Abstract
Osteosarcoma is the most common primary malignant bone tumor in children. Validated biological markers for disease prognosis available at diagnosis are lacking. No genome-wide DNA methylation studies linked to clinical outcomes have been reported in osteosarcoma to the best of our knowledge. To address this, we tested the methylome at over 1.1 million loci in 15 osteosarcoma biopsy samples obtained prior to the initiation of therapy and correlated these molecular data with disease outcomes. At more than 17% of the tested loci, samples obtained from patients who experienced disease relapse were more methylated than those from patients who did not have recurrence while patients who did not experience disease relapse had more DNA methylation at fewer than 1%. In samples from patients who went on to have recurrent disease, increased DNA methylation was found at gene bodies, intergenic regions and empirically-annotated candidate enhancers, whereas candidate gene promoters were unusual for a more balanced distribution of increased and decreased DNA methylation with 6.6% of gene promoter loci being more methylated and 2% of promoter loci being less methylated in patients with disease relapse. A locus at the TLR4 gene demonstrates one of strongest associations between DNA methylation and 5 y event-free survival (P-value = 1.7 × 10−6), with empirical annotation of this locus showing promoter characteristics. Our data indicate that DNA methylation information has the potential to be predictive of outcome in pediatric osteosarcoma, and that both promoters and non-promoter loci are potentially informative in DNA methylation studies.
Abbreviations
COG | = | Children's Oncology Group |
MSC | = | mesenchymal stem cell |
EFS | = | event free survival |
PCA | = | principal components analysis |
TLR4 | = | Toll-like receptor 4 |
Introduction
New diagnoses of osteosarcoma are made in ∼400 children in the United States annually, making it the most common pediatric primary bone cancer.Citation1 With aggressive treatment combining chemotherapy and surgical resection, 70–75 percent of patients are cured. At present, the most powerful prognostic marker in patients presenting with localized disease is the tumor response to neoadjuvant chemotherapy as graded by the Huvos system. The presence of more than 10 percent viable tumor following neoadjuvant chemotherapy (Grades I-II) is associated with a worse clinical outcome.Citation2 Aside from the presence of metastasis at diagnosis and histologic response to neoadjuvant chemotherapy, validated prognostic markers have been elusive.Citation3,4
Focused studies of DNA methylation in osteosarcoma have found aberrant methylation in several genes related to tumorigenesis and resistance to chemotherapy. Methylation of the reduced folate carrier (SLC19A1) gene promoter may help explain the need to use doses of methotrexate that are significantly higher than those used in other cancers.Citation5,6 Other studies have found instances of promoter methylation silencing the genes encoding the p53 regulatory proteins p16INK4A and p14ARF (both encoded by the CDKN2A gene).Citation7–9 However, few genome-wide studies on patient samples have been reported,Citation10,11 and these did not correlate DNA methylation patterns with patient survival. In this pilot study, we evaluated the DNA methylomes of 15 osteosarcoma biopsy samples and correlated these methylation profiles with patient clinical data.
Results
Genome-wide DNA methylation profiling
The HELP-tagging assay allows quantification of DNA methylation at up to 2.2 million HpaII sites throughout the genome.Citation12 Genomic representations by the methylation-sensitive HpaII enzyme are more accurately reflective of DNA methylation when compared with its methylation-insensitive isoschizomer MspI, which controls for differences in the ability of individual loci to be amplified. In tumors, gains and losses of chromosome regions can confound many genome-wide assays, but the concurrent generation of the MspI representation from our osteosarcoma samples allows adjustment to account for this source of variability. We show in Figure S1 that there is a strong correlation (R2 = 0.72) between HELP-tagging and targeted bisulfite MassArray data. The ability of the MspI representation to generate different representations in regions of copy number gains or losses is illustrated by Figure S2, showing representative examples of amplifications and deletions. The data therefore appeared to be sufficiently robust for further analyses.
Genome-wide DNA methylation at the time of diagnosis is correlated with future relapse
We analyzed the DNA methylation values of 1.1 million loci located on autosomes from primary osteosarcoma biopsy specimens obtained from 15 pediatric patients (). Unsupervised hierarchical clustering identified 2 major groups (). The clinical correlate best represented by this clustering pattern was 5 y event free survival (EFS), with one cluster composed entirely of patients who had disease relapse. We used principal components analysis (PCA) and linear modeling of both clinical and technical covariates to further confirm the association between DNA methylation and 5 y EFS ( and Fig. S3). Because of the worse prognosis for children with overt metastasis at diagnosis, we ascertained that DNA methylation was more strongly associated with disease relapse status as compared to the presence of metastasis at diagnosis (Fig. S3).
Table 1. Patient characteristics (n = 15)
Figure 1. Unsupervised hierarchical clustering and heatmap of osteosarcoma samples based on DNA methylation values using 10,000 random loci chosen from among the 10 percent most variable autosomal loci (standard deviation >27 ). Clinical correlates are plotted above the heatmap. The clustering pattern is similar to the hierarchical clustering obtained using all 1.1 million evaluable loci from HELP-tagging and segregates the samples into 2 main groups, with one group composed solely of samples from patients who would experience disease relapse.
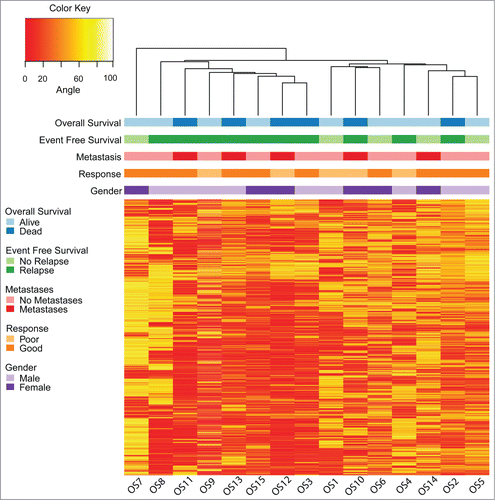
Increased genome-wide DNA methylation correlates with osteosarcoma relapse
Having found that differences in DNA methylation in osteosarcoma biopsy samples are associated with event-free survival, we explored the data further to identify how DNA methylation patterns differed based on relapse status. We observed that tissue from patients who would go on to develop disease relapse exhibited greater overall DNA methylation as compared to tumors from patients who did not relapse ().
