Abstract
A Bayesian network expansion algorithm called BN+1 was developed to identify undocumented gene interactions in a known pathway using microarray gene expression data. In our recent paper, the BN+1 algorithm has been successfully used to identify key regulators including uspE in the E. coli ROS pathway and biofilm formation.18 In this report, a synthetic network was designed to further evaluate this algorithm. The BN+1 method was found to identify both linear and nonlinear relationships and correctly identify variables near to the starting network. Using experimentally derived data, the BN+1 method identifies the gene fdhE as a potentially new ROS regulator. Finally, a range of possible score cutoff methods are explored to identify a set of criteria for selecting BN+1 calls.
Acknowledgements
This research was supported in part by NIH Grant U54-DA-021519, NIH Training Grant (5 T32 GM070449-04), 2008 Rackham Spring/Summer Research Grant at the University of Michigan, and the University of Michigan Bioinformatics Program.
Figures and Tables
Figure 1 Synthetic network and corresponding BN+1 results for two-variable core expansion. (A) A synthetic eight-variable network. (B) Seven distinct core networks composed of two adjacent variables were used for BN+1 expansion analysis. In each row, integers represent the ranks of the BN+1 variables (where 1 = top scoring gene, etc.,). (C) The posterior score distribution of BN+1 variables identified in the first row of (A). (D) Plot of absolute values of pair-wise Pearson correlations for all variables. The black star denotes a relationship (between F and G) that has a poor Pearson correlation (coefficient = 0.056). White stars denote good relations between variables with correlation coefficient ≥0.5 and separated by at least one variable in the synthetic network (A). (E) A nonlinear relationship between variables F and G.
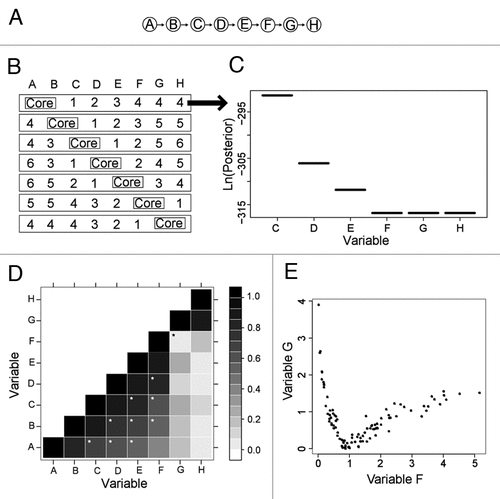
Figure 2 Analysis of the potential RO S gene fdhE predicted by BN+1. (A) Consensus Bayesian network generated from 13 networks sharing the same top log posterior score. (B) Selected relationships between fdhE and its associated genes. Nonlinear relationships were often observed. The ellipse in the fnr-fdhE plot highlights a group of fnr-fdhE associations that are discussed in the text.
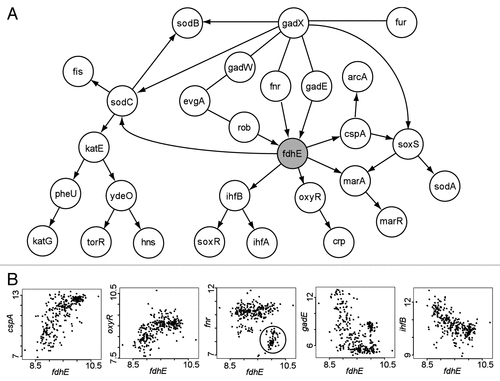
Figure 3 Analysis of top BN+1 genes in the RO S use case. (A) Generic plot of best score for top 200 BN+1 genes. (B) Variation in scores for top 10 genes. The BN+1 genes are ranked by maximum scores of all networks containing the core genes plus one additional gene. Genes sorted by posterior scores are shown in horizontal axis. Box plots for the set of scores pertaining to each gene are displayed. The variations are calculated based on various simulations in different computers. To perform each simulation, a simulated annealing approach was used with an unfixed structural prior (i.e., the core network edges) with multiple replicates and moderate simulation time to allow a comprehensive though non-exhaustive search.
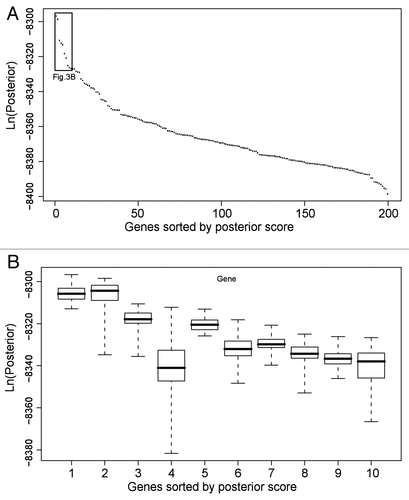
Addendum to: