Abstract
Aberrant DNA methylation often occurs in colorectal cancer (CRC). In our study we applied a genome-wide DNA methylation analysis approach, MethylCap-seq, to map the differentially methylated regions (DMRs) in 24 tumors and matched normal colon samples. In total, 2687 frequently hypermethylated and 468 frequently hypomethylated regions were identified, which include potential biomarkers for CRC diagnosis. Hypermethylation in the tumor samples was enriched at CpG islands and gene promoters, while hypomethylation was distributed throughout the genome. Using epigenetic data from human embryonic stem cells, we show that frequently hypermethylated regions coincide with bivalent loci in human embryonic stem cells. DNA methylation is commonly thought to lead to gene silencing; however, integration of publically available gene expression data indicates that 75% of the frequently hypermethylated genes were most likely already lowly or not expressed in normal tissue. Collectively, our study provides genome-wide DNA methylation maps of CRC, comprehensive lists of DMRs, and gives insights into the role of aberrant DNA methylation in CRC formation.
Introduction
Colorectal cancer (CRC) is the third most common cancer, and the third leading cause of cancer death in the world.Citation1 CRC arises as a consequence of accumulation of genetic mutations and epigenetic alterations in colonic cells. Common genetic alterations in CRC include mutations in the APC gene and in DNA mismatch repair genes. At the epigenetic level, promoter DNA methylation causing the silencing of tumor suppressor genes, such as CDKN2A (the p16/INK4a gene), is a common alteration.Citation2-Citation4
Identification of differential DNA methylation patterns between normal and cancer cells may shed light on the processes underlying tumorigenesis. Another objective of studying DNA methylation is to identify biomarkers for diagnostic and prognostic use. Specifically, aberrant methylation of a particular gene (or group of genes) can provide a useful clinical tool for early detection of CRC or for stratifying tumors into subtypes.
Extensive efforts have been invested in identifying regions with aberrant DNA methylation in CRC and in finding correlations with tumor development or phenotype. Until recently, most studies were performed on small sets of loci, but now large-scale methods are emerging.Citation5-Citation8
Here, we investigated DNA methylation in CRC genome-wide using MethylCap-seq.Citation9 Our study provides comprehensive DNA methylation maps of CRC and matched normal colon tissue, and identifies differentially methylated regions (DMRs). We determined characteristics of these DMRs using publically available expression and epigenetic data sets, and select a list of candidate biomarkers for CRC detection.
Results
DNA methylation profiles of CRC cases and matched controls
To investigate DNA methylation in CRC in a genome-wide unbiased fashion, we applied MethylCap-seq. This method involves capture of methylated DNA using the MBD domain of MeCP2, and subsequent next-generation Illumina sequencing of eluted DNA as schematically outlined in Figure S1.Citation9 MethylCap-seq was performed on 24 tumors and an equal number of matched normal samples (48 DNA methylation profiles in total).
A representative screen shot of the normalized DNA methylation data visualized in the UCSC genome browser is shown in . This example shows DNA methylation shared by normal and tumor samples, as well as sample-specific methylation and an occasional region that is differentially methylated between tumors and normal samples.
Figure 1. Comparison of DNA methylation in tumor and normal tissue. (A) A representative screen shot of the normalized DNA methylation data uploaded in the UCSC genome browser. 14T indicates a tumor sample; 14N indicates the matched normal sample. In total, 5 sample pairs are shown. The read-out of MethylCap-seq is semi-quantitative; the number of sequence reads homologous to a genomic region gives a measure for DNA methylation at that region. In the screenshot several regions show DNA methylation. Most peaks of methylation are present in all samples (indicated with gray boxes at the top), except for the methylation at the promoter of LHX5 (red box), which is only present in the tumors and not in the normal tissue. (B) Scatterplot of the median methylation level of normal tissue and tumor. (C) Scatterplot of the standard deviation of normal tissue and tumor. (D) The first two principal components resulting from PCA of all DNA methylation profiles separate the tumors and normal colon samples. The red dots are tumors; the blue dots normal samples.
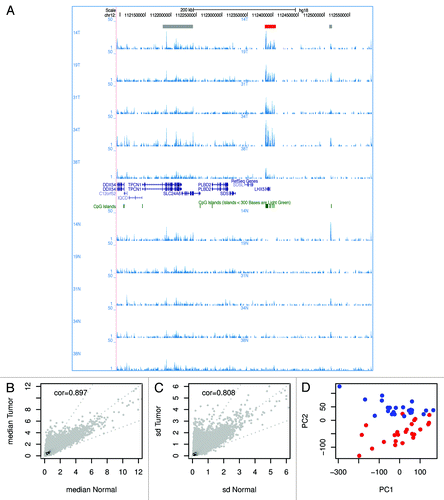
For each of the 48 samples, the genomic regions with elevated numbers of aligned sequence reads were identified and merged, yielding 329,613 regions of potential interest, with a median length of 2,192 bp. These regions are distributed across all chromosomes (Fig. S2). Per sample, the number of normalized sequence reads overlapping each region of interest was calculated, which is referred to as read density and used as a measure of DNA methylation.
No strong associations were found between the first two principal components of the samples in the methylation space and any of the sample preparation and processing factors (Table S2 and ), indicating that there are no strong batch effects present in the data.
Previously, we extensively reported that methylated regions identified by MethylCap-seq could be validated with classical bisulfite sequencing.Citation9-Citation12 To validate the current MethylCap-seq, four fragments were selected and assessed in two sample pairs. These fragments overlap with the promoters of SDC2, SIM2, ROBO1 and CYP1B1. In all cases, bisulfite sequencing corroborated the MethylCap-seq data: hypermethylation at SDC2, and SIM2 in the two tumors, ROBO1 promoter hypermethylation in one of the two tumors, and no differential methylation of CYP1B1 (Fig. S3). Thus, MethylCap-seq provides a good representation of DNA methylation.
Differential DNA methylation in tumor vs. normal tissue
For each tumor and matched normal sample the read densities in the peaks of interest were compared. The Pearson correlation coefficients for the pairs range from 0.337 to 0.897 (median = 0.723), whereby only four sample pairs have a correlation below 0.5 (Fig. S4A). Furthermore, for most regions the median methylation level of tumor and normal and the standard deviation for tumor and normal are very similar (). Nevertheless, principal component analysis (PCA) separated normal tissue from tumor (). This suggests that, although the genome methylation generally remains preserved, there are distinct loci that differentiate tumor from normal.
