Abstract
Receptor-mediated signal transduction networks, comprised of multiple biochemical pathways, control cell responses and are therefore central to normal and aberrant physiological processes. An appreciation for the inherent complexities of these networks has matured in recent years, to the point where it is now apparent that experimental measurements will need to be combined with computational modeling and analysis to best interpret and predict how individual mechanisms (protein-protein interactions, enzymatic reactions, etc.) are integrated at the network level. To progress along these lines, there is a major barrier to overcome: although a deep mechanistic understanding of signal transduction has been achieved, data sets of a suitably quantitative nature are still lacking. Based on our efforts to systematically acquire and analyze such measurements, we contend that the level of detail in models of signaling networks ought to be limited by the availability of quantitative data, rather than by the much greater availability of qualitative information about signaling interactions. Although this approach is sensible from a data-driven modeling perspective, it is controversial because it gives the false impression that molecular-level details are being ignored.
Signal transduction networks in mammalian cells are accessed by various growth factor and cytokine receptors to elicit responses such as cell cycle progression, death or survival and cell migration. The extracellular signal-regulated kinase (ERK) signaling pathway is a master controller of these and other responses in normal and transformed cells.Citation1,Citation2 In our recent paper,Citation3 we presented a systematic analysis of the signaling network accessed by platelet-derived growth factor (PDGF) receptors in mouse fibroblasts. In this system, ERK is activated through two parallel pathways: the canonical pathway involving the small GTPase Ras and, perhaps even more potently, through phosphoinositide-3-kinase (PI3K)-dependent signaling.Citation4 Once activated, ERK is strongly regulated by negative feedback attenuation. The complexity of this system, involving multiple pathways and modes of dynamic regulation, is probably typical of signal transduction networks and demands an integrative approach combining quantitative measurements, kinetic modeling and computational analysis.Citation5,Citation6 Although many different modeling modalities are available, the most common (and usually most sensible) approach is to express the conservation of each chemical species as an ordinary differential equation in time. Such a model is formulated according to the modeler's style and sensibilities, both biological and mathematical; two experienced modelers could study the same network and arrive at unique sets of equations with different molecularlevel details explicitly described. As our knowledge of the underlying biochemical mechanisms expands, there is a natural tendency to formulate mathematical descriptions with progressively greater detail. One might ask, is this not a sign of progress? In this addendum article, we argue that data-driven models as typically formulated should be less detailed than the mechanistic information in the literature might allow.
The complexity of a kinetic model may be measured in terms of its number of constant, adjustable parameters, which include rate and equilibrium constants together with initial species concentrations.Citation7 In the literature, one can find several examples of data-driven models of signaling networks, encompassing at least two pathways, with well over 100 adjustable parameters.Citation8–Citation11 To illustrate how the level of mechanistic detail dictates model complexity, consider the receptor-mediated recruitment of guanine nucleotide exchange factor (GEF) activity, which promotes conversion of Ras to its active, GTP-bound state (). The most common description of this process in models is based on the knowledge that a prominent GEF, Sos, is recruited via association with an adaptor protein Grb2, which in turn binds to a second adaptor, Shc. Shc binds to activated receptors and is phosphorylated while in the receptor complex, a prerequisite for Grb2-Sos binding. If one were to take activated receptors as an input and apply mass-action kinetics, this small part of a network-level model minimally requires 10 parameters (total amounts of Shc, Grb2 and Sos, association and dissociation rate constants for each of the Shc-receptor, Grb2-Shc and Sos-Grb2 interactions and a catalytic rate constant for Shc phosphorylation) plus a few more to describe Shc dephosphorylation by protein tyrosine phosphatase (PTP) activity ().
There are two issues with this approach. First, it would only be supremely justified if one had quantitative measurements and molecular perturbations of each of the individual interactions as they occur in cells. Data at that level of detail are as yet non-existent; values of rate constants determined using purified components (which are not often the full-length proteins) should not be taken as “known.” Second, such a description of the system is still a far cry from the level of mechanistic detail that is currently known. It ignores information about multiple receptor phosphorylation sites, multi-valency of the interactions and different protein isoforms, not to mention Ras-specific GEFs other than Sos.
