Abstract
We have developed a method that enriches for methylated cytosines by capturing the fraction of bisulfite-treated DNA with unconverted cytosines. The method, called streptavidin bisulfite ligand methylation enrichment (SuBLiME), involves the specific labeling (using a biotin-labeled nucleotide ligand) of methylated cytosines in bisulfite-converted DNA. This step is then followed by affinity capture, using streptavidin-coupled magnetic beads. SuBLiME is highly adaptable and can be combined with deep sequencing library generation and/or genomic complexity-reduction. In this pilot study, we enriched methylated DNA from Csp6I-cut complexity-reduced genomes of colorectal cancer cell lines (HCT-116, HT-29 and SW-480) and normal blood leukocytes with the aim of discovering colorectal cancer biomarkers. Enriched libraries were sequenced with SOLiD-3 technology. In pairwise comparisons, we scored a total of 1,769 gene loci and 33 miRNA loci as differentially methylated between the cell lines and leukocytes. Of these, 516 loci were differently methylated in at least two promoter-proximal CpG sites over two discrete Csp6I fragments. Identified methylated gene loci were associated with anatomical development, differentiation and cell signaling. The data correlated with good agreement to a number of published colorectal cancer DNA methylation biomarkers and genomic data sets. SuBLiME is effective in the enrichment of methylated nucleic acid and in the detection of known and novel biomarkers.
Introduction
Methylation at the 5′ position of cytosines yielding 5-methyl-cytosine (5-mC) is an epigenetic mark that regulates the expression of genes.Citation1 DNA methylation is catalyzed by DNA methyltransferases,Citation2 is a dynamic processCitation3 and, along with other epigenetic regulators, guides differentiation and development.Citation1 While the large majority of cytosine methylation in vertebrate genomes is found in the context of symmetric CpG dinucleotides, methylation at CpNpG sites is also common in plant genomes and recent data has indicated the presence of significant non-CpG methylation in mammalian embryonic stem cells.
Presently, there is great interest in establishing correlations between phenotype and DNA methylation state and in discovering epigenetic biomarkers of disease. Most effort in studying and comparing methylomes has been directed toward identifying differentially methylated regions (DMRs) between human neoplastic and normal cells. It is well established that aberrant epigenetic regulation observed in cancer is manifested as global changes that alter chromatin packaging and localized changes at gene promoters, which influence the transcription of genes. Relative to normal cells, cancerous cells exhibit genome-wide hypomethylation in large blocks, juxtaposed with hypermethylation at select gene promoters.Citation4-Citation7 Aberrant methylation can drive carcinogenesis through the silencing of tumor suppressor genes and erosion of chromosome stability.Citation5,Citation8 Accordingly, there is much interest in detecting DMRs between normal and cancerous cells, as these might be driving cancer progression.
The current DNA methylation gold standard technique for quantifying methylation at nucleotide-base resolution across a genome is whole-genome bisulfite shotgun sequencing (WGBS). Unfortunately, WGBS is still prohibitively expensive for most laboratories, particularly when many replicates are required; significantly, a high fraction of reads contain no methylated cytosines. To reduce cost and/or enable analysis of higher sample numbers, typically some form of genomic complexity-reduction and/or DNA methylation enrichment procedure followed by microarray or deep sequencing is used.