Figure 3. (a.) Top panel: Density plot of distribution of differentially methylated loci for all genetic loci (black line) and differentially methylated loci with a P-value < 0.05 (dashed line). Bottom panel: Plot of DNA methylation differences between samples from patients who had osteosarcoma relapse and patients who did not have disease relapse (x-axis) and statistical significance (y-axis). DNA methylation tends to be greater in loci from patients with disease relapse as compared to patients who did not have relapse with more than 17% of loci being more methylated in the relapse group as compared to <1 % being more methylated in the no relapse group. (b.) Top panel: Density plot of distribution of differentially methylated loci for loci located within gene promoters (black line) and differentially methylated loci with a P-value < 0.05 (dashed line). Bottom panel: Plot of DNA methylation differences and statistical significance for loci located with gene promoters. Unlike DNA methylation differences in other genomic compartments, fewer loci within gene promoters are differentially methylated. Additionally, there is no predominance of increased DNA methylation in samples from patients with disease relapse.
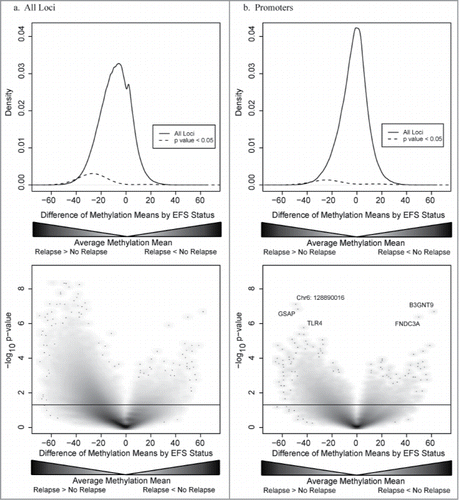
The majority of the normal genome is composed of methylated CpGs, excluding CpG islands, which are typically unmethylated.Citation13 The cancer genome has been found to reverse this pattern, with CpG islands gaining DNA methylation, while the rest of the genome loses methylationCitation14. More recently, attention has turned to CpG island shores (the 2 kb flanking CpG islands) as the sites of significant methylation change in cancer.Citation15 Given the overall greater DNA methylation in osteosarcoma samples obtained from patients who eventually relapsed, we reviewed how these methylation differences were distributed based on their relationship to CpG islands. DNA methylation changes were overrepresented in regions of the genome more than 2 kb from CpG islands (oceans) with an observed to expected ratio of 1.20. Conversely, both CpG islands and CpG island shores had fewer differentially methylated loci than expected (0.33 and 0.65, respectively). Nearly all of the differentially methylated loci in oceans and CpG island shores were characterized by greater DNA methylation in patients who had disease relapse. In contrast, while few CpG islands were differentially methylated, the direction of differential methylation was more balanced, with 3.8% of all loci within CpG islands (7,024/186,378 HpaII sites) being more methylated in patients who had osteosarcoma relapse and 2.2% less methylated (4,074/186,378).
The relationship of DNA methylation with gene expression varies based on genomic context.Citation16 Apart from fixed annotations like CpG islands, cell type-specific annotations using histone modifications can also be used. These post-translational modifications are dynamic markers of gene expression, with different histone marks and combinations correlating with different genomic functions.Citation17 Although the cell of origin of osteosarcoma remains unknown, it may ultimately derive from a bone marrow derived mesenchymal stem cell (MSC).Citation18 Using Roadmap ChIP-seq data from 3 human bone marrow-derived MSC samples, we divided the genome into 5 functional compartments—gene promoters, gene enhancers, transcribed gene bodies, non-transcribed gene bodies, and intergenic regions (). This annotation allows for the identification of the cis-regulatory landscape for MSCs, rather than the fixed, non-specific annotations of gene promoters using RefSeq, CpG island, or other annotations. Candidate enhancer regions are also identified, loci where DNA methylation may be more related to gene expression than promoters,Citation19 but which are not generally annotated in public databases.
Figure 4. Top: UCSC genome browser view of genomic function annotation generated using Roadmap Epigenomics bone marrow derived mesenchymal stem cell histone modification data for region surrounding TLR4. Bottom: Boxplots of angle value distributions for osteosarcomas grouped by relapse status at indicated HpaII restriction sites. HpaII restriction site 4 (denoted by asterisk) located within the TLR4 promoter is one of the most significantly differentially methylated loci (P-value 1.7 × 10−6) identified in this study.
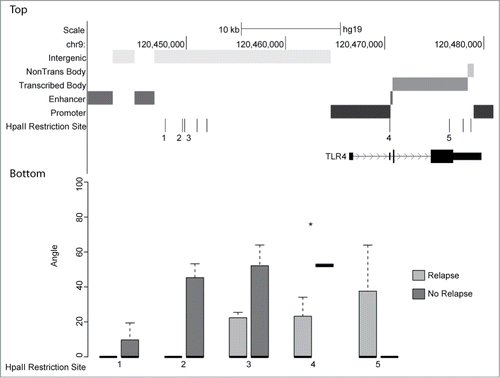
Loci within gene enhancers, gene bodies (both transcribed and not transcribed in mesenchymal stem cells), and intergenic regions demonstrated greater levels of DNA methylation in tumor samples obtained from patients who would experience disease relapse (Fig. S4). More than 18% of the 49,879 loci located within gene enhancers were differentially methylated (observed/expected ratio 1.01). Of the 504,727 loci within gene bodies, 16.3% of the loci were differentially methylated, slightly fewer than would have been expected (observed/expected ratio 0.91). Intergenic loci were differentially methylated at 23.6% of the 448,193 assayed loci, many more than would have been expected (observed/expected ratio 1.32). Gene promoter loci, while retaining this same pattern of increased methylation in patients who would relapse, had many fewer differentially methylated loci than would have been expected (observed/expected ratio 0.48). Additionally, nearly 2% of the 193,292 loci located within gene promoters were more methylated in patients who remained relapse free, while 6.6% of promoter loci were more methylated in patients with disease relapse ().
