Abstract
Regular endurance exercise training induces beneficial functional and health effects in human skeletal muscle. The putative contribution to the training response of the epigenome as a mediator between genes and environment has not been clarified. Here we investigated the contribution of DNA methylation and associated transcriptomic changes in a well-controlled human intervention study. Training effects were mirrored by significant alterations in DNA methylation and gene expression in regions with a homogeneous muscle energetics and remodeling ontology. Moreover, a signature of DNA methylation and gene expression separated the samples based on training and gender. Differential DNA methylation was predominantly observed in enhancers, gene bodies and intergenic regions and less in CpG islands or promoters. We identified transcriptional regulator binding motifs of MRF, MEF2 and ETS proteins in the proximity of the changing sites. A transcriptional network analysis revealed modules harboring distinct ontologies and, interestingly, the overall direction of the changes of methylation within each module was inversely correlated to expression changes. In conclusion, we show that highly consistent and associated modifications in methylation and expression, concordant with observed health-enhancing phenotypic adaptations, are induced by a physiological stimulus.
Abbreviations
β-HAD | = | 3-hydroxyacyl-CoA dehydrogenase |
CpG | = | cytosine-guanine dinucleotide |
CS | = | citrate synthase |
DEG | = | differentially expressed gene |
DMP | = | differentially methylated position |
FDR | = | false discovery rate |
LUMA | = | luminometric methylation assay |
MDS | = | multidimensional scaling |
MEF | = | myocyte enhancer factor |
MRF | = | myogenic regulatory factor |
PCA | = | principal component analysis |
Introduction
Endurance exercise training is a strong physiological stimulus that leads to a multitude of health and functional improvements when performed regularly. The health benefits include prevention and/or treatment of a multitude of the most common diseases, e.g., cardiovascular disease, type II diabetes, and several forms of cancer.Citation1 The health benefits following exercise training are elicited by gene expression changes in skeletal muscle, which are fundamental to the remodeling process.Citation2 Epigenetic modifications through DNA methylation regulate gene transcription.Citation3 DNA methylation has traditionally been considered to be a mitotically stable modification that could change only over long periods of time, e.g., in disease development Citation4-6 and during the general aging process.Citation7,8 However, there is increasing evidence that more short-term environmental factors can influence DNA methylation. For example, dietary factors have the potency to alter the degree of DNA methylation in different tissues, Citation9,10 including skeletal muscle,Citation11 while exercise is less well studied. In one study, a single bout of endurance-type exercise was shown to affect methylation at a few promoter CpG sites.Citation12 In the context of diabetes, exercise training has been shown to affect genome-wide methylation pattern in skeletal muscle,Citation13 as well as in adipose tissue.Citation14 Together, those data indicate that physiological stressors can indeed affect DNA methylation.
Adaptation in skeletal muscle depends on successive transient increases in mRNA encoding regulatory, metabolic, and structural proteins.Citation15 However, the acute changes in gene expression are quite different from the more robust basal alterations that characterize a well-adapted muscle after a major lifestyle change, e.g., months of regular exercise.
In order to obtain a deeper understanding of the mechanisms underlying the massive functional and health benefits of regular exercise, we conducted a 3-month fully supervised human one-legged exercise training study. Training only one leg allowed for an intraindividual control, thereby excluding potential influence of diet, seasonal changes or unknown environmental factors, which are expected to affect both legs equally. We observed that the training intervention reshapes the epigenome and induces significant changes in DNA methylation, and the global fractions of decreased and increased methylation sites were similar. Importantly, changes in DNA methylation were enriched in regulatory enhancer regions. Functional categories related to muscle biology (e.g., regulation of cellular carbohydrate metabolism and structural remodeling) were overrepresented among differentially methylated sites. In addition, a coordinated transcriptional and epigenetic response was identified through network analysis.
Together, the findings from this tightly controlled human study strongly suggest that the regulation and maintenance of exercise training adaptation is to a large degree associated to epigenetic changes, especially in regulatory enhancer regions.
Results
Endurance exercise training induces global alterations in DNA methylation
Twenty-three young volunteers (), not regularly performing intense exercise, performed supervised one-legged knee-extension exercise training for 3 months (45 min, 4 sessions per week; Figure S1A), training only one randomized leg (trained leg), while the second leg (untrained leg) was used as an intraindividual control leg. Skeletal muscle biopsies from the vastus lateralis were taken from both legs at rest, before and after the training period (see Supplementary Methods S9 for details). Performance improvements and enzyme activity increases in the trained leg confirmed that the training response was highly significant (P < 10−4, and Figure S1B-C).
Table 1. Baseline subject characteristics for males (n = 12), females (n = 11) and all subjects (n = 23). Data is presented as mean ± SEM. For details on the training program see Figure S1.
Figure 1. Effects of endurance exercise training are mirrored by alterations in DNA methylation and gene expression. The significant (* = P < 10−4) effect of training in the trained leg is shown by the increase in a 15 min performance test (A) and the citrate synthase activity in muscle biopsies (B) in T2 (after training) vs. T1 (before training). For comparison, the untrained leg is also shown, where a smaller change is observed in performance and no change in CS activity. # indicates significant differences between the changes in the 2 legs. Additional physiological measurements are shown in Figure S1B-C. The physiological changes are mirrored by modifications in DNA methylation (C) and gene expression (D). For DNA methylation, the effect size is measured as the difference in M-values and points in black correspond to DMPs with FDR < 0.05. For gene expression, the log2 (Fold Change) is plotted against the average log2(Counts Per Million) and red points correspond to genes with FDR <0.05. Correlation between changes in DNA methylation and CS activity exists and results are shown in Figure S5. The clustering of the samples is shown in (E) using either DNA methylation (upper panels) or gene expression (lower panels). A segment connects to measurements from the same subject obtained before and after training. Samples are alternatively colored by group (T1 = blue, T2 = red) or by gender (M = green, F = magenta). For DNA methylation, Principal Component Analysis was employed using only autosomal DMPs, while for gene expression the top 1000 genes with largest biological variation were chosen and the biological coefficient of variation used to produce a multidimensional scaling plot.
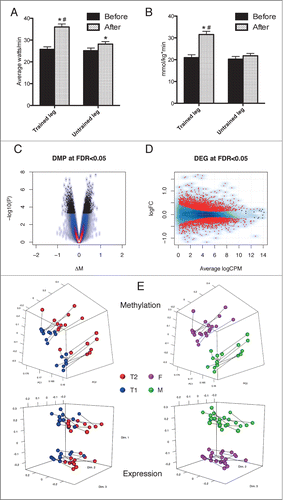
To address the effect of training on DNA methylation of specific sites across the human skeletal muscle genome we used the Illumina Infinium HumanMethylation450 arrays. Endurance training [after training (T2) vs. before training (T1)] induced significant (false discovery rate, FDR< 0.05) methylation changes at 4919 sites across the genome in the trained leg (, Dataset S13). Of these, 839 sites had an absolute change of at least 5% in their mean methylation level (β-value) after training, with a maximum of 9%. The corresponding transcriptional analysis was performed using RNA sequencing, which identified 4076 differentially expressed genes (DEGs; , Data set S13). Ontology and pathway analysis of DEGs showed that the transcriptional response robustly reflected key pathways involved in training adaptation and a trained muscle phenotype (Figure S2-S3 and Table S10). Clustering analysis identified training and gender as the major determinants of variability on autosomal DNA methylation and gene expression data (). The clustering was independent of gene expression on sex chromosomes, as a corresponding grouping was obtained excluding genes on X and Y chromosomes (data not shown). Moreover, a complementary approach revealed that over 600 CpG sites correlated to the increase in citrate synthase activity, an objective measure of training response (Figure S4 and Dataset S14). This might imply that some of these sites could influence the degree of training response.
Global cytosine methylation was also analyzed to look for globally unidirectional changes by luminometric methylation assay (LUMA),Citation16 revealing no change in overall methylation levels with training, and no difference between the trained and the untrained leg (Figure S5A). Global CpG methylation was remarkably similar both within the same subject and between all subjects. This is consistent with the specific observations from the array data of approximately equal number of sites increasing and decreasing in methylation and no significant change in the sample average methylation after training. Analysis of global hydroxymethylation levels resulted in no overall change with training (Figure S5B).
As expected by a physiological environmental trigger on adult tissue, the observed effect size on DNA methylation was small in comparison to disease states such as cancer.Citation9 Hence, to exclude possible technical artifacts leading to false positive associations, we selected several genomic positions for bisulfite pyrosequencing validation. Overall, the methylation levels significantly correlated with the 450K array data (Figure S5C). Out of 7 selected sites, we observed the same direction of change for 6 positions (up/down/no change), thus providing support on the reliability of our data. The untrained leg was also included in the validation to verify that the observed changes were indeed an effect of the training itself and not due to other environmental effects. No significant change was detected in the untrained leg. For details, see Figure S5D-J and the corresponding text. Moreover a Q-Q plot for the array results is showed in Figure S8.
Enrichment of training-induced DMPs in enhancers
Annotation of the differentially methylated positions (DMPs) revealed a preferential localization outside of CpG Islands/Shelves/Shores. When considering the positioning of the DMPs with respect to the genes, we could also detect an enrichment of the relative fraction of DMPs in gene bodies and intergenic regions and fewer DMPs in promoter regions (TSS200), as compared to the relative distribution of the probes present in the array (). Given these observations, we asked whether the major source of the DMPs could originate from enhancers. Indeed, using the standard array annotation, DMPs were significantly enriched in enhancers (). This finding was further corroborated using external sources of data and annotations (see Supplementary Methods S9). For example, when defining enhancers based on histone marks (either H3K4me1, H3K27ac, or H3K4me1 and H3K27ac jointly) and using completely independent data from human skeletal muscle tissue, we also confirmed that the changing sites were observed predominantly in enhancers (). Additional evidence came from the definition of chromatin states by a chromatin segmentation algorithm and the corresponding public data available for human myoblast cultures.Citation17 Here we observed an enrichment, relative to the array background, for strong and weak enhancers, but not for promoters (Fig. 2D). We also observed a slight preferential enrichment in regions of accessible chromatin, as defined by DNase Hypersensitive Sites (Figure S6). These observations have potential implications on the role of DNA methylation on regulating gene expression, a phenomenon likely mediated by altering the methylation status of enhancers and other regulatory elements.Citation18-20
Figure 2. DNA methylation changes are primarily localized in enhancers. (A–C) For each annotation category, the relative fraction of positions located within each feature type is calculated for DMPs (red bars), non-DMPs (blue bars) and the entire position on the array (green bar). Panels A-B were obtained using Illumina annotation, while for panel C we used Illumina annotation (Enhancers) or publicly available ChIP-seq experiments on HSMM cells from which we derived H3K4me1 and H3K27ac peaks. The presence of H3K27ac mark denotes an active regulatory element, while H3K4me1+H3K27ac marks active enhancers and other distal elements. (D) The log2 fold enrichment for DMPs vs. the array was calculated for the relative fraction of probes falling in each category; data from chromatin segmentation algorithms in HSMM cells were used. For additional information, see Supplementary Methods S9. Significance codes: *P < 0.05; ***P < 0.001; Fisher's exact test.
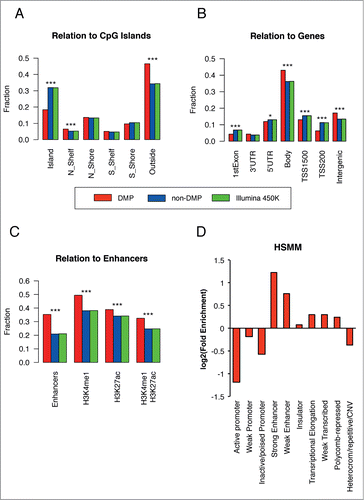
The finding that endurance training especially influences enhancers is indubitably novel in the context of tissue adaptation to a physiological stimulus in humans. Therefore, we sought to identify the biological processes corresponding to these regulatory regions.
Differential methylation is related to genes governing muscle related processes
To assess the putative physiological relevance of the DMPs, we performed a functional annotation and ontology enrichment analysis, where we defined an association rule that considered gene regulatory domains (see Supplementary Methods S9). Indeed, we detected a clear enrichment of muscle ontology related processes for the genes in the vicinity of DMPs, demonstrating that the top enriched categories of molecular function, biological process and cellular component were linked to myogenesis as well as muscle structure, function and bioenergetics, in concordance with a trained muscle phenotype (). This prompted to a deeper characterization of DMPs, by extending bidirectionally the sequence of the position (±100 bp) and examining the enrichment of known transcriptional motifs. Concordantly, we observed a significant enrichment for binding motifs of the MRF (Myogenic Regulatory Factors), and MEF2 (Myocyte Enhancer Factor 2) classes in DMPs increasing in methylation after training ( and Table S11). Conversely, for DMPs that decreased their methylation level, an enrichment for the ETS family binding domain was observed ( and Table S11). The ETS transcription factors have been strongly implicated for example in angiogenesis,Citation21 a key process in endurance exercise adaptation.
Figure 3 (See previous page). Muscle related processes and factors are enriched in the up-methylated DMPs. GREAT analysis was performed to retrieved functional categories associated with DMPs increasing or decreasing methylation after training. Up to top 5 categories passing the threshold (see Supplementary Methods S9) are shown for GO Molecular Function, Biological Process and Cellular Component. We tested the presence of known enriched motif on a symmetrical 200 bp window around each DMP (P < 10−10, consensus motif shown). Known profiles were clustered and a familial logo was drawn. For a corresponding ontology analysis of gene expression, see Figures S2-S3.