Broadly, there are three main methods used to derive methylome data: bisulfite sequencing, affinity purification of methylated DNA and enzymatic restriction using enzymes with methylation sensitivity. While bisulfite conversion of unmethylated cytosines offers nucleotide level resolution of the methylome, it results in a loss of DNA “information content,” makes deep sequencing technologies more error-prone and effectively doubles the genome size—as Watson and Crick strands no longer are complementary. This poses challenges in the alignment of short bisulfite DNA reads back to large genomes, both from the loss of unique alignments and the large increase in alignment candidates per read. The computational burden can be reduced significantly with genomic complexity-reduction, usually implemented via restriction enzyme-based methods. In these instances, the methylome is sampled around restriction sites. For example, reduced representation bisulfite sequencing (RRBS) involves cutting DNA using the methylation-insensitive MspI, and then bisulfite-treating the DNA before sequencing the appropriately sized DNA fragments.Citation9 Restriction enzymes may also be used to directly enrich for regions of methylation, like in the modified methylation-specific digital karyotyping (MMSDK) method, where a combination of a methylation-sensitive mapping enzyme and a fragmenting enzyme is used.Citation10 Similarly, the HELP assay uses MspI and the methylation-sensitive isoschizomer HpaII.Citation11 More exotically, the use of the McrBC enzyme, which cuts at around two 5-mC sites preceded by purines and separated by:55–103 nt [RmC(N)55–103RmC], allows effective fractionation of the unmethylated component of the genome.Citation12 While restriction enzyme complexity-reduction methods are attractive for their simplicity, the fraction of the genome that may be assayed is rather inflexible and arbitrary.
Recently, a number of affinity-based methylation enrichment technologies have become popular, in particular, methyl-DNA immunoprecipitation (MeDIP) and MethylCap. The former makes use of antibodies specific for 5-mC while the latter exploits recombinant methyl-CpG binding domains for capture and affinity purification.Citation13,Citation14 The domains are derived from either human methyl-binding domain protein 2 (MBD2) or methyl-CpG binding protein 2 (MeCP2) or, in the case of the methylated CpG island recovery assay (MIRA), from a complex of methyl-binding domain protein 2b (MBD2b) and methyl-binding domain protein 3L1 (MBD3L1).Citation15-Citation17 Comparisons show MeDIP exhibits a smaller dynamic range than MethylCap.Citation18-Citation22 Enrichment methods reduce methylome resolution to the scale of the captured fragments, allow only relative estimates of methylation levels and are biased toward the capture of regions containing multiple methylated CpGs. However, enrichment methods that avoid bisulfite treatment are extremely advantageous in terms of cost and alleviate the alignment difficulties posed by bisulfite sequencing. Local smoothing, as implemented in the comprehensive high-throughput arrays for relative methylation (CHARM) method, also considerably improves enrichment data.Citation23 The recent discovery of 5-hmC in human DNA further complicates cytosine methylation quantification. MethylCap and MeDIP do not detect 5-hmC, while bisulfite DNA modification-based methods detect the aggregate amount of 5-mC and 5-hmC modifications.Citation24
Here we describe the streptavidin bisulfite ligand methylation enrichment (SuBLiME) method, a hybrid bisulfite sequencing and methylation enrichment technology that is also compatible with enzymatic complexity-reduction. SuBLiME offers the resolution of bisulfite sequencing with the added benefit of methylation enrichment and optional complexity-reduction to lower sequencing costs. The method involves the labeling of bisulfite-treated nucleic acid in the presence of biotin-labeled nucleotide triphosphate, so that biotin-labeled nucleotides are only incorporated at methylated sites. The single stranded bisulfite-treated nucleic acid may be labeled opposite 5-meC sites via primer extension in the presence of biotin-dGTP, or the strand may be converted to double-stranded material and this new strand copied using biotin-dCTP. DNA is enriched for methylation by capturing labeled material using streptavidin-coupled magnetic beads. Quantitative PCR assays of biotin-labeled spike-in controls show significant enrichment for biotin-labeled material on the magnetic beads. We applied SuBLiME technology to the study of differential DNA methylation between three colorectal cancer (CRC) cell lines and normal blood leukocyte DNA. In the pilot study, we also incorporated enzymatic complexity-reduction. This involved restricting libraries to regions adjacent to Csp6I (5′-G^TAC) sites, with one linker ligated to random-sheared ends and one to the restriction sites. Using 50-bp SOLiD-3 read technology, this allowed resolution of over 13% of genome-wide CpG sites.
