Abstract
Purpose: To identify and examine polymorphisms of genes associated with aggressive and clinical significant forms of prostate cancer among a screening cohort.
Experimental Design: We conducted a genome-wide association study among patients with aggressive forms of prostate cancer and biopsy-proven normal controls ascertained from a prostate cancer screening program. We then examined significant associations of specific polymorphisms among a prostate cancer screened cohort to examine their predictive ability in detecting prostate cancer.
Results: We found significant associations between aggressive prostate cancer and five single nucleotide polymorphisms (SNPs) in the 10q26 (rs10788165, rs10749408, and rs10788165, p value for association 1.3 × 10−10 to 3.2 × 10−11) and 15q21 (rs4775302 and rs1994198, p values for association 3.1 × 10−8 to 8.2 × 10−9) regions. Results of a replication study done in 3439 patients undergoing a prostate biopsy, revealed certain combinations of these SNPs to be significantly associated not only with prostate cancer but with aggressive forms of prostate cancer using an established classification criterion for prostate cancer progression (odds ratios for intermediate to high-risk disease 1.8–3.0, p value 0.003–0.001). These SNP combinations were also important clinical predictors for prostate cancer detection based on nomogram analysis that assesses prostate cancer risk.
Conclusions: Five SNPs were found to be associated with aggressive forms of prostate cancer. We demonstrated potential clinical applications of these associations.
Introduction
Several genome-wide association studies (GWAS) have identified a number of genomic variants which are associated with an increased risk of prostate cancer, particularly from the 8q24 region.Citation1-Citation3 Although these associations are statistically significant, it remains unclear to what extent these high-risk genotypes are associated with aggressive forms of prostate cancer.Citation4,Citation5
A primary goal of evaluating biomarkers for the early detection of prostate cancer is to distinguish patients who will eventually develop metastases from those with more indolent forms of cancer. Recently, a large, multi-centered study, including subjects from the Physicians’ Health Study, failed to show any associations with aggressive and lethal forms of prostate cancer with these and other SNPs found from past GWAS.Citation4 Also, a recent study examining the most significant SNPs found by past GWAS study found no associations to prostate cancer outcomes including measures of aggressiveness and cancer-specific mortality.Citation5 Thus, although new SNP associations have been numerous, the clinical benefit has been limited since in part that these SNPs cannot identify patients with aggressive forms of prostate cancer.
In a typical GWAS, the cases and controls are not derived from the same patient sample; however, this is not the case when screened subjects are studied. In a screening study, controls may be selected from men who screen negative for the cancer. Recent data from the Prostate Cancer Prevention TrialCitation6 has established that men who are judged to be at low risk for prostate cancer (i.e., normal patients), but who undergo a prostate biopsy, have a prevalence rate of 25% for prostate cancer. One-quarter of these are high-grade, aggressive cancers.Citation7 Thus, the misclassification of cases as controls may diminish the potential of discovering SNPs that help to identify men with aggressive, high grade prostate cancer. By using men with negative biopsies as controls, the potential for misclassification is minimized.
To identify new SNP variants for aggressive prostate cancer, we conducted a GWAS among men who had a prostate biopsy, using a two-stage approach. In the first stage, 316 cases and 229 controls were genotyped using the Affymetrix 500K SNP array (443,816 SNPs). Cases were patients with aggressive forms of prostate cancer using the established D’Amico classification criteria,Citation8 and controls were biopsy proven normal patients. In the second stage, we genotyped positive SNPs found from stage 1 among 3439 patients who underwent prostate biopsy for prostate cancer screening. We investigated their clinical significance by examining their association with D’Amico criteria outcomes and by nomogram analysis in predicting prostate risk.
Results
Stage 1: identification of novel SNPs from GWAS
We chose three criteria to select positive SNPs from the 316 cases and 229 controls that underwent GWAS analysis and chose 20 SNPs from them (): (1) a Bonferroni corrected p value of less than 0.01, and the SNP was from a region which has previously been shown to harbor a locus associated with prostate cancer (n = 5); (2) two or more SNPs were in linkage disequilibrium and the Bonferroni corrected p value for each was less than 0.01 (n = 9); or (3) the Bonferroni corrected p value was 10−5 or less (n = 6).