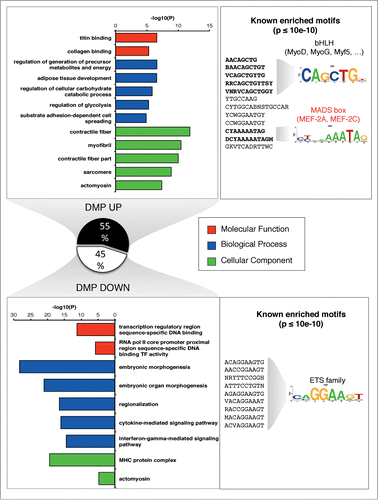
Specific differential methylation correlates with expression changes in an exercise-dependent manner
The finding that differential DNA methylation is observed at enhancers and regulatory regions with the associated enrichment of muscle ontology processes suggests a functional link between DNA methylation and RNA expression. To integrate those 2 data types, we initially performed correlation analysis, in order to highlight pairs of genes/methylation positions that showed dependence after training. Overall, when all probe/position pairs were considered, the Spearman correlation was centered on zero (), revealing no sign of global trend. However, selection of the pairs resulting from a DMP and a DEG revealed that an effect of exercise training exists in specific genomic regions, indicated by peaks of both positive and negative correlation () that were preferentially observed according to the relation to gene regions.Citation3 In particular, negative correlation was more prominent for probes in promoter/5′UTR/1st exon regions, while gene bodies had a stronger peak of positive correlation ().
Figure 4. Identification of regions exhibiting positive or negative correlations between methylation and expression. (A) Distribution of Spearman correlation between DNA methylation and gene expression calculated either including all pairs of genes/methylation positions (gray line) or only pairs formed by a DMP and a DEG (black line). The 2 distributions are strongly different (P < 2.2e-16 Kolmogorov-Smirnov Test) and peaks of positive and negative correlation are highlighted after selecting changing sites after training. (B) The distribution of the Spearman correlation was stratified according to the genomic location of the DMP with respect to the linked gene. (C) A Q-Q plot was obtained by plotting the observed P-values from the above correlation analysis against those obtained under a uniform distribution. (D) A starburst plot illustrates the relationship between DNA methylation and expression changes, with a color density representation of the scatterplot of the pairs of genes/methylation positions. The horizontal axis is the FDR for each gene multiplied by the sign of the fold change, while the vertical axis is the FDR for methylation multiplied by the sign of the fold change. Green dots correspond to pairs where FDR<0.05. Numbers on each quadrant show the pairs (black) or genes (red) that fall in each region. Examples of expression/methylation pairs are given for the indicated probes and genes. Points represent samples and are colored as shown on the corresponding legends (training: T1 = black, T2 = red; gender: M = blue, F = red). The correlation for the cases taken from the significant quadrants is visually explained by the training, while the correlation obtained for non-significant cases may be explained because of the individual levels of methylation and gene expression being correlated at the subject level.
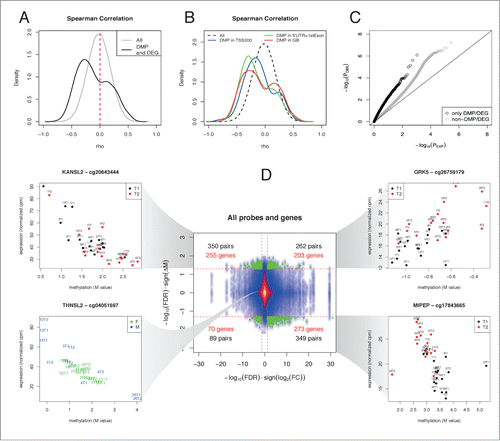
In principle, since we are analyzing longitudinal observations on the same subject before and after training, any detected pattern of correlation between expression and methylation could arise from the individual levels of methylation and gene expression being correlated at the subject level and not as a result of the experimental intervention. Indeed, a Q-Q plot () revealed that, after excluding DMP/DEG pairs, some residual correlation remained and this behavior is most likely explained by the presence of some baseline level of correlation between DNA methylation and gene expression, that is not explained by training, but instead by inter-individual factors (e.g., gender). However, when considering only the DMP/DEG pairs, we reduced the number of false positives or, in other words, we decreased the possibility that the dependence is not explained by the endurance training. This phenomenon is further exemplified by a starburst plot that illustrates the relationship between DNA methylation and expression changes (). This integrative analysis identified 255 downregulated genes with significant increase in methylation, 203 upregulated genes with significant increase in methylation, 273 upregulated genes with significant decrease in methylation, and 70 downregulated genes with significant decrease in methylation. Those gene/position pairs correspond to interesting examples of changes shaped by the training-specific intervention and, therefore, provide the fraction of genes whose expression-methylation correlation is changed in different directions. Individual examples selected among the top-correlated genes are given in . We found several interesting genes in the context of muscle physiology and metabolism. Examples include the MIPEP gene that encodes for a mitochondrial peptidase, primarily involved in the maturation of proteins involved in oxidative phosphorylation,Citation22 and GRK5, a GPCR kinase involved in multiple biological processes, proposed as a positive regulator of insulin sensitivity in mouse.Citation23 In turn, some of the highly correlated gene/methylation probes were not differentially regulated after training, including many HLA genes and other genes whose expression could possibly be influenced by individual genotypesCitation24 and not by the experimental intervention. This is exemplified by THNSL2 (in the bottom left panel of ), whose bimodal expression has been linked to a cis-eQTL in muscle tissue.Citation25 For more examples, see Table S12.
Integrating transcriptional network analysis with DNA methylation identifies a coordinated training response
Having established that DNA methylation alterations are induced by training and are linked to corresponding genes that are also regulated by exercise training, we asked whether we could visualize epigenomic changes superimposed on a reconstructed transcriptional network. Hence, we reverse-engineered a mutual information-based transcriptional network (p < 10−10) using count data from DEGs alone and, in a second independent step, we overlaid the DNA methylation data by summarizing the methylation changes of the linked DMPs (). This analysis uncovered 3 major network domains and some smaller modules (Figures S7) that were grouped according to their relative fold change (T2 vs. T1) that corresponded to distinct ontologies. Domain A was overall downregulated (average log2FC = −0.31) and was populated with genes annotated for regulation of gene expression, DNA replication and cell cycle. Examples include MDM2, a E3 ubiquitin-protein ligase that mediates ubiquitination of p53, ZNF638, a transcription factor associated to PPARG expression,Citation26 UACA involved in regulation of stress-induced apoptosis Citation27 and CUL3, part of an E3 ubiquitin-protein ligase complex and previously shown to decrease in expression with endurance training.Citation28 At the other extreme, domain C contained upregulated genes (average log2FC = 0.60) involved in morphological changes, including cell adhesion, blood vessel development and extracellular matrix organization. This domain included genes that have been shown to increase in expression with training, Citation28 such as several collagens (e.g., COL4A1 and COL4A2), the protease inhibitor A2M, the adhesive glycoprotein gene THBS4, and PDGFRB involved in blood vessel formation. Domain B contained a majority of upregulated genes, with an intermediate magnitude as compared to domain C (average log2FC = 0.25). This domain was clearly associated with cellular energetics, mainly oxidative phosphorylation. Many mitochondrial enzymes were found in this group, including CS, SDHA, HADHA, COX7A2, ATP5B and several NADH dehydrogenases.