With the numerous reports of DNA methylation of promoter genes and differential gene expression in osteosarcoma, we wanted to confirm that despite finding a low percentage of differentially methylated loci in promoter regions, the differentially methylated loci that we identified were indeed most closely correlated with event-free survival. We therefore performed PCA of DNA methylation for the loci located within gene promoters and fit linear models predicting each component by clinical and technical covariates to confirm a significant correlation between DNA methylation status and event free survival (P-value =0.03) ().
Figure 5. Heatmap correlating principal component of promoter DNA methylation with clinical and technical variables. Shades of gray reflect the statistical significance of the correlation, with darker shades being more significant. The variable most closely correlated with principal component 1 (PC1) is event free survival (EFS) with a P-value of 0.03. Technical variables do not contribute to the DNA methylation patterns.
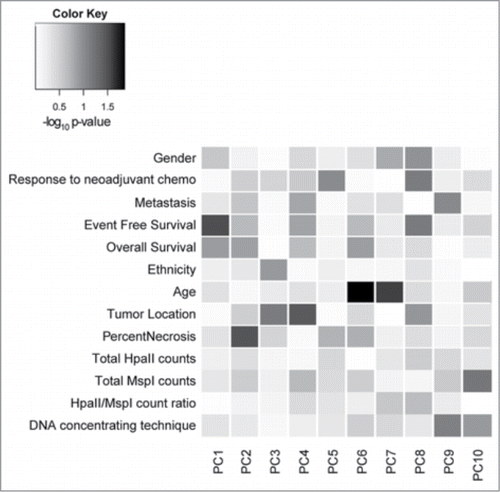
TLR4 promoter methylation is predictive of disease relapse
We noted that among the most differentially methylated genes in this cohort of osteosarcoma samples was a locus within the promoter of Toll like receptor 4 (TLR4) (). Identification that this locus located more than 4 kb downstream from the transcription start site was a gene promoter would not have been likely without our annotation of the genome using the Roadmap ChIP-seq data. DNA methylation of this promoter was significantly higher in samples obtained from patients who would go on to have disease relapse (P-value = 1.7 × 10−6, false discovery rate = 0.02) (). Patients with the least DNA methylation of the TLR4 promoter did not experience disease relapse within 5 y ().
Discussion
Progress in improving osteosarcoma therapy has been limited in the past 3 decades. For the 55% of patients with a good response to neoadjuvant chemotherapy, 5 y EFS approaches 70%, while for the other 45%, 5 y EFS remains under 40%.Citation2 Most disease relapses occur within the first 2 y following therapy.Citation20 The most recent international clinical trial assigned patients to different treatment intensifications based on response to neoadjuvant chemotherapy. However, treatment assignment could not be determined until after more than 20% of treatment was completed because of the lack of prognostic markers available at diagnosis.
Previous genome-wide studies in osteosarcoma have focused on DNA methylation changes at promoter regions and have found both hyper- and hypomethylation as compared to osteoblasts.Citation10,11 Single locus studies, again at promoter regions, also identified genes that have either increased or decreased DNA methylation. For example, methylation of the O6 methylguanine DNA methyltransferase (MGMT) promoter was associated with increased tumor necrosis following chemotherapy.Citation21 However, these studies typically did not focus on patient survival, which limits our ability to contextualize our results with the previously observed DNA methylation differences in osteosarcoma.
We undertook a genome-wide approach and assessed the DNA methylation status at over 1.1 million loci to obtain a comprehensive survey of DNA methylation in osteosarcoma to identify prognostic markers for disease relapse. Whereas previous genome-wide studies in osteosarcoma used microarray-based approaches that preferentially focused on gene promoters, we were able to assay multiple genomic functional compartments, interpreting these additional loci using the annotation that we derived from Roadmap ChIP-seq data. We also correlated these DNA methylation patterns with clinical data potentially allowing us to translate these findings to patient care.
The importance of differences in global methylation patterns is recognized in other human cancers. Figueroa et al identified 5 AML subtypes that could only be distinguished by their methylation profiles, with 2 of these subtypes having a better 2 y survival than the other 3 subtypes.Citation22 Colon cancer classification is partly based on its CpG island methylator phenotype (CIMP).Citation23 In accordance with these studies, our data suggest that DNA methylation patterns may have a role in classifying osteosarcoma patients according to their likelihood of relapse.
Classical DNA methylation studies in cancer have focused on fixed genomic elements, such as CpG islands and, more recently, CpG island shores, and heuristically defined promoter regions (usually ranging between 2-5 kilobases from annotated transcription start sites). However, these annotations do not account for the functional relevance of genomic loci within the cell type of interest and ignore large portions of the genome. Recent evidence has suggested that nearly 10% of the genome serves as enhancer elements.Citation19 The uncertainty of the association between specific enhancers and genes complicates the interpretation of an enhancer annotation. Advances in chromatin conformation capture and computational methods to integrate chromatin marks with gene expression data will improve these associations.Citation17 In the shorter term, our annotation approach serves as a valuable initial means of understanding the functional consequences of the DNA methylation changes that we identified.
The more balanced distribution of differentially methylated loci in gene promoters in our study suggests that, as would be expected from prior cancer epigenomics research, some gene promoters are more methylated in tumors that are more likely to relapse, while other genes are less methylated. Multiple gene expression signatures correlating with disease prognosis in osteosarcoma have been reported,Citation24,25 potentially indicating the consequence of altered epigenetic regulatory mechanisms. It remains to be tested if an epigenetic signature will be more robustly predictive of disease outcome.
Compared with promoters, differential methylation in other genomic regions in cancer is less well examined. Some of these loci fall within annotated genes and may be indicative of active gene transcription or repression. However, most fall within intergenic regions, indicating a role for differential methylation at these intergenic loci. While a subset of these intergenic loci is likely to encode cis-regulatory elements, changes in DNA methylation on a global level have potential influences in maintaining chromosomal stability, with hypomethylation and instability in cancer described previously.Citation26,27 The osteosarcoma genome is very unstable, with a complex karyotype that can even vary even within the same tumor. Whole genome sequencing has demonstrated that structural abnormalities, rather than single nucleotide variation, is the principal source of recurrent mutations in osteosarcoma.Citation28 In addition to these numerical abnormalities, chromosomal structure anomalies, such as double minute and ring chromosome may be present.Citation29 Increased chromosomal instability has been associated with poorer outcome in osteosarcoma.Citation30 We took into account the possibility that copy number variability in the specimens we studied could be influencing our DNA methylation data, using parallel MspI profiling and showing that these assays did indeed appear to be capable of detecting these regions of copy number variation (Fig. S2). Previous studies have evaluated both copy number and DNA methylation and did not identify a relationship between DNA methylation changes and copy number aberrations.Citation10,11 However, these studies focused on cell lines, which do not maintain the same DNA methylation patterns as patient-derived samples,Citation31 limiting their applicability to patient-derived samples such as those used here. The DNA hypermethylation that we found in non-transcribed genomic regions of tumors from patients who went on to relapse may possibly help to stabilize these aberrant chromosomes, linking these 2 unfavorable prognostic markers.
The emergence of TLR4 as one of the top significantly methylated genes may reflect the importance of the immune system in eradicating osteosarcoma. The importance of stimulating the immune response in osteosarcoma has been well studied. Multiple clinical trials of immune system stimulation have been conducted with agents such as GM-CSF and muramyl tripeptide. Toll-like receptors play a critical role in the immune response to bacterial infections. They also play a role in detecting endogenous damage associated patterns, oftentimes associated with cellular release of heat-shock proteins, components of the extracellular matrix, and mitochondrial DNA.Citation32 Divergent roles for TLR4 expression have been described in different cancers, with increased TLR4 expression in breast cancer correlating with disease progression,Citation33 while in colon cancer TLR4 expression correlated with less metastasis.Citation34 A more meaningful model may call for examining the role that TLR4 plays in mesenchymal stem cells where different Toll-like receptors play different immunomodulatory roles. TLR4 expression promotes a pro-inflammatory state,Citation35 which may explain the correlation of TLR4 promoter hypomethylation and decreased disease relapse that we identified. In osteosarcoma, TLR4 expression by the tumor might elicit a more robust host immune response that in conjunction with systemic chemotherapy eradicates micrometastases. Conversely, silencing of TLR4 may be a mechanism of immune evasion that enables other tumors to persist.
Most of the genes that we identified as being differentially methylated in this cohort of patients in osteosarcoma have not been well studied. We have focused our attention on TLR4 because of the well-established influence of the immune system on osteosarcoma growth. We also identified a locus adjacent to a DNase hypersensitivity region on chromosome 6 that is nearly 50 kb from the nearest annotated gene, suggesting the presence of a regulatory element in this region. Gamma secretase associated protein (GSAP),Citation36 part of the gamma secretase regulatory network, has been well-studied in Alzheimer disease, but its importance to tumorigenesis and survival, especially because of its interactions with gamma secretase (currently the target of multiple therapeutic trials in cancer) remains unexplored. Several members of the β-1–3-N-acetylglucosaminyltransferase family have been implicated in cell invasion and migration of multiple tumor types,Citation37-39 suggesting that β-1–3-N-acetylglucosaminyltransferase 9 (B3GNT9) may play a similar function. Finally, the fibronectins, including FNDC3A, mediate cell adhesion with potential consequences for cancer metastasis.Citation40
The limited number of samples available for our study precludes definitive statements regarding the prognostic significance of global DNA methylation patterns or of specific genomic loci in osteosarcoma disease recurrence risk; prospective validation of these findings is necessary. Concurrent gene expression testing would also have been valuable to verify that the DNA methylation differences that we identified led to gene expression alterations. Furthermore, different tumor samples may use different mechanisms (deletion/amplification, mutation, or DNA methylation) to alter gene expression. Thus, for optimal definition of the mechanisms of molecular pathogenesis in osteosarcoma, an integrative genomic approach will be necessary. This should include comprehensive genome, methylome, and transcriptome profiling of a substantial number of osteosarcoma samples. Our study demonstrates the importance of using a whole-genome methylation profiling approach, rather than a more narrow approach that focuses on gene promoters or CpG-dense regions of the genome.
The costs of whole genome bisulfite sequencing remain daunting and may preclude the use of this assay for large scale projects in osteosarcoma. Our use of histone modification data provided by the Roadmap Epigenomics project may allow for a more targeted approach. The generation of a functional genome annotation in osteosarcoma, similar to the annotation that we generated using bone marrow derived mesenchymal stem cells, would decrease the size of the methylome to be studied, by focusing on candidate cis-regulatory elements at which altered DNA methylation is more likely to be consequential in terms of gene expression and resulting cellular properties defining disease prognosis.
In summary, genome-wide DNA methylation profiles show evidence for correlation with disease outcome in pediatric osteosarcoma. Using a genome annotation derived from publicly available histone modification data for bone marrow derived mesenchymal stem cells, we were able to determine that tumor samples from patients who developed disease relapse were more methylated in most genomic contexts, though to a lesser extent at gene promoters. Future studies of DNA methylation in osteosarcoma may identify the specific differentially methylated genes that are most predictive of disease relapse and new targets of therapy.