In pairwise comparisons between the colorectal cancer cell lines and blood, we scored a total of 1,802 discrete gene loci as promoter-proximal differentially methylated regions (PP-DMR). Of these, 516 PP-DMRs were differentially methylated in at least two discrete promoter-proximal locations around Csp6I cut sites. In comparisons to 78 published colorectal cancer hypermethylation biomarkers, we found the SuBLiME method to be highly sensitive. Gene set enrichment and pathway analysis found the methylated gene loci to be associated with anatomical development, differentiation and cell signaling.
Results
Capture of biotinylated DNA
In principle, 5-mC-containing bisulfite-treated DNA can be labeled with biotin by either a direct or indirect method. In the first instance, a complementary strand is synthesized in the presence of biotin-dGTP, so that biotin-labeled nucleotides will only be incorporated opposite to unconverted cytosines. In the indirect method, the bisulfite-treated DNA first has a complementary strand synthesized, then this strand is, in turn, labeled in the presence of biotin-dCTP, so that the locations of biotin-dCTP incorporation correspond directly to the locations of non-conversion in the original bisulfite treated DNA. Since biotin-dCTP was more readily available at the time of method development, we chose to concentrate our efforts on the indirect method. In order to prepare enriched libraries of methylated DNA suitable for deep sequencing, we ligated modified SOLiD adaptors () using a custom protocol (outlined in ). While potentially applicable to all sequencing platforms, we developed SuBLiME technology using SOLiD sequencing, as linker sequences were readily amenable to necessary modification for our protocol (Table S1). While the SuBLiME method can be readily applied to the complete methylome, we introduced a complexity-reduction step by anchoring one end to Csp6I restriction sites. This enabled us to obtain sufficient depth of coverage with technical duplicates across the four DNA samples studied. We chose this particular enzyme as its restriction site is not GC rich and will not bias cutting to CpG islands, allowing inspection of a different subset of the methylome in comparison to methods such as RRBS or HELP.
Figure 1. (A) Modified oligonucleotide sequences of adaptors and (B) a schematic showing the particular implementation of SuBLiME biotin labeling used in this study with minor steps emitted for clarity. First, sheared and repaired genomic DNA (gDNA) has annealed adaptor 2 (P2-BtnA and P2-BtnB) ligated to the repaired and A-tailed ends of the gDNA (shown in green). Next, the DNA is cut with Csp6I before ligation with the annealed hemi-methylated adaptor 1 (P1-BtnAM and P1-BtnB), which contains an overhanging 5′-TA-3′ (shown in red). The gDNA is then bisulfite-treated and the denatured DNA primed opposite the ligated P2-BtnA oligomer (shown in blue) and strand extension allowed to complete. Unconverted cytosines in the original bisulfite-treated material are now guanines in the newly copied DNA, while the polymerase adds adenines opposite converted uracils. Next, the original bisulfite-converted strand is degraded before priming in the other direction complementary to the P1 adaptor end. To label the DNA, the primer P1-BtnA (blue) was extended by Taq polymerase in the presence of 100 µM biotin-14-dCTP and the unlabeled deoxynucleotide triphosphates dTTP, dATP and dGTP. Finally, labeled material was enriched using streptavidin-coupled magnetic beads. Note that by design P2-BtnB contains no guanosines so no biotinylated-CTP can be added in the linker region. Therefore biotin labeled dCTP should only be added at sites of bisulfite non-conversion of cytosines.
