved into interior forests.
▪ Climatic variables & their correlations with fires
Fires across the tropics are closely related to climate anomalies, which influence both fuel loads, and the intensity and length of the dry season Citation[26,58]. Precipitation during the dry season usually increases the moisture of the vegetation, soil and the atmosphere, thereby decreasing the probability of ignition, and also rates of fire spread and fuel consumption. Precipitation during the wet season, however, may promote the buildup of herbaceous aboveground biomass, and, thus, increase the density of fuels during the following dry season. Recent studies have shown that sea surface temperatures in the surrounding tropical oceans Citation[47,58], and terrestrial water storage observations from the Gravity Recovery and Climate Experiment (GRACE) Citation[59], can provide important early warning information about fire season severity in tropical and subtropical South American forests. For arid ecosystems, satellite-derived estimates of fire activity have been shown to be limited by the density of available fuels Citation[60,61].
Here we explored the relationship between fire season severity (defined as the sum of MODIS observed active FC during the 9-month period centered at the peak fire month) and two climatic data sets sampled during different seasons. The precipitation data we used are the monthly TRMM 3B43 product (version 6) at 0.25° spatial resolution. We also used monthly gridded (1°) terrestrial water storage data that was derived from GRACE Citation[62,63]. These observations were compiled by the Tellus group of NASA’s Jet Propulsion Laboratory (release 04). The GRACE mission includes twin satellites that detect the changes in the gravity field of the Earth. After suitable adjustments for mass redistribution (e.g., postglacial rebound and large earthquakes), as well as specific destriping and smoothing of the data, the GRACE observations have been used to measure the changes in water storage on land masses Citation[64–66]. We specifically calculated trends in precipitation and terrestrial water storage averaged over the early wet season, the later wet season and the dry season, and their correlations with the fire season severity. In South America, the 3 months immediately before the fire peak month are often the driest months Citation[47]. Therefore, we defined the early wet season, the late wet season and the dry season in this study as the 3-month periods that centered at 8, 5 and 2 months before the peak fire month, respectively.
Results
▪ Satellite-observed fire patterns in South America
Spatial distributions of active fire densities & FP
Satellite observations illustrate the heterogeneous spatial distribution of active fires in South America . Here the active fire density was defined as the total annual active FC per unit of land area in each 0.05° grid cell, with units of number of active fires per million hectares per year. The southern and eastern arc of deforestation in the Amazon showed the highest density of fire detections corresponding to areas with rapid rates of deforestation . Active fires were also concentrated in other biomes, including DecFs (mostly in the Gran Chaco in southern Bolivia, northern Argentina and western Paraguay), savannas (e.g., the Cerrado in Brazil, and the Llanos in Colombia and Venezuela) and Agri in southern Brazil. Overall, active fires in evergreen forests and savannas accounted for approximately 90% of the total MOD FC in South America (Supplementary Table 1). Within the evergreen forest region, active fires were observed in all sub-areas stratified by level of deforestation (Supplementary Table 2). Fire detections were more numerous in L1 (accounting for ∼22% of the total FC in L1–L8) than other deforestation zones, yet active fire densities were higher on a per-area basis with increasing deforestation rates between L1 and L8 (see column FD in Supplementary Table 2). Geographically, Brazil had the largest number of active fire observations (∼60% of that in South America), followed by Bolivia (∼10%) and Argentina (∼9%) (Supplementary Table 3). Spatial patterns from other satellites (Supplementary Figure 1), including MYD, VIRS and ATSR, were similar to the MOD observations, although the numbers of active fires detected by these sensors varied significantly (Supplementary Tables 1–3).
FP provides insight about the patterns of land use and the level of fuel consumption available for landscape fires. In evergreen and DecFs, FP values were often elevated in regions with deforestation for agricultural expansion , and Supplementary Figure 2 & Table 1). Evidence for active fires in these forest regions at the same pixel location during multiple satellite overpasses is consistent with higher fuel loads and/or management activities that allow fires to persist for several days. Relative to other evergreen forest regions, FP in regions with extensive deforestation (e.g., L7–L8) was even higher (Supplementary Table 2 & Supplementary Figure 2), consistent with repeated burning of felled trees multiple times during the dry season Citation[56]. In savannas and grasslands, however, consumption of available fuels and fire spread often occur quickly Citation[67]. The MODIS-derived FP values in savanna regions were therefore often very low (Supplementary Table 1 & Supplementary Figure 2). Taken together, these results confirm the value of FP as an indicator of fires associated with human-driven forest clearing.
Trends & interannual variability of active fires
Long-term trends of active fire density derived from MOD, MYD, VIRS and ATSR during the first 12 years of the 21st century are presented in & Supplementary Figure 3 at a 0.5° spatial resolution. For each grid cell, we calculated the trend using a linear regression on the annual time series of active fire observations. All four satellite data sets showed a significant decreasing trend along the arc of deforestation in Brazil, with the largest decrease in the Brazilian state of Mato Grosso (Supplementary Figure 3). To the north and west of the arc of deforestation, satellite active fire observations showed a positive trend, consistent with the advance of the deforestation frontier towards the interior of the Amazon Basin during this period. In these areas, deforestation and degradation fires have increased during the past decade, including in areas with low deforestation activity during 2000–2005. also shows a significant positive trend in the savannas of Cerrado and Gran Chaco regions, a small positive trend in the croplands of southern Brazil and a negative trend in northern hemisphere savannas (the Llanos).