Materials and Methods
Subject recruitment and DNA extraction
Fresh frozen pretreatment biopsy samples of primary osteosarcoma were obtained from the Children's Oncology Group (COG). Due to limitations in adequate tissue availability (in terms of tumor content, volume, and DNA quality) for this pilot study, tissue samples were from patients treated on one of 3 COG therapeutic protocols—INT0133 (1 patient), P9754 (9 patients), and AOST0121 (5 patients with metastatic disease). All patients and/or guardians provided written consent for enrollment on a COG osteosarcoma biology study. All patients received methotrexate, doxorubicin, and cisplatin, with the possible addition of ifosfamide or trastuzumab.Citation41-43 Patient outcome data through June 2012 were obtained from the COG. A single patient with metastatic disease at initial presentation had only completed 3.4 y of off-treatment follow up. As the median time to disease relapse in patients with metastatic osteosarcoma is 1.2 years,Citation44 this patient was considered as not having disease relapse in all analyses. DNA was extracted using proteinase K and RNase A followed by phenol/chloroform extraction with either ethanol precipitation or dialysis against a 0.2× saline sodium citrate buffer and dehydration with PEG 20,000. DNA quality was assessed with gel electrophoresis and spectrophotometry. This study was approved by the Albert Einstein College of Medicine Institutional Review Board.
DNA methylation analysis
DNA methylation patterns were characterized using the massively parallel sequencing-based HELP-tagging assay, as previously described.Citation12 Genomic DNA was digested with either the methylation sensitive restriction enzyme, HpaII, or its methylation insensitive isoschizomer, MspI. An Illumina sequencing adapter containing a T7 transcriptional promoter and an EcoP15I restriction site at the 3′ end was ligated to the digested DNA, enabling digestion with EcoP15I and the generation of a 27 base pair genomic DNA fragment. After end repair and dA tailing, a second Illumina sequencing adapter was added. Following in vitro transcription, reverse transcription, and PCR amplification, the completed library was sequenced on the Illumina HiSeq 2000 platform. Chromosomal instability in osteosarcoma leads to frequent amplifications and deletions, some of which occur recurrently, while others are unique to each tumor.Citation45-47 Therefore, MspI references for each osteosarcoma sample were generated to improve the accuracy of our DNA methylation measurements by taking into account the differences in copy number using the MspI reference libraries.
Massarray verification
Genomic DNA was bisulfite converted using the EZ DNA Methylation Lightning Kit (Zymo Research, Irvine, CA) following the manufacturer's protocol. Bisulfite primers for 4 regions of TLR4 including HpaII/MspI restriction sites, in addition to 10 other loci, were designed using MethPrimer.Citation48 Primer sequences and melting temperatures are listed in Table S1. PCR was performed using the FastStart High Fidelity Taq polymerase (Roche, Branchburg, NJ) with the following conditions: 95°C for 5 min followed by 38 cycles at 95°C for 30 s, primer specific Tm for 30 s, and 72°C extension for 1 min, and finally 72°C for 5 min for the final extension. Methylation values were calculated with the MassArray packageCitation49 and correlated with the DNA methylation values obtained from HELP-tagging (Figs. S1 and S5).
Data analysis
Primary sequence alignment for HELP-tagging data was performed using CASAVA allowing for a maximum of 2 mismatches. To control for differing numbers of total reads for each sample, DNA methylation values expressed as angle values were calculated as previously describedCitation12 after rescaling the log and z transformations of the raw HpaII and MspI counts. Data are available on the Gene Expression Omnibus (GSE59200). Only loci with angle values for more than 80% of the samples were retained for analysis. Samples were grouped by unsupervised hierarchical clustering of the Euclidean distance of DNA methylation values using the complete agglomeration method. To ensure that technical variables were not biasing the DNA methylation results, principal components analysis of the DNA methylation results was performed and each principal component was fit into separate linear models containing known clinical and technical covariates.
Differentially methylated loci were defined as loci where the group angle means differed by >20. Significantly differentially methylated loci were identified using an unpaired T-test. Using a variation on a previously described analysis,Citation50 in which the MspI read number was used to define regions of increased or decreased copy number, the MspI reference was used to demonstrate copy number changes in the tumors.
Genomic elements (gene enhancers, promoters, transcribed gene bodies, non-transcribed gene bodies, and intergenic regions) were identified using publicly-available ChIP-seq data from 3 bone marrow derived mesenchymal stem cell (MSC) samples studied as part of the Roadmap Epigenomics project. For each MSC sample, peak calls were made for each histone modification using SICER.Citation51 Consensus peaks for each histone modification were then generated by identifying peaks present in at least 2 of the 3 samples. Genomic cis-regulatory elements were defined by the co-localization of the constitutive different histone modifications characterizing each element (Table S2). Non-transcribed gene bodies were distinguished from intergenic regions by the absence of associated histone modifications within a genomic region annotated to have a RefSeq gene.
Event-free survival probabilities were calculated using Kaplan-Meier analysis. Groups were identified based on locus specific methylation status, using a cutoff angle of 50 to define hyper- and hypomethylation. The difference in survival was assessed using the log rank test with a threshold of α = 0.05 for statistical significance. All statistical analyses were performed using R statistical software.
Disclosure of Potential Conflicts of Interest
No potential conflicts of interest were disclosed.
SupplementalFiles.docx
Download MS Word (274.2 KB)Supplemental_Tables_and_Figures.pdf
Download PDF (278.3 KB)Funding
This work was supported by the Albert Einstein College of Medicine Center for Epigenomics and by the Chair's Grant U10 CA98543 and Human Specimen Banking Grant U24 CA114766 of the Children's Oncology Group from the National Cancer Institute, National Institutes of Health, Bethesda, MD USA. Additional support for research is provided by a grant from the WWWW (QuadW) Foundation, Inc. (www.QuadW.org) to the Children's Oncology Group. JMR is supported by a Paul Calabresi Career Development Award in Clinical Oncology (NCI K12 CA132783).