Table 1. List of 20 SNPs found by genome wide association study (GWAS) using the 500K Affymetrix single nucleotide polymorphism (SNP) chip to be associated with aggressive prostate cancer.
Stage 2: replication of 20 SNPs from prostate biopsy cohort
The 20 SNPs were tested in an independent sample of 3439 men of various ethnicities. All 3439 men underwent a prostate biopsy; 1663 (48.4%) were diagnosed with prostate cancer, and 1776 (51.6%) did not have any evidence of cancer from biopsy (). Among the 1663 men with cancer, 413 (24.8%) had a low risk cancer (based on the D’Amico classification of prostate cancer aggressiveness), 944 (56.8%) had an intermediate risk cancer, and 306 (18.4%) had a high risk prostate cancer.
Table 2. Comparison between cases and controls of risk factors, tumor markers for prostate cancer and the genotype frequencies of selected SNPs
In the replication set, nine of the 20 SNPs were significantly associated with prostate cancer. The strongest associations were found from five SNPs: three SNPs at region 10q26 (p value 6 × 10−7 to 3 × 10−10) and two SNPs at 15q21 (p value 7 × 10−6 to 1 × 10−7) (). The remaining four SNPs were from other regions, at 10q23 (rs7089868, rs11596082 and rs2351337) and at 8q13 (rs2053140), but the p values of association ranged from 0.05–0.02. Because of the large difference in the level of significance from the first five SNPs, the remaining four SNPs were excluded from further study.
The associations between the five SNPs and prostate cancer were only present among white subjects. No associations were found for men of Asian or African ancestries, although the sample sizes for these ethnic groups were smaller (Table S1). No other significant association was found with the other 15 SNPs. Of the three SNPs from region 10q26, two SNPs (rs11199874 and rs10788165) were in strong linkage disequilibrium (r2 = 0.97 in controls). The third SNP, rs10749408, was not in linkage disequilibrium (LD) with either of the other two. The two SNPs from region 15q21, rs4775302 and rs1994198, were in strong linkage disequilibrim (r2 = 0.99 in controls).
We asked whether these SNPs were associated with prostate cancer, after adjusting for established risk factors and other variables [age, family history of prostate cancer, ethnicity, urinary symptoms, prostate specific antigen (PSA) level and digital rectal examination]. The adjusted odds ratios, based on the risk genotype classes for the five SNPs, ranged from 1.26–1.42 (). To determine whether these variants were also associated with aggressive prostate cancer, we used the D’Amico criteria to divide the cases into low, intermediate and high-risk categories for prostate cancer progression. This criteria combines Gleason Score grade, clinical stage and PSA level at diagnosis into risk group categories and is a well-established method of predicting prostate cancer mortality.Citation8
Table 3. Crude and adjusted odds ratio based on variant genotypes. Analysis for 10q26 was based on a recessive model and for 15q21 on a dominant model. Multivariate model variables included age at biopsy, ethnicity, family history of prostate cancer, the presence of urinary voiding symptoms, prostate specific antigen (PSA) level and digital rectal exam (DRE) status.
We chose one SNP to represent each of the two haplotype blocks (the one with the highest odds ratio for prostate cancer) resulting in three SNPs (rs11199874, rs10749408, and rs4775302). We combined the risk alleles from the three SNPs and compared the frequencies of patients with low, intermediate, high risk prostate cancer and with no cancer, by the number of risk alleles (). Within each risk category, the odds ratios for cancer increased by the number of variant alleles (Table S2). In particular, for patients who were diagnosed with high risk prostate cancer, those with three variant alleles had a 3-fold increase in risk (odds ratio being 3.0, 95% CI: 1.5–5.8) for having high risk prostate cancer, compared with patients with no variant allele. The odds ratios for high risk cancer for patients with two variant alleles was 1.8 (95% CI: 0.9–3.4) and for one variant allele was 1.2 (95% CI: 0.6–2.4) with a significant increase in trend by the number of variant alleles (p < 0.0001) (Table S2). There was a significant positive trend in the odds ratios for having low, intermediate and high risk prostate cancer for patients with the three variant risk alleles (p = 0.0002). No significant trends were observed for patients with one or two variant alleles across disease risk categories. Overall, there was a global positive trend association by risk group and number of variant risk alleles (p = 0.048).