The alternative approach is to identify and then model in a realistic way only the essential feature(s) of each subprocess in the network. In the case of Ras-GEF recruitment, the essence of the process is the reversible association of the enzymatic activity in complex with activated receptors (). Roughly speaking, this is how the process is described in our recent models; from a data-driven point of view, this simplified (coarse-grained) module adequately captures the saturable relationship between two measured variables: phosphorylated PDGF receptors and GTP-bound Ras.Citation3,Citation4
Based on these ideas, we propose the following three-tiered stratification of kinetic models at the network level: coarsegrained (typically requiring tens of parameters), minimal mass action (typically requiring hundreds of parameters) and fully mechanistic (parameter requirements unknown, but easily >1,000). Our model of the PDGF receptor network,Citation3 with 57 parameters, is of the coarse-grained type. The parameters were serially fit to different subsets of new and archived data acquired in our laboratory;Citation4,Citation12,Citation13 considering the various biochemical readouts, stimulation conditions and molecular perturbations, there are more than 400 distinct measurements. Trained on this diverse data set, the resulting model was demonstrated to be quantitatively predictive.
Our formulation of the model equations was based mainly on experience and intuition; hence, after successfully aligning the model to all of the available data, we wondered what minimal level of detail would have been allowed without significantly affecting the quality of fit. Considering parameters that were pegged at arbitrarily high or low values, we conservatively estimated that a model with about 20 parameters would have been sufficient. For example, a model assuming that all kinases and phosphatases operate far from saturation (allowing each Vmax and KM pair to be lumped into a single parameter) would have performed almost equally as well. Thus, the volume of data available in this case allowed for a reexamination of the model, with the prospect of reducing rather than increasing parametric complexity.
Why are simpler kinetic models often sufficient to capture trends in quantitative data, even relatively large data sets? Assuming that the underlying assumptions of the model are reasonable, only a certain number of adjustable parameters are needed to capture distinct features presented by the data. For example, consider a readout such as ERK phosphorylation, which exhibits partial adaptation with time (). In a single time course, the number of data features for such a readout is roughly four (). Considering how the shape of the time course changes across different conditions, however, yields a total number of distinct features that is far less than the number of time courses multiplied by four. This is because a systematic data set will contain measurements for multiple readouts, which are mathematically coupled to one another in the model. To illustrate this point, suppose that one were to measure phosphorylation of both ERK and the upstream kinase, MEK, for a number of common time courses (16 in our data setCitation3). Regardless of the number of conditions for which both phospho-MEK and phospho-ERK are measured, one should only need to invoke a certain number of kinetic parameters to describe the mechanisms coupling MEK activity to ERK phosphorylation (); in other words, unless there are clear inconsistencies in the data, we are inclined to assume that these parameters apply across all experimental conditions.
By its nature, data-driven modeling is an iterative process. Obviously, as new data are acquired, a model's granularity needs to be reevaluated, especially if one were to use the model to make comparisons across cell backgrounds. Thus, we envision that the process of model building would be driven as much by the content of the available data as the data gathering process is driven by model predictions.
Figures and Tables
Figure 1 Mathematical descriptions of the same biology can vary in mechanistic complexity. Both panels here depict the same subprocess encountered in growth factor receptor signaling networks: receptor-mediated recruitment of guanine nucleotide exchange factor (GEF) activity, which promotes conversion of the small GTPase Ras to its GTP-bound form. (A) A minimal mass-action description requires explicit accounting of all molecular constituents (as described in the text), resulting in a large number of adjustable parameters. (B) A coarse-grained description instead considers only the association of the GEF with the receptor complex, limited by the availability of a certain, sparingly abundant component (the GEF itself or an intermediary adaptor). Other steps in the formation of the complex are effectively lumped.