Figure 5. Transcriptional network analysis reveals coordinated alternations in methylation and gene expression. A transcriptional network was reconstructed using RNA expression data, showing 3 major domains (see Supplementary Methods S9 and Figure S7). The whole network is shown inside the rectangle at the bottom of the figure, where each node is colored according to the corresponding fold change. The 3 major domains (A, B, C) are zoomed and only genes with corresponding significant changes in DNA methylation are labeled. The color indicates whether the gene has corresponding DMPs that significantly increase (red) or decrease (blue) their methylation levels. A dark gray color indicates that the gene has significant but discordant changes in DNA methylation.
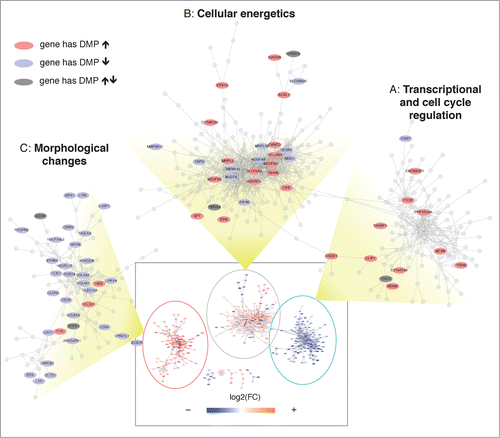
Importantly, the independent integration of DNA methylation information on top of the transcriptional network identified a consistent pattern of inverse changes, possibly reflecting a coordinate transcriptional plan ( and Data set S13). In fact, the upregulated domain C was associated with a significant decrease in DNA methylation, whereas the downregulated domain A had almost entirely corresponding positive changes in DNA methylation. Concordantly, domain B contained a comparable fraction of genes with corresponding positive or negative changes in DNA methylation. Interesting genes whose methylation changes were opposite to those of transcription included the collagens COL4A1 and COL4A2, and laminin LAMA4 that form the basement membrane around skeletal muscle cells. For oxidative metabolism, the enzymes MDH1 and a NADH dehydrogenase of the electron transport chain NDUFA8 represent interesting examples. In domain A, the myosin phosphatase PPP1R12A and TRDN, likely involved in regulation of calcium release from the sarcoplasmic reticulum, both increase in methylation and decrease in expression.
Discussion
From this well-controlled, prospective and extensive human study we were able to create a map of coherent, consistent and biologically relevant DNA methylation changes in skeletal muscle tissue in response to a lifestyle intervention known to improve function and health. The significant changes in DNA methylation, that primarily occurred in enhancer regions, were to a large extent associated with relevant changes in gene expression, even if no causal relationship could be definitely determined.
The main findings of this study were that 3 months of endurance training in healthy human volunteers induced significant methylation changes at almost 5000 sites across the genome and significant differential expression of approximately 4000 genes. The genes associated with the DMPs that increased and decreased in methylation, respectively, with training, represent distinct ontologies. DMPs that increased in methylation were mainly associated to structural remodeling of the muscle and glucose metabolism, while the DMPs with decreased methylation were associated to inflammatory/immunological processes and transcriptional regulation. This suggests that the changes in methylation seen with training were not a random effect across the genome but rather a controlled process that likely contributes to skeletal muscle adaptation to endurance training.
CpG methylation is subjected to spatial (tissue- or cell-specific methylation) or temporal variability (age-dependent, disease-associated, or environmental-mediated differential methylation). In order to eliminate confounding factors, we formulated a paired study design, where 2 samples from the same individual were contrasted before and after the training intervention. We verified, for selected sites, that the observed effect, despite small, was specific for the trained leg, corroborating the hypothesis that the measured changes in DNA methylation represent explicit molecular manifestations of exercise training. Unlike certain diseases, e.g., cancer, environmentally induced changes in DNA methylation are typically small, and susceptible to large individual variation.Citation9,29 By employing an intraindividual control leg for validation of methylation and physiological changes, we minimize any influence of diet or other environmental factors that may otherwise be a challenge in environmental epigenomics.Citation29,30 Here we successfully report the identification of significant and ontologically coherent changes in DNA methylation in a human genome-wide study of the effect of endurance exercise in muscle tissue, which was made possible by the combination of a well-controlled, prospective study design with a comprehensive, integrative bioinformatic analysis.
A well-known source of heterogeneity in DNA methylation studies is represented by the cell composition of the analyzed sample. The epigenome varies between cell types and correcting for cellular composition has been shown to reduce confounding due to cell type bias in blood.Citation24,31 Nevertheless, for complex solid tissue samples, the correction for cell composition is not straightforward, as it would require the isolations and profiling of individual cell types. An increased vascularization following endurance exercise results in a higher endothelial cell content per skeletal muscle fiber.Citation32 In line with this, we confirmed that some exclusive and non-exclusive endothelial markers were differentially expressed after training (data not shown). Hence, we cannot completely rule out the possibility that a minor fraction of the observed changes in DNA methylation and/or gene expression could reflect changes in cellular fractions.
Correlation of the changes in DNA methylation to the changes in gene expression showed that the majority of significant methylation/expression pairs were found in the groups representing either increases in expression with a concomitant decrease in methylation or vice versa. The fraction of genes showing both significant decrease in methylation and upregulation was 7.5% of the DEGs or 2.3% of all genes detected in muscle tissue with at least one measured DNA methylation position. Correspondingly, 7.0% of the DEGs or 2.1% of all genes showed both significant increase in methylation and downregulation. This could reflect the classical view on promoter methylation with a reciprocal relationship between methylation and expression. The pairs showing a concordant change were skewed in the positive direction. In fact, 5.6% of the DEGs or 1.7% of all genes were significantly changing in the positive direction, whereas only 1.9% of the DEGs or 0.6% of all genes had significantly negative changes. In total, we show that DNA methylation changes are associated to gene expression changes in roughly 20% of unique genes that significantly changed with training.
One previous study has investigated genome-wide alterations in DNA methylation after long-term training using MeDIP-ChIP and related those to gene expression changes by microarrays.Citation13 Various methods are now available for determining CpG methylation status, each potentially exhibiting advantages and limitations and differing in the ability to detect differential methylation.Citation29,Citation33-35. We employed a method (450K arrays) that quantifies methylation levels at specific loci and does not require correction for CpG bias,Citation35 coupled to RNA-seq, in order to uncover the connection between the epigenetic and transcriptional responses, and therefore obtain a deeper understanding of the impact of regular exercise. The methodological differences render a direct comparison more difficult, however, both studies show reduced methylation of some genes involved in muscle physiology, for example RUNX1 and COL4A1. In contrast, for the gene THADA also highlighted in Nitert et al.Citation13 we observe an increased methylation of 2 CpG sites. Our sites are, however, located in the gene body, while changes in Nitert et al. occur in promoter regions, something that likely produces different effects on transcription.
