Abstract
Epigenetic alterations during cellular differentiation are a key molecular mechanism which both instructs and reinforces the process of lineage commitment. Within the haematopoietic system, progressive changes in the DNA methylome of haematopoietic stem cells (HSCs) are essential for the effective production of mature blood cells. Inhibition or loss of function of the cellular DNA methylation machinery has been shown to lead to a severe perturbation in blood production and is also an important driver of malignant transformation. HSCs constitute a very rare cell population in the bone marrow, capable of life-long self-renewal and multi-lineage differentiation. The low abundance of HSCs has been a major technological barrier to the global analysis of the CpG methylation status within both HSCs and their immediate progeny, the multipotent progenitors (MPPs). Within this Extra View article, we review the current understanding of how the DNA methylome regulates normal and malignant hematopoiesis. We also discuss the current methodologies that are available for interrogating the DNA methylation status of HSCs and MPPs and describe a new data set that was generated using tagmentation-based whole genome bisulfite sequencing (TWGBS) in order to comprehensively map methylated cytosines using the limited amount of genomic DNA that can be harvested from rare cell populations. Extended analysis of this data set clearly demonstrates the added value of genome-wide sequencing of methylated cytosines and identifies novel important cis-acting regulatory regions that are dynamically remodeled during the first steps of haematopoietic differentiation.
Introduction
Mature blood cells exert highly specific functions in adaptive immunity, innate immune response, wound healing, oxygen supply and other physiological functions that are driven by unique gene expression profiles. Despite this diversity, all blood cells originate from haematopoietic stem cells (HSCs). Once HSCs start to differentiate, they lose self-renewal capacity and develop into multipotent progenitor cells (MPPs), which differentiate further toward lineage-restricted progenitors and subsequently develop into mature blood cells. Murine HSCs are one of the most extensively characterized mammalian adult stem cell populations. Flow cytometry-based protocols allow the prospective isolation of bone marrow HSCs and MPPs based upon correlations between immunophenotype and functional characteristics following transplantation.Citation1-4 MPPs are capable of differentiating into all mature haematopoietic lineages but, in contrast to quiescent HSCs, harbor only restricted self-renewal capacity as reflected by their transient reconstitution capacity following transplantation. Sub-fractions of the MPP compartment demonstrate differences in proliferative status under homeostatic conditions and also show divergent functional behavior upon transplantation.Citation5-7
Gene expression studies have revealed important insights into how biological processes are regulated at the transcriptional level in haematopoietic stem and progenitor cells (HSPC).Citation8-10 However, the global molecular mechanisms acting during physiological HSC commitment remain elusive. In a recent resource study, we investigated the global molecular mechanisms acting during physiological HSC commitment by performing an integrative analysis of proteome, transcriptome and DNA methylome, which enabled a detailed characterization of the regulatory networks active during early HSC commitment.Citation5
In this ‘Extra Views’, we will discuss recent technological developments for methylome profiling and summarize their application to the HSC field. We will extend the analysis of the molecular landscape of haematopoietic stem and multipotent progenitor cells provided in our recent resource article,Citation5 with a focus on early changes in DNA-methylation to gain further insight into the molecular processes involved in the early commitment steps. The data will be integrated with existing published work and placed into the context of epigenetic alterations observed in leukemia.
DNA methylation changes during differentiation
The epigenetic remodeling of both histone modifications and DNA methylation alters chromatin structure and has been recognized as a molecular mechanism regulating differentiation during development and in regenerating adult tissues such as the haematopoietic system.Citation11-13 DNA methylation represents a stable modification of DNA which can either restrict or enforce the differentiation potential of a cell by permanently placing certain genes in a chromatin conformation that is either permissive or restrictive for transcription.Citation11,13 The identification of discrete DNA methylation changes that either gain or lose DNA methylation during HSC differentiation and which are highly enriched in cis-regulatory regions for gene expression demonstrates the power of this approach to discover novel putative gene-regulatory regions.Citation5,14 As shown in mice with targeted deletions of either DNA methyltransferase 1 or 3a (Dnmt1 or Dnmt3a), DNA methylation plays a major role in regulating the production of mature haematopoietic cells from HSC. Mice carrying knockouts of either Dnmt1 or Dnmt3a show perturbed multilineage differentiation and HSC self-renewal capacity, while conditional knock-out of both Dnmt3a and Dnmt3b in HSCs resulted in loss of long-term reconstitution potential.Citation15-18
Epigenetic alterations in hematological malignancies
The importance of epigenetics in hematopoiesis is further highlighted by studies on various hematological malignancies. Multiple studies using single genes, groups of genes or genome-wide profiling technologies have demonstrated massive changes in the promoters of genes resulting in loss of expression.Citation19-23 Early estimates of the amount of CG-rich (or CpG island) promoter methylation determined that 2000 - 3000 genes could be targeted by promoter methylation in acute myeloid leukemiaCitation19 or chronic lymphocytic leukemia.Citation23 Recent genome-wide methylation studies demonstrated that DNA methylation changes not only occur in the promoters of genes but also affect intra- and intergenic regions.Citation24-27 In myeloid malignancies, recent large scale sequencing projects identified recurrent mutations in enzymes involved in the establishment of epigenetic patterns including recurrent mutations in DNMT3A, IDH1/2, or the TET enzymes.Citation28,29 This complements the observation that several recurrent translocations involve rearrangements of epigenetic enzymes, for example, t(9;11) which results in the expression of the MLL-AF9 fusion protein.Citation30, 31 Many of these mutations are associated with disease subgroups carrying distinct methylomes,Citation20,28,32,33 however the underlying molecular mechanisms are currently unknown. Dnmt3a loss of function has been identified as a driver of hematologic malignancy, presumably due to the subsequent loss of epigenome integrity.Citation16,34,35 Indeed, for acute myeloid leukemia it was shown that DNMT3A mutations occur early, possibly in HSCs, and mutant cells represent a pre-leukemic HSC.Citation36 Taken together, the occurrence of epigenetic alterations in hematologic malignancies highlights the importance of tightly regulated epigenetic patterns that govern the cellular differentiation process.