Year-by-year maps of active fires (Supplementary Figure 4) and the time series of total active FC in different subregions & Supplementary Tables 1–3) indicated that fires in South America varied considerably on interannual timescales (normalized SDs ranged from approximately 16% for Savn to 37% for EvgF). Fires associated with the deforestation frontier in evergreen forests increased in the first half of the decade, peaked during 2004–2005, and then showed a decreasing trend and high interannual variability thereafter . Although these patterns are clearly observable in MOD active fires , the trajectory of deforestation and associated fire activity during the MODIS era may not be captured in the linear trend analysis . Fires in DecFs in the southern hemisphere were characterized by a consistent increasing trend , although the total active FC in these regions were much smaller than in evergreen forests and savannas. Savanna fires were less variable than forest fires during 2001–2005, but varied significantly during 2005–2012, with high extremes observed in 2007 and 2010.
Trends and interannual variability in Terra MODIS active fires for these biomes were corroborated by other satellite active fire products. Most satellites showed similar decreasing trends for evergreen forests and savannas during the latter part of the record, and an increasing trend for DecFs . Note that the decreasing trends for MYD active fires were larger because the first full-year set of observations from Aqua was from 2003, and thus two relatively low-fire years (2001 and 2002) were excluded from the trend analysis.
Within evergreen broadleaf forests, the decreasing trend of active fires was larger in regions with a higher cumulative deforestation rate during 2000–2005 & . The decline in active fires was consistent with regional declines in deforestation during 2006–2012 (see section titled ‘Fires & deforestation in the Brazilian Amazon’) and the advance of the deforestation frontier during the past 12 years relative to the scale of analysis. Interannual variability of active FC in low-deforestation regions (e.g., L1) was similar to that of savanna fires, with small interannual variability in early years and exceptionally high numbers of fires observed during 2007 and 2010 . Total active FC in evergreen forests declined after 2005 at a rate of -11.1% yr-1 (p < 0.08), indicating lower levels of burning overall within this biome. The decline in fires and deforestation in evergreen forests has been attributed, in part, to economic drivers such as the change of soy price in global market Citation[27,68] and new monitoring activities, including industry-led initiatives to remove deforestation from their direct supply chains and government actions to improve enforcement of existing environmental laws. Although it is difficult to systematically quantify the individual impacts of climate, economic and policy drivers, a recent study by Macedo et al. suggests that improved forest conservation measures may have had a larger influence than the changes in the soybean market during the latter part of the 2001–2010 decade Citation[68]. Declining deforestation since 2005 is also consistent with the timing of international attention on REDD, where Brazil has been a leader in setting deforestation reduction targets Citation[69], and implementing new programs for property registration, fire permits and satellite-based monitoring to support REDD objectives.
Trends in active fires for different countries and states varied considerably and reflected, in part, the mixture of biomes and levels of deforestation activity within each of the administrative regions . Among the South American countries, Peru, Chile and Bolivia showed the largest rates of increase in active fire observations during the study period, while Colombia and Venezuela had the largest rates of decrease. The state of Mato Grosso in Brazil had one of the largest regional reductions in active fires during the 2001–2012 period (-8.4% yr-1 from Terra MODIS observations).
Trends & interannual variability of FP
The interannual variability of FP in different biomes and regions in South America provided important evidence for the changing role of deforestation for regional fire activity. Mean FP in savannas was constantly low (∼1.15; see & Supplementary Figures 2 & 5) despite significant interannual variability (measured by the SD of annual time series) in active FC (Supplementary Table 1). Mean FP in DecFs increased from approximately 1.3 in 2001 to 1.45 in 2003, and then remained relatively constant, despite declining fire detections in Argentina and Paraguay . FP in evergreen forests, particularly in regions with high levels of deforestation during 2000–2005, had an increasing trend from 2001 to 2004 and then a significant decline after 2005. In later years, FP in regions with extensive deforestation during 2000–2005 (L5–L8) was actually lower than that in areas with less extensive deforestation (L1–L4) . This result is consistent with the advance of the deforestation frontier in the evergreen forests and a changing pattern of fire use in existing cleared areas.
FP was positively correlated with active fire density in forest regions , suggesting that the level of fuel accumulation and consumption was generally higher during years with higher levels of fire activity. The concurrent increases in total FC and area-weighted mean FP from 2001 to 2005 were more apparent in regions with higher deforestation rates . This implies that a significant change in the deforestation rate may have been mainly responsible for the year-by-year increase in active FC during this period. Climate conditions during the latter part of this period (i.e., the 2005 drought) may have further increased fire severity, thus amplifying fuel consumption and carbon emissions. The relationship between FC and FP changed significantly after 2005, when the EvgF FP decreased monotonically and largely independent of active FC . In this later stage, the climate variation likely had a larger role in determining the interannual variability of the number of active fires.
BA & fire carbon emissions
The long-term trends in BA and carbon emissions from GFED3 also confirmed the satellite-observed fire front moving toward the interior Amazon . The positive trend of fire emissions along the leading edge of the arc of deforestation had a larger slope and was more significant than the trends of active FC and BA, which is consistent with the fire front moving into regions with higher-biomass forest types Citation[64].