The network reconstruction resulted in domains classified mainly as structural, metabolic and regulatory and suggested a coordinated pattern of change in DNA methylation and gene expression. Examples of structural genes include COL4A1, COL4A2 and LAMA4. These genes have also been identified as important for differences in responsiveness to endurance training,Citation36 where methylation status could be part of the mechanism behind variable training response. Among the metabolic genes, MDH1 catalyzes the reversible oxidation of malate to oxaloacetate, utilizing the NAD/NADH cofactor system in the citric acid cycle and NDUFA8 plays an important role in transferring electrons from NADH to the respiratory chain. Regulatory genes downregulated with training include MDM2, targeting p53 for proteasomal degradation, and PPP1R12A, a subunit of myosin phosphatase that is also capable of inhibiting HIF1AN-dependent suppression of HIF1A activity. The resulting inhibition of HIF1A could be an advantage for endurance adaptation as HIF1A has a negative effect on oxidative metabolism.Citation37,38
Recently it was shown that enhancers have dynamic methylation and that their methylation levels are more closely associated with gene expression alterations than promoter methylation in cancer.Citation19,20,39 In the present study, methylation predominantly changed in enhancer regions with enrichment for binding motifs for different transcription factors suggesting that enhancer methylation may be highly relevant also in exercise biology. Regions with sites increasing in methylation were enriched for myogenic regulatory factors or MRFs and myocyte enhancer factors, or MEFs. The MRFs include Myf5, MyoD, myogenin, and Mrf4. These are basic helix-loop-helix transcription factors of the myogenic lineage that control the determination and differentiation of skeletal muscle cells in the embryoCitation40. Of special interest in the biology of endurance training may be that MRFs, through binding to the PGC-1α core promoter, can regulate this well-studied co-factor for mitochondrial biogenesis.Citation41 Animal studies indicate that MyoD promotes slow-to-fast fiber transformation while myogenin could possibly work as an ultimate transcription factor binding directly to promoters of mitochondrial enzymes, thereby promoting a more oxidative profile of muscle tissue.
The myocyte enhancer factor 2 (MEF2) transcription factor links extracellular signals (partly through regulation of class IIa histone deacetylases, HDACs) to the activation of genetic programs involved in cell differentiation, proliferation, morphogenesis, survival and apoptosis in many different cell types.Citation42 KO experiments suggest that some of the MEF2 isoforms promote slow fiber phenotype during development.Citation40
That endurance training led to an increased methylation in enhancer regions containing motifs for the MRFs and MEFs is somewhat counterintuitive since it should lead to the repression of the action of the above discussed transcription factors. Possible explanations include either: a) a dynamic regulation of enhancer accessibility and activity, involving active methylation as an inactivation mechanism at the time (i.e., 3 months) when morphological changes have already taken place and a negative feedback should be provided; b) a confounding effect due to increased representation of different cell types after training, which would result in the detection of a change in methylation because of the underlying differences in methylomes between muscle and non muscle cell types and c) possible incompletely understood phenomena such as the differential methylation that inhibits transcription of an inhibitory RNA molecule, as it was shown for p15.Citation43 However, these factors regulate a large number of genes and there are several that decrease with training in this study, including CDCH15, MYH3, TNNT2, RYR1 and SH3GLB1, which would be expected from an increased enhancer methylation. Also, the expression of MEF2A itself decreased with training.
Regions with sites decreasing in methylation with endurance training were enriched for the ETS family of transcription factors, a large group with a well-conserved binding motif. A reduction in methylation with training in enhancer regions containing this motif potentially allows for further activation of their response genes. There are several members of this group that are known to regulate exercise-responsive genes, thus proposing an epigenetic regulation with endurance training. GABPA, also known as nuclear respiratory factor 2, controls expression of several genes involved in mitochondrial respiration in human skeletal muscleCitation44 and is itself regulated by the transcriptional coactivator PGC1α.Citation45 Another interesting example is ETS1, that targets genes important for endothelial migration e.g., matrix metalloproteinases, angiopoietin 2 Citation46 and the vascular endothelial growth factor receptor 2 (KDR),Citation21 which increase in expression in this study. ELF1 targets TIE1 and TIE2,Citation21 also important factors in angiogenesis known to increase with endurance training in human skeletal muscle.Citation47 The ETS family of transcription factors have been shown to interact with RUNX1Citation48 which has been identified as one potential key transcription factor in the regulation of the endurance training induced transcriptome.Citation36 The mechanisms responsible for inducing these training specific effects are yet to be described. However, altered metabolism is known to induce epigenetic changes and metabolites from the citric acid cycle such as α-ketoglutarate are used as substrates for several of the enzymes known to catalyze methylation reactions.Citation49
In conclusion, this study demonstrates that the transcriptional alterations in skeletal muscle in response to a long-term endurance exercise intervention are coupled to DNA methylation changes. We suggest that the training-induced coordinated epigenetic reprogramming mainly targets enhancer regions, thus contributing to differences in individual response to lifestyle interventions. We provide a valuable and novel perspective on the fields of human physiology and environmental epigenomics, showing that a physiological health-enhancing stimulus can induce highly consistent modifications in DNA methylation that are associated to gene expression changes concordant with observed phenotypic adaptations.
Materials and Methods
Human physiological measurements
The study, labeled EpiTrain (“Epigenetics in Training”), was approved by the Ethics Committee of Karolinska Institutet and conformed to the Declaration of Helsinki. Twenty-three young, sedentary volunteers () trained only one randomized leg during 3 months, and the other leg was used as an untrained intraindividual control leg. Two one-legged knee-extension performance tests were conducted before and after the training period. Skeletal muscle biopsies from vastus lateralis were taken before and 24h after the last training session from both legs. The post-training performance tests were conducted 3–6 d after the biopsies. Enzyme activity assays included citrate synthase (CS) and β-HAD activity. For details, see Supplementary Methods S9.
DNA methylation methods
The total amount of DNA methylation (at CCGG sites) in the genome, before and after training in both legs, was analyzed with LUMA (LUminometric Methylation Assay).Citation16 Genome-wide DNA methylation profiling was generated with the Infinium HumanMethylation450 BeadChip array on bisulfite-treated DNACitation50 from biopsies collected before (T1) and after training (T2, trained leg), for 17 subjects in total. Bisulfite pyrosequencing was adopted for technical validation. We analyzed global hydroxymethylation with a colorimetric antibody-based method from Epigentek according to the manufacturer's specifications. More details are given in Supplementary Methods S9.
Bioinformatics analysis of DNA methylation data
We employed a pre-processing and normalization pipeline as reported previously.Citation51. Color-bias adjustment, quantile normalization, probe type bias adjustment Citation52 and batch correction were performed. For further details see Supplementary Methods S9 and Marabita et al.,Citation51 where the current dataset was named “Data set B.” Differentially methylated positions (DMPs) were defined using limma on M values,Citation53 including the group (T2 vs. T1) and the subject as covariates, in order to account for the paired design. DMPs were selected if FDR < 0.05. For sample clustering, principal component analysis was performed using only data from the DMPs. Standard Illumina annotation was used to annotate methylation data. Additional sources included NIH Roadmap Epigenomic (http://www.roadmapepigenomics.org) data for skeletal muscle and ChromHMM chromatin segmentation tracks for HSMM cell line in Encode (http://encodeproject.org). For each annotation category the relative fraction of positions located within each feature type was calculated for DMPs, non-DMPs and the entire array. GREAT (http://great.stanford.edu) was used to discover functional categories associated with DMPs. The enrichment for known transcriptional motifs was tested with HOMER (http://homer.salk.edu/homer/) and motifs were clustered using STAMP (http://www.benoslab.pitt.edu/stamp/index.php). Full details are given in Supplementary Methods S9.