Epigenetic profiling technologies
Methodologies to study the DNA methylome have advanced from technologies interrogating the methylation of single or a few CpG-rich gene promoters,Citation37-39 to modern next-generation sequencing-based approaches interrogating DNA methylation levels at single CpG resolution ().Citation40-42 Restriction landmark genome scanning (RLGS) was the first method to measure quantitatively the methylation status of a few thousand CpG-sites, mostly located in CpG islands, within a single 2-dimensional gel.Citation43,44 RLGS was replaced by array technologies measuring the methylation status of preselected sequences, either CpG-islands or later non-CpG-island promoters, intragenic or intergenic regions.Citation45-50 With the advent of next generation sequencing, whole genome bisulfite sequencing (WGBS) and sequencing of reduced representations of the genome (e.g. reduced representation bisulfite sequencing, RRBS) were introduced to the scientific community for methylome analysis.Citation40-42,51 In parallel, methods employing enrichment of methylated DNA sequences also took advantage of next-generation sequencing read-out (). While these enrichment-based methodologies represent a cost-efficient way to interrogate DNA-methylation in a genome-wide fashion, they have the disadvantage of only indirectly measuring DNA-methylation as a function of relative enrichment levels as compared to a control sample. In contrast, bisulfite sequencing-based methods enable a direct measurement of methylation on the individual DNA molecules. gives a brief overview on the general workflow of the most relevant bisulfite sequencing methods that are currently used. Using RRBS, genome-wide single-CpG resolution analysis of CpG-rich regions like promoters and CpG-islands became possible at relatively low costs. RRBS was also compatible with low-input DNA samples, which enabled the study of methylomes from rare cell populations.Citation14 However, RRBS covers only about 8–10% of all CpGs within the mammalian genome and is biased toward GC-rich sequences,Citation52,53 while the different whole genome bisulfite sequencing approaches cover a nearly complete and rather unbiased representation of CpGs throughout the genome.Citation53 Recent experimental data on a variety of human tissues suggests that only a small proportion of the methylome is actually prone to changes during development, many of which may not be covered by RRBS.Citation54 Therefore, WGBS is still considered to be the gold standard for methylome analysis, but the high sequencing costs prohibit the evaluation of large sample numbers (). Furthermore, a requirement for high levels of starting genomic DNA restricts the use of classical WGBS to cell culture models and tissues where sufficient DNA is available. A breakthrough in the use of WGBS was made with the development of technologies enabling the use of low nanogram to picogram amounts of DNA.Citation55,56 For example, tagmentation-based whole-genome bisulfite sequencing (TWGBS) requires only 5–30 ng of DNA as starting material in order to prepare high-complexity sequencing libraries and is suitable for as few as 10,000 FACS-sorted cells.Citation5,55,57 Very recently it was demonstrated, that WGBS libraries could even be established from single cells (single-cell WGBS, scWGBS).Citation58 Genome-wide, scWGBS covers between 18% and 48% of CpG-sites in individual cells. Single-cell WGBS will enable methylome studies on very rare cell populations as well as to study epigenetic heterogeneity of both normal and tumor tissues. summarizes strengths and weaknesses of bisulfite sequencing-based technologies for DNA methylation analysis. With the discovery of 5-hydroxymethylcytosine (5 hmC), 5-formylcytosine and 5-carboxylcytosine as additional DNA modification, there is now an increasing need for new methods that enable the specific detection of these modifications.Citation59-63 Currently, 5 hmC is of special interest, as it appears to be a stable epigenetic mark rather than just an intermediate in the active DNA demethylation process. As classic bisulphite sequencing cannot distinguish between 5 mC and 5 hmC, several methods specific for one or the other modification have been developed and are based on modification of the 5 hmC followed by enrichment and subsequent sequenicng. Recent methodological advancements for the detection of the new cytosine modifications have been reviewed elsewhere.Citation64
Figure 1. Comparison of sequencing-based methods for genome-wide methylation analysis. (A) Overview of major next-generation sequencing-based methods for DNA methylation screening. Due to their practical relevance, we have also included quantitative array-based methylation screening methods. (B) General workflow for the most relevant bisulfite sequencing based methods RRBS, classic WGBS, PBAT and TWGBS. For methodological detail, please refer to the original reports describing the respective methods.Citation40-42,51,55-57 (C) Table showing advantages and disadvantages for bisulfite sequencing methods.
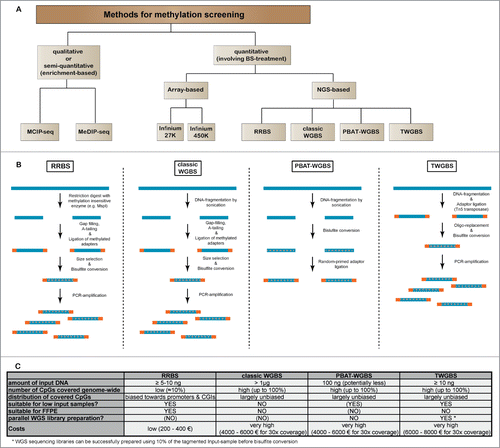
Genome-wide DNA-methylation maps in haematopoietic stem and progenitor cells
Until recently, the DNA methylation patterns of HSC and their dynamics during differentiation had not been characterized. The first study which investigated the DNA methylation patterns of murine HSCs and their progeny used the RRBS technology.Citation14 Numerous differentially methylated regions (more hyper- than hypomethylated regions) could be identified as HSCs differentiated into mature progeny, supporting the importance of DNA methylation during haematopoietic commitment. Key observations from this study included the identification of epigenetic switches that are retained in terminally differentiated cells and correlate with expression of associated genes including transcription factors regulating hematopoiesis (e.g., Sfpi, or Lmo2), cell surface antigens (Cd27, Cd93), or cytokines (Il16). Nevertheless, at the global level, the study by Bock and colleagues did not find a general correlation between DNA methylation changes and alterations in gene-expression.Citation14 This was in line with other recently published data, where the correlation of DNA methylation changes and gene-expression was rather weak.Citation65 Based on these data, the importance of DNA-methylation instructing gene-expression has been questioned.
While RRBS favors the detection of differentially methylated cytosines in GC-rich promoter and CpG-island (CGI) regions, recent studies in healthy tissues employing WGBS have demonstrated that the majority of dynamically methylated CpG-sites are located distal to transcription start sites and CGIs.Citation54,65-67 Jeong and colleagues provided the first whole genome methylation map of murine HSCs (LSK, SP+, CD150+).Citation34 This study identified 1,104 large (>3.5 kbp in length) conserved regions displaying low DNA methylation levels, so called undermethylated regions (UMRs). These UMRs are maintained by Dnmt3a, since HSCs from Dnmt3a knockout mice showed erosion of UMRs. Genes silenced in these regions are under the control of repressive chromatin marks including H3K27me3, whereas active gene regions in UMRs are marked by H3K4me3.Citation34 Together, this study demonstrated the effects of Dnmt3a on the epigenome integrity of murine HSC using an optimized classical WGBS protocol, which enabled the use of 300 ng of input DNA for library preparation.
To date, the most comprehensive molecular dataset on HSCs and their immediate progeny was published by Cabezas-Wallscheid and colleagues.Citation5 In this study, isolated HSCs (LSK, CD34-, CD48-, CD150+), and 3 sub-populations of the multipotent progenitor (MPP) compartment, namely MPP1 (LSK, CD34+, CD48-, CD150+), MPP2 (LSK, CD34+, CD48+, CD150+), and MPP3/4 (LSK, CD34+, CD48+, CD150-) were analyzed by TWGBS. Cabezas-Wallscheid and colleagues provided an integrated view on genome-wide DNA methylation at the single-CpG dinucleotide level, whole-transcriptome including long non-coding RNAs and mass-spectrometry-based proteome data. The work described early molecular changes, occurring during the transition from HSC to MPPs. In total, proteomic analysis of HSCs and MPP1 identified 6,389 proteins, and 56 of them were found to be differentially expressed. On the mRNA-level, 6,487 genes were found to be significantly differentially expressed across the haematopoietic stem and progenitor cell (HSPC) compartment, which included 492 transcription factors. While the global DNA methylation levels were similar in all cell populations, 15,987 discrete differentially methylated regions (DMRs) were identified across the individual HSPC populations. In stark contrast to previous reports, the majority of DMRs occurred in intragenic regions (introns + exons), likely reflecting the fact that this methodology does not skew toward overrepresentation of CpG dense regions of the genome.Citation5,65,68
Results
HSPCs exhibit progressive and cell type-specific changes in DNA methylation patterns
Further refinement of DNA methylation changes by unsupervised clustering revealed 9 clusters that best described the DNA methylation dynamics. The majority of methylation changes were unidirectional, either demonstrating a progressive gain or loss of methylation marks (). Clusters 1 to 4 exhibited a gradual decrease in levels of DNA methylation with differentiation, although the exact kinetics varied. Likewise, clusters 6 through 9 exhibited a gradual gain in methylation with cluster-specific kinetics. In contrast, the behavior of cluster 5 seemed to be unique, in that the DMRs demonstrated a progressive loss in methylation from HSC through MPP2 followed by a subsequent gain in methylation to MPP3/4.