We proceeded to identify these regions that are differentially methylated. The DMR calling algorithm used is essentially identical to the one described previously (see Material and Methods).Citation10 In total, 28% of the merged peaks were differentially methylated in one or more sample pairs ().
Figure 2. Differentially methylated regions. (A) Pie chart of the 32,9613 analyzed regions. For 9% of the regions hypermethylation and for 14% hypomethylation was observed in at least one patient; for 4% both hypo- and hyper-methylation was detected. (B) Bar graph of the frequency of detection of DMRs. We defined the “support” as the number of sample pairs in which a region was differentially methylated. (C) Hierarchical clustering was performed using the 3,155 high-confidence DMRs and a heatmap of the DNA methylation was made. Columns represent the individual samples; rows the DMRs. Red indicates high methylation; yellow low. Above the columns tumors are marked in red and normal samples in blue. (D) Stacked bar charts showing the distribution of the 329,613 analyzed regions over six genomic elements. The regions are binned by support. Note: the number of differentially methylated regions reduces with the level of support (). Only two hypermethylated regions were recurrent in 23 tumors; one hypomethylated region was recurrent in 10 tumors, and another region in 13 tumors.
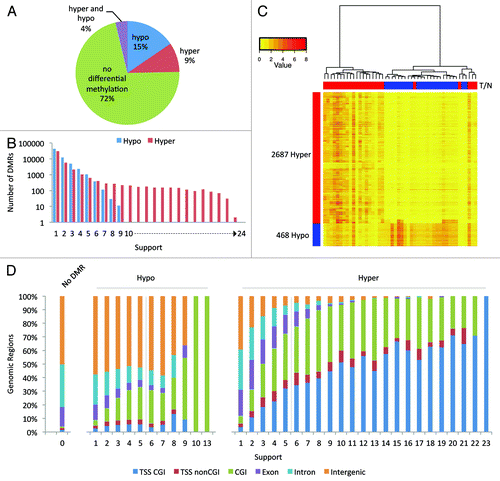
The number of DMRs identified per sample pair varied greatly (between 515 and 33,576) (Fig. S4B) without displaying a clear correlation between the number of DMRs and the available clinical data (Table S3), such as tumor grade or the presence of a mutation in the tumor suppressor gene KRAS (lowest p value = 0.33). Tumor purity and copy number aberrations could in theory affect the MethylCap-seq profiles and hence the number of DMRs; however, such data are not available. In 14 out of the 24 sample pairs more hypermethylated than hypomethylated regions were observed in the tumor; in the remaining nine pairs this was reversed. Especially, sample pair 41 shows many regions with hypomethylation in the tumor compared with the matched normal. A large fraction of these hypo DMRs are sample pair specific. Our quality control analyses revealed only a slight increase in median normalized read density for 41N as compared with others samples. This could be due to both biological and experimental factors, which cannot be address easily.
When combining all DMRs identified in the individual sample pairs it becomes apparent that many DMRs are patient-specific or occur in a small subset of the patients. Nevertheless, a handful of DMRs occur frequently in sample pairs: 184 DMRs are common to more than 19 samples. Furthermore, frequently occurring DMRs are predominantly hypermethylated in the tumors compared with normal tissue, whereas sporadic DMRs (e.g., as present in less than six sample pairs) can be either hyper- or hypo-methylated in the tumors ().
High-confidence colon cancer DMRs
To assess whether differential methylation at a given site is recurrent in CRC, we determined the “support” for all DMRs. We defined the “support” as the number of tumors in which a region was differentially methylated as compared with the matched normal tissues. As 24 samples were analyzed, the support values of hypermethylation for a given region are integer numbers between 0 and 24. We modeled the differential methylation events by Bernoulli trials and thus calculated the probability of a given support value based on a binomial distribution. We set a threshold for “high-confidence CRC DMRs” at probability ≤ 1 × 10−3, which corresponds to a minimum support of six. Consequently, a hypermethylated region was regarded with high-confidence if: (1) the support for hypermethylation of this region is at least six, and (2) the support for hypomethylation is at most one. The high-confidence hypomethylated regions in CRC were defined analogously. In total, 2,687 hypermethylated and 468 hypomethylated regions were selected (). shows 10 regions with the highest support for hypermethylation and Table S4 shows the full list.
Table 1. Top 10 of high-confidence DMRs. DMRs were sorted on support for hypermethylation and fold difference in expression between HCT116 WT and DKO cells
To confirm that the selected DMRs are also recurrent outside the analyzed 24 sample pairs, we performed MethylCap-seq on 5 independently obtained sample pairs. We find that 95% of the high-confidence hyper DMRs were a DMR in the additional samples. Specifically, all 184 DMRs that are common to at least 80% of the samples of the main set (support > 19) are also a DMR in at least 3 of the additional samples (Fig. S5).
Sample subgroup analysis based on DNA methylation profiles
Hierarchical clustering was performed to categorize the tumors into different methylation subgroups. Using the read densities for the most variable regions or the high-confidence hypermethylated regions we could not identify stable clusters that were enriched for a particular tumor grade, DUKES staging, microsatellite instability, or KRAS mutation. In addition, the elastic net method, which is a regularized regression method designed for genomic applications where the number of features greatly exceeds the number of analyzed samples,Citation13 was unable to identify a stable predictive pattern for the clinical properties. However, in the hierarchical clustering with the high-confidence hypermethylated regions, three tumors (2T, 31T and 33T) appeared to be different (Fig. S6A). These tumors are grade 3, wild type for KRAS, and two are microsatellite instable (MSI) (Table S3). No regions were found to be significantly characteristic for this group. This is partially due to the low ratio of samples to regions, which restricts the power of the applied Mann-Whitney U test followed by false discovery rate correction.
Several labs showed that a subset of the CRCs has relatively high methylation in specific regions in the genome. These CRCs are said to have the CpG island methylator phenotype (CIMP). Weisenberger et al.Citation14 developed a marker panel of five regions (CACNA1G, IGF2, NEUROG1, RUNX3 and SOCS1) to characterize CIMP. Strikingly, 31T and 33T had particularly high read densities at these regions. In addition, 44 of the 50 regions with the lowest p-values for differential methylation are CIMP-specific regions as determined by Xu et al.Citation6 (Fig. S6B). This indicates that these three samples could be CIMP.