Supplemental Material
Supplemental data for this article can be accessed on the publisher's website.
References
- Janeway K, Gorlick R, Bernstein M. Osteosarcoma. Oncology of Infancy and Childhood Philadelphia: Saunders Elsevier 2009:871-910.
- Bielack SS, Kempf-Bielack B, Delling G, Exner GU, Flege S, Helmke K, Kotz R, Salzer-Kuntschik M, Werner M, Winkelmann W, et al. Prognostic factors in high-grade osteosarcoma of the extremities or trunk: an analysis of 1,702 patients treated on neoadjuvant cooperative osteosarcoma study group protocols. J Clin Oncol 2002; 20:776-90; PMID:11821461; http://dx.doi.org/10.1200/JCO.20.3.776
- Bramer JA, van Linge JH, Grimer RJ, Scholten RJ. Prognostic factors in localized extremity osteosarcoma: a systematic review. Eur J Surg Oncol 2009; 35:1030-6; PMID:19232880; http://dx.doi.org/10.1016/j.ejso.2009.01.011
- Clark JC, Dass CR, Choong PF. A review of clinical and molecular prognostic factors in osteosarcoma. J Cancer Res Clin Oncol 2008; 134:281-97; PMID:17965883; http://dx.doi.org/10.1007/s00432-007-0330-x
- Yang R, Li WW, Hoang BH, Kim H, Banerjee D, Kheradpour A, Healey JH, Meyers PA, Bertino JR, Gorlick R. Quantitative correlation between promoter methylation and messenger RNA levels of the reduced folate carrier. BMC Cancer 2008; 8:124; PMID:18452618; http://dx.doi.org/10.1186/1471-2407-8-124
- Yang R, Qin J, Hoang BH, Healey JH, Gorlick R. Polymorphisms and methylation of the reduced folate carrier in osteosarcoma. Clin Orthop Relat Res 2008; 466:2046-51; PMID:18528741; http://dx.doi.org/10.1007/s11999-008-0323-3
- Patino-Garcia A, Pineiro ES, Diez MZ, Iturriagagoitia LG, Klussmann FA, Ariznabarreta LS. Genetic and epigenetic alterations of the cell cycle regulators and tumor suppressor genes in pediatric osteosarcomas. J Pediatr Hematol Oncol 2003; 25:362-7; PMID:12759621; http://dx.doi.org/10.1097/00043426-200305000-00003
- Hou P, Ji M, Yang B, Chen Z, Qiu J, Shi X, Lu Z. Quantitative analysis of promoter hypermethylation in multiple genes in osteosarcoma. Cancer 2006; 106:1602-9; PMID:16502411; http://dx.doi.org/10.1002/cncr.21762
- Oh JH, Kim HS, Kim HH, Kim WH, Lee SH. Aberrant methylation of p14ARF gene correlates with poor survival in osteosarcoma. Clin Orthop Relat Res 2006; 442:216-22; PMID:16394764; http://dx.doi.org/10.1097/01.blo.0000188063.56091.69
- Sadikovic B, Yoshimoto M, Al-Romaih K, Maire G, Zielenska M, Squire JA. In vitro analysis of integrated global high-resolution DNA methylation profiling with genomic imbalance and gene expression in osteosarcoma. PLoS One 2008; 3:e2834; PMID:18698372; http://dx.doi.org/10.1371/journal.pone.0002834
- Kresse SH, Rydbeck H, Skarn M, Namlos HM, Barragan-Polania AH, Cleton-Jansen AM, Serra M, Liestol K, Hogendoorn PC, Hovig E, et al. Integrative analysis reveals relationships of genetic and epigenetic alterations in osteosarcoma. PLos One 2012; 7:e48262; PMID:23144859; http://dx.doi.org/10.1371/journal.pone.0048262
- Suzuki M, Jing Q, Lia D, Pascual M, McLellan A, Greally JM. Optimized design and data analysis of tag-based cytosine methylation assays. Genome Biol 2010; 11:R36; PMID:20359321; http://dx.doi.org/10.1186/gb-2010-11-4-r36
- Rauch TA, Wu X, Zhong X, Riggs AD, Pfeifer GP. A human B cell methylome at 100-base pair resolution. Proc Natl Acad Sci U S A 2009; 106:671-8; PMID:19139413; http://dx.doi.org/10.1073/pnas.0812399106
- Esteller M. Epigenetics in cancer. N Engl J Med 2008; 358:1148-59; PMID:18337604; http://dx.doi.org/10.1056/NEJMra072067
- Irizarry RA, Ladd-Acosta C, Wen B, Wu Z, Montano C, Onyango P, Cui H, Gabo K, Rongione M, Webster M, et al. The human colon cancer methylome shows similar hypo- and hypermethylation at conserved tissue-specific CpG island shores. Nat Genet 2009; 41:178-86; PMID:19151715; http://dx.doi.org/10.1038/ng.298
- Ball MP, Li JB, Gao Y, Lee JH, LeProust EM, Park IH, Xie B, Daley GQ, Church GM. Targeted and genome-scale strategies reveal gene-body methylation signatures in human cells. Nat Biotechnol 2009; 27:361-8; PMID:19329998; http://dx.doi.org/10.1038/nbt.1533
- Ernst J, Kheradpour P, Mikkelsen TS, Shoresh N, Ward LD, Epstein CB, Zhang X, Wang L, Issner R, Coyne M, et al. Mapping and analysis of chromatin state dynamics in nine human cell types. Nature 2011; 473:43-9; PMID:21441907; http://dx.doi.org/10.1038/nature09906
- Mutsaers AJ, Walkley CR. Cells of origin in osteosarcoma: mesenchymal stem cells or osteoblast committed cells? Bone 2014; 62:56-63; PMID:24530473; http://dx.doi.org/10.1016/j.bone.2014.02.003