Table 4. Distribution of patients by aggressiveness of prostate cancer using low, intermediate and high risk categories for prostate cancer (D’Amico Classification). Variant alleles based on a combination of a 3 SNP model (rs11199874, rs10749408 and rs4775302)
To determine whether or not these SNPs could be used clinically in diagnosing prostate cancer, we examined the effect of SNPs within a multivariate logistic regression model in predicting prostate cancer using nomograms, and also assessed the clinical validity of these SNPs using sensitivity, specificity, positive predictive value (NPV) and negative predictive (NPV) analysis, as proposed by Kraft et al.Citation14 To construct the nomogram, all 20 SNPs were tested within the multivariate model. Based on the concordance indices of bias-corrected probabilities for any and aggressive prostate cancer, for each of these SNPs, the performance of each of the 20 SNPs and the three variant risk alleles were similar. Among white subjects, the nomogram with the three risk alleles was a significant predictor (p = 0.0002) of any cancer and high grade (Gleason Score 7 or more) cancer (). When examining the importance of each predictor from area under the curve (AUC) analysis, the three SNP model was the fourth (out of six) most important predictor for cancer (incremental drop of AUC being 0.004, ). It was a more important predictor than family history of prostate cancer and urinary symptoms. When considering the clinical performance of the three SNP model alone, the sensitivity was 94.4% for any cancer for patients with one or more variant alleles and the specificity was 78.2% for any cancer for patients with three variant alleles (). The positive and negative predictive values improved with the number of variant alleles, but the absolute levels were low, compared with the baseline prevalence. Also, the ROC curve for the 3 SNP model was better at diagnosing aggressive cancer than for any cancer ().
Figure 1. Nomogram analysis based on 2,810 white subjects: 1,428 with no evidence of cancer, 637 with low grade PCa (GS < = 6) and 745 with high grade PCa (GS 7–10). The nomogram is used by first locating a patient’s position for each variable on its horizontal scale and then a point value is assigned according to the points scale (top axis) and summed for all variables. Total points correspond to a probability value for having prostate cancer or aggressive prostate cancer. PSA, prostate specific antigen; FH, family history of prostate cancer; DRE, digital rectal examination; AUA Score, American Urological Prostate Urinary Symptom Score; and MOD3, 3 SNP risk allele model. For calibration plots, see Figure S2.
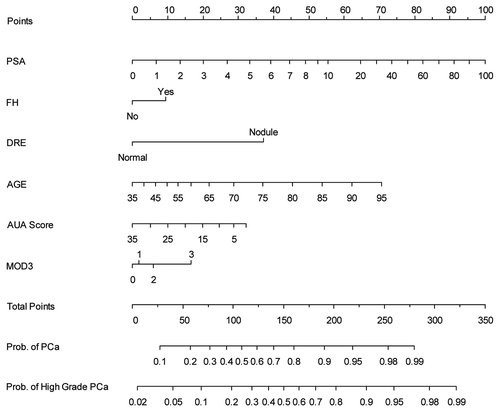
Table 5. Comparisons of sensitivity, specificity, positive predictive value, and negative predictive value based on the combination of the risk alleles from the three SNP model among white subjects only
Discussion
It is well established that men diagnosed with intermediate or high-risk prostate cancer based on the D’Amico criteria have a high chance for progression to metastasis. It is of primary importance to identify these men in a screening program. Our study is the first to demonstrate association of 10q26 and 15q21 region SNPs with prostate cancer, and to show that a combination of these risk alleles (three SNP model) is associated with aggressive prostate cancer. From nomogram analysis, the clinical performance of our SNP variants was not superior to PSA or age, but was important as other established risk factors, such as family history of prostate cancer.