Genomic distribution of DMRs
Analysis of the distribution of all DMRs over six genomic elements showed that DMRs occurring only in one or two tumors appear randomly distributed compared with those that occur more frequent (). Strikingly, the percentage of DMRs with hypermethylation in the tumor at CpG islands (CGIs) overlapping transcription start sites (TSSs) increases with the support for these DMRs. The regions that are hypermethylated in 1 tumor (n = 30,280) coincide for only 4% with TSSs with CGI, whereas for regions that are hypermethylated in 22 tumors (n = 31) this value rises to 71%. Furthermore, the two regions hypermethylated in 23 tumors also both coincide with a TSS with CGI.
The high-confidence hypermethylated regions coincide for 52% with CGIs with promoter and for 40% with CGIs without promoter (i.e., orphan CGIs), whereas this is only 5% and 24% for high-confidence hypomethylated regions (Fig. S7A and B). The overlap of the DMRs with the CGIs was analyzed in more detail: 90% of the high-confidence hypermethylated DMRs covered more than 50% of the CGI (Fig. S7B), and only 1% of the high-confidence hypermethylated or hypomethylated DMRs overlapped specifically with CGI shores (defined as the 2,000 kb region up- or downstream of a CGI, Fig. S7A and B).
Functional annotation
To gain insight into the biological functions that could be altered by hypermethylation, gene ontology analysis was performed with the web based tool DAVID.Citation15,Citation16 Analysis of the 1,667 genes with high-confidence hypermethylated DMRs over their promoter showed that the encoded proteins are enriched for localization at cellular membranes, and DNA binding and/or transcription factor activity (Table S5). Furthermore, there is enrichment of proteins that play a role in neuroactive ligand-receptor interactions, calcium signaling, and pathways in cancer (Table S5). Genes in cancer pathways include components of the Wnt-, MAPK-signaling pathway, or proteins with a role in interactions between cells and the extracellular matrix (ECM).
Examples of previously described, frequently hypermethylated genes are CDKN2A, SEPT9, VIM and CSPG2/VCAN. They were detected as hypermethylated in 9, 9, 17 and 20 tumors, respectively. Notably, CSPG2/VCAN was identified by Fernandez et al.Citation17 to be hypermethylated in CRC, and not in 29 other cancers analyzed in their study.
The most frequently hypermethylated regions (in 23 of the 24 tumors) overlap with SDC2, TLX1 and TLX1NB (). SDC2 is a transmembrane heparan sulfate proteoglycan that functions as a cell surface receptor interacting with ECM components and cell-to-cell signaling molecules such as growth factors.Citation18 TLX1 and TLX1NB are divergent genes, TLX1 (also known as HOX11) is a nuclear transcription factor important for development of the spleen during embryogenesis, specification of neuronal cell fates, and is associated with certain T-cell acute lymphoblastic leukemias.Citation19-Citation21 There is little knowledge about TLX1NB.
Figure 3. DMRs with the highest support. Two regions are hypermethylated in 23 of the 24 tumors. (A) Screenshots show that the regions overlap with the promoters of the genes TLX1, TLX1NB, and SDC2. (B) Strip charts with the MethylCap-seq read densities of all samples. The dotted line indicates the mean read density. (C) Bar charts for the RNA-seq results for HCT116 WT and DKO cells. For SDC2 the expression is 25× higher in the DKO compared with the WT; for TLX1 5×; for TLX1NB no sequence reads were obtained in WT and only few in the DKO.
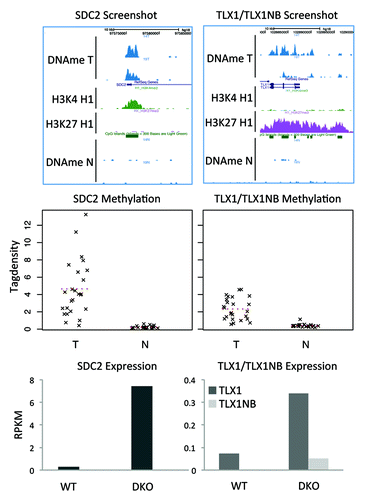
Analysis of HCT116 colon cancer cell lines
Next, we compared MethylCap-seq with RNA-seq and ChIP-seq profiles of H3K4me3 and H3K27me3 of the colon cancer tumor cell line HCT116 (HCT116 WT) and HCT116 with the DNA methyltransferases DNMT1 and DNMT3b knocked-out (HCT116 DKO).Citation22 These two cell lines are frequently used to analyze DNA methylation and its role in regulating gene expression in colon cancer. The regions frequently hypermethylated in tumors generally also displayed high methylation in HCT116 WT and reduced methylation in HCT116 DKO (Fig. S8A and B). Correlations between the support for hypermethylation and occupancy of the epigenetic marks were calculated (Fig. S8C) and intensity plots were made to visualize the occupancy of DNA methylation and the other marks in the HCT116 cells at the high-confidence DMRs (). The intensity plot of DNA methylation in HCT116 WT shows a clear positive correlation: the regions with the highest support have higher levels of DNA methylation compared with the lower support regions. Furthermore, the intensity plots show that H3K4me3 and H3K27me3 are somewhat depleted in the high-confidence hypermethylated regions in line with the antagonism between DNA methylation and H3K27me3 described for mouse embryonic stem cells and HCT116.Citation12
Figure 4. Correlation between HCT116 epigenetic marks and transcripts. (A) Color profiles of MethylCap-seq, H3K4me3, H3K27me3 and CTCF ChIP-seq and DNaseI hypersensitivity sequencing read densities from HCT116. Each profile shows the 10kb regions surrounding the high-confidence hypermethylated DMRs. The DMRs were sorted based on their support. The bar on the left shows the support (high support is red; low support is yellow). Average profiles are shown on top of the color profiles. (B) Density plot of the ratio of the RNA-seq data of HCT116 WT and DKO. The ratios of the RPKM values for DKO over WT are plotted for high-confidence (support hypermethylation ≥ 6; support hypomethylation ≤ 1), low-confidence (support hypermethylation < 6; support hypomethylation ≤ 1), and no DMRs (support hyper- and hypomerhylation = 0).
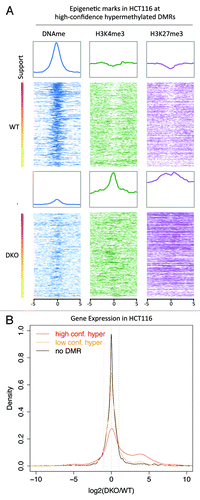
For differential expression analysis between HCT116 WT and DKO, sequence reads per kilo base of transcript per million mapped reads (RPKM values) were calculated. For 17% of all annotated genes the expression was more than 2-fold higher in the DKO compared with WT HCT116. We focused on transcripts with a DMR at their promoter. Transcripts that overlap with DMRs with high support tend to show a larger increase in expression in the DKO compared with WT HCT116 than in those with lower support (Fig. S8D). For 44% of the promoters frequently hypermethylated in CRC, expression of the associated gene was more than 2-fold higher in the DKO than in WT HCT116 (). ITGA4, for example, is frequently hypermethylated at its promoter in tumors (support 21) and is highly differentially expressed in DKO vs. WT (9-fold increase in DKO). The transcripts associated with the two DMRs with support 23 showed increased expression in the DKO of at least 4-fold ().