BA and fire emissions from GFED3 were highly correlated with MOD active fire density in savanna and evergreen forest biomes . The relationship between GFED3 fire emissions and MOD active fire observations was expected in evergreen forests, given that MODIS active FC and FP were both used to determine fire-driven forest clearing in GFED model Citation[12]. The slope of the relationship between mean annual BA and active FC was much higher in savannas than in evergreen forests; previous work has shown that rapid movement of the fire front in grasslands limits the number of active fire detections per unit of BA Citation[47].
▪ Fires & deforestation in the Brazilian Amazon
Since a majority of the deforestation area in South America was located in Brazil , we focused on the relationship between annual fire activity and deforestation in the Brazilian Amazon based on three levels of annual deforestation: LoDef, MedDef and HiDef. Total active FC in the Brazilian Amazon increased by more than a factor of 2 between 2001 and 2004 . Total deforested area also increased during this period, but by a smaller amount . Fires increased in all three deforestation zones, while most of the increase in deforested area occurred in the HiDef region. This suggests that the increase of active FC in the Brazilian Amazon during 2001–2004 was caused in part by greater numbers of fires in the deforestation process, which has been linked in past work with large-scale mechanized conversion of forests to intensively managed croplands Citation[56]. The growing fraction of the basin under different forms of Agri management, in which fires are often used for pasture maintenance and other purposes, may also contribute to increasing fire detections in these regions. Not surprisingly, the FP values also increased during this period, most notably in the HiDef region .
In contrast, during the latter part of the record (2005–2012), year-to-year variability in active fires within the Brazilian Amazon was high and total fire detections had a significant decreasing trend (-15% yr-1, p < 0.03). Fires associated with large-scale (HiDef) and medium (MedDef) deforestation dropped to very low levels. The mean FP , the mean DFI and total fire carbon emissions in these regions also decreased significantly. Active fire observations in regions where deforestation was dominated by small-scale activities (LoDef), however, declined by a smaller amount during this period (-7.3% yr-1, p < 0.23). Declines in active fires were smaller than the drop in deforestation rates (-8.6% yr-1, p < 0.01). As a consequence, the DFI, which represents the number of fires potentially used for forest clearing, also showed an increase trend in this region . The number of annual active fires was observed to have a positive trend during 2001–2012 in 31% of the Brazilian Amazonia area where the deforestation rate concurrently decreased . Although this fraction was smaller than that reported by Aragao and Shimabukuro for 2000–2007, it still represents a large area where reducing deforestation did not limit the number of satellite-detected fires Citation[21].
Multiple lines of evidence suggest that the rise in fire activity in 2007 and 2010 was driven by climate, rather than deforestation activity. The increase in fire detections during 2007 and 2010 was concentrated in the LoDef region . Furthermore, FP values remained low in these years and overall deforestation area was consistent with other years (e.g., 2008 and 2009). Given that the FP values in evergreen forests of South America were lower than that expected from the active fire–FP relationship , it suggests that other fire types were responsible for the anomalies during these years. Supplementary Figure 4 further indicates that increases in the number of fires observed during 2007 and 2010 occurred across the eastern border of the Amazon, where woodlands and savannas were more common, and FP was small (Supplementary Figure 5). At the same time, we found that BA (relative to active FC) in these 2 years was also exceptionally high . Together, these findings indicated that a significant number of the fires in 2007 and 2010 observed across the arc of deforestation were not those typically associated with deforestation, but more likely woodland, savanna and understory forest fires that had lower levels of fuel consumption per unit of BA.
▪ Climate influences on the interannual variability of fires
The interannual variability of fire season severity in the South American deforestation region was negatively correlated with precipitation during the late wet season, and terrestrial water storage during the late wet season and the dry season . Fire season severity in the savannas of South America (e.g., Brazilian Cerrado) was found to be positively correlated with terrestrial water storage during the early wet season, suggesting that accumulation of fuel loads in savannas may increase levels of fire activity during the following dry season Citation[60]. Agricultural fires in southeastern Brazil were also positively correlated to terrestrial water storage before the fire season.
Within the Brazilian Amazon, fire season severity in the MedDef and HiDef regions showed the largest negative correlation with terrestrial water storage in the late wet season . The fire season severity time series were also negatively correlated with the mean precipitation rate, but the correlation between fire and precipitation was much weaker. In regions dominated by small-scale deforestation (LoDef), fire season severity responded to climate variation in a different way. In some locations, higher terrestrial water storage during the early wet season was associated with higher active FC observed in the fire season. Terrestrial water storage and precipitation rate in the late wet season and dry season, however, showed negative correlations with fire season severity . The diversity of fire types in the LoDef region may partially explain the complex climate–fire interactions at the leading edge of the deforestation frontier.
Discussion
In this article, we used satellite-based estimates of fire activity, precipitation and terrestrial water storage to quantitatively assess long-term trends and interannual variability of vegetation fires during 2001–2012 in South America. The consistent trends across the different fire products allowed us to begin to identify the role of land-use change and climate variability drivers. The time period was not long enough, however, to examine the direct or indirect effects of long-term climate change, including the impacts of anthropogenic climate warming on fire weather or plant functional type composition. Attribution of long-term fire trends to various forms of global environmental change remains an important goal of the Earth observation community.
Four primary themes emerge from our analysis. First, economic and climate drivers of fire activity in South America leave distinct fingerprints in the record of satellite observations. The location, timing and frequency of fire use for deforestation, as captured in FP, provided critical information to assess the contribution from deforestation activity to total fire detections in South America. Positive trends in fire activity were common at the leading edge of the deforestation frontier. In contrast, regions of the arc of deforestation with substantial deforestation in 2000–2005 generally experienced a decline in FP and total fire activity during the latter half of the study period. These findings suggest a reduction in the contribution of deforestation fires to the total number of observed fires after 2005 in South America.
Our analysis showed that total fire activity declined at a slower rate than deforestation. Several factors may contribute to this difference. Deforestation fires may continue after the initial clearing, either to remove residual biomass to permit mechanized agriculture Citation[56], or to allow for removal of higher biomass levels in more recent clearings Citation[70]. Concurrently, expansion of the area under agricultural management may elevate the base level of fire activity associated with land management Citation[71–73]. Finally, other fire types such as understory forest fires probably account for some fraction of the observed increase in DFI in areas with small clearing sizes Citation[74,75]. The parallel reductions in FP during 2004–2012 suggest that the latter two explanations may be more plausible. Although more research is needed to understand the relative importance of these drivers, the overall trends in active FC, deforestation areas and DFI suggest that the contribution of fires to the sum of deforestation and forest degradation carbon losses may be increasing within the Brazilian Amazon. Our findings suggest that increases in fire activity observed in interior forests during 2001–2007 by Aragao and Shimabukuro continued through the end of our study period (2012) Citation[21], with important consequences for total fire emissions from deforestation and forest degradation in the region.
The second major theme is the identification of the significant increasing trend in DecF fires in southern Bolivia, northern Argentina and western Paraguay (i.e., the Gran Chaco region; , & Supplementary Figure 3). These fires may reduce carbon stocks and affect air quality within the region, offsetting carbon storage gains associated with tropical deforestation reductions in Brazil and in other regions in recent years. Further research is needed using higher resolution imagery to quantify postfire tree mortality and other longer-term changes in ecosystem function associated with the changing fire regime in this biome. Managing fires in DecFs to stabilize or reduce BA may yield opportunities for REDD+ that extend beyond traditional tropical forest boundaries.
The third major theme from the analysis of fire activity in South America is the similar climate sensitivity of fires in savanna and tropical forest biomes. As Amazon deforestation declined, active fire detections in evergreen forest and savanna biomes of the southern hemisphere of South America covaried from year to year . Traditionally, fire ecology has pointed to different climate drivers of fire risk in closed-canopy tropical forests Citation[28,76], and savanna or grassland ecosystems Citation[77]. What factors might synchronize regional fire activity in South America, even across biome boundaries? We specifically considered the roles of precipitation and terrestrial water storage for fire season severity, the sum of all active fire detections in a year. Dry season terrestrial water storage was negatively correlated with fire season severity in both the Cerrado and deforestation regions in the Brazilian Amazon . Soil water deficits could decrease local fuel moisture, thereby increasing fire risk. Additionally, soil water deficits may influence regional fire risk through evapotranspiration (ET). Lower ET could influence atmospheric humidity and downwind precipitation Citation[78]. Synoptic events that transport dry air into central South America could be enhanced or maintained by periods with reduced ET. Previous studies have pointed to low nighttime relative humidity as one potential control on multiday fires in Amazon forests Citation[75].
The fourth key theme from our analysis is the seasonal fire–climate relationship in South America. Terrestrial water storage at the end of the wet season is an important controller of fire activity during the subsequent dry season in many forest and savanna-dominated regions . This finding has important implications for fire forecasts and drought management Citation[47,59], as well as scientific understanding of potential changes in regional fire activity under scenarios of climate change. Previous studies have focused projections of dry season precipitation to assess the likelihood of changes in Amazon fire activity Citation[79]. However, fewer models in the IPCC Fourth Assessment Report indicated a decrease in wet season precipitation over the Amazon Citation[79], a key control on terrestrial water storage Citation[59].
Our analysis utilized complementary data on fire activity from satellite-based active fire detections, BA mapping and carbon emissions estimates. However, there are several limitations of this approach that could be addressed in future work. First, we used a linear detection approach for trend analysis. Some trends in deforestation fire activity were likely underestimated in this study, given the pattern of increasing (2000–2005) and decreasing (2006–2012) deforestation during the period of analysis. Second, active fire time observations can have false detections (commission errors) under certain land surface conditions. Although Terra MODIS data are the one of the most validated time series of fire activity in South America since 2000, false detections do occur, particularly at the deforestation frontier Citation[5]. Third, in this study we used only active fire detections from satellites in low altitude orbits. These satellites offer global coverage and generally have moderate spatial resolution. Geostationary platforms, which provide continuous observations throughout the day and night, have also been used with considerable success for fire detection in tropical regions Citation[80,81]. Creating a fusion of fire information from both types of sensors has been a focus of several recent studies Citation[82–85]. Fourth, new higher resolution approaches are needed to more accurately partition fires according to different land management activities. Specifically, although FP is a useful metric at a regional scale, not all deforestation fires are detected by MODIS. This limits the ability of this metric to separate deforestation and other fire types at the deforestation frontier. Within biogeochemical models, such as GFED, an important remaining challenge is to separately quantify carbon emissions from degradation and deforestation fires.