- Ziller MJ, Gu H, Muller F, Donaghey J, Tsai LT, Kohlbacher O, De Jager PL, Rosen ED, Bennett DA, Bernstein BE, et al. Charting a dynamic DNA methylation landscape of the human genome. Nature 2013; 500:477-81; PMID:23925113; http://dx.doi.org/10.1038/nature12433
- Kempf-Bielack B, Bielack S, Jürgens H, Branscheid D, Berdel W, Exner G, Göbel U, Helmke K, Jundt G, Kabisch H, et al. Osteosarcoma relapse after combined modality therapy: an analysis of unselected patients in the Cooperative Osteosarcoma Study Group (COSS). J Clin Oncol 2005; 23:559-627; PMID:15659502; http://dx.doi.org/10.1200/JCO.2005.04.063
- Cui Q, Jiang W, Guo J, Liu C, Li D, Wang X, Zeng Y. Relationship between hypermethylated MGMT gene and osteosarcoma necrosis rate after chemotherapy. Pathol Oncol Res 2011; 17:587-91; PMID:21424568; http://dx.doi.org/10.1007/s12253-010-9354-7
- Figueroa ME, Lugthart S, Li Y, Erpelinck-Verschueren C, Deng X, Christos PJ, Schifano E, Booth J, van Putten W, Skrabanek L, et al. DNA methylation signatures identify biologically distinct subtypes in acute myeloid leukemia. Cancer Cell 2010; 17:13-27; PMID:20060365; http://dx.doi.org/10.1016/j.ccr.2009.11.020
- Toyota M, Ahuja N, Ohe-Toyota M, Herman JG, Baylin SB, Issa JP. CpG island methylator phenotype in colorectal cancer. Proc Natl Acad Sci U S A 1999; 96:8681-6; PMID:10411935; http://dx.doi.org/10.1073/pnas.96.15.8681
- Man TK, Chintagumpala M, Visvanathan J, Shen J, Perlaky L, Hicks J, Johnson M, Davino N, Murray J, Helman L, et al. Expression profiles of osteosarcoma that can predict response to chemotherapy. Cancer Res 2005; 65:8142-50; PMID:16166288; http://dx.doi.org/10.1158/0008-5472.CAN-05-0985
- Mintz MB, Sowers R, Brown KM, Hilmer SC, Mazza B, Huvos AG, Meyers PA, Lafleur B, McDonough WS, Henry MM, et al. An expression signature classifies chemotherapy-resistant pediatric osteosarcoma. Cancer Res 2005; 65:1748-54; PMID:15753370; http://dx.doi.org/10.1158/0008-5472.CAN-04-2463
- Eden A, Gaudet F, Waghmare A, Jaenisch R. Chromosomal instability and tumors promoted by DNA hypomethylation. Science 2003; 300:455; PMID:12702868; http://dx.doi.org/10.1126/science.1083557
- Gaudet F, Hodgson JG, Eden A, Jackson-Grusby L, Dausman J, Gray JW, Leonhardt H, Jaenisch R. Induction of tumors in mice by genomic hypomethylation. Science 2003; 300:489-92; PMID:12702876; http://dx.doi.org/10.1126/science.1083558
- Chen X, Bahrami A, Pappo A, Easton J, Dalton J, Hedlund E, Ellison D, Shurtleff S, Wu G, Wei L, et al. Recurrent somatic structural variations contribute to tumorigenesis in pediatric osteosarcoma. Cell Rep 2014; 7:104-12; PMID:24703847; http://dx.doi.org/10.1016/j.celrep.2014.03.003
- Bridge JA, Nelson M, McComb E, McGuire MH, Rosenthal H, Vergara G, Maale GE, Spanier S, Neff JR. Cytogenetic findings in 73 osteosarcoma specimens and a review of the literature. Cancer Genet Cytogenet 1997; 95:74-87; PMID:9140456; http://dx.doi.org/10.1016/S0165-4608(96)00306-8
- Smida J, Baumhoer D, Rosemann M, Walch A, Bielack S, Poremba C, Remberger K, Korsching E, Scheurlen W, Dierkes C, et al. Genomic alterations and allelic imbalances are strong prognostic predictors in osteosarcoma. Clin Cancer Res 2010; 16:4256-67; PMID:20610556; http://dx.doi.org/10.1158/1078-0432.CCR-10-0284
- Bork S, Pfister S, Witt H, Horn P, Korn B, Ho AD, Wagner W. DNA methylation pattern changes upon long-term culture and aging of human mesenchymal stromal cells. Aging Cell 2010; 9:54-63; PMID:19895632; http://dx.doi.org/10.1111/j.1474-9726.2009.00535.x
- Yu L, Wang L, Chen S. Endogenous toll-like receptor ligands and their biological significance. J Cell Mol Med 2010; 14:2592-603; PMID:20629986; http://dx.doi.org/10.1111/j.1582-4934.2010.01127.x
- Yang H, Zhou H, Feng P, Zhou X, Wen H, Xie X, Shen H, Zhu X. Reduced expression of Toll-like receptor 4 inhibits human breast cancer cells proliferation and inflammatory cytokines secretion. J Exp Clin Cancer Res 2010; 29:92; http://dx.doi.org/10.1186/1756-9966-29-92
- Simiantonaki N, Kurzik-Dumke U, Karyofylli G, Jayasinghe C, Michel-Schmidt R, Kirkpatrick CJ. Reduced expression of TLR4 is associated with the metastatic status of human colorectal cancer. Int J Mol Med 2007; 20:21-9; PMID:17549384
- Waterman RS, Tomchuck SL, Henkle SL, Betancourt AM. A new mesenchymal stem cell (MSC) paradigm: polarization into a pro-inflammatory MSC1 or an Immunosuppressive MSC2 phenotype. PLoS One 2010; 5:e10088; PMID:20436665; http://dx.doi.org/10.1371/journal.pone.0010088