RNA sequencing
Total RNA was used to prepare libraries, which were sequenced as paired-end, 2 × 100 bp on an Illumina HIseq2000 and generated an average of 21 million paired-end reads per sample.
Briefly, we performed quality control, trimming, mapping, PCR duplicate removal and gene count summarization. EdgeR Citation54 was used to normalize the data and extract differentially expressed genes (DEGs). We included the group (T2 vs. T1), the library preparation and the individual as covariates. DEG were selected if FDR<0.05. Multidimensional scaling plots were used for sample clustering. Gene ontology analysis was done taking length bias into account, as implemented in goseq,Citation55 and enriched KEGG pathways were visualized with pathview.Citation56 Full details are given in Supplementary Methods S9. The baseline muscle transcriptome has been described elsewhere,Citation57 where a subset of the samples before training (n = 12) has been analyzed.
Integration between DNA methylation and transcriptomics
A list of genes and the corresponding measured DNA methylation positions was obtained and correlation was calculated for each pair. The distribution of Spearman rho statistics between DNA methylation and gene expression was calculated either including all pairs of genes/methylation positions or only pairs formed by a DMP and a DEG. Transcriptional networks were reconstructed from gene expression data applying the Mutual Information (MI) method developed in ARACNE.Citation58 The resulting network components were visualized and analyzed with Cytoscape. For each independent network domain, gene ontology was tested using the BiNGO tool (http://www.psb.ugent.be/cbd/papers/BiNGO/Home.html). For additional information see Supplementary Methods S9.
Data availability
Data are available on GEO under the accession numbers GSE60655 (DNA methylation), GSE58608 and GSE60590 (gene expression).
Disclosure of Potential Conflicts of Interest
No potential conflicts of interest were disclosed.
Author Contributions
The study was designed and conducted by M.E.L. and C.J.S. H.R. and T.J.E. participated in design and conceptual discussions. F.M., D.G.C. designed and performed bioinformatic analysis of gene expression, DNA methylation and their integration, network reconstruction and analysis. D.G.C. and J.T. supervised the bioinformatic analysis and gave conceptual advices. M.E.L. performed molecular, physiological and statistical analyses. M.E.L. and F.M. drafted the manuscript. All authors participated in interpretation of data, manuscript preparation and have read and approved the final manuscript.
982445_Supplementary_Materials.zip
Download Zip (8.4 MB)Acknowledgments
We thank our Karolinska Institutet co-workers Eva-karin Gidlund for assistance with physiological testing and biopsy handling, Charlotte Schönbeck for assistance with enzymatic measurements and bisulfite validation and Helene Fischer for help with primer design for bisulfite pyrosequencing. We thank Science for Life Laboratory (SciLifeLab Stockholm), National Genomics Infrastructure and Uppmax for providing massive parallel sequencing and computational infrastructure.
Funding
This work was supported by the Swedish National Center for Research in Sports, Torsten Söderberg Foundation, STATegra, Stockholm County Council and Swedish Research Council. Malene Lindholm has a PhD training grant from Karolinska Institutet. The funders had no role in study design, data collection and analysis, decision to publish, or preparation of the manuscript.
Supplemental Material
Supplemental data for this article can be accessed on the publisher's website.
References
- Matheson GO, Klügl M, Engebretsen L, Bendiksen F, Blair SN, Börjesson M, Budgett R, Derman W, Erdener U, Ioannidis JPA, et al. Prevention and management of non-communicable disease: the IOC consensus statement, Lausanne 2013. Sports Med 2013; 43:1075-88; PMID:24129783; http://dx.doi.org/10.1007/s40279-013-0104-3
- Coffey VG, Hawley JA. The Molecular Bases of Training Adaptation. Sports Med 2007; 37:737-63; PMID:17722947; http://dx.doi.org/10.2165/00007256-200737090-00001
- Jones PA. Functions of DNA methylation: islands, start sites, gene bodies and beyond. Nat Rev Genet 2012; 13:484-92; PMID:22641018; http://dx.doi.org/10.1038/nrg3230
- Heyn H, Esteller M. DNA methylation profiling in the clinic: applications and challenges. Nat Rev Genet 2012; 13:679-92; PMID:22945394; http://dx.doi.org/10.1038/nrg3270
- Jones PA, Baylin SB. The epigenomics of cancer. Cell 2007; 128:683-92; PMID:17320506; http://dx.doi.org/10.1016/j.cell.2007.01.029
- Feinberg AP. Epigenetics at the epicenter of modern medicine. JAMA 2008; 299:1345-50; PMID:18349095; http://dx.doi.org/10.1001/jama.299.11.1345
- Teschendorff AE, West J, Beck S. Age-associated epigenetic drift: implications, and a case of epigenetic thrift? Human Molecular Genetics 2013; 22:R7-R15; PMID:23918660; http://dx.doi.org/10.1093/hmg/ddt375
- Bjornsson HT, Sigurdsson MI, Fallin MD, Irizarry RA, Aspelund T, Cui H, Yu W, Rongione MA, Ekström TJ, Harris TB, et al. Intra-individual change over time in DNA methylation with familial clustering. JAMA 2008; 299:2877-83; PMID:18577732; http://dx.doi.org/10.1001/jama.299.24.2877
- Feil R, Fraga MF. Epigenetics and the environment: emerging patterns and implications. Nat Rev Genet 2011; 13:97-109.
- Lim U, Song M-A. Dietary and Lifestyle Factors of DNA Methylation. In: Methods in Molecular Biology. Totowa, NJ: Humana Press; 2012. pages 359-76. Available from: .
- Jacobsen SC, Brøns C, Bork-Jensen J, Ribel-Madsen R, Yang B, Lara E, Hall E, Calvanese V, Nilsson E, Jørgensen SW, et al. Effects of short-term high-fat overfeeding on genome-wide DNA methylation in the skeletal muscle of healthy young men. Diabetologia 2012; 55:3341-9; PMID:22961225; http://dx.doi.org/10.1007/s00125-012-2717-8
- Barrès R, Yan J, Egan B, Treebak JT, Rasmussen M, Fritz T, Caidahl K, Krook A, O’Gorman DJ, Zierath JR. Acute Exercise Remodels Promoter Methylation in Human Skeletal Muscle. Cell Metab 2012; 15:405-11; http://dx.doi.org/10.1016/j.cmet.2012.01.001
- Nitert MD, Dayeh T, Volkov P, Elgzyri T, Hall E, Nilsson E, Yang BT, Lang S, Parikh H, Wessman Y, et al. Impact of an exercise intervention on DNA methylation in skeletal muscle from first-degree relatives of patients with type 2 diabetes. Diabetes 2012; 61:3322-32; PMID:23028138; http://dx.doi.org/10.2337/db11-1653
- Rönn T, Volkov P, Davegårdh C, Dayeh T, Hall E, Olsson AH, Nilsson E, Tornberg Å, Dekker Nitert M, Eriksson K-F, et al. A Six Months Exercise Intervention Influences the Genome-wide DNA Methylation Pattern in Human Adipose Tissue. PLoS Genet 2013; 9:e1003572.