Figure 2. For figure legend, see page 3481.Figure 2 (See previous page). Conserved haematopoietic tissue-specific DNA-methylation. (A) Heatmap of all DMRs (n = 15,987) detected between the 4 HSPC populations (HSC, MPP1, MPP2 and MPP3/4). Each horizontal dash represents the ratio of methylated/unmethylated CpGs in each cell population. R1-R3: biological replicates. Nine distinct DNA methylation clusters were identified by unsupervised cluster analysis and can be grouped into 3 categories: Clusters 1–4 demonstrate a loss of methylation (blue) and clusters 6–9 a gain of methylation (red) from HSC to MPP3/4. Cluster 5 shows a loss of methylation from HSC to MPP2 followed by a gain of methylation to MPP3/4 (purple). (B) Display of mean methylation differences (ΔMeth) ±SD in DMRs of each cluster. ΔMeth is defined as the mean difference in methylation levels in a given subpopulation relative to the mean methylation level of all 4 subpopulations. Blue arrow downwards: loss of methylation; red arrow upwards: gain of methylation; purple arrows: bidirectional methylation change. N indicates the number of DMRs in each cluster. (C) Gene-ontology (GO) analysis of DMRs from clusters 1–9 (seeA) using GREAT with default association rule settings and “whole genome” as background.Citation69 Displayed are the enrichments of the 5 most significantly overrepresented GO-terms (P-value) for each cluster. Colors represent standard scores of log2 P-values. Rows were normalized to have a mean of 0 and a standard deviation of 1. (D) Analysis of DNA methylation changes across different tissues. Relative DNA methylation levels for all DMRs detected during early haematopoietic differentiation are displayed as a heatmap for 24 different cell and tissue types. DNA methylation levels were normalized relative to the mean level of all DMRs within each sample (blue and red indicate DNA methylation lower and higher than the mean methylation level, respectively). Samples analyzed include HSC, MPP1, MPP2 and MPP3/4 (3 replicates each; (R1-R3)), mouse embryonic stem cell data from 3 different experiments (mESC_XT, mESC_E14, and mESC_E14r) and WGBS data for 17 murine adult tissues.Citation66,78 DMRs are sorted as in A according to clusters 1–9.
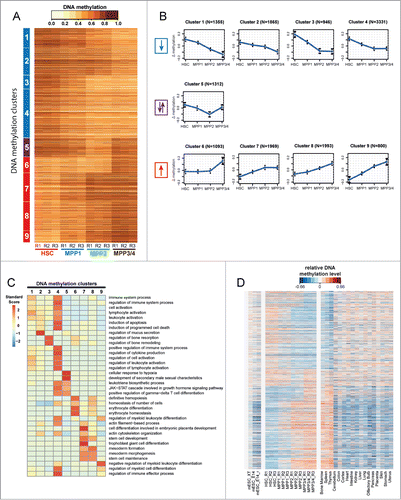
In a further analysis of this data, DMRs were assigned to genes using the “Genomic Regions Enrichment of Annotations Tool” (GREAT), and a gene-ontology (GO) analysis was performed.Citation69 Individual clusters were found enriched for distinct GO-terms that correlated to specific steps of haematopoietic differentiation (e.g. “regulation of myeloid differentiation," “erythrocyte differentiation," “regulation of lymphocyte activation”), suggesting that stage-specific methylation changes in HSPCs regulate sequential steps of haematopoietic commitment ().
In order to determine whether these early methylation changes are maintained in mature terminally differentiated haematopoietic cell types, HSPC-specific DMRs were compared with WGBS data from 17 adult mouse tissues and murine embryonic stem cell.Citation66 This comparison demonstrated that the methylation changes observed in early commitment steps between HSC and their immediate progeny are maintained as a signature in differentiated adult haematopoietic organs (i.e. bone marrow, spleen and thymus; ). Conversely, this signature is neither apparent in other adult tissues nor in murine embryonic stem cell (). This data suggests that the DMRs identified between HSPCs represent haematopoietic -specific methylation events, which remain in a naive status in undifferentiated embryonic stem cells and non-haematopoietic adult tissues.
Differentially methylated regions are enriched in cis-regulatory regions that instruct gene-expression
The DMRs identified frequently overlapped with cis-regulatory elements and it was shown on the global level that methylation changes correlate inversely with gene expression.Citation5 In line with a previous report on in vitro ES-cell differentiation,Citation65 this correlation was even more pronounced when we considered associated gene expression levels of more distant differentiation states: genes associated with DMRs detected between HSC and MPP1 showed more pronounced anti-correlated expression levels in MPP4 as compared to those in MPP1. Thus, this high-resolution methylation data enables us to discern epigenetic changes that precede and predict gene-expression changes at subsequent differentiation levels. To further investigate this observation, and to identify additional genes regulated by DNA methylation, gene-sets were generated based on the DMRs between each of the HSPC populations. More specifically, we annotated all DMRs for each pair-wise comparison to the nearest RefSeq gene. If multiple DMRs mapped to the same gene, only the single DMR closest to a gene's TSS was kept for further analysis. These gene-sets were then used to interrogate RNA-seq data obtained from equivalent cell populations using Gene-Set Enrichment Analysis (GSEA).Citation5,70 In order to filter for consistent direction of gene-expression changes, this analysis was performed under the assumption as if the expression data-sets from all populations upstream or downstream of the observation point were biological replicates. A strong global association between differential methylation and changes in gene-expression was observed. Across all differentiation steps, gene-sets comprised of loci that corresponded to gain of methylation were strongly enriched for transcripts that showed decreased levels of expression during differentiation (ES-score: 1.883 to 2.276, all P-values <0 .0001; ). Likewise, transcripts that were up-regulated during differentiation were enriched by gene-sets consisting of loss of methylation loci (ES-score: −2.326 to −1.706, all P-values <0 .0001; ). In line with this, a unidirectional pattern of gene expression along HSC differentiation was observed when core-enriched genes (also named “leading edge set”) were considered. For example, among the core-enriched genes that become methylated during the HSC to MPP1 transition and which correspondingly show a decrease of gene-expression, there were a number of well-documented mediators of HSC function, such as Gata2, Mecom/Evi1, Prdm11, Prdm16, Mycn, and a number of Hoxa-, and Hoxb-family members (). Additionally, numerous genes which have not been characterized in the context of hematopoiesis and which are likely to play a role in HSPC biology given their progressive pattern of methylation and gene-expression were identified. Indeed, a GeneMANIA analysis of the core-enriched genes identified during the HSC to MPP1 transition (59 genes with methylation gain and down-regulated expression, 205 genes with methylation loss and upregulated expression) demonstrated that the genes associated with these early occurring DMRs are functionally linked ( & Figure S1).Citation71 This again underlines the non-random nature of the DNA methylation changes observed and suggests that changes in DNA methylation status act to coordinately regulate the process of cellular differentiation.
Figure 3. DNA-methylation changes correlate with gene-expression. (A) Left panel: Gene set enrichment analysis (GSEA) of genes associated with DMRs using the GSEA tool (Subramanian et al., 2005). GSEA is provided for DMRs identified between HSC−MPP1, MPP1−MPP2, MPP1−MPP3/4 and split into gain of methylation (red) or loss of methylation (blue) DMRs. NES: normalized enrichment score, FDR: false discovery rate. Right panel: Relative gene expression levels of core enriched set of genes normalized per row (purple: upregulated; green: downregulated). (B) Protein-protein interaction network based on GeneMANIA depicting the interactions of the “core enriched genes” for the HSC−MPP1 transition correlating with gain of methylation and decreased expression. The interaction network of the “core enriched genes” for the HSC−MPP1 transition correlating with loss of methylation and increased expression is depicted in Figure S1.
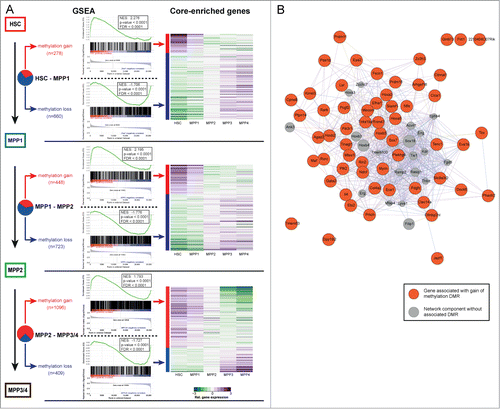
Taken together, this data highlights the global reciprocal relationship between gene-expression and DNA methylation status during haematopoietic differentiation.
Differentiation stage specific haematopoietic transcription factors associate with DMRs
Within the list of differentially methylated and expressed genes, we observed a number of transcription factors (TF) that may comprise master regulators of gene expression. Gene expression data from 2013 TFs were extracted (Panther database, http://www.pantherdb.org/ and were then ranked according to their P-values for altered expression across all HSPC populations ().Citation5,72 In total, 242 out of the 490 (49.4%, p = 2.2 × 10−16, Fisher's exact test) differentially expressed TFs were associated with at least one DMR, which is significantly more than expected by chance. Most of the DMRs are found within a 10 kb window centered on the TSS (). At every commitment step, there was a significant association of DMRs with differentially expressed TFs (). Detailed analysis of regions surrounding the TSS of well-characterized TFs that regulate haematopoietic differentiation identified stage-specific DMRs that overlapped with previously defined cis-acting regulatory regions.Citation5 In addition, specific DMRs associated with TFs such as Mecom (Evi1/Mds1), Pbx1, Jun, Hoxa5, and Gata1 were identified, which potentially define novel cis-regulatory regions for these genes (). In summary, coordinated analysis of TF expression and characterization of DMRs in the proximity of their TSS allows the putative identification of genomic regions that may play an important role in regulating TFs during HSC maintenance and differentiation.
Figure 4. Correlation of DNA methylation and expression of transcription factors. (A) DMR frequency in 10 kb windows around the transcription start site (TSS) of transcription factors (TF) either differentially expressed (brown) or not differentially expressed (beige). The bottom part displays a heatmap of DMRs around TSS of TFs sorted according to P-value for overall differential expression within the HSPC compartment. Red dashes represent DMRs. Asterisks indicate statistical significance (Fisher's exact test; *: P-value 0.01–0.001; **: P-value <0 .001). (B) Left panel: Number of differentially expressed TFs in the transitions from HSC−MPP1, MPP1−MPP2 and MPP1−MPP3/4. Differentially expressed TFs with a DMR annotated are depicted in red, TFs without DMR are depicted in gray. Statistical enrichment of DMRs in differentially expressed TFs was calculated using Chi-squared test. Right panel: Mean expression values based on RNA-seq dataCitation5 of 4 representative differentially expressed TFs associated with DMRs are shown for each commitment step.
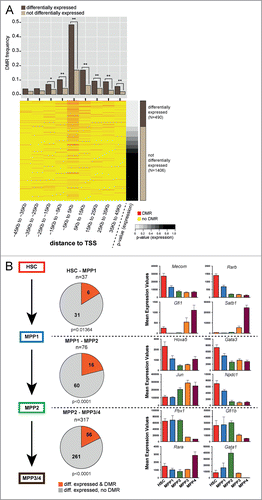
Transcription factor binding motifs are enriched within stage-specific DMRs
Using canonical TF binding motifs (TRANSFAC database), differential motif enrichment across all DMR clusters was calculated. Out of 981 TF motifs interrogated, 45 were found to be significantly enriched, including 29 which have well documented regulatory function within the haematopoietic system (e.g., GATA1, GATA2, RUNX1 (AML1), LMO2, PBX1). Since some of these TF motifs shared a high level of similarity, STAMP motif clustering was used to reduce the 45 enriched motifs into 14 different “motif classes” (). TF motif enrichment was not uniform across the DMR clusters. For example, binding sites for GATA-like TFs all correlated strongly to clusters 5 and 6 and to a lesser extent to clusters 8 and 9. In contrast, binding sites for the AML-like TFs (RUNX-family and core-binding factor) are enriched in clusters 1, 2, 3, and 4. Of interest, cluster 7 showed a distinct pattern of enrichment for p53, PBX1-like, MEF-like and OCT-like binding motifs (). Overall, it appears that TF motif enrichment correlates with DNA methylation dynamics, which indicates that DNA methylation either passively reflects or even actively regulates TF binding patterns during early hematopoiesis. In either case, our data pin-points toward regions occupied by specific TFs and possibly involves these TFs in regulating early haematopoietic commitment. To gain further insight into the interplay between TF motif methylation and TF expression, we have extracted the expression data of a total of 146 TFs that could be associated with the enriched motifs. This analysis identified a number of TFs that show dynamic expression during early HSC commitment among them are Pbx1, Gata1, Gata2, Ap1m1, Mecom, Tcf25, Tcf4, Myo1c, Myo1f, Myo1g, Runx2, and Runx3 some of which may bind to similar sequence motifs (Figure S2). Further studies employing ChIP-seq are needed to establish genome-wide TF binding patterns and the impact of DNA methylation on TF binding.
Figure 5. Motif enrichment in differentially methylated regions associated with transcription factors. (A) Cluster analysis of row Z-scores for TF motif enrichments in DNA methylation clusters. All DMRs were scored using 981 TRANSFAC position weight matrices and motifs showing a significant enrichment (Z > 2) or depletion (Z < −2) in any of the DMR clusters are displayed. Green: motif enrichment, blue: motif depletion. (B) Cluster analysis of Z-scores for pairwise TF-motif co-occurrences in all DMRs (see Material and Methods for details). Green: enrichment of TF-motif co-occurrence, blue: depletion of TF-motif co-occurrence.
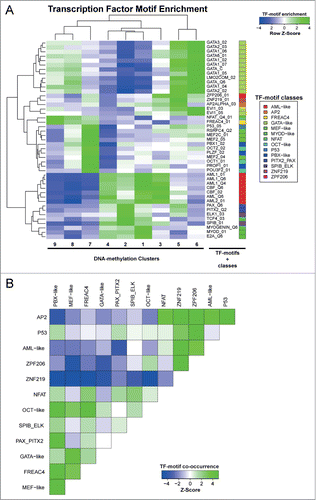
Co-occurrence of TF motifs was tested after merging related motif matrices to more general consensus matrices. Strong co-occurrence was found among TF-motifs for AP2, NFAT, ZNF219, ZPF206, AML-like and p53 within our DMRs, while the same regions are largely depleted for PBX-like, MEF-like, GATA-like, OCT-like, FREAC4, PAX_PITX2, and SPIB_ELK motifs. Conversely, a strong co-occurrence pattern was observed among the latter group of TF motifs. This observation suggests a potential functional relatedness of the TFs recognizing the respective sequence motifs ().
In summary, analysis of HSPC DMRs shows that relevant TF binding sites are methylated in a stage-specific manner and further refines the characterization of cis-acting regulatory regions that likely play an important role in the process of haematopoietic differentiation.
Future perspectives and challenges
Cellular differentiation is regulated by epigenetic mechanisms.Citation73,74 However, the detailed molecular mechanisms as well as the genomic regions under epigenetic control are currently poorly characterized. Recent advances in global epigenetic profiling technologies allow the interrogation of small cell numbers, which is a prerequisite to generate genome-wide high-resolution DNA methylation maps from the early transition steps of rare stem cell populations into more differentiated cell populations.Citation5,55-58
The data set by Cabezas-Wallscheid and colleagues provides a wealth of information for further analysis. While early molecular changes in gene expression and their associated epigenetic alterations have been uncovered, several unanswered questions and/or validation experiments remain. First, very few detailed histone modifications maps exist that could be integrated with the DNA methylation and transcription data. Second, functional validation of altered genes will be necessary to determine the relevance of a particular gene expression change. Finally, the question which molecular mechanisms regulate these dynamic changes in the DNA methylome remains to be elucidated. Two scenarios can be envisioned: either the epigenetic changes seen as DMRs occur secondary to the occupancy by the transcription machinery. Alternatively, one could envision a mechanism that directs epigenetic changes to certain target regions and thereby enabling the occupancy by the transcription machinery. Known examples for such mechanisms are mediated by long non-coding RNAs that act as guide RNAs to direct chromatin repressive or activating complexes to target genes.Citation75-77 Regardless of these uncertainties, it is clear that DNA methylation is an important regulator of haematopoietic differentiation and that sensitive methodologies such as TWGBS allow one to dissect regulatory epigenetic commitment steps that occur during the very early stages of HSC differentiation.
Data Access
Methylation data from murine haematopoietic stem and progenitor cells
Published tagmentation-based whole-genome bisulfite sequencing data from murine haematopoietic stem and progenitor cells can be accessed under Gene Expression Omnibus (GEO; accession: GSE52709).Citation5
Methylation data from murine embryonic stem cells
Published whole-genome bisulfite sequencing data from murine embryonic stem cells were downloaded from GEO (http://www.ncbi.nlm.nih.gov/geo/):Citation78
GEO-acc GEO Sample Name Short Label
GSM1027571: DNA_Methylation_serum_LIF_E14 mESC_E14
GSM1127953: DNA_Methylation_E14_serum_LIF_replica mESC_E14_r
GSM1127955: DNA_Methylation_female_XT67E1_serum_LIF mESC_XT
Methylation data from 17 murine adult tissues
Published whole-genome bisulfite sequencing data for 17 murine adult tissues were downloaded from GEO (http://www.ncbi.nlm.nih.gov/geo/; GEO-accession number: GSE42836).Citation66
RNA-seq data
RNA-seq data from murine haematopoietic stem and progenitor cells can be accessed on ArrayExpress (http://www.ebi.ac.uk/arrayexpress) under accession number E-MTAB-2262.Citation5
Experimental Procedures
Clustering of DMRs
The methylation level of each DMR was estimated based on the unsmoothed methylation data. For each DMR, the methylation levels were normalized by subtracting the median of all sample replicates in the study. The R package kmeans was used to cluster the DMRs based on the normalized methylation levels. The number of clusters was determined as the minimal number of clusters whose sum of within-cluster sum of squares is less than 10% of that of a single cluster.
Motif enrichment analysis
Sequences corresponding to all identified DMRs were scored using 981 TRANSFAC vertebrate matrices (TRANSFAC Version 2011), using a total binding affinity score. For a given motif, all sequences were ranked according to this score. Next, for a given set of sequences from one of the DMR clusters, an enrichment Z-score was computed for this set respective to all other DMR sequences. Finally, motifs showing a significant enrichment (Z > 2) or depletion (Z < −2) in any of the DMR clusters were represented as a heatmap. Given the similarity between some of these motifs, they were clustered using the STAMP method, yielding 14 “motifs classes," which are indicated by a colored bar on the heatmap.
Motif co-occurrences
For each DMR, motif occurrences were determined for all enriched TF-motifs. For each pair of motifs, the number of common DMRs (i.e., DMRs in which both of these motifs are present at least once) was divided by the size of the union (i.e. the number of DMRs in which at least one TF-motif is present). To determine a random distribution, motif occurrences were shuffled among DMRs, and the previous ratio was computed again. This shuffling was done 1000 times to evaluate dispersion. Finally, a Z-score was computed for each pair of motifs, indicating how significantly enriched or depleted a specific pair of motifs is.
Disclosure of Potential Conflicts of Interest
No potential conflicts of interest were disclosed.
Acknowledgments
The authors acknowledge excellent support by the FACS sorting Core Facility as well as by the Genomics and Proteomics Core Facility at the DKFZ. We further thank Jenny Hansson for critical reading of the manuscript.
973334_Supplementary_Materials.zip
Download Zip (410.1 KB)Funding
This work was supported in part by an intramural grant from the German Cancer Research Center (DKFZ) to DBL, MDM and Rainer Claus (Epigenetics@DKFZ). DBL, BB and CP were supported by the DFG Priority Program SP1463 “Epigenetic regulation of normal hematopoiesis and its dysregulation in myeloid neoplasia.” QW was supported by the Humboldt Research Fellowship for Postdoctoral Researchers. DB is supported by the German-Israeli Helmholtz Research School in Cancer Biology. MM, AT and AL were supported by the BioRN Leading-Edge Cluster “Cell-Based and Molecular Medicine” funded by the German Federal Ministry of Education and Research, and the Dietmar Hopp Foundation. AT, LvP, DK and NCW were supported by the Sonderforschungsbereich SFB-873 funded by the Deutsche Forschungsgemeinschaft. AT received further support by the EU FP7 Programs “EuroSyStem” and “Radiant.”
References
- Kiel MJ, Yilmaz OH, Iwashita T, Terhorst C, Morrison SJ. SLAM family receptors distinguish hematopoietic stem and progenitor cells and reveal endothelial niches for stem cells. Cell 2005; 121:1109-21; PMID:15989959; http://dx.doi.org/10.1016/j.cell.2005.05.026.
- Osawa M, Hanada K, Hamada H, Nakauchi H. Long-term lymphohematopoietic reconstitution by a single CD34-low/negative hematopoietic stem cell. Science 1996; 273:242-5; PMID:8662508; http://dx.doi.org/10.1126/science.273.5272.242
- Szilvassy SJ, Weller KP, Chen B, Juttner CA, Tsukamoto A, Hoffman R. Partially differentiated ex vivo expanded cells accelerate hematologic recovery in myeloablated mice transplanted with highly enriched long-term repopulating stem cells. Blood 1996; 88:3642-53; PMID:8896435
- Yamamoto R, Morita Y, Ooehara J, Hamanaka S, Onodera M, Rudolph KL, Ema H, Nakauchi H. Clonal analysis unveils self-renewing lineage-restricted progenitors generated directly from hematopoietic stem cells. Cell 2013; 154:1112-26; PMID:23993099; http://dx.doi.org/10.1016/j.cell.2013.08.007
- Cabezas-Wallscheid NK, Daniel, Hansson Jenny, Lipka, Daniel B, Reyes, Alejandro; Wang Qi, Weichenhan, et al. Identification of Regulatory Networks in HSCs and Their Immediate Progeny via Integrated Proteome, Transcriptome, and DNA Methylome Analysis. Cell stem cell 2014; PMID:25158935
- Wilson A, Laurenti E, Oser G, van der Wath RC, Blanco-Bose W, Jaworski M, Offner S, Dunant CF, Eshkind L, Bockamp E, et al. Hematopoietic Stem Cells Reversibly Switch from Dormancy to Self-Renewal during Homeostasis and Repair. Cell 2008; 135:1118-29; PMID:19062086; http://dx.doi.org/10.1016/j.cell.2008.10.048
- Wilson A, Laurenti E, Trumpp A. Balancing dormant and self-renewing hematopoietic stem cells. Curr Opin Genet Dev 2009; 19:461-8; PMID:19811902; http://dx.doi.org/10.1016/j.gde.2009.08.005
- Gazit R, Garrison Brian S, Rao Tata N, Shay T, Costello J, Ericson J, Kim F, Collins James J, Regev A, Wagers Amy J, et al. Transcriptome Analysis Identifies Regulators of Hematopoietic Stem and Progenitor Cells. Stem Cell Rep 2013; 1:266-80; PMID:24319662; http://dx.doi.org/10.1016/j.stemcr.2013.07.004
- McKinney-Freeman S, Cahan P, Li H, Lacadie SA, Huang HT, Curran M, Loewer S, Naveiras O, Kathrein KL, Konantz M, et al. The transcriptional landscape of hematopoietic stem cell ontogeny. Cell Stem Cell 2012; 11:701-14; PMID:23122293; http://dx.doi.org/10.1016/j.stem.2012.07.018
- Kent DG, Dykstra BJ, Cheyne J, Ma E, Eaves CJ. Steel factor coordinately regulates the molecular signature and biologic function of hematopoietic stem cells. Blood 2008; 112:560-7; PMID:18502833; http://dx.doi.org/10.1182/blood-2007-10-117820
- Bernstein BE, Meissner A, Lander ES. The mammalian epigenome. Cell 2007; 128:669-81; PMID:17320505; http://dx.doi.org/10.1016/j.cell.2007.01.033
- Smith ZD, Meissner A. DNA methylation: roles in mammalian development. Nat Rev Genet 2013; 14:204-20; PMID:23400093; http://dx.doi.org/10.1038/nrg3354
- Waddington CH. The strategy of the genes; a discussion of some aspects of theoretical biology. 1957
- Bock C, Beerman I, Lien WH, Smith ZD, Gu H, Boyle P, Gnirke A, Fuchs E, Rossi DJ, Meissner A. DNA methylation dynamics during in vivo differentiation of blood and skin stem cells. Mol Cell 2012; 47:633-47; PMID:22841485; http://dx.doi.org/10.1016/j.molcel.2012.06.019
- Broske AM, Vockentanz L, Kharazi S, Huska MR, Mancini E, Scheller M, Kuhl C, Enns A, Prinz M, Jaenisch R, et al. DNA methylation protects hematopoietic stem cell multipotency from myeloerythroid restriction. Nat Genet 2009; 41:1207-15; PMID:19801979; http://dx.doi.org/10.1038/ng.463
- Challen GA, Sun D, Jeong M, Luo M, Jelinek J, Berg JS, Bock C, Vasanthakumar A, Gu H, Xi Y, et al. Dnmt3a is essential for hematopoietic stem cell differentiation. Nat Genet 2012; 44:23-31; http://dx.doi.org/10.1038/ng.1009
- Trowbridge JJ, Snow JW, Kim J, Orkin SH. DNA methyltransferase 1 is essential for and uniquely regulates hematopoietic stem and progenitor cells. Cell Stem Cell 2009; 5:442-9; PMID:19796624; http://dx.doi.org/10.1016/j.stem.2009.08.016
- Tadokoro Y, Ema H, Okano M, Li E, Nakauchi H. De novo DNA methyltransferase is essential for self-renewal, but not for differentiation, in hematopoietic stem cells. J Exp Med 2007; 204:715-22; PMID:17420264; http://dx.doi.org/10.1084/jem.20060750
- Costello JF, Fruhwald MC, Smiraglia DJ, Rush LJ, Robertson GP, Gao X, Wright FA, Feramisco JD, Peltomaki P, Lang JC, et al. Aberrant CpG-island methylation has non-random and tumour-type-specific patterns. Nat Genet 2000; 24:132-8; PMID:10655057; http://dx.doi.org/10.1038/72785
- Figueroa ME, Abdel-Wahab O, Lu C, Ward PS, Patel J, Shih A, Li Y, Bhagwat N, Vasanthakumar A, Fernandez HF, et al. Leukemic IDH1 and IDH2 mutations result in a hypermethylation phenotype, disrupt TET2 function, and impair hematopoietic differentiation. Cancer Cell 2010; 18:553-67; PMID:21130701; http://dx.doi.org/10.1016/j.ccr.2010.11.015
- Olk-Batz C, Poetsch AR, Nollke P, Claus R, Zucknick M, Sandrock I, Witte T, Strahm B, Hasle H, Zecca M, et al. Aberrant DNA methylation characterizes juvenile myelomonocytic leukemia with poor outcome. Blood 2011; 117:4871-80; PMID:21406719; http://dx.doi.org/10.1182/blood-2010-08-298968
- Rush LJ, Dai Z, Smiraglia DJ, Gao X, Wright FA, Fruhwald M, Costello JF, Held WA, Yu L, Krahe R, et al. Novel methylation targets in de novo acute myeloid leukemia with prevalence of chromosome 11 loci. Blood 2001; 97:3226-33; PMID:11342453; http://dx.doi.org/10.1182/blood.V97.10.3226
- Rush LJ, Raval A, Funchain P, Johnson AJ, Smith L, Lucas DM, Bembea M, Liu TH, Heerema NA, Rassenti L, et al. Epigenetic profiling in chronic lymphocytic leukemia reveals novel methylation targets. Cancer Res 2004; 64:2424-33; PMID:15059895; http://dx.doi.org/10.1158/0008-5472.CAN-03-2870
- Kulis M, Heath S, Bibikova M, Queiros AC, Navarro A, Clot G, Martinez-Trillos A, Castellano G, Brun-Heath I, Pinyol M, et al. Epigenomic analysis detects widespread gene-body DNA hypomethylation in chronic lymphocytic leukemia. Nat Genet 2012; 44:1236-42; PMID:23064414; http://dx.doi.org/10.1038/ng.2443
- Oakes CC, Claus R, Gu L, Assenov Y, Hullein J, Zucknick M, Bieg M, Brocks D, Bogatyrova O, Schmidt CR, et al. Evolution of DNA methylation is linked to genetic aberrations in chronic lymphocytic leukemia. Cancer Discov 2014; 4:348-61; PMID:24356097; http://dx.doi.org/10.1158/2159-8290.CD-13-0349
- Pei L, Choi JH, Liu J, Lee EJ, McCarthy B, Wilson JM, Speir E, Awan F, Tae H, Arthur G, et al. Genome-wide DNA methylation analysis reveals novel epigenetic changes in chronic lymphocytic leukemia. Epigenetics 2012; 7:567-78; PMID:22534504; http://dx.doi.org/10.4161/epi.20237