The stage 1 analysis of this study did not reveal any associations between prostate cancer and previously-identified risk loci on 8q24 and 17q. This discrepancy likely reflects our specific analysis of patients with aggressive prostate cancer, as our prior analysis of the patient cohort included in stage 2, did reveal associations between 8q24 and 17q SNPs and prostate cancer, but not aggressive cancer.Citation15 Similarly, results of two recent large, multi-centered studies revealed no association of prostate cancer aggressiveness and mortality with these or other putative prostate cancer SNPs found by past large GWAS.Citation4,Citation16
The odds ratios for the five SNPs identified by our Stage 1 analysis were larger than those identified in Stage 2. This result could reflect a “Winner’s Curse” phenomenon,Citation17,Citation18 wherein the estimated effect of a marker allele from the initial GWAS may be exaggerated related to the estimated effect in a confirmatory study. Such a possibility could relate to our selection of “hypernormal” controls in Stage 1—men who had normal PSA levels or had multiple biopsies with no evidence of cancer.
No previous studies have reported prostate cancer association with the chromosomal 10q26 and 15q21 regions or with genes in these regions. The three disease-associated SNPs on chromosome 10q26 map within 70 kb from one another and the two SNPs in LD (rs11199874 and rs10788165) are separated by 12 kb. These three SNPs span a 590 kb region encompassing two genes, WDR11 and FGFR2, that have been linked to glioblastomasCitation19 and breast cancer.Citation20 The two SNPs on 15q21 are 13 kb apart and map 880 kb from the closest gene, GATM, which encodes a mitochondrial enzyme.
A limitation of our study is that a proportion of our controls may be misclassified due to false-negative results of the prostate biopsy. Approximately 10–20% of patients have been found to have cancer from repeat prostate biopsy after an initial negative biopsy.Citation21,Citation22 In Stage 1, this bias was minimized by selecting control patients who had a low risk for having prostate cancer with low PSA levels (< 10 ng/mL, with half having a normal PSA level of < 4.0 ng/mL).
Thus, from a GWAS based on cases with aggressive prostate cancer and controls with no evidence of cancer from biopsy, we have found new associations of SNPs at 10q26 and 15q21. Certain combinations of these SNPs are associated with aggressive forms of prostate cancer and have similar clinical importance compared with other risk factors for prostate cancer. Further elucidation of additional SNPs or genes in these new regions would be important to examine before these and potentially other SNPs from these regions can be used clinically to predict prostate cancer.
Materials and Methods
Study subjects
Patients were drawn from a sample of 4573 men who underwent a prostate biopsy from a prostate cancer screening program between 1999 and 2008 within an urban, North American-based population. Patients of any age were included in the study if they had an abnormal prostate specific antigen (PSA) value (> 4.0 ng/mL) or digital rectal exam (DRE). Patients were also included if they had a normal PSA level (< 4.0 ng/mL) or DRE, but were willing to undergo a prostate biopsy for the purpose of prostate cancer screening (n = 408). Patients eligible for this study were unselected and were accrued consecutively. No patient had a past history of prostate cancer. All patients underwent one or more transrectal ultrasonography (TRUS)-guided needle core biopsies. Patients were excluded if they were not capable of giving consent to participate in a research study (n = 46); or if they could not provide sufficient baseline information (n = 53). Blood samples were obtained prior to prostate biopsy. Six to fifteen ultrasound-guided needle core biopsies were performed (median = 8), using an 18-gauge spring loaded biopsy device. Samples were obtained using a systematic pattern and additional targeted samples were obtained from suspicious areas. The primary endpoint was the histologic presence of adenocarcinoma of the prostate in the biopsy specimen. All grading was based on the Gleason scoring system.Citation9 A urological voiding history (AUA Symptom ScoreCitation10), DRE results, serum PSA level, family history of prostate cancer information, and ethnic background were obtained. This study was approved by the research ethics board (Sunnybrook Health Sciences Center).