- Williams RS, Neufer PD. Regulation of gene expression in skeletal muscle by contractile activity. In: Rowell LB, Shepherd JT, editors. Exercise: regulation and integration of multiple systems. Published for the American Physiological Society by Oxford University Press; 1996. pages 1124-50.
- Karimi M, Johansson S, Stach D, Corcoran M, Grandér D, Schalling M, Bakalkin G, Lyko F, Larsson C, Ekström TJ. LUMA (LUminometric Methylation Assay)-a high throughput method to the analysis of genomic DNA methylation. Exp Cell Res 2006; 312:1989-95; PMID:16624287; http://dx.doi.org/10.1016/j.yexcr.2006.03.006
- Ernst J, Kheradpour P, Mikkelsen TS, Shoresh N, Ward LD, Epstein CB, Zhang X, Wang L, Issner R, Coyne M, et al. Mapping and analysis of chromatin state dynamics in nine human cell types. Nature 2011; 473:43-9; PMID:21441907; http://dx.doi.org/10.1038/nature09906
- Wiench M, John S, Baek S, Johnson TA, Sung M-H, Escobar T, Simmons CA, Pearce KH, Biddie SC, Sabo PJ, et al. DNA methylation status predicts cell type-specific enhancer activity. EMBO J 2011; 30:3028-39; PMID:21701563; http://dx.doi.org/10.1038/emboj.2011.210
- Aran D, Sabato S, Hellman A. DNA methylation of distal regulatory sites characterizes dysregulation of cancer genes. Genome Biol 2013; 14:R21; PMID:23497655; http://dx.doi.org/10.1186/gb-2013-14-3-r21
- Stadler MB, Murr R, Burger L, Ivanek R, Lienert F, Schöler A, van Nimwegen E, Wirbelauer C, Oakeley EJ, Gaidatzis D, et al. DNA-binding factors shape the mouse methylome at distal regulatory regions. Nature 2011; 480:490-5; PMID:22170606
- Randi AM, Sperone A, Dryden NH, Birdsey GM. Regulation of angiogenesis by ETS transcription factors. Biochem Soc Trans 2009; 37:1248-53; PMID:19909256; http://dx.doi.org/10.1042/BST0371248
- Chew A, Buck EA, Peretz S, Sirugo G, Rinaldo P, Isaya G. Cloning, expression, and chromosomal assignment of the human mitochondrial intermediate peptidase gene (MIPEP). Genomics 1997; 40:493-6; PMID:9073519; http://dx.doi.org/10.1006/geno.1996.4586
- Wang L, Shen M, Wang F, Ma L. GRK5 ablation contributes to insulin resistance. Biochem Biophys Res Commun 2012; 429:99-104; PMID:23111327; http://dx.doi.org/10.1016/j.bbrc.2012.10.077
- Liu Y, Aryee MJ, Padyukov L, Fallin MD, Hesselberg E, Runarsson A, Reinius L, Acevedo N, Taub M, Ronninger M, et al. Epigenome-wide association data implicate DNA methylation as an intermediary of genetic risk in rheumatoid arthritis. Nat Biotechnol 2013; 31:142-7; PMID:23334450; http://dx.doi.org/10.1038/nbt.2487
- Mason CC, Hanson RL, Ossowski V, Bian L, Baier LJ, Krakoff J, Bogardus C. Bimodal distribution of RNA expression levels in human skeletal muscle tissue. BMC Genomics 2011; 12:98; PMID:21299892; http://dx.doi.org/10.1186/1471-2164-12-98
- Meruvu S, Hugendubler L, Mueller E. Regulation of Adipocyte Differentiation by the Zinc Finger Protein ZNF638. J Biol Chem 2011; 286:26516-23; PMID:21602272; http://dx.doi.org/10.1074/jbc.M110.212506
- Sakai T, Liu L, Teng X, Mukai-Sakai R, Shimada H, Kaji R, Mitani T, Matsumoto M, Toida K, Ishimura K, et al. Nucling recruits Apaf-1/pro-caspase-9 complex for the induction of stress-induced apoptosis. J Biol Chem 2004; 279:41131-40; PMID:15271982; http://dx.doi.org/10.1074/jbc.M402902200
- Timmons JA, Knudsen S, Rankinen T, Koch LG, Sarzynski M, Jensen T, Keller P, Scheele C, Vollaard NBJ, Nielsen S, et al. Using molecular classification to predict gains in maximal aerobic capacity following endurance exercise training in humans. J Appl Physiol 2010; 108:1487-96; PMID:20133430; http://dx.doi.org/10.1152/japplphysiol.01295.2009
- Rakyan VK, Down TA, Balding DJ, Beck S. Epigenome-wide association studies for common human diseases. Nat Rev Genet 2011; 12:529-41; PMID:21747404; http://dx.doi.org/10.1038/nrg3000
- Michels KB. Epigenetic epidemiology. Springer; 2012.
- Houseman EA, Accomando WP, Koestler DC, Christensen BC, Marsit CJ, Nelson HH, Wiencke JK, Kelsey KT. DNA methylation arrays as surrogate measures of cell mixture distribution. BMC Bioinformatics 2012; 13:86; PMID:22568884; http://dx.doi.org/10.1186/1471-2105-13-86
- Gustafsson T. Vascular remodelling in human skeletal muscle. Biochem Soc Trans 2011; 39:1628-32; PMID:22103498; http://dx.doi.org/10.1042/BST20110720
- Harris RA, Wang T, Coarfa C, Nagarajan RP, Hong C, Downey SL, Johnson BE, Fouse SD, Delaney A, Zhao Y, et al. Comparison of sequencing-based methods to profile DNA methylation and identification of monoallelic epigenetic modifications. Nat Biotechnol 2010; 28:1097-105; PMID:20852635; http://dx.doi.org/10.1038/nbt.1682
- Robinson MD, Stirzaker C, Statham AL, Coolen MW, Song JZ, Nair SS, Strbenac D, Speed TP, Clark SJ. Evaluation of affinity-based genome-wide DNA methylation data: effects of CpG density, amplification bias, and copy number variation. Genome Research 2010; 20:1719-29; PMID:21045081; http://dx.doi.org/10.1101/gr.110601.110
- Bock C, Tomazou EM, Brinkman AB, Müller F, Simmer F, Gu H, Jäger N, Gnirke A, Stunnenberg HG, Meissner A. Quantitative comparison of genome-wide DNA methylation mapping technologies. Nat Biotechnol 2010; 28:1106-14; PMID:20852634; http://dx.doi.org/10.1038/nbt.1681
- Keller P, Vollaard NBJ, Gustafsson T, Gallagher IJ, Sundberg CJ, Rankinen T, Britton SL, Bouchard C, Koch LG, Timmons JA. A transcriptional map of the impact of endurance exercise training on skeletal muscle phenotype. J Appl Physiol 2011; 110:46-59; PMID:20930125; http://dx.doi.org/10.1152/japplphysiol.00634.2010
- Papandreou I, Cairns RA, Fontana L, Lim AL, Denko NC. HIF-1 mediates adaptation to hypoxia by actively downregulating mitochondrial oxygen consumption. Cell Metab 2006; 3:187-97; PMID:16517406; http://dx.doi.org/10.1016/j.cmet.2006.01.012
- Lindholm ME, Fischer H, Poellinger L, Johnson RS, Gustafsson T, Sundberg CJ, Rundqvist H. Negative regulation of HIF in skeletal muscle of elite endurance athletes: a tentative mechanism promoting oxidative metabolism. Am J Physiol Regul Integr Comp Physiol 2014; 307:R248-55; PMID:24898836; http://dx.doi.org/10.1152/ajpregu.00036.2013
- Heintzman ND, Hon GC, Hawkins RD, Kheradpour P, Stark A, Harp LF, Ye Z, Lee LK, Stuart RK, Ching CW, et al. Histone modifications at human enhancers reflect global cell-type-specific gene expression. Nature 2009; 459:108-12; PMID:19295514; http://dx.doi.org/10.1038/nature07829
- Gundersen K. Excitation-transcription coupling in skeletal muscle: the molecular pathways of exercise. Biol Rev Camb Philos Soc 2011; 86:564-600; PMID:21040371; http://dx.doi.org/10.1111/j.1469-185X.2010.00161.x
- Amat R, Planavila A, Chen SL, Iglesias R, Giralt M, Villarroya F. SIRT1 controls the transcription of the peroxisome proliferator-activated receptor-gamma Co-activator-1alpha (PGC-1alpha) gene in skeletal muscle through the PGC-1alpha autoregulatory loop and interaction with MyoD. J Biol Chem 2009; 284:21872-80; PMID:19553684; http://dx.doi.org/10.1074/jbc.M109.022749
- Potthoff MJ, Olson EN. MEF2: a central regulator of diverse developmental programs. Development 2007; 134:4131-40; PMID:17959722; http://dx.doi.org/10.1242/dev.008367
- Yu W, Gius D, Onyango P, Muldoon-Jacobs K, Karp J, Feinberg AP, Cui H. Epigenetic silencing of tumour suppressor gene p15 by its antisense RNA. Nature 2008; 451:202-6; PMID:18185590; http://dx.doi.org/10.1038/nature06468
- Fredriksson K, Tjäder I, Keller P, Petrovic N, Ahlman B, Scheele C, Wernerman J, Timmons JA, Rooyackers O. Dysregulation of mitochondrial dynamics and the muscle transcriptome in ICU patients suffering from sepsis induced multiple organ failure. PLoS ONE 2008; 3:e3686; PMID:18997871; http://dx.doi.org/10.1371/journal.pone.0003686
- Mootha VK, Handschin C, Arlow D, Xie X, St Pierre J, Sihag S, Yang W, Altshuler D, Puigserver P, Patterson N, et al. Erralpha and Gabpa/b specify PGC-1alpha-dependent oxidative phosphorylation gene expression that is altered in diabetic muscle. Proc Natl Acad Sci USA 2004; 101:6570-5; PMID:15100410; http://dx.doi.org/10.1073/pnas.0401401101
- Oettgen P. Regulation of vascular inflammation and remodeling by ETS factors. Circul Res 2006; 99:1159-66; PMID:17122446; http://dx.doi.org/10.1161/01.RES.0000251056.85990.db
- Timmons JA, Sundberg CJ. Oligonucleotide microarray expression profiling: human skeletal muscle phenotype and aerobic exercise training. IUBMB Life 2006; 58:15-24; PMID:16540428; http://dx.doi.org/10.1080/15216540500507390
- Hollenhorst PC, Shah AA, Hopkins C, Graves BJ. Genome-wide analyses reveal properties of redundant and specific promoter occupancy within the ETS gene family. Genes Dev 2007; 21:1882-94; PMID:17652178; http://dx.doi.org/10.1101/gad.1561707
- Lu C, Thompson CB. Metabolic Regulation of Epigenetics. Cell Metab 2012; 16:9-17; PMID:22768835; http://dx.doi.org/10.1016/j.cmet.2012.06.001
- Bibikova M, Barnes B, Tsan C, Ho V, Klotzle B, Le JM, Delano D, Zhang L, Schroth GP, Gunderson KL, et al. High density DNA methylation array with single CpG site resolution. Genomics 2011; 98:288-95; PMID:21839163; http://dx.doi.org/10.1016/j.ygeno.2011.07.007
- Marabita F, Almgren M, Lindholm ME, Ruhrmann S, Fagerström-Billai F, Jagodic M, Sundberg CJ, Ekström TJ, Teschendorff AE, TegnEr J, et al. An evaluation of analysis pipelines for DNA methylation profiling using the Illumina HumanMethylation450 BeadChip platform. Epigenetics 2013; 8:333-46; PMID:23422812; http://dx.doi.org/10.4161/epi.24008
- Teschendorff AE, Marabita F, Lechner M, Bartlett T, TegnEr J, Gomez-Cabrero D, Beck S. A beta-mixture quantile normalization method for correcting probe design bias in Illumina Infinium 450 k DNA methylation data. Bioinformatics (Oxford, England) 2013; 29:189-96; PMID:23175756; http://dx.doi.org/10.1093/bioinformatics/bts680
- Smyth GK. Linear Models and Empirical Bayes Methods for Assessing Differential Expression in Microarray Experiments. Stat Appl Genet Mol Biol 2004; 3; PMID:16646809; http://dx.doi.org/10.2202/1544-6115.1027
- Robinson MD, McCarthy DJ, Smyth GK. edgeR: a Bioconductor package for differential expression analysis of digital gene expression data. Bioinformatics (Oxford, England) 2010; 26:139-40; PMID:19910308; http://dx.doi.org/10.1093/bioinformatics/btp616
- Young MD, Wakefield MJ, Smyth GK, Oshlack A. Gene ontology analysis for RNA-seq: accounting for selection bias. Genome Biol 2010; 11:R14; PMID:20132535; http://dx.doi.org/10.1186/gb-2010-11-2-r14
- Luo W, Brouwer C. Pathview: an R/Bioconductor package for pathway-based data integration and visualization. Bioinformatics (Oxford, England) 2013; 29:1830-1; PMID:23740750; http://dx.doi.org/10.1093/bioinformatics/btt285
- Lindholm ME, Huss M, Solnestam BW, Kjellqvist S, Lundeberg J, Sundberg CJ. The human skeletal muscle transcriptome: sex differences, alternative splicing, and tissue homogeneity assessed with RNA sequencing. FASEB J 2014.
- Basso K, Margolin AA, Stolovitzky G, Klein U, Dalla-Favera R, Califano A. Reverse engineering of regulatory networks in human B cells. Nat Genet 2005; 37:382-90; PMID:15778709; http://dx.doi.org/10.1038/ng1532