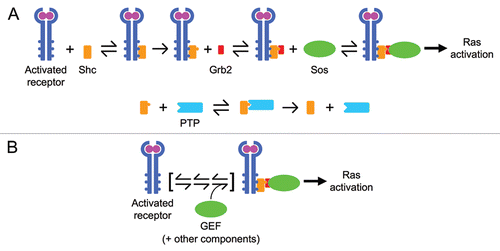
Figure 2 The number of model parameters needed should be much fewer than the number of distinct measurements to which the model is compared. (A) A signaling readout exhibiting partial adaptation has as few as four distinct features: the peak magnitude, the time at which it is reached, the characteristic time or rate of decay from the peak to the subsequent plateau, and the magnitude of the plateau. (B) We systematically measured both MEK and ERK phosphorylation for an array of stimulation and perturbation conditions; a portion (25%) of those data are shown here. The symbols and solid curves are, respectively, the means of the data and the model output aligned globally to all of the data, color-coded according to the dose of growth factor (adapted from Fig. 5 of ref. Citation3). Michaelis Menten-type equations coupling ERK phosphorylation to the active form of MEK are shown below; simplification of these equations to neglect enzyme saturation would reduce the number of adjustable parameters from 8 to 4.
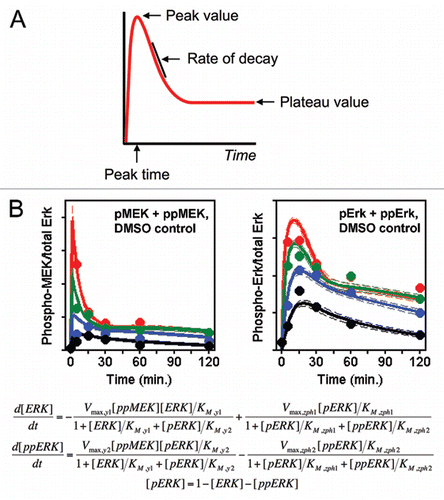
Acknowledgements
This work was supported by the National Institutes of Health (GM088987).
Addendum to:
References
- Dhillon AS, Hagan S, Rath O, Kolch W. MAP kinase signalling pathways in cancer. Oncogene 2007; 26:3279 - 3290
- Roberts PJ, Der CJ. Targeting the Raf-MEK-ERK mitogen-activated protein kinase cascade for the treatment of cancer. Oncogene 2007; 26:3291 - 3310
- Cirit M, Wang CC, Haugh JM. Systematic quantification of negative feedback mechanisms in the extracellular signal-regulated kinase (ERK) signaling network. J Biol Chem 2010; 285:36736 - 36744
- Wang CC, Cirit M, Haugh JM. PI3K-dependent crosstalk interactions converge with Ras as quantifiable inputs integrated by Erk. Mol Syst Biol 2009; 5:246
- Iyengar R. Why we need quantitative dynamic models. Sci Signal 2009; 2:3
- Kreeger PK, Lauffenburger DA. Cancer systems biology: a network modeling perspective. Carcinogenesis 2010; 31:2 - 8
- Weng G, Bhalla US, Iyengar R. Complexity in biological signaling systems. Science 1999; 284:92 - 96
- Bhalla US, Ram PT, Iyengar R. MAP kinase phosphatase as a locus of flexibility in a mitogen-activated protein kinase signaling network. Science 2002; 297:1018 - 1023
- Kiyatkin A, Aksamitiene E, Markevich NI, Borisov NM, Hoek JB, Kholodenko BN. Scaffolding protein Grb2-associated binder 1 sustains epidermal growth factor-induced mitogenic and survival signaling by multiple positive feedback loops. J Biol Chem 2006; 281:19925 - 19938
- Birtwistle MR, Hatakeyama M, Yumoto N, Ogunnaike BA, Hoek JB, Kholodenko BN. Ligand-dependent responses of the ErbB signaling network: experimental and modeling analyses. Mol Syst Biol 2007; 3:144
- Chen WW, Schoeberl B, Jasper PJ, Niepel M, Nielsen UB, Lauffenburger DA, et al. Input-output behavior of ErbB signaling pathways as revealed by a mass action model trained against dynamic data. Mol Syst Biol 2009; 5:239
- Park CS, Schneider IC, Haugh JM. Kinetic analysis of platelet-derived growth factor receptor/phosphoinositide-3-kinase/Akt signaling in fibroblasts. J Biol Chem 2003; 278:37064 - 37072
- Schneider IC, Haugh JM. Quantitative elucidation of a distinct spatial gradient-sensing mechanism in fibroblasts. J Cell Biol 2005; 171:883 - 892