Stage analysis
To maximize the probability of identifying SNPs that can predict patients with aggressive forms of prostate cancer we conducted our study in two stages. Stage 1 consisted of a GWAS to identify novel SNPs. We restricted our GWAS analysis to patients diagnosed with intermediate to high risk prostate cancer for progression to metastasis defined by the D`Amico criteriaCitation8 of prostate cancer risk in order to maximize the probability of finding clinically significant SNPs (rather than those associated with indolent forms of prostate cancer). Stage 2 consisted of a replication study among a separate cohort to determine whether the SNPs from Stage 1 could predict aggressive forms of prostate cancer among a prostate biopsy screening cohort who underwent a prostate biopsy. To fully evaluate whether SNPs can be used in a clinical setting within the context of prostate cancer detection and screening, we evaluated whether these SNPs could predict any prostate cancer and its aggressive forms.
For Stage 1, subjects were derived from the first 1,000 subjects who underwent a prostate biopsy. We identified 545 cases and controls from the 1,000 eligible patients for SNP array analysis. Of the 545 patients, 316 cases and 229 controls were genotyped using the Affymetrix 500K SNP array (443,816 SNPs). Cases were white patients with screen-detected prostate cancer and who had a high risk of progression based on the D’Amico criteria which is and established method of risk stratification for prostate cancer progression; i.e., either a Gleason Score of 7 and a PSA level of > 10 ng/mL or a Gleason Score of 8–10. Controls were white patients who had no evidence of cancer, based on one or more systematic multi-core (median number = 8 cores per biopsy session) prostate biopsies; 105 controls had a normal PSA level and 124 controls had a PSA level between 4.0 and 10.0 ng/mL.
For Stage 2, the remaining 3573 patients were included in the replication study. The primary endpoint was the presence of adenocarcinoma of the prostate. We assessed whether the SNPs from Stage 1 was associated with any prostate cancer and aggressive forms defined by the D’Amico criteria. This criteria categorizes patients with prostate cancer into three groups: low, intermediate and high risk based Gleason score, clinical stage and PSA level. Because of the three categories of aggressiveness and the potential multiple number of SNP genotypes found, to assess the association with aggressive forms of prostate cancer, we grouped the SNPs in combination and compared them to the D’Amico risk groups. We also examined their ability to predict prostate and aggressive forms of prostate within a nomogram model to assess its clinical significance.
SNP array analysis and genotyping
In Stage 1, using the 500K Affymetrix SNP array, 443,816 SNPs were analyzed. Of those SNPs, 401,593 were retained after being excluded for not being in Hardy-Weinberg equilibrium (HWE)(p > 0.001), had a minor allele frequency of < 0.05, or a call rate of < 95%. To deal with population stratification, we used principal component analysis to adjust for variances within ethnic subgroups with EIGENSTRAT (Golden HelixTree, V6.4.1).Citation11 Five eigenvectors were used and three clusters were observed. A Q-Q plot is provided in Figure S1.
Genotyping was conducted using mass-spectrometry-based genotyping analysis and matrix-assisted laser desorption ionization—time of flight (MassArray System, Sequenom Inc.) following the manufacturer’s instructions. A standard protocol for multiplex homogeneous mass extend assay developed by Sequenom Inc. was utilized and modified according to designed primers. For quality control, we assigned negative controls for each test plate (Microseal TM 384 V2.0).