- Brocks D, Assenov Y, Minner S, Bogatyrova O, Simon R, Koop C, Oakes C, Zucknick M, Lipka DB, Weischenfeldt J, et al. Intratumor DNA methylation heterogeneity reflects clonal evolution in aggressive prostate cancer. Cell Rep 2014; 8:798-806; PMID:25066126; http://dx.doi.org/10.1016/j.celrep.2014.06.053
- Cancer Genome Atlas Research N. Genomic and epigenomic landscapes of adult de novo acute myeloid leukemia. N Engl J Med 2013; 368:2059-74; PMID:23634996; http://dx.doi.org/10.1056/NEJMoa1301689
- Yoshida K, Sanada M, Shiraishi Y, Nowak D, Nagata Y, Yamamoto R, Sato Y, Sato-Otsubo A, Kon A, Nagasaki M, et al. Frequent pathway mutations of splicing machinery in myelodysplasia. Nature 2011; 478:64-9; PMID:21909114; http://dx.doi.org/10.1038/nature10496
- Bernt KM, Zhu N, Sinha AU, Vempati S, Faber J, Krivtsov AV, Feng Z, Punt N, Daigle A, Bullinger L, et al. MLL-rearranged leukemia is dependent on aberrant H3K79 methylation by DOT1L. Cancer Cell 2011; 20:66-78; PMID:21741597; http://dx.doi.org/10.1016/j.ccr.2011.06.010
- Krivtsov AV, Figueroa ME, Sinha AU, Stubbs MC, Feng Z, Valk PJ, Delwel R, Dohner K, Bullinger L, Kung AL, et al. Cell of origin determines clinically relevant subtypes of MLL-rearranged AML. Leukemia 2013; 27:852-60; PMID:23235717; http://dx.doi.org/10.1038/leu.2012.363
- Figueroa ME, Lugthart S, Li Y, Erpelinck-Verschueren C, Deng X, Christos PJ, Schifano E, Booth J, van Putten W, Skrabanek L, et al. DNA methylation signatures identify biologically distinct subtypes in acute myeloid leukemia. Cancer Cell 2010; 17:13-27; PMID:20060365; http://dx.doi.org/10.1016/j.ccr.2009.11.020
- Figueroa ME, Skrabanek L, Li Y, Jiemjit A, Fandy TE, Paietta E, Fernandez H, Tallman MS, Greally JM, Carraway H, et al. MDS and secondary AML display unique patterns and abundance of aberrant DNA methylation. Blood 2009; 114:3448-58; PMID:19652201; http://dx.doi.org/10.1182/blood-2009-01-200519
- Jeong M, Sun D, Luo M, Huang Y, Challen GA, Rodriguez B, Zhang X, Chavez L, Wang H, Hannah R, et al. Large conserved domains of low DNA methylation maintained by Dnmt3a. Nat Genet 2014; 46:17-23; PMID:24270360; http://dx.doi.org/10.1038/ng.2836
- Ley TJ, Ding L, Walter MJ, McLellan MD, Lamprecht T, Larson DE, Kandoth C, Payton JE, Baty J, Welch J, et al. DNMT3A mutations in acute myeloid leukemia. N Engl J Med 2010; 363:2424-33; PMID:21067377; http://dx.doi.org/10.1056/NEJMoa1005143
- Shlush LI, Zandi S, Mitchell A, Chen WC, Brandwein JM, Gupta V, Kennedy JA, Schimmer AD, Schuh AC, Yee KW, et al. Identification of pre-leukaemic haematopoietic stem cells in acute leukaemia. Nature 2014; 506:328-33; PMID:24522528; http://dx.doi.org/10.1038/nature13038
- Gonzalez-Zulueta M, Bender CM, Yang AS, Nguyen T, Beart RW, Van Tornout JM, Jones PA. Methylation of the 5’ CpG island of the p16/CDKN2 tumor suppressor gene in normal and transformed human tissues correlates with gene silencing. Cancer Res 1995; 55:4531-5; PMID:7553622
- Herman JG, Graff JR, Myohanen S, Nelkin BD, Baylin SB. Methylation-specific PCR: a novel PCR assay for methylation status of CpG islands. Proc Natl Acad Sci U S A 1996; 93:9821-6; PMID:8790415; http://dx.doi.org/10.1073/pnas.93.18.9821
- Herman JG, Merlo A, Mao L, Lapidus RG, Issa JP, Davidson NE, Sidransky D, Baylin SB. Inactivation of the CDKN2/p16/MTS1 gene is frequently associated with aberrant DNA methylation in all common human cancers. Cancer Res 1995; 55:4525-30; PMID:7553621
- Gu H, Bock C, Mikkelsen TS, Jäger N, Smith ZD, Tomazou E, Gnirke A, Lander ES, Meissner A. Genome-scale DNA methylation mapping of clinical samples at single-nucleotide resolution. Nat Methods 2010; 7:133-6; PMID:20062050; http://dx.doi.org/10.1038/nmeth.1414
- Lister R, O’Malley RC, Tonti-Filippini J, Gregory BD, Berry CC, Millar AH, Ecker JR. Highly Integrated Single-Base Resolution Maps of the Epigenome in Arabidopsis. Cell 2008; 133:523-36; PMID:18423832; http://dx.doi.org/10.1016/j.cell.2008.03.029
- Lister R, Pelizzola M, Dowen RH, Hawkins RD, Hon G, Tonti-Filippini J, Nery JR, Lee L, Ye Z, Ngo QM, et al. Human DNA methylomes at base resolution show widespread epigenomic differences. Nature 2009; 462:315-22; PMID:19829295; http://dx.doi.org/10.1038/nature08514
- Kawai J, Hirotsune S, Hirose K, Fushiki S, Watanabe S, Hayashizaki Y. Methylation profiles of genomic DNA of mouse developmental brain detected by restriction landmark genomic scanning (RLGS) method. Nucl Acids Res 1993; 21:5604-8; PMID:8284204; http://dx.doi.org/10.1093/nar/21.24.5604
- Plass C, Shibata H, Kalcheva I, Mullins L, Kotelevtseva N, Mullins J, Kato R, Sasaki H, Hirotsune S, Okazaki Y, et al. Identification of Grf1 on mouse chromosome 9 as an imprinted gene by RLGS-M. Nat Genet 1996; 14:106-9; PMID:8782830; http://dx.doi.org/10.1038/ng0996-106
- Wang Y, Yu Q, Cho AH, Rondeau G, Welsh J, Adamson E, Mercola D, McClelland M. Survey of differentially methylated promoters in prostate cancer cell lines. Neoplasia 2005; 7:748-60; PMID:16207477; http://dx.doi.org/10.1593/neo.05289
- Huang TH, Perry MR, Laux DE. Methylation profiling of CpG islands in human breast cancer cells. Hum Mol Genet 1999; 8:459-70; PMID:9949205; http://dx.doi.org/10.1093/hmg/8.3.459
- Lippman Z, Gendrel AV, Colot V, Martienssen R. Profiling DNA methylation patterns using genomic tiling microarrays. Nat Methods 2005; 2:219-24; PMID:16163803; http://dx.doi.org/10.1038/nmeth0305-219
- Sandoval J, Heyn H, Moran S, Serra-Musach J, Pujana MA, Bibikova M, Esteller M. Validation of a DNA methylation microarray for 450,000 CpG sites in the human genome. Epigenetics 2011; 6:692-702; PMID:21593595; http://dx.doi.org/10.4161/epi.6.6.16196
- Bibikova M, Lin Z, Zhou L, Chudin E, Garcia EW, Wu B, Doucet D, Thomas NJ, Wang Y, Vollmer E, et al. High-throughput DNA methylation profiling using universal bead arrays. Genome Res 2006; 16:383-93; PMID:16449502; http://dx.doi.org/10.1101/gr.4410706
- Teschendorff AE, Menon U, Gentry-Maharaj A, Ramus SJ, Weisenberger DJ, Shen H, Campan M, Noushmehr H, Bell CG, Maxwell AP, et al. Age-dependent DNA methylation of genes that are suppressed in stem cells is a hallmark of cancer. Genome Res 2010; 20:440-6; PMID:20219944; http://dx.doi.org/10.1101/gr.103606.109
- Meissner A, Mikkelsen TS, Gu H, Wernig M, Hanna J, Sivachenko A, Zhang X, Bernstein BE, Nusbaum C, Jaffe DB, et al. Genome-scale DNA methylation maps of pluripotent and differentiated cells. Nature 2008; 454:766-70; PMID:18600261
- Bock C, Tomazou EM, Brinkman AB, Muller F, Simmer F, Gu H, Jager N, Gnirke A, Stunnenberg HG, Meissner A. Quantitative comparison of genome-wide DNA methylation mapping technologies. Nat Biotech 2010; 28:1106-14; http://dx.doi.org/10.1038/nbt.1681
- Gu H, Smith ZD, Bock C, Boyle P, Gnirke A, Meissner A. Preparation of reduced representation bisulfite sequencing libraries for genome-scale DNA methylation profiling. Nat Protocols 2011; 6:468-81; http://dx.doi.org/10.1038/nprot.2010.190
- Ziller MJ, Gu H, Muller F, Donaghey J, Tsai LT, Kohlbacher O, De Jager PL, Rosen ED, Bennett DA, Bernstein BE, et al. Charting a dynamic DNA methylation landscape of the human genome. Nature 2013; 500:477-81; PMID:23925113; http://dx.doi.org/10.1038/nature12433
- Adey A, Shendure J. Ultra-low-input, tagmentation-based whole-genome bisulfite sequencing. Genome Res 2012; 22:1139-43; PMID:22466172; http://dx.doi.org/10.1101/gr.136242.111
- Miura F, Enomoto Y, Dairiki R, Ito T. Amplification-free whole-genome bisulfite sequencing by post-bisulfite adaptor tagging. Nucl Acids Res 2012; 40:e136
- Wang Q, Gu L, Adey A, Radlwimmer B, Wang W, Hovestadt V, Bahr M, Wolf S, Shendure J, Eils R, et al. Tagmentation-based whole-genome bisulfite sequencing. Nat Protoc 2013; 8:2022-32; PMID:24071908; http://dx.doi.org/10.1038/nprot.2013.118
- Smallwood SA, Lee HJ, Angermueller C, Krueger F, Saadeh H, Peat J, Andrews SR, Stegle O, Reik W, Kelsey G. Single-cell genome-wide bisulfite sequencing for assessing epigenetic heterogeneity. Nat Meth 2014; advance online publication
- Kriaucionis S, Heintz N. The nuclear DNA base 5-hydroxymethylcytosine is present in Purkinje neurons and the brain. Science 2009; 324:929-30; PMID:19372393; http://dx.doi.org/10.1126/science.1169786
- Ito S, Shen L, Dai Q, Wu SC, Collins LB, Swenberg JA, He C, Zhang Y. Tet proteins can convert 5-methylcytosine to 5-formylcytosine and 5-carboxylcytosine. Science 2011; 333:1300-3; PMID:21778364; http://dx.doi.org/10.1126/science.1210597
- He YF, Li BZ, Li Z, Liu P, Wang Y, Tang Q, Ding J, Jia Y, Chen Z, Li L, et al. Tet-mediated formation of 5-carboxylcytosine and its excision by TDG in mammalian DNA. Science 2011; 333:1303-7; PMID:21817016; http://dx.doi.org/10.1126/science.1210944
- Pfaffeneder T, Hackner B, Truss M, Munzel M, Muller M, Deiml CA, Hagemeier C, Carell T. The discovery of 5-formylcytosine in embryonic stem cell DNA. Angewandte Chemie 2011; 50:7008-12; PMID:21721093; http://dx.doi.org/10.1002/anie.201103899
- Tahiliani M, Koh KP, Shen Y, Pastor WA, Bandukwala H, Brudno Y, Agarwal S, Iyer LM, Liu DR, Aravind L, et al. Conversion of 5-methylcytosine to 5-hydroxymethylcytosine in mammalian DNA by MLL partner TET1. Science 2009; 324:930-5; PMID:19372391; http://dx.doi.org/10.1126/science.1170116
- Song C-X, Yi C, He C. Mapping recently identified nucleotide variants in the genome and transcriptome. Nat Biotech 2012; 30:1107-16; http://dx.doi.org/10.1038/nbt.2398
- Gifford CA, Ziller MJ, Gu H, Trapnell C, Donaghey J, Tsankov A, Shalek AK, Kelley DR, Shishkin AA, Issner R, et al. Transcriptional and epigenetic dynamics during specification of human embryonic stem cells. Cell 2013; 153:1149-63; PMID:23664763; http://dx.doi.org/10.1016/j.cell.2013.04.037
- Hon GC, Rajagopal N, Shen Y, McCleary DF, Yue F, Dang MD, Ren B. Epigenetic memory at embryonic enhancers identified in DNA methylation maps from adult mouse tissues. Nat Genet 2013; 45:1198-206; PMID:23995138; http://dx.doi.org/10.1038/ng.2746
- Xie W, Schultz MD, Lister R, Hou Z, Rajagopal N, Ray P, Whitaker JW, Tian S, Hawkins RD, Leung D, et al. Epigenomic analysis of multilineage differentiation of human embryonic stem cells. Cell 2013; 153:1134-48; PMID:23664764; http://dx.doi.org/10.1016/j.cell.2013.04.022
- Stadler MB, Murr R, Burger L, Ivanek R, Lienert F, Scholer A, van Nimwegen E, Wirbelauer C, Oakeley EJ, Gaidatzis D, et al. DNA-binding factors shape the mouse methylome at distal regulatory regions. Nature 2011; 480:490-5; PMID:22170606
- McLean CY, Bristor D, Hiller M, Clarke SL, Schaar BT, Lowe CB, Wenger AM, Bejerano G. GREAT improves functional interpretation of cis-regulatory regions. Nat Biotechnol 2010; 28:495-501; PMID:20436461; http://dx.doi.org/10.1038/nbt.1630
- Subramanian A, Tamayo P, Mootha VK, Mukherjee S, Ebert BL, Gillette MA, Paulovich A, Pomeroy SL, Golub TR, Lander ES, et al. Gene set enrichment analysis: a knowledge-based approach for interpreting genome-wide expression profiles. Proc Natl Acad Sci U S A 2005; 102:15545-50; PMID:16199517; http://dx.doi.org/10.1073/pnas.0506580102
- Warde-Farley D, Donaldson SL, Comes O, Zuberi K, Badrawi R, Chao P, Franz M, Grouios C, Kazi F, Lopes CT, et al. The GeneMANIA prediction server: biological network integration for gene prioritization and predicting gene function. Nucl Acids Res 2010; 38:W214-20; PMID:20576703; http://dx.doi.org/10.1093/nar/gkq537
- Mi H, Muruganujan A, Thomas PD. PANTHER in 2013: modeling the evolution of gene function, and other gene attributes, in the context of phylogenetic trees. Nucl Acids Res 2013; 41:D377-86; PMID:23193289; http://dx.doi.org/10.1093/nar/gks1118
- Cedar H, Bergman Y. Epigenetics of haematopoietic cell development. Nat Rev Immunol 2011; 11:478-88; PMID:21660052; http://dx.doi.org/10.1038/nri2991
- Reik W, Dean W, Walter J. Epigenetic reprogramming in mammalian development. Science 2001; 293:1089-93; PMID:11498579; http://dx.doi.org/10.1126/science.1063443
- Guttman M, Rinn JL. Modular regulatory principles of large non-coding RNAs. Nature 2012; 482:339-46; PMID:22337053; http://dx.doi.org/10.1038/nature10887
- Rinn JL. lncRNAs: Linking RNA to Chromatin. Cold Spring Harb Perspect Biol 2014; 6; PMID:25085913; http://dx.doi.org/10.1101/cshperspect.a018614
- Arab K, Park YJ, Lindroth AM, Schafer A, Oakes C, Weichenhan D, Lukanova A, Lundin E, Risch A, Meister M, et al. Long Noncoding RNA TARID Directs Demethylation and Activation of the Tumor Suppressor TCF21 via GADD45A. Mol Cell 2014; PMID:25087872
- Habibi E, Brinkman Arie B, Arand J, Kroeze Leonie I, Kerstens Hindrik HD, Matarese F, Lepikhov K, Gut M, Brun-Heath I, Hubner Nina C, et al. Whole-genome bisulfite sequencing of two distinct interconvertible DNA methylomes of mouse embryonic stem cells. Cell Stem Cell 2013; 13:360-9; PMID:23850244; http://dx.doi.org/10.1016/j.stem.2013.06.002