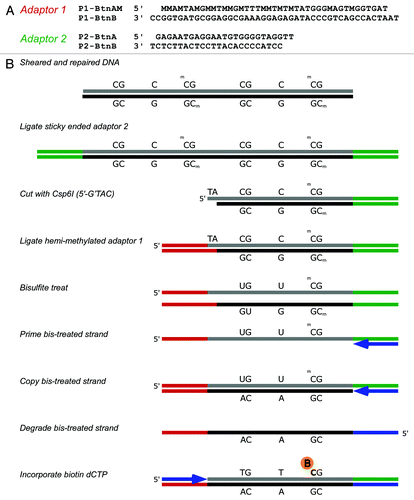
DNA was first sheared to a size of about 100–300 bp and ligated with modified adaptor P2 linkers, P2-BtnA and P2-BtnB (). The standard SOLiD adaptor P2 sequence contains only four cytosines in strand A. These were replaced with other nucleotides so that the modified P2 adaptor no longer contained any cytosines on the A strand (P2-BtnA and P2-BtnB). This is important for subsequent specific labeling in the presence of biotin dCTP. The linked DNA was then cut with the restriction enzyme Csp6I (5′-C’TAG) and ligated with a modified adaptor P1-BtnAM and P1-BtnB (). Here, in strand A of the adaptor (P1-BtnAM), cytosines were replaced by 5-mCs, so that they would resist modification during bisulfite treatment. This results in the formation of a directional library in which one end of each fragment (adaptor P2) is random and the end from which sequencing is initiated (adaptor P1) is located at a Csp6I site. The linked DNA fragments (~125–200 bp) were selected on an agarose gel before purification and reaction with sodium bisulfite under standard conditions. Strand B of adaptor P2 (P2-BtnB) was used to prime synthesis of the strand complementary to the bisulfite-treated DNA and the original DNA strand then degraded with USER enzyme mix. Following this, adaptor 1 strand A (P1-BtnA) was used to prime DNA synthesis in the presence of biotin-dCTP. Since the adaptor P2 sequence has been modified to remove cytosines from this strand, the incorporation of biotin only occurs at positions corresponding to methylated cytosines. DNA synthesis steps were done using Taq polymerase with extension at 65°C, so that strand-displacement synthesis would result in incorporation of biotin-dCTP into the complementary guanine-rich strand. After capture of the biotin-containing DNA using streptavidin magnetic beads, DNA was eluted and amplified for a minimal number of cycles using standard SOLiD linkers prior to 50 base deep sequencing using SOLiD-3 chemistry. This protocol results in the formation of a reduced-representation genomic library of bisulfite-treated DNA fragments, with sequence reads starting at Csp6I sites. Adaption of the SOLiD-based SuBLiME scheme to whole genomes is relatively straightforward and involves only the modification of the adaptor ends (Fig. S1A and B).
In order to demonstrate the specificity in the capture of methylated DNA, biotinylated and non-biotinylated oligonucleotide duplex controls were spiked into library preparations (Table S1). These controls demonstrated capture of about 2/3 of the biotinylated DNA in the streptavidin capture step, with < 1% contamination with non-biotinylated oligonucleotides (Fig. S2A and B). To examine the efficiency of the biotin-dCTP labeling step, we extended, under similar conditions, a HEX-labeled primer annealed to a model substrate. We found that biotin-labeled cytosine was added by Taq polymerase in a very robust fashion. The template contained 23 guanines, including some adjacent guanines, to potentially be labeled with biotin-dCTP. Full-length extension was obtained, even in the absence of a chase with non-biotinylated dCTP (Fig. S3A and B).
Within the SOLiD reads, analysis of cytosines outside of a CpG context demonstrated very efficient bisulfite cytosine conversion rate in the captured material (about 99.74%, assuming no methylation of CpH sites). Approximately 58.7% of reads contained a bisulfite-unconverted cytosine (). In unenriched material, the expectation is that 30.6% reads would contain non-converted cytosines at CpG sites, so the enriched library contained approximately twice the methylated reads expected in unenriched material. There are a number of reasons why all reads did not contain an unconverted cytosine, the foremost being that sequencing read only traverses the first 50 bp of the library fragments, which are approximately 140 bp in average size. Also, the SuBLiME method is sensitive to bisulfite non-conversion at CpH sites. Given the observed CpH non-conversion rate in the reads and the average library size, we predict over 10% of library material will have sites of non-conversion at CpH sites (for further discussion see Supplemental Materials).