Data analysis for replication study
For Stage 2, we excluded 40 samples that failed on two or more of the assays used. The call rates were at least 95% for each SNP. Genotype distributions for all tested SNPs were in Hardy-Weinberg Equilibrium (HWE). Cases were defined as patients with prostate cancer and controls were men with no evidence of cancer. Allele frequencies for each SNP were calculated for cases and controls and the distributions were compared. Allelic odds ratios were calculated based on a multiplicative model. For genotypes, frequency comparisons were compared for an additive, dominant or recessive genetic model for each of the SNPs with unconditional logistic regression. The model with the highest likelihood was considered to be the best fitting model for each SNP.Citation12 We tested the cumulative effects of selected SNPs for each model by counting the number of genotypes associated with prostate cancer on the basis of the best-fitting genetic model from single-SNP analysis; the odds ratios for prostate cancer for patients with one or more variant genotypes were estimated. In the multivariate analysis, we adjusted for age, ethnic group, family history of prostate cancer, the presence of lower urinary tract voiding symptoms, the total PSA level, and the digital rectal examination. Unconditional logistic regression analysis was used to examine how each of these factors, alone and in combination, would predict the presence of prostate cancer and aggressive forms of prostate cancer, defined by the D’Amico classification.Citation8
Nomogram construction
To develop a clinical instrument that incorporates the SNP findings, we considered all SNPs in a nomogram multivariate model, restricted to white subjects only. This nomogram was designed to predict both prostate cancer and aggressive cancer defined as having intermediate to high-grade prostate cancer (Gleason Score 7 or more). Ordinal logistic regression was used to model the probability of having low or high grade cancer. Three outcome levels were defined: (1) no cancer; (2) low-grade cancer (Gleason Score 6 or less; and (3) intermediate to high-grade cancer (Gleason Score 7 or more). Continuous variables were modeled with restricted cubic splines to avoid linearity assumptions. The logistic regression model was the basis for constructing a nomogram. All patients were used to develop and validate the nomogram. Bootstrapping was used to correct for optimism in the evaluation of discrimination and calibration. All analyses were performed using S-Plus 2000 Professional software (Statistical Sciences) with the Design and Hmisc libraries added.Citation13
Acknowledgments
This study was funded in part by the Canadian Institute of Health Research, Operating Grant MOP-79530 and the National Cancer Institute Grants 010294 and 017092 and CCSRI 019038
Disclosure of Potential Conflicts of Interest
No potential conflicts of interest were disclosed.
Note
Supplemental material can be found at: http://www.landesbioscience.com/journals/cbt/article/18366/.
References
- Eeles RA, Durocher F, Edwards S, Teare D, Badzioch M, Hamoudi R, et al. Linkage analysis of chromosome 1q markers in 136 prostate cancer families. Am J Hum Genet 1998; 62:653 - 8; http://dx.doi.org/10.1086/301745; PMID: 9497242
- Gudmundsson J, Sulem P, Manolescu A, Amundadottir LT, Gudbjartsson D, Helgason A, et al. Genome-wide association study identifies a second prostate cancer susceptibility variant at 8q24. Nat Genet 2007; 39:631 - 7; http://dx.doi.org/10.1038/ng1999; PMID: 17401366
- Yeager M, Orr N, Hayes RB, Jacobs KB, Kraft P, Wacholder S, et al. Genome-wide association study of prostate cancer identifies a second risk locus at 8q24. Nat Genet 2007; 39:645 - 9; http://dx.doi.org/10.1038/ng2022; PMID: 17401363
- Penney KL, Salinas CA, Pomerantz M, Schumacher FR, Beckwith CA, Lee GS, et al. Evaluation of 8q24 and 17q risk loci and prostate cancer mortality. Clin Cancer Res 2009; 15:3223 - 30; http://dx.doi.org/10.1158/1078-0432.CCR-08-2733; PMID: 19366828