- He G, Luo W, Li P, Remmers C, Netzer WJ, Hendrick J, Bettayeb K, Flajolet M, Gorelick F, Wennogle LP, et al. Gamma-secretase activating protein is a therapeutic target for Alzheimer's disease. Nature 2010; 467:95-8; PMID:20811458; http://dx.doi.org/10.1038/nature09325
- Ho WL, Che MI, Chou CH, Chang HH, Jeng YM, Hsu WM, Lin KH, Huang MC. B3GNT3 expression suppresses cell migration and invasion and predicts favorable outcomes in neuroblastoma. Cancer Sci 2013; 104:1600-8; PMID:24118321; http://dx.doi.org/10.1111/cas.12294
- Lu CH, Wu WY, Lai YJ, Yang CM, Yu LC. Suppression of B3GNT7 gene expression in colon adenocarcinoma and its potential effect in the metastasis of colon cancer cells. Glycobiology 2014; 24:359-67; PMID:24418929; http://dx.doi.org/10.1093/glycob/cwu002
- Ni J, Jiang Z, Shen L, Gao L, Yu M, Xu X, Zou S, Hua D, Wu S. beta3GnT8 regulates the metastatic potential of colorectal carcinoma cells by altering the glycosylation of CD147. Oncol Rep 2014; 31:1795-801; PMID:24573103
- Shan SW, Lee DY, Deng Z, Shatseva T, Jeyapalan Z, Du WW, Zhang Y, Xuan JW, Yee SP, Siragam V, et al. MicroRNA MiR-17 retards tissue growth and represses fibronectin expression. Nat Cell Biol 2009; 11:1031-8; PMID:19633662; http://dx.doi.org/10.1038/ncb1917
- Meyers PA, Schwartz CL, Krailo M, Kleinerman ES, Betcher D, Bernstein ML, Conrad E, Ferguson W, Gebhardt M, Goorin AM, et al. Osteosarcoma: a randomized, prospective trial of the addition of ifosfamide and/or muramyl tripeptide to cisplatin, doxorubicin, and high-dose methotrexate. J Clin Oncol 2005; 23:2004-11; PMID:15774791; http://dx.doi.org/10.1200/JCO.2005.06.031
- Schwartz CL, Wexler LH, Devidas M, Goorin A, Grier H, Meyers P, Bernstein M. P9754 therapeutic intensification in non-metastatic osteosarcoma: a COG trial. J Clin Oncol 2004; 22:802s-s; http://dx.doi.org/10.1200/JCO.2004.04.086
- Ebb D, Meyers P, Grier H, Bernstein M, Gorlick R, Lipshultz SE, Krailo M, Devidas M, Barkauskas DA, Siegal GP, et al. Phase II trial of trastuzumab in combination with cytotoxic chemotherapy for treatment of metastatic osteosarcoma with human epidermal growth factor receptor 2 overexpression: a report from the children's oncology group. J Clin Oncol 2012; 30:2545-51; PMID:22665540; http://dx.doi.org/10.1200/JCO.2011.37.4546
- Kager L, Zoubek A, Potschger U, Kastner U, Flege S, Kempf-Bielack B, Branscheid D, Kotz R, Salzer-Kuntschik M, Winkelmann W, et al. Primary metastatic osteosarcoma: presentation and outcome of patients treated on neoadjuvant cooperative osteosarcoma study group protocols. J Clin Oncol 2003; 21:2011-8; PMID:12743156; http://dx.doi.org/10.1200/JCO.2003.08.132
- Ozaki T, Schaefer KL, Wai D, Buerger H, Flege S, Lindner N, Kevric M, Diallo R, Bankfalvi A, Brinkschmidt C, et al. Genetic imbalances revealed by comparative genomic hybridization in osteosarcomas. Int J Cancer 2002; 102:355-65; PMID:12402305; http://dx.doi.org/10.1002/ijc.10709
- Bayani J, Zielenska M, Pandita A, Al-Romaih K, Karaskova J, Harrison K, Bridge JA, Sorensen P, Thorner P, Squire JA. Spectral karyotyping identifies recurrent complex rearrangements of chromosomes 8, 17, and 20 in osteosarcomas. Genes Chromosomes Cancer 2003; 36:7-16; PMID:12461745; http://dx.doi.org/10.1002/gcc.10132
- Atiye J, Wolf M, Kaur S, Monni O, Bohling T, Kivioja A, Tas E, Serra M, Tarkkanen M, Knuutila S. Gene amplifications in osteosarcoma-CGH microarray analysis. Genes Chromosomes Cancer 2005; 42:158-63; PMID:15540165; http://dx.doi.org/10.1002/gcc.20120
- Li LC, Dahiya R. MethPrimer: designing primers for methylation PCRs. Bioinformatics 2002; 18:1427-31; PMID:12424112; http://dx.doi.org/10.1093/bioinformatics/18.11.1427
- Thompson RF, Suzuki M, Lau KW, Greally JM. A pipeline for the quantitative analysis of CG dinucleotide methylation using mass spectrometry. Bioinformatics 2009; 25:2164-70; PMID:19561019; http://dx.doi.org/10.1093/bioinformatics/btp382
- Oda M, Glass JL, Thompson RF, Mo Y, Olivier EN, Figueroa ME, Selzer RR, Richmond TA, Zhang X, Dannenberg L, et al. High-resolution genome-wide cytosine methylation profiling with simultaneous copy number analysis and optimization for limited cell numbers. Nucleic Acids Res 2009; 37:3829-39; PMID:19386619; http://dx.doi.org/10.1093/nar/gkp260
- Zang C, Schones DE, Zeng C, Cui K, Zhao K, Peng W. A clustering approach for identification of enriched domains from histone modification ChIP-Seq data. Bioinformatics 2009; 25:1952-8; PMID:19505939; http://dx.doi.org/10.1093/bioinformatics/btp340