Future perspective
The spatial and temporal variability of fire activity in South America during 2001–2012 highlighted the importance of human activity as a driver of the location and frequency of burning. Trends in fire activity indicated a regional decline in fire-driven deforestation, and a shift in the location of the leading edge of deforestation activity in the Amazon and the Gran Chaco during this period. The synchrony of year-to-year variations in fire activity in forest and savanna cover types, strongly coupled to interannual variability in wet season terrestrial water storage, provided information about regional fire–climate interactions. These patterns may have implications for fire management in South America, including the potential to allocate resources to regions or years with a higher likelihood of widespread fire risk. The combination of high and low fire years during the MODIS era may also inform modeling activities of future fire activity and carbon emissions from South America based on a range of future demands for agricultural expansion Citation[86] and changing climate Citation[87,88]. An important direction for future research is to understand how these contemporary and future trends in fires may contribute to feedbacks with regional and global climate, by releasing gases and aerosol particles and by modifying land cover.
Table 1. List of the fire, deforestation and climatic data sets used in this study.
Table 2. Long-term trends of fire characteristics during 2001–2012 in different regions of South America stratified by biome type.
Table 3. Long-term trends of fire characteristics for evergreen forest regions stratified by the amount of deforestation during 2000–2005.
Table 4. Long-term trends of fire characteristics for regions stratified by political division (country and state).
Total water storage at or below the Earth’s terrestrial surface, including vegetation water content, soil moisture, groundwater, surface water, river and stream volume, and snow and ice.
Number of fires detected each year by a satellite sensor per unit of land area.
Anthropogenic fires that contribute to tree mortality and carbon emissions, but are not directly associated with intentional forest clearing.
Loss of water from the canopy and soil, by evaporation and transpiration from plant leaves.
Satellite-observed fire patterns in South America
▪ The number of annual active fires detected by four satellites showed a consistent increasing trend in South America during 2001–2005. The trends were mostly driven by deforestation fires in tropical evergreen forests. | |||||
▪ Fires between 2005 and 2012 had a small decreasing trend and large year-to-year variations. The decreasing trend was caused in part by a reduction in the annual deforestation rates in Brazil, whereas the interannual variations were likely associated with climate variability, including severe droughts in 2005, 2007 and 2010. | |||||
▪ A significant increasing trend was identified for deciduous forest fires in the Gran Chaco, which may partly offset carbon storage gains associated with tropical deforestation reductions in Brazil and other regions of South America in recent years. |
Fires & deforestation in the Brazilian Amazon
▪ The observed trends of deforestation fire intensity and fire persistence within the Brazilian Amazon indicate that fires associated with forest degradation are becoming an increasingly important component of the fire regime and driver of carbon emissions from the region. |
Climate influences on the interannual variability of fires
▪ Fires in savanna and tropical forest biomes concurrently increased in response to drought events in 2005, 2007 and 2010, highlighting the role of terrestrial water storage in influencing regional fire season severity. | |||||
▪ Although projections of climate–fire relationships in Amazonia typically emphasize dry season precipitation, our results suggest that wet season precipitation may be a more important control on regional fire activity and associated carbon emissions. |
tcmt_a_10816404_sm0001.pdf
Download PDF (694.5 KB)Acknowledgements
We thank the Brazilian National Institute for Space Research (São José dos Campos, Brazil) for making the Program for the Estimation of Deforestation in the Brazilian Amazon deforestation data freely and publicly available. We thank L Giglio (University of Maryland, MD, USA) for comments on an earlier draft.
Financial & competing interests disclosure
This research was supported by NASA grants NNX08AF64G and NNX11AF96G, and the Gordon and Betty Moore Foundation (CA, USA; GBMF3269). The authors have no other relevant affiliations or financial involvement with any organization or entity with a financial interest in or financial conflict with the subject matter or materials discussed in the manuscript apart from those disclosed.
No writing assistance was utilized in the production of this manuscript.
References
- Taylor D. Biomass burning, humans and climate change in Southeast Asia. Biodivers. Conserv.19(4),1025–1042 (2010).
- Mistry J. Fire in the cerrado (savannas) of Brazil: an ecological review. Prog. Phys. Geogr.22(4),425–448 (1998).
- Romero-Ruiz M, Etter A, Sarmiento A, Tansey K. Spatial and temporal variability of fires in relation to ecosystems, land tenure and rainfall in savannas of northern South America. Glob. Chang. Biol.16(7),2013–2023 (2010).
- Setzer AW, Pereira MC. Amazonia biomass burnings in 1987 and an estimate of their tropospheric emissions. Ambio20(1),19–22 (1991).
- Schroeder W, Alencar A, Arima E, Setzer A. The spatial distribution and interannual variability of fire in Amazonia. In: Amazonia and Global Change. Gash J, Keller M, Silva Dias P (Eds). American Geophysical Union, Washington, DC, USA, 43–60 (2009).
- Fearnside PM. Deforestation in Brazilian Amazonia: history, rates, and consequences. Conserv. Biol.19(3),680–688 (2005).
- Nepstad DC, Verissimo A, Alencar A et al. Large-scale impoverishment of Amazonian forests by logging and fire. Nature398(6727),505–508 (1999).
- Siegert F, Ruecker G, Hinrichs A, Hoffmann AA. Increased damage from fires in logged forests during droughts caused by El Niño. Nature414(6862),437–440 (2001).
- Cochrane MA. Fire science for rainforests. Nature421(6926),913–919 (2003).
- Bowman D, Balch JK, Artaxo P et al. Fire in the Earth System. Science324(5926),481–484 (2009).