- Wiklund FE, Adami HO, Zheng SL, Stattin P, Isaacs WB, Gronberg H, et al. Established prostate cancer susceptibility variants are not associated with disease outcome. Cancer Epidemiol Biomarkers Prev 2009; 18:1659 - 62; http://dx.doi.org/10.1158/1055-9965.EPI-08-1148; PMID: 19423541
- Thompson IM, Goodman PJ, Tangen CM, Lucia MS, Miller GJ, Ford LG, et al. The influence of finasteride on the development of prostate cancer. N Engl J Med 2003; 349:215 - 24; http://dx.doi.org/10.1056/NEJMoa030660; PMID: 12824459
- Thompson IM, Pauler DK, Goodman PJ, Tangen CM, Lucia MS, Parnes HL, et al. Prevalence of prostate cancer among men with a prostate-specific antigen level < or =4.0 ng per milliliter. N Engl J Med 2004; 350:2239 - 46; http://dx.doi.org/10.1056/NEJMoa031918; PMID: 15163773
- D'Amico AV, Whittington R, Malkowicz SB, Schultz D, Blank K, Broderick GA, et al. Biochemical outcome after radical prostatectomy, external beam radiation therapy, or interstitial radiation therapy for clinically localized prostate cancer. JAMA 1998; 280:969 - 74; http://dx.doi.org/10.1001/jama.280.11.969; PMID: 9749478
- Gleason DF, Mellinger GT. Prediction of prognosis for prostatic adenocarcinoma by combined histological grading and clinical staging. J Urol 1974; 111:58 - 64; PMID: 4813554
- Barry MJ, Fowler FJJ, O'Leary MP, Bruskewitz RC, Holtgrewe HL, Mebust WK, et al. The American Urological Association symptom index for benign prostatic hyperplasia. The Measurement Committee of the American Urological Association. J Urol 1992; 148:1549 - 57; PMID: 1279218
- Price AL, Patterson NJ, Plenge RM, Weinblatt ME, Shadick NA, Reich D. Principal components analysis corrects for stratification in genome-wide association studies. Nat Genet 2006; 38:904 - 9; http://dx.doi.org/10.1038/ng1847; PMID: 16862161
- Zheng SL, Sun J, Wiklund F, Smith S, Stattin P, Li G, et al. Cumulative association of five genetic variants with prostate cancer. N Engl J Med 2008; 358:910 - 9; http://dx.doi.org/10.1056/NEJMoa075819; PMID: 18199855
- Harrell FE. Regression modeling strategies with applications to linear models. In: Logistic Regression, and Survival Analysis. New York: Springer-Verlag 2001.
- Kraft P, Wacholder S, Cornelis MC, Hu FB, Hayes RB, Thomas G, et al. Beyond odds ratios–communicating disease risk based on genetic profiles. Nat Rev 2009; 10:264 - 9
- Nam RK, Zhang WW, Trachtenberg J, Seth A, Klotz LH, Stanimirovic A, et al. Utility of Incorporating Genetic Variants for the Early Detection of Prostate Cancer. Clin Cancer Res 2009; 15:1787 - 93; http://dx.doi.org/10.1158/1078-0432.CCR-08-1593; PMID: 19223501
- Kader AK, Sun J, Isaacs SD, Wiley KE, Yan G, Kim ST, et al. Individual and cumulative effect of prostate cancer risk-associated variants on clinicopathologic variables in 5,895 prostate cancer patients. Prostate 2009; In press http://dx.doi.org/10.1002/pros.20970; PMID: 19434657
- Kraft P. Curses—winner's and otherwise—in genetic epidemiology. In: Epidemiology. Cambridge, Mass 2008:649-51; discussion 57-8.
- Lohmueller KE, Pearce CL, Pike M, Lander ES, Hirschhorn JN. Meta-analysis of genetic association studies supports a contribution of common variants to susceptibility to common disease. Nat Genet 2003; 33:177 - 82; http://dx.doi.org/10.1038/ng1071; PMID: 12524541
- Chernova OB, Hunyadi A, Malaj E, Pan H, Crooks C, Roe B, et al. A novel member of the WD-repeat gene family, WDR11, maps to the 10q26 region and is disrupted by a chromosome translocation in human glioblastoma cells. Oncogene 2001; 20:5378 - 92; http://dx.doi.org/10.1038/sj.onc.1204694; PMID: 11536051
- Liang J, Chen P, Hu Z, Zhou X, Chen L, Li M, et al. Genetic variants in fibroblast growth factor receptor 2 (FGFR2) contribute to susceptibility of breast cancer in Chinese women. Carcinogenesis 2008; 29:2341 - 6; http://dx.doi.org/10.1093/carcin/bgn235; PMID: 18845558
- Nam RK, Toi A, Trachtenberg J, Jewett MA, Klotz L, Fleshner N, et al. Variation in patterns of practice in diagnosing screen-detected prostate cancer. BJU Int 2004; 94:1239 - 44; http://dx.doi.org/10.1111/j.1464-410X.2004.05150.x; PMID: 15610097
- Presti JC Jr. Prostate biopsy strategies. Nat Clin Pract Urol 2007; 4:505 - 11; http://dx.doi.org/10.1038/ncpuro0887; PMID: 17823604