Hyper DMRs are bivalent in hESC
To further characterize the set of high-confidence hypermethylated DMRs, publically available data sets on epigenetic marks in the human embryonic stem cells H1 from the ENCODE project were mined.Citation23 The correlations between support for hypermethylation and occupancy of H3K4me3, H3K27me3, H3K36me3, Pol2, CTCF and DNaseI hypersensitive sites were assessed (Fig. S9A). We find a strong positive correlation between the support for hypermethylation and the repressive mark H3K27me3 in H1 ESC (Fig. S9A and B). H3K4me3 and DNaseI hypersensitivity, which are indicators for transcription/promoters and chromatin accessibility, respectively, also show positive correlations (Fig. S9A, C and D). In other words, regions frequently hypermethylated in CRC often show higher levels of these marks than regions sporadically hypermethylated.
To visualize the epigenetic patterns at the high-confidence DMRs intensity plots showing the occupancy of these six marks in H1 were generated (). We separated the regions into CGI with TSSs, orphan CGIs, and regions not overlapping with annotated CGI. A notable observation is that 70% of the high-confidence hypermethylated “orphan” CGIs display elevated H3K4me3, are DNaseI hypersensitive and lack H3K27me3 in H1 cells (; Fig. S10), suggesting that these regions are likely alternative or un-annotated TSSs, reinforcing the notion that hypermethylation in CRC is strongly biased toward promoters.
Figure 5. Overlap with bivalent regions in hESC. (A) Color profiles of H3K4me3, H3K27me3, H3K36me3, PolII, and CTCF ChIP-seq and DNaseI hypersensitivity sequencing read densities from hESC H1 (ENCODE). The DMRs are divided into regions overlapping with CGIs with TSS, regions overlapping with CGIs without TSS/Orphan CGIs, and regions not overlapping with CGIs. The regions were sorted on H3K27me3 density. (B) Pie chart showing the overlap of the high-confidence hypermethylated DMRs with H3K4me3 and H3K27me3 peaks from H1. For comparison a set of random regions obtained from the total set of peaks analyzed is included.
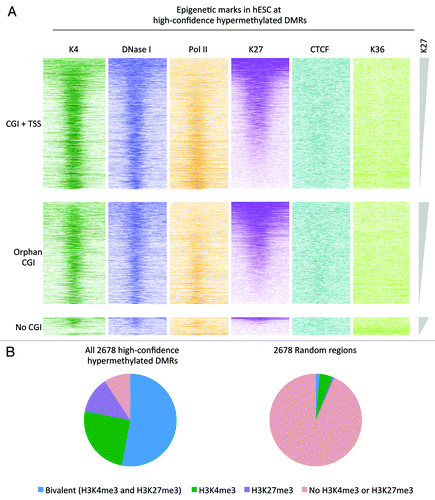
Furthermore, these plots show that the hypermethylated CRC DMRs overlap with regions with elevated H3K27me3 and H3K4me3 and are DNaseI hypersensitive in H1 cells, so-called bivalent regions ().Citation24,Citation25 This is confirmed with a comparison of peak tracks of H3K4me3 and H3K27me3 in H1 cells (called by MACSCitation26) and the high-confidence hypermethylated DMRs ().
Expression of the DMR-associated genes
In the absence of matching expression analysis, two publically available expression data sets (GSE25070 and GSE21815) were mined.Citation5,Citation27 The first data set consists of transcript levels of 26 CRC and 26 matched normal colon tissues obtained with Illumina beadchips.Citation5 From the second data set, obtained with Agilent arrays, transcript levels of 32 CRC and five normal colon tissues were analyzed.Citation27 The probes were linked to gene transcripts and subsequently to the MethylCap-seq peaks that overlap with promoters. Analysis was done on the mean expression level in tumor vs. normal.
For the data set by Hinoue et al.,Citation5 17,683 probes were linked to 16,215 regions of interest, corresponding to 13,545 genes. Interestingly, 73% of the probes that overlap with the high-confidence hypermethylated regions in tumors (corresponding to 75% of the genes) show an expression level below the median in normal colon (). This percentage is a relatively large fraction when compared with the probes linked to low-confidence hypermethylated DMRs or the probes without a DMR (). Thus, many genes that become hypermethylated in tumors were already silent or lowly expressed in normal colon.
Figure 6. Correlation with gene expression. Analysis of the publically available gene expression data from 26 CRC and matched normal colon samples (GSE25070). On the left data for the high-confidence hyper DMRs; in the middle low-confidence hyper DMRs; on the right regions without differential methylation. (A) Density plots of the mean expression level in normal samples. Dotted line indicates the median expression level calculated from all probes associated with a MethylCap-seq peak. These plots show that a large fraction of the high-confidence hypermethylated DMRs is lowly expressed in normal colon. (B) Scatter plots of the ratio of the mean expression of tumors and normal. Only probes that were expressed above median level in normal showed a more than 2-fold reduction in expression in tumor compared with normal. (C) Pie charts for probes with normal expression above median level. 13.3% of the selected probes that associated with the high-confidence hyper DMRs shows more than 2-fold lower mean expression in tumor compared with normal, whereas this is 4.7% and 4.2% for low-confidence hyper DMRs and peaks without differential methylation, respectively.
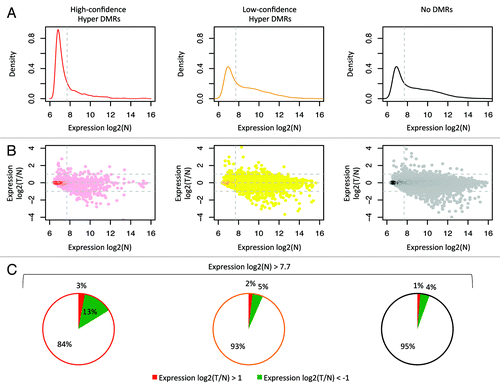
For 96% of all analyzed probes the mean expression level between tumor and normal differed less than 2-fold () and only probes that were expressed above median level in normal tissue showed a more than 2-fold reduction in expression in tumor (). Of the probes linked to high-confidence hypermethylated DMRs and with expression above median in normal, only 13.3% showed a more than 2-fold reduction in the mean expression level of tumor as compared with normal (). Analysis of the data set by Kogo et al.Citation27 produced similar results (Fig. S11).
Taken together, 35 genes that were linked to high-confidence hypermethylated DMRs had a 2-fold or higher loss of the mean expression in tumors compared with normal samples in both data sets (Table S6). DAVID analysis of these 35 genes showed that at least seven genes are involved in tissue morphogenesis—BMP2, EYA2, KLF4, LAMA1, RGMA, SLIT2 and TCF21 (Bonferroni adjusted p value = 8.8 × 10−4). The carbonic anhydrase IV gene (CA4) that is hypermethylated in 8/24 tumors, showed the highest reduction of expression in both data sets (about 90 and 20-fold, respectively). SFRP1, that encodes the secreted frizzled-related protein 1 and functions as modulator of Wnt signaling, was hypermethylated in 17/24 tumors and showed an approximate four and 7-fold reduction in expression. This gene was previously also reported as differentially methylated and expressed in CRC.Citation5,Citation28
Discussion
DNA methylation is strongly associated with cancer, and studies on DNA methylation are likely to shed light on the process of tumorigenesis as well as identify biomarkers. In this study, we used MethylCap-seq, a genome-wide DNA methylation capture method, to analyze differential DNA methylation in 24 CRCs and matched normal colon tissues. We show that the tumor and normal profiles can be clearly distinguished from one another (), and that DMRs are detected for each tumor compared with its matched normal sample. Applying a stringent cut-off, we identified 2,678 and 468 high-confidence hyper- and hypomethylated DMRs, respectively (). However, this approach and the limited cohort size did not allow us to identify statistically significant methylation signatures for subgroups of the analyzed tumor set.
The high-confidence hypermethylated DMRs overlap almost exclusively with CGIs and promoters (; Fig. S6 and S9), whereas the high-confidence hypomethylated DMRs are mainly focused in intragenic regions and not CGI shores (Fig. S7).
Classical DNA methylation analyses have shown overall hypomethylation in (colorectal) cancers, which occur at unique sequences, but especially at repetitive elements.Citation29-Citation31 To detect differentially methylated regions we focused in this study at regions for which we detect DNA methylation above the peak-calling threshold in at least one sample. We did not analyze global hypomethylation because MethylCap-seq is not the best method to study this. Mainly because MethylCap-seq, as well as other capture assays by means of MBD proteins, is biased toward regions with high methylated CpGs content, and hence regions with low methylated CpG density are covered less well.Citation9
The analysis of epigenetic marks in the human embryonic stem cells showed that hypermethylated CRC DMRs overlap for 53% with regions with elevated H3K27me3 (). Notably, hESC bivalent regions are enriched for developmental regulators,24,32 and silencing of these regulators by aberrant DNA methylation could contribute to cancer formation. Possibly, methylation factors are preferentially targeted to bivalent regions in cancer, and in this way reversible gene silencing in stem cells is replaced by permanent gene silencing in cancer.Citation33,Citation34
We performed an integrated analysis of our data with publically available expression data. Generally, we did not observe strong changes in expression between tumor and normal for the genes associated with the high-confidence hypermethylated DMRs (). Indeed, hypermethylation frequently occurred at genes not or lowly expressed in normal colon (). Other studies also reported marginal effects of aberrant DNA methylation on overall gene expression in cancer.Citation5,Citation33,Citation35 The question arises whether these hypermethylated regions are bystander/passengers or causative. It seems safe to assume that lowly expressed genes are “easier” to become methylated than actively transcribed genes, and this can result in sites of non-functional DNA methylation. However, it is also possible that methylation is an important mechanism that ensures a permanent inactive state (a hard switch). In line with this hypothesis, a small fraction of the genes that become hypermethylated in tumors and do not show differences in expression between tumor and normal, do gain expression in the HCT116 DKO compared with the corresponding WT HCT116 cells (). In the DKO cells maintenance of methylation is lost, and this suggests that DNA methylation is important to suppress the expression at these sites.
The majority of the identified high-confidence hypermethylated DMRs (~95%) co-localize with genes that are not known to be cancer-related. However, at least 9% of these regions are at the promoters of transcription factors. For example, seven different members of the Sox protein family (SOX1, SOX2, SOX5, SOX7, SOX11, SOX14 and SOX21) are targeted. It is well known that these and many other transcription factors are involved in development; they turn on/off genes that induce changes in cell morphology, proliferation, interaction, and movement. It is conceivable that deregulation of some of these transcription factors by hypermethylation could contribute to tumorigenesis. In addition, we also find frequent DMRs positioned at genes associated with the extracellular matrix, such as IGFBP3, MMP9, FBN2, NRCAM, NTNG1, GPNMB, GPC6, RELN, COL4 and ITGA4. Since the ECM affects adhesion, motility, viability, and proliferation, deregulation of this pathway could contribute to malignant transformation of colon cells. Functional assays for these genes, such as siRNA-mediated knockdown or overexpression in model cell lines, will be required to clarify their significance and could provide new insights in tumorigenesis.
The 184 DMRs occurring in over 80% of the tumors (support > 19) constitute potential biomarkers for CRC detection. The fact that hypermethylation of these regions was found in different subtypes of CRC suggests that hypermethylation at these sites may occur relatively early in tumorigenesis. Examples of genes that coincide with these DMRs are SDC2 and KCNK12. SDC2 is a cell surface receptor and appears to play diverse and possibly conflicting roles in cancer: SDC2 downregulation was associated with poor prognosis in esophageal squamous cell carcinoma,Citation36 whereas SDC2 overexpression was associated with poor prognosis in prostate cancer tissue.Citation37 KCNK12 encodes a member of the potassium channel superfamily, and was shown to be hypermethylated in 32 out of 77 colorectal tumors.Citation7 Analysis of the DMRs in a large independent cohort of CRC and preferably using an orthogonal analysis method should be performed to evaluate their frequency of hypermethylation in CRC, as well as their clinical value.
In summary, we have performed a comparative and comprehensive genome-wide DNA methylation analysis of 24 CRC and matched normal colon samples. We provide a resource containing genome-wide DNA methylation maps and comprehensive lists of DMRs. From our analyses, it is evident that hypermethylation in CRC does not only occur at tumor suppressor-like genes, but affects a wide spectrum of coding loci. This suggests that only a small fraction of the DMRs plays a direct role in tumorigenesis. Furthermore, this study corroborates and extends the intriguing link between promoter region hypermethylated in tumors and H3K27me3 in human embryonic stem cells.
Material and Methods
Patient samples
Twenty-four patients with primary sporadic CRC from the Fatebenefratelli Hospital in Benevento and five from the Catalan Institute of Oncology in Barcelona were included in this study. Each tumor sample was matched with adjacent apparently normal mucosa removed during the same surgery. The tumor samples were excised from the central part of the tumor mass, reducing the percentage of contaminating normal cells. The liquid nitrogen frozen specimens were micro-dissected and genomic DNA was isolated. Only sample pairs from which sufficient and good high molecular weight DNA was available were included in this study. Patients had no familial history of intestinal dysfunction or CRC. The study was performed according to the principles of the Declaration of Helsinki and approved by the Institutional Review Board of the Fatebenefratelli Hospital and the Catalan Institute of Oncology.
MethylCap-seq
Genomic DNA was sonicated to generate fragments of on average 400 bp. MethylCap was performed using the IP-STAR robot (Diagenode) as described before.Citation9 In short, 1 µg DNA was incubated with paramagnetic beads coated with the MBD domain of MeCP2 fused to GST. After washing with 200 mM, 400 mM and 500 mM NaCl, the bound methylated DNA was eluted in two fractions using 600 mM and 800 mM NaCl, respectively. The eluates were each prepared for Illumina sequencing according to Illumina's protocols. Sequence reads were generated on the Illumina Genome Analyzer IIx using a standard 36-base protocol. The total number of sequenced reads per sample is shown in Table S1.
Processing of MethylCap-seq data
Initial data processing, base calling, and alignment to the human genome (hg18) was done with Illumina software. Further data analysis was done using in-house generated scripts written in LINUX shell, Python, Perl and R. The uniquely mapped sequence reads were directionally extended to 234 bp, which was the estimated median length of MethylCap-seq DNA fragments. Uniquely mapped reads within satellite repeats were discarded. Mapped reads from the medium and high library were combined and the data was converted to Browser Extensible Data (BED) files for downstream analysis.
Enriched regions (peaks) were called on the basis of a Poisson distribution of overlapping sequence reads within a dynamic window. A false discovery rate (FDR) was calculated relative to the total covered sequence, and peaks with an FDR of ≤ 1 × 10−6 were selected. All peaks from the 48 samples were merged. Per sample the number of reads overlapping each region of interest was calculated and normalized by dividing by the total number of aligned reads of the sample, and the length of the peak.
Wiggle (WIG) files for viewing the data in the UCSC Genome Browser were generated. First, to compensate for differences in sequencing depth among samples, the total number of unique mapped reads of each sample was uniformly equalized relative to the sample with the lowest number of sequence reads. Next, for each base pair in the genome the number of overlapping sequence reads was determined and averaged over a 10 bp window.
Calling of differentially methylated regions
The DMR calling algorithm we applied is essentially identical to the one described previously.Citation10 We analyzed per sample pair each region and applied two criteria. First, we tested for statistically significant differences between the two samples using Fisher’s exact test. After executing Fisher’s exact test we performed multiple testing correction using the Benjamini-Hochberg algorithm and selected those regions for which the adjusted p value was lower than 0.01. Second, the difference in the normalized peak densities between tumor and normal should at least be 2-fold.
Validation with bisulfite sequencing
Genomic DNA was treated with sodium bisulfite using the EpiTect Kit (QIAGEN). The bisulfite-treated DNA was used for PCR (primers are listed in Table S7). The PCR products were isolated from gel, cloned into the pGEM-T vector (Promega), sequenced with the BigDye Terminator kit v1.1 (Life Technologies), and analyzed on a 3730(XL) DNA sequencer. Alignment and calculations were performed using the BiQ Analyzer tool.Citation38
ENCODE data
ChIP-seq data (H3K4me3, H3K27me3, H3K36me3, Pol2, CTCF) and DNaseI hypersensitivity data for hESC H1 was downloaded from http://hgdownload.cse.ucsc.edu/goldenPath/hg18/encodeDCC. See Table S8 for the complete links. The reads were extended to 200 bp, and BED files and WIG files were generated. The BED files were used to calculate the reads in and around regions of interest. In addition, peaks were called for H3K4me3 and H3K27me3 using MACS with mfold = 4 and p value = 1 × 10−14 and 1 × 10−6, respectively.
The annotation of the genomic features such as intergenic regions, exons, introns, CGIs were obtained from the UCSC Genome Browser.
Expression data
Two data sets GSE21815 and GSE25070 were downloaded from the Gene Expression Omnibus (www.ncbi.nlm.nih.gov/geo). GSE21815 contains data from Agilent arrays and GSE25070 data from Illumina Beadchips. These were normalized using the R package “limma” and “lumi,” respectively.
Analysis of HCT116 cells
The HCT116 DK0 cells were a gift from B. Vogelstein, John Hopkins Kimmel Cancer Center, Baltimore, MD. BJ.HCT116 WT and DKO cells were cultured in McCoy’s 5A medium supplemented with 10% fetal bovine serum and 1% penicillin/streptomycin (all Gibco/Invitrogen) at 37°C in 5% CO2 atmosphere. Genomic DNA was isolated with a standard protocol and MethylCap-seq was preformed as described above.
RNA isolation, ds-cDNA synthesis, chromatin harvesting and ChIPs were performed as described.Citation12,Citation39 The following antibodies were used: anti-H3K27me3 (Millipore, 07-449), anti-H3K4me3 (Diagenode).
ds-cDNA and ChIP DNA were prepared for Illumina sequencing according to Illumina's protocols. Sequence reads were generated on the Genome Analyzer IIx. Transcript expression levels were expressed as RPKM values (reads per kilo base of transcript per million mapped reads). The total number of sequenced reads, mapped reads or reads in transcripts are listed in Table S1.
Data access
The data generated for this work have been deposited in the NCBI Gene Expression Omnibus (GEO) (www.ncbi.nlm.nih.gov/ geo) and are accessible through GEO Series accession number GSE39068.
Abbreviations: | ||
CRC | = | colorectal cancer |
DMR | = | differentially methylated region |
BED | = | browser extensible data |
FDR | = | false discovery rate |
PCA | = | principal component analysis |
MSI | = | microsatellite instability |
CIMP | = | CpG island methylator phenotype |
CGI | = | CpG island |
TSS | = | transcription start site |
ECM | = | extracellular matrix |
RPKM | = | reads per kilo base of transcript per million mapped reads |
Additional material
Download Zip (1.4 MB)Acknowledgments
We thank E.M. Janssen-Megens, Y. Tan, and K.-J. Francoijs for technical support. This work was supported by the CancerDIP EU Collaborative project HEALTH-F2-2007-200620.
Disclosure of Potential Conflicts of Interest
No potential conflicts of interest were disclosed.
Financial Disclosures
CancerDIP EU Collaborative project HEALTH-F2-2007-200620, Breast Cancer Somatic Genetics Study [BASIS] HEALTH-2009-2.1.1-2-242006, Dutch Cancer Foundation (KWF) grant KUN 2008-4130.
Author Contributions
H.G.S., L.A., C.B., F.S. and A.B.B designed the study; L.S., V.C., A.V., D.H. and M.E. provided patient material; F.S., A.B.B. and F.M. acquired the data; A.K. provided technical support; F.S., A.B.B, Y.A. and H.G.S. analyzed and interpreted the data; F.S. and H.G.S. wrote the manuscript; A.B.B., Y.A., C.B. and T.L. provided advice and proofread the manuscript; H.G.S. supervised the study.
Supplemental Materials
Supplemental materials may be found here: www.landesbioscience.com/journals/epigenetics/article/22552
References
- Ferlay J, Shin H-R, Bray F, Forman D, Mathers C, Parkin DM. Estimates of worldwide burden of cancer in 2008: GLOBOCAN 2008. Int J Cancer 2010; 127:2893 - 917; http://dx.doi.org/10.1002/ijc.25516; PMID: 21351269
- Laird PW. Cancer epigenetics. Hum Mol Genet 2005; 14:Spec No 1 R65 - 76; http://dx.doi.org/10.1093/hmg/ddi113; PMID: 15809275
- Issa J-P. CpG island methylator phenotype in cancer. Nat Rev Cancer 2004; 4:988 - 93; http://dx.doi.org/10.1038/nrc1507; PMID: 15573120
- Rashid A, Issa J-PJ. CpG island methylation in gastroenterologic neoplasia: a maturing field. Gastroenterology 2004; 127:1578 - 88; http://dx.doi.org/10.1053/j.gastro.2004.09.007; PMID: 15521024
- Hinoue T, Weisenberger DJ, Lange CPE, Shen H, Byun HM, Van Den Berg D, et al. Genome-scale analysis of aberrant DNA methylation in colorectal cancer. Genome Res 2012; 22:271 - 82; http://dx.doi.org/10.1101/gr.117523.110; PMID: 21659424
- Xu Y, Hu B, Choi AJ, Gopalan B, Lee BH, Kalady MF, et al. Unique DNA methylome profiles in CpG island methylator phenotype colon cancers. Genome Res 2012; 22:283 - 91; http://dx.doi.org/10.1101/gr.122788.111; PMID: 21990380
- Kober P, Bujko M, Olędzki J, Tysarowski A, Siedlecki JA. Methyl-CpG binding column-based identification of nine genes hypermethylated in colorectal cancer. Mol Carcinog 2011; 50:846 - 56; http://dx.doi.org/10.1002/mc.20763; PMID: 21438024
- Øster B, Thorsen K, Lamy P, Wojdacz TK, Hansen LL, Birkenkamp-Demtröder K, et al. Identification and validation of highly frequent CpG island hypermethylation in colorectal adenomas and carcinomas. Int J Cancer 2011; 129:2855 - 66; http://dx.doi.org/10.1002/ijc.25951; PMID: 21400501
- Brinkman AB, Simmer F, Ma K, Kaan A, Zhu J, Stunnenberg HG. Whole-genome DNA methylation profiling using MethylCap-seq. Methods 2010; 52:232 - 6; http://dx.doi.org/10.1016/j.ymeth.2010.06.012; PMID: 20542119
- Bock C, Tomazou EM, Brinkman AB, Müller F, Simmer F, Gu H, et al. Quantitative comparison of genome-wide DNA methylation mapping technologies. Nat Biotechnol 2010; 28:1106 - 14; http://dx.doi.org/10.1038/nbt.1681; PMID: 20852634
- Bogdanovic O, Long SW, van Heeringen SJ, Brinkman AB, Gómez-Skarmeta JL, Stunnenberg HG, et al. Temporal uncoupling of the DNA methylome and transcriptional repression during embryogenesis. Genome Res 2011; 21:1313 - 27; http://dx.doi.org/10.1101/gr.114843.110; PMID: 21636662
- Brinkman AB, Gu H, Bartels SJJ, Zhang Y, Matarese F, Simmer F, et al. Sequential ChIP-bisulfite sequencing enables direct genome-scale investigation of chromatin and DNA methylation cross-talk. Genome Res 2012; 22:1128 - 38; http://dx.doi.org/10.1101/gr.133728.111; PMID: 22466170
- Friedman J, Hastie T, Tibshirani R. Regularization Paths for Generalized Linear Models via Coordinate Descent. J Stat Softw 2010; 33:1 - 22; PMID: 20808728
- Weisenberger DJ, Siegmund KD, Campan M, Young J, Long TI, Faasse MA, et al. CpG island methylator phenotype underlies sporadic microsatellite instability and is tightly associated with BRAF mutation in colorectal cancer. Nat Genet 2006; 38:787 - 93; http://dx.doi.org/10.1038/ng1834; PMID: 16804544
- Huang DW, Sherman BT, Lempicki RA. Systematic and integrative analysis of large gene lists using DAVID bioinformatics resources. Nat Protoc 2008; 4:44 - 57; http://dx.doi.org/10.1038/nprot.2008.211
- Huang W, Sherman BT, Lempicki RA. Bioinformatics enrichment tools: paths toward the comprehensive functional analysis of large gene lists. Nucleic Acids Res 2009; 37:1 - 13; http://dx.doi.org/10.1093/nar/gkn923; PMID: 19033363
- Fernandez AF, Assenov Y, Martin-Subero JI, Balint B, Siebert R, Taniguchi H, et al. A DNA methylation fingerprint of 1628 human samples. Genome Res 2012; 22:407 - 19; http://dx.doi.org/10.1101/gr.119867.110; PMID: 21613409
- Essner JJ, Chen E, Ekker SC. Syndecan-2. Int J Biochem Cell Biol 2006; 38:152 - 6; http://dx.doi.org/10.1016/j.biocel.2005.08.012; PMID: 16198615
- Hatano M, Roberts CW, Minden M, Crist WM, Korsmeyer SJ. Deregulation of a homeobox gene, HOX11, by the t(10;14) in T cell leukemia. Science 1991; 253:79 - 82; http://dx.doi.org/10.1126/science.1676542; PMID: 1676542
- Dear TN, Colledge WH, Carlton MB, Lavenir I, Larson T, Smith AJ, et al. The Hox11 gene is essential for cell survival during spleen development. Development 1995; 121:2909 - 15; PMID: 7555717
- Qian Y, Shirasawa S, Chen CL, Cheng L, Ma Q. Proper development of relay somatic sensory neurons and D2/D4 interneurons requires homeobox genes Rnx/Tlx-3 and Tlx-1. Genes Dev 2002; 16:1220 - 33; http://dx.doi.org/10.1101/gad.982802; PMID: 12023301
- Rhee I, Bachman KE, Park BH, Jair K-W, Yen R-WC, Schuebel KE, et al. DNMT1 and DNMT3b cooperate to silence genes in human cancer cells. Nature 2002; 416:552 - 6; http://dx.doi.org/10.1038/416552a; PMID: 11932749
- ENCODE Project Consortium. A user’s guide to the encyclopedia of DNA elements (ENCODE). PLoS Biol 2011; 9:e1001046; http://dx.doi.org/10.1371/journal.pbio.1001046; PMID: 21526222
- Bernstein BE, Mikkelsen TS, Xie X, Kamal M, Huebert DJ, Cuff J, et al. A bivalent chromatin structure marks key developmental genes in embryonic stem cells. Cell 2006; 125:315 - 26; http://dx.doi.org/10.1016/j.cell.2006.02.041; PMID: 16630819
- Shu W, Chen H, Bo X, Wang S. Genome-wide analysis of the relationships between DNaseI HS, histone modifications and gene expression reveals distinct modes of chromatin domains. Nucleic Acids Res 2011; 39:7428 - 43; http://dx.doi.org/10.1093/nar/gkr443; PMID: 21685456
- Zhang Y, Liu T, Meyer CA, Eeckhoute J, Johnson DS, Bernstein BE, et al. Model-based analysis of ChIP-Seq (MACS). Genome Biol 2008; 9:R137; http://dx.doi.org/10.1186/gb-2008-9-9-r137; PMID: 18798982
- Kogo R, Shimamura T, Mimori K, Kawahara K, Imoto S, Sudo T, et al. Long noncoding RNA HOTAIR regulates polycomb-dependent chromatin modification and is associated with poor prognosis in colorectal cancers. Cancer Res 2011; 71:6320 - 6; http://dx.doi.org/10.1158/0008-5472.CAN-11-1021; PMID: 21862635
- Baylin SB, Ohm JE. Epigenetic gene silencing in cancer - a mechanism for early oncogenic pathway addiction?. Nat Rev Cancer 2006; 6:107 - 16; http://dx.doi.org/10.1038/nrc1799; PMID: 16491070
- Feinberg AP, Vogelstein B. Hypomethylation distinguishes genes of some human cancers from their normal counterparts. Nature 1983; 301:89 - 92; http://dx.doi.org/10.1038/301089a0; PMID: 6185846
- Gama-Sosa MA, Slagel VA, Trewyn RW, Oxenhandler R, Kuo KC, Gehrke CW, et al. The 5-methylcytosine content of DNA from human tumors. Nucleic Acids Res 1983; 11:6883 - 94; http://dx.doi.org/10.1093/nar/11.19.6883; PMID: 6314264
- Ehrlich M. DNA methylation in cancer: too much, but also too little. Oncogene 2002; 21:5400 - 13; http://dx.doi.org/10.1038/sj.onc.1205651; PMID: 12154403
- Lee TI, Jenner RG, Boyer LA, Guenther MG, Levine SS, Kumar RM, et al. Control of developmental regulators by Polycomb in human embryonic stem cells. Cell 2006; 125:301 - 13; http://dx.doi.org/10.1016/j.cell.2006.02.043; PMID: 16630818
- Keshet I, Schlesinger Y, Farkash S, Rand E, Hecht M, Segal E, et al. Evidence for an instructive mechanism of de novo methylation in cancer cells. Nat Genet 2006; 38:149 - 53; http://dx.doi.org/10.1038/ng1719; PMID: 16444255
- Easwaran H, Johnstone SE, Van Neste L, Ohm J, Mosbruger T, Wang Q, et al. A DNA hypermethylation module for the stem/progenitor cell signature of cancer. Genome Res 2012; 22:837 - 49; http://dx.doi.org/10.1101/gr.131169.111; PMID: 22391556
- Sproul D, Nestor C, Culley J, Dickson JH, Dixon JM, Harrison DJ, et al. Transcriptionally repressed genes become aberrantly methylated and distinguish tumors of different lineages in breast cancer. Proc Natl Acad Sci U S A 2011; 108:4364 - 9; http://dx.doi.org/10.1073/pnas.1013224108; PMID: 21368160
- Huang X, Xiao D-W, Xu L-Y, Zhong H-J, Liao L-D, Xie Z-F, et al. Prognostic significance of altered expression of SDC2 and CYR61 in esophageal squamous cell carcinoma. Oncol Rep 2009; 21:1123 - 9; PMID: 19288017
- Popović A, Demirović A, Spajić B, Stimac G, Kruslin B, Tomas D. Expression and prognostic role of syndecan-2 in prostate cancer. Prostate Cancer Prostatic Dis 2010; 13:78 - 82; http://dx.doi.org/10.1038/pcan.2009.43; PMID: 19786981
- Bock C, Reither S, Mikeska T, Paulsen M, Walter J, Lengauer T. BiQ Analyzer: visualization and quality control for DNA methylation data from bisulfite sequencing. Bioinformatics 2005; 21:4067 - 8; http://dx.doi.org/10.1093/bioinformatics/bti652; PMID: 16141249
- Denissov S, van Driel M, Voit R, Hekkelman M, Hulsen T, Hernandez N, et al. Identification of novel functional TBP-binding sites and general factor repertoires. EMBO J 2007; 26:944 - 54; http://dx.doi.org/10.1038/sj.emboj.7601550; PMID: 17268553
- Irizarry RA, Ladd-Acosta C, Wen B, Wu A, Montano C, Onyango P, et al. The human colon cancer methylome shows similar hypo- and hypermethylation at conserved tissue-specific CpG island shores. Nat Genet 2009; 41:178 - 86
- Karpinski P, Ramsey D, Grzenieniak Z, Sasiadek MM, Blin N. The CpG island methylator phenotype correlates with long-range epigenetic silencing in the colorectal cancer. Molecular Cancer Research 2008; 6:585 - 91