- Saatchi SS, Harris NL, Brown S et al. Benchmark map of forest carbon stocks in tropical regions across three continents. Proc. Natl Acad. Sci. USA108(24),9899–9904 (2011).
- van Der Werf GR, Randerson JT, Giglio L et al. Global fire emissions and the contribution of deforestation, savanna, forest, agricultural, and peat fires (1997–2009). Atmos. Chem. Phys.10,11707–11735 (2010).
- Andreae MO, Merlet P. Emission of trace gases and aerosols from biomass burning. Global Biogeochem. Cycles15(4),955–966 (2001).
- Martin ST, Andreae MO, Artaxo P et al. Sources and properties of Amazonian aerosol particles. Rev. Geophys.48(2),RG2002 (2010).
- Andreae MO, Rosenfeld D, Artaxo P et al. Smoking rain clouds over the Amazon. Science303(5662),1337–1342 (2004).
- Yu H, Fu R, Dickinson RE, Zhang Y, Chen M, Wang H. Interannual variability of smoke and warm cloud relationships in the Amazon as inferred from MODIS retrievals. Remote Sens. Environ.111(4),435–449 (2007).
- Koren I, Martins JV, Remer LA, Afargan H. Smoke invigoration versus inhibition of clouds over the Amazon. Science321(5891),946–949 (2008).
- Patadia F, Gupta P, Christopher SA, Reid JS. A Multisensor satellite-based assessment of biomass burning aerosol radiative impact over Amazonia. J. Geophys. Res.113(D12),D12214 (2008).
- Zhang Y, Fu R, Yu HB et al. Impact of biomass burning aerosol on the monsoon circulation transition over Amazonia. Geophys. Res. Lett.36(10),L10814 (2009).
- Koren I, Remer LA, Longo K. Reversal of trend of biomass burning in the Amazon. Geophys. Res. Lett.34(20),L20404 (2007).
- Aragao L, Shimabukuro YE. The incidence of fire in Amazonian forests with implications for REDD. Science328(5983),1275–1278 (2010).
- Hoffmann WA, Schroeder W, Jackson RB. Regional feedbacks among fire, climate, and tropical deforestation. J. Geophys. Res.108(D23),4721 (2003).
- Li WH, Fu R, Dickinson RE. Rainfall and its seasonality over the Amazon in the 21st century as assessed by the coupled models for the IPCC AR4. J. Geophys. Res.111(D2),D02111 (2006).
- Cox PM, Betts RA, Collins M, Harris PP, Huntingford C, Jones CD. Amazonian forest dieback under climate-carbon cycle projections for the 21st century. Theor. Appl. Climatol.78(1–3),137–156 (2004).
- Cochrane MA, Alencar A, Schulze MD et al. Positive feedbacks in the fire dynamic of closed canopy tropical forests. Science284(5421),1832–1835 (1999).
- van der Werf GR, Dempewolf J, Trigg SN et al. Climate regulation of fire emissions and deforestation in equatorial Asia. Proc. Natl Acad. Sci. USA105(51),20350–20355 (2008).
- Morton DC, Defries RS, Shimabukuro YE et al. Cropland expansion changes deforestation dynamics in the southern Brazilian Amazon. Proc. Natl Acad. Sci. USA103(39),14637–14641 (2006).
- Nepstad DC, Stickler CM, Almeida OT. Globalization of the Amazon soy and beef industries: Opportunities for conservation. Conserv. Biol.20(6),1595–1603 (2006).
- Asner GP, Alencar A. Drought impacts on the Amazon forest: the remote sensing perspective. New Phytol.187(3),569–578 (2010).
- Arino O, Rosaz J-M, Goloub P. The ATSR world Fire atlas. A synergy with “Polder” aerosol products. Earth Obs. Quarterly64,1–6 (1999).
- Giglio L, Kendall JD, Mack R. A multi-year active fire dataset for the tropics derived from the TRMM VIRS. Int. J. Remote Sens.24(22),4505–4525 (2003).
- Giglio L, Csiszar I, Justice CO. Global distribution and seasonality of active fires as observed with the Terra and Aqua Moderate Resolution Imaging Spectroradiometer (MODIS) sensors. J. Geophys. Res. Biogeosci.111(G2),G02016 (2006).
- Giglio L, Csiszar I, Restas A et al. Active fire detection and characterization with the advanced spaceborne thermal emission and reflection radiometer (ASTER). Remote Sens. Environ.112(6),3055–3063 (2008).
- Schroeder W, Prins E, Giglio L et al. Validation of GOES and MODIS active fire detection products using ASTER and ETM plus data. Rremote Sens. Environ.112(5),2711–2726 (2008).
- Randerson JT, Chen Y, van der Werf GR, Rogers BM, Morton DC. Global burned area and biomass burning emissions from small fires. J. Geophys. Res. Biogeosci.117(G4),G04012 (2012).
- Roy DP, Boschetti L, Justice CO, Ju J. The collection 5 MODIS burned area product – global evaluation by comparison with the MODIS active fire product. Remote Sens. Environ.112(9),3690–3707 (2008).
- Tansey K, Gregoire JM, Defourny P et al. A new, global, multi-annual (2000–2007) burnt area product at 1 km resolution. Geophys. Res. Lett.35,L01401 (2008).
- Giglio L, Randerson JT, van der Werf GR et al. Assessing variability and long-term trends in burned area by merging multiple satellite fire products. Biogeosciences7(3),1171–1186 (2010).
- Langmann B, Duncan B, Textor C, Trentmann J, van der Werf GR. Vegetation fire emissions and their impact on air pollution and climate. Atmos. Environ.43(1),107–116 (2009).
- Hoelzemann JJ, Schultz MG, Brasseur GP, Granier C, Simon M. Global wildland fire emission model (GWEM): evaluating the use of global area burnt satellite data. J. Geophys. Res.109,D14S04 (2004).
- Ito A, Penner JE. Global estimates of biomass burning emissions based on satellite imagery for the year 2000. J. Geophys. Res.109,D14S05 (2004).
- Wooster MJ, Roberts G, Perry GLW, Kaufman YJ. Retrieval of biomass combustion rates and totals from fire radiative power observations: FRP derivation and calibration relationships between biomass consumption and fire radiative energy release. J. Geophys. Res.110,D24311 (2005).
- Ichoku C, Giglio L, Wooster MJ, Remer LA. Global characterization of biomass-burning patterns using satellite measurements of fire radiative energy. Remote Sens. Environ.112(6),2950–2962 (2008).
- Vermote E, Ellicott E, Dubovik O et al. An approach to estimate global biomass burning emissions of organic and black carbon from MODIS fire radiative power. J. Geophys. Res.114,D18205 (2009).
- Kaiser JW, Heil A, Andreae MO et al. Biomass burning emissions estimated with a global fire assimilation system based on observed fire radiative power. Biogeosciences9,527–554 (2012).
- Friedl MA, Mciver DK, Hodges JCF et al. Global land cover mapping from MODIS. algorithms and early results. Remote Sens. Environ.83(1–2),287–302 (2002).
- Chen Y, Randerson JT, Morton DC et al. Forecasting fire season severity in South America using sea surface temperature anomalies. Science334(6057),787–791 (2011).
- Morisette JT, Giglio L, Csiszar I et al. Validation of MODIS active fire detection products derived from two algorithms. Earth Interact.9,1–24 (2005).
- Morisette JT, Giglio L, Csiszar I, Justice CO. Validation of the MODIS active fire product over Southern Africa with ASTER data. Int. J. Remote Sens.26(19),4239–4264 (2005).
- Giglio L, van der Werf GR, Randerson JT, Collatz GJ, Kasibhatla P. Global estimation of burned area using MODIS active fire observations. Atmos. Chem. Phys.6,957–974 (2006).
- Schroeder W, Csiszar I, Morisette J. Quantifying the impact of cloud obscuration on remote sensing of active fires in the Brazilian Amazon. Remote Sens. Environ.112(2),456–470 (2008).
- Elvidge CD, Ziskin D, Baugh KE et al. A fifteen year record of global natural gas flaring derived from satellite data. Energies2(3),595–622 (2009).
- Giglio L. Characterization of the tropical diurnal fire cycle using VIRS and MODIS observations. Remote Sens. Environ.108(4),407–421 (2007).
- Arino O, Plummer S, Casadio S. Fire disturbance: the twelve years time series of the ATSR world fire atlas. Proceedings of the ENVISAT Symposium 2007. Montreux, Switzerland, 23–27 April 2007.
- Hansen MC, Stehman SV, Potapov PV et al. Humid tropical forest clearing from 2000 to 2005 quantified by using multitemporal and multiresolution remotely sensed data. Proc. Natl Acad. Sci. USA105(27),9439–9444 (2008).
- Morton DC, Defries RS, Randerson JT, Giglio L, Schroeder W, van der Werf GR. Agricultural intensification increases deforestation fire activity in Amazonia. Glob. Chang. Biol.14(10),2262–2275 (2008).
- Keeley JE, Zedler PH. Large, high-intensity fire events in southern California shrublands: debunking the fine-grain age patch model. Ecol. Appl.19(1),69–94 (2009).
- Fernandes K, Baethgen W, Bernardes S et al. North Tropical Atlantic influence on western Amazon fire season variability. Geophys. Res. Lett.38,L12701 (2011).
- Chen Y, Velicogna I, Famiglietti JS, Randerson JT. Satellite observations of terrestrial water storage provide early warning information about drought and fire season severity in the Amazon. J. Geophys. Res.Biogeosci.118(2),495–504 (2013).
- Randerson JT, van der Werf GR, Collatz GJ et al. Fire emissions from C-3 and C-4 vegetation and their influence on interannual variability of atmospheric CO2 and delta(CO2)-C-13. Global Biogeochem. Cycles19,GB2019 (2005).
- van der Werf GR, Randerson JT, Giglio L, Gobron N, Dolman AJ. Climate controls on the variability of fires in the tropics and subtropics. Global Biogeochem. Cycles22,GB3028 (2008).
- Swenson S, Wahr J. Post-processing removal of correlated errors in GRACE data. Geophys. Res. Lett.33(8),L08402 (2006).
- Landerer FW, Swenson SC. Accuracy of scaled GRACE terrestrial water storage estimates. Water Resour. Res.48(4),W04531 (2012).
- Rodell M, Velicogna I, Famiglietti JS. Satellite-based estimates of groundwater depletion in India. Nature460(7258),999–1002 (2009).
- Famiglietti JS, Lo M, Ho SL et al. Satellites measure recent rates of groundwater depletion in California’s Central Valley. Geophys. Res. Lett.38(3),L03403 (2011).
- Velicogna I, Tong J, Zhang T, Kimball JS. Increasing subsurface water storage in discontinuous permafrost areas of the Lena River basin, Eurasia, detected from GRACE. Geophys. Res. Lett.39(9),L09403 (2012).
- Scott JH, Burgan RE. Standard Fire Behavior Fuel Models: A Comprehensive Set For Use With Rothermel’s Surface Fire Spread Model. US Department of Agriculture Forest Service, Washington, DC, USA (2005).
- Macedo MN, Defries RS, Morton DC, Stickler CM, Galford GL, Shimabukuro YE. Decoupling of deforestation and soy production in the southern Amazon during the late 2000s. Proc. Natl Acad. Sci. USA109(4),1341–1346 (2012).
- Nepstad D, Soares BS, Merry F et al. The end of deforestation in the Brazilian Amazon. Science326(5958),1350–1351 (2009).
- Loarie SR, Asner GP, Field CB. Boosted carbon emissions from Amazon deforestation. Geophys. Res. Lett.36,L14810 (2009).
- Aragao LEOC, Shimabukuro YE. The incidence of fire in Amazonian forests with implications for REDD. Science328(5983),1275–1278 (2010).
- Schroeder W, Morisette JT, Csiszar I, Giglio L, Morton D, Justice CO. Characterizing vegetation fire dynamics in Brazil through multisatellite data: common trends and practical issues. Earth Interact.9,1–26 (2005).
- Balch JK, Nepstad DC, Brando PM, Alencar A. Comment on “The incidence of fire in Amazonian forests with implications for REDD.” Science330(6011),1627 (2010).
- Morton DC, Defries RS, Nagol J et al. Mapping canopy damage from understory fires in Amazon forests using annual time series of Landsat and MODIS data. Remote Sens. Environ.115(7),1706–1720 (2011).
- Morton DC, Le Page Y, Defries R, Collatz GJ, Hurtt GC. Understorey fire frequency and the fate of burned forests in southern Amazonia. Philos. Trans. R. Soc. B Biol. Sci.368(1619),20120163 (2013).
- Ray D, Nepstad D, Moutinho P. Micrometeorological and canopy controls of fire susceptibility in a forested amazon landscape. Ecol. Appl.15(5),1664–1678 (2005).
- Uhl C, Kauffman JB. Deforestation, fire susceptibility, and potential tree responses to fire in the eastern Amazon. Ecology71(2),437–449 (1990).
- Spracklen DV, Arnold SR, Taylor CM. Observations of increased tropical rainfall preceded by air passage over forests. Nature489(7415),282–285 (2012).
- Malhi Y, Roberts JT, Betts RA, Killeen TJ, Li WH, Nobre CA. Climate change, deforestation, and the fate of the Amazon. Science319(5860),169–172 (2008).
- Prins EM, Menzel WP. Geostationary satellite detection of biomass burning in South America. Int. J. Remote Sens.13(15),2783–2799 (1992).
- Xu W, Wooster MJ, Roberts G, Freeborn P. New GOES imager algorithms for cloud and active fire detection and fire radiative power assessment across North, South and Central America. Remote Sens. Environ.114(9),1876–1895 (2010).
- Freeborn PH, Wooster MJ, Roberts G, Malamud BD, Xu WD. Development of a virtual active fire product for Africa through a synthesis of geostationary and polar orbiting satellite data. Remote Sens. Environ.113(8),1700–1711 (2009).
- Roberts G, Wooster M, Freeborn PH, Xu W. Integration of geostationary FRP and polar-orbiter burned area datasets for an enhanced biomass burning inventory. Remote Sens. Environ.115(8),2047–2061 (2011).
- Mu M, Randerson JT, van der Werf GR et al. Daily and 3-hourly variability in global fire emissions and consequences for atmospheric model predictions of carbon monoxide. J. Geophys. Res.116,D24303 (2011).
- Zhang XY, Kondragunta S, Quayle B. Estimation of biomass burned areas using multiple-satellite-observed active fires. IEEE Trans. Geosci. Remote Sens.49(11),4469–4482 (2011).
- Hurtt GC, Chini LP, Frolking S et al. Harmonization of land-use scenarios for the period 1500–2100: 600 years of global gridded annual land-use transitions, wood harvest, and resulting secondary lands. Clim. Change109(1–2),117–161 (2011).
- Le Page Y, van der Werf GR, Morton DC, Pereira JMC. Modeling fire-driven deforestation potential in Amazonia under current and projected climate conditions. J. Geophys. Res.Biogeosci.115,G03012 (2010).
- Thomson AM, Calvin KV, Chini LP et al. Climate mitigation and the future of tropical landscapes. Proc. Natl Acad. Sci. USA107(46),19633–19638 (2010).
Websites
- Global Fire Data. http://globalfiredata.org
- Program for the Estimation of Deforestation in the Brazilian Amazon. www.obt.inpe.br/prodes
- MODIS Active Fire & Burned Area Products. http://modis-fire.umd.edu/AF_getdata.html
- ATSR World Fire Atlas http://dup.esrin.esa.it/wfa
- Geographic Information Science Center of Excellence, Dakota State University. http://globalmonitoring.sdstate.edu/projects/gfm/humidtropics/data.html
- NASA Goddard Earth Sciences Data and Information Services Center. Mirador. http://mirador.gsfc.nasa.gov
- GRACE Tellus. Jet Propulsion Laboratory, California Institute of Technology. http://grace.jpl.nasa.gov/data