Table 1. Complexity-reduction and enrichment statistics
Quality control, alignment, normalization and testing
The human build 19 genome contains a total of 5.05 M Csp6I sites, with approximately 4.93 M “well-spaced” Csp6I sites at least 70 bp distant to each other. Around these “well-spaced” sites, reads can align to 8.70 M locations. With enrichment for methylation, reads will align particularly to the 2.66 M locations containing at least 1 CpG site within 50 bp of the cut site. In total, 3.68 M discrete CpG sites (~13% of all CpGs in the genome) are covered by 50 bp sequencing around “well-spaced” Csp6I sites ().
Four biological samples with two technical replicates each were sequenced on one 8-partition slide using SOLiD-3 sequencing. A total of 158.04 M reads were produced. While the initial SOLiD sequencing ligations were of good quality (Fig. S4), the Csp6I cutting complexity-reduction step introduced problems with machine interpretation of the reads, which had to be corrected bioinformatically (Supplemental Materials). Repaired and pre-filtered reads were aligned with SHRiMP 1.3.2.Citation25 Reads mapped adjacent to 1.94 M discrete Csp6I cut sites with coverage (by at least one read across the eight samples) of 3.50 M CpG sites. The alignments and then Csp6I sites were post-filtered (with steps described in the Supplementary Methods and Results). We only considered “well-mapped” Csp6I sites, defined as those with greater than 50% of reads aligning uniquely and less than 10% of reads aligning randomly (; Fig. S5A and B). We also removed Csp6I cut fragments with the highest 0.1% count of aligned reads, which were enriched in certain repeats (Supplemental Materials and Fig. S6). Methylation rates were estimated by inspecting read sequence and recording the location of CpGs relative to the reference genome. After all the filtering steps, data was obtained for 2.35 M discrete CpG sites. Summed CpG site counts were normalized within the “edgeR” statistical packageCitation26 to derive a common library size of 8.843 M normalized counts before pairwise comparisons were made between the cancer cell line and leukocyte DNA CpG sites using an exact test (implemented in “edgeR”). Before each test, CpG sites without sufficient coverage were removed (). An overview of the DNA methylation enrichment and analysis procedures are presented diagrammatically in . Methylation data for each cancer cell line DNA was compared separately to normal leukocytes using three pairwise comparisons of normalized data. CpG sites were classified as a DMC if the evidence to reject the null hypothesis had a p value of 0.01 or less. The analysis was sufficiently powered for the detection of DMCs, as the three pairwise comparisons yielded 2.26- to 5.02-fold more DMCs (38,008 to 84,183 in pairwise comparisons) than expected by chance (). Interestingly, 10,513 of these DMCs were significant in all three pairwise comparisons. Methylation at CpH sites was also analyzed. The average methylation rate was very low (~7%) and there were only 26 DMC. In general, we noted that CpH methylation was enriched around genes but the sparseness of the data made conclusions difficult (see Supplemental Materials for a discussion).
Table 2. Bioinformatics and pairwise comparison statistics
Genome-wide distribution of methylation
To obtain a genome-wide overview, normalized methylation, sequenceable CpG sites and DMC data were grouped into 2 Mbp bins, averaged and plotted with Circos V0.52.Citation27 Relative to leukocytes, cell lines show regions of strong collective hypermethylation on chromosomes 8, 11, 13, 17, 19 and 20 (). Conversely, the q-arm of chromosome 18 and a region of chromosome 21 are particularly hypomethylated in cell lines. Coordinate silencing of large chromosomal regions is a known phenomenon in carcinogenesis.Citation28 Consistent hypermethylation of chromosome regions 11p and 17p in colorectal cancers has been noted some years ago.Citation29-Citation31 Hypermethylation at 17p is an early event that precedes the typical loss of heterozygosity observed in this region.Citation30 Interestingly, we note the extensive hypermethylation of the 8q24.21 region, which is known to be associated with colorectal cancer.Citation32 The widespread apparent 18q hypomethylation and 20q hypermethylation in the colorectal cancer cell lines relative to normal leukocytes is most likely due to alterations in chromosomal copy number. These regions are well documented to undergo loss of heterozygosity and duplication, respectively.Citation33 As most differentially methylated CpGs (DMCs) arise from instances of hypermethylation in tumors relative to normal tissue, the detection of DMCs is quite robust in the presence of copy number changes. There is a requirement for tumor DNA to be present and highly methylated to call a DMC. Often, high densities of DMCs were observed in pericentromeric and telomeric regions of chromosomes. Of particular interest, a number of 2 Mbp bins within the relatively copy number-stable q-arm of chromosome 19 showed high density of DMCs. We also considered the distribution within genome annotations. DMC, Csp6I cut site and “sequenceable” CpG site locations were aggregated across various annotations into densities per Mbp (). As DMCs can only arise from “observed CpGs” within “well-mapped” reads, there is a bias against detection of differential methylation in genomic regions with a high frequency of repeat sequences, such as introns. To account for this bias, the “sequenceable” CpG sites were subdivided into repeat and non-repeat DNA (as defined by RepeatMasker). There is a large variance in “sequenceable” CpG and DMC density between various genome annotations (). Introns have the lowest “sequenceable” CpG density (1,325 CpG per Mbp). This number is similar in the 5′ UTR, 3′ UTR or 2 kb downstream regions, but is half of that in the genic upstream region, 3-fold lower than in exons and over 6-fold lower than in CpG islands (CGIs), which show a density of 9,176 CpG per Mbp. The observed DMC density suggests that gene-proximal differential methylation between leukocytes and cancer cell lines is partially a function of CpG density, with CpG-rich areas having more DMC per Mbp. To inspect the relationship between CpG density and DMC density, the rate of DMCs per “sequenceable” CpG was derived. Exons and CGIs have a remarkably higher rate than other genic annotations (10.73% and 15.13% of “sequenceable” CpGs are DMCs, respectively). If the rate is adjusted to exclude counting “sequenceable” CpGs in repeat-region DNA, the richness of DMCs in exons and CGIs is still apparent (11.41% and 15.9%), suggesting these regions attract more epigenetic reprogramming in neoplastic cells. To further characterize exons and CGIs, the set of exons were partitioned into first, middle and last exons. Quite often, a CGI border may fall within the first exon, so that the set of first exons were again subdivided into regions within and outside CGI borders and into coding regions (between the coding start and the first splice site). Regions of first exons also contained within a CGI are remarkably rich in CpGs (11,982 CpGs/Mbp), twice the number observed for the whole first exon, and more than the average number for CGIs (9,176 CpGs/Mbp). First exon regions within a CGI also attract most of the epigenetic reprogramming, with 11.41% of “sequenceable” CpGs classified as differentially methylated. Interestingly, while the last exon has, on average, only half of the CpG density of middle exons, the percentage of DMC per CpG is relatively high. Therefore, these relatively few CpG sites, compared with other exons, are being epigenetically reprogrammed to a greater extent in cancer cells.
Figure 3. Methylation summary across the genome in 1 Mbp bins. Genome-wide data by chromosome are presented in a circle. The outermost track is a chromosome cytogenetic band ideogram with centromeres shaded in red. Heading inwards, the next track displays the difference in methylation of colorectal cell lines relative to normal leukocytes in a 10 color purple-green scale, with deep purple bins the most hypermethylated in colorectal cell lines. The next four tracks denote, from outermost in, the mean normalized methylated CpG per “sequenceable” CpG across normal leukocytes, HCT-116, HT-29 and SW-480. Data are presented in a 10 color red-yellow-blue scale with dark red and dark blue denoting hyper- and hypomethylation, respectively. The innermost histogram yields the ‘sequenceable’ CpG sites per bin (green) and a line graph (black) of CpG sites significant in at least two pairwise comparisons with normal leukocytes.
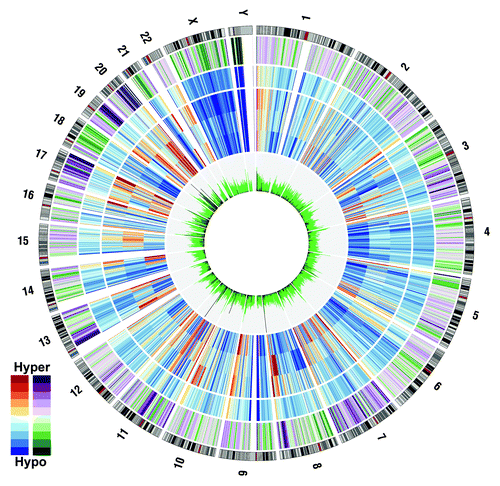
Table 3. CpG site and DMC density around Csp6I cut sites grouped by gene proximal annotations
Identification and ranking of putative biomarkers
To conserve power in the detection of putative new colorectal cancer biomarkers, we did not rank CpG sites by p value nor did we use multiplicity correction. As the data follows an exponential distribution and only two replicates were sequenced per biological sample, most of the normalized count difference between a given cell line and blood is by chance. With considerably more replicates in each comparison and with sufficient sequencing depth, ranking by p values is a more valid approach. Neighboring CpG sites are known to be coordinately methylated with autocorrelation sometimes observed across a number of kilobases.Citation34 Also, bisulfite sequencing data shows that the great majority of CpG sites have a rather bimodal methylation state: the CpG site is either almost completely methylated or unmethylated. This autocorrelation between CpG sites around a gene promoter was used to bioinformatically filter for potential new biomarkers using a “weight of evidence” approach. Essentially, CpG sites around Csp6I cut sites in promoter-proximal regions that showed significant differential methylation status in at least two of the three pairwise comparisons to blood were summed over all three cell lines. Promoter-proximal was defined as 2 kb upstream to 1 kb downstream of a gene locus cTSS or pre-miRNA start coordinate. This subset of gene loci was ordered by a weighted significance ratio, which is defined as the average number of DMC across the three cell lines. While this approach biases toward gene loci hypermethylated in all three cell lines with a higher number of methylated CpG sites around Csp6I cut sites, it allows confidence in downstream validation efforts and directs biomarker discovery toward CpG-rich promoters that are densely methylated in colorectal cell lines but not in blood. A total of 1,769 gene and 33 miRNA loci were scored as differentially methylated in this fashion (Table S2). Of these, 516 loci had at least two discrete Csp6I fragments contributing to observations (166 of the loci had at least three fragments contributing to observations, whereas 57 loci had at least four fragments).
Ideally, for a locus to be suitable as a plasma-based biomarker, no methylation should be observed in leukocytes. The 24 gene loci with no observed methylation in leukocytes and at least four Csp6I fragments are listed on . The top ranked marker, Ikaros family zinc finger protein 1 (IKZF1), has recently been reported as hypermethylated in a range of colorectal cancer cell lines and in ~64% of primary colorectal adenocarcinomas.Citation35 The other genes in the 1,769-member list contained both novel genes and genes previously identified in other studies as commonly hypermethylated in colorectal cancer.
Table 4. The 24 genes with promoter-proximal DMC, no observed methylation in blood and cut in at least 4 discrete places in the promoter-proximal region by Csp6I
Comparison to BeadChip Data
For comparison, we ran two of the cell lines, HCT-116 and HT-29, on Infinium HumanMethylation450 (450k) BeadChips. These BeadChips interrogate the methylation status of 485,577 CpGs in the human genome and are known to have high accuracy.Citation36 We compared SuBLiME normalized count data with BeadChip absolute methylation value estimates in the 45,774 instances where the same CpG site was examined by both methods. Comparison between SuBLiME-normalized read counts and BeadChip absolute DNA methylation levels showed a sigmoid relationship (Fig. S7). This is expected, as β values are logistic functions that are severely heteroscedastic outside the middle methylation range.Citation37 HT-29, the sample with the most reads, had a Pearson correlation coefficient of r = 0.49, while HCT-116 had a r = 0.45. These correlations would be expected to increase with increasing read depth. The correlation coefficients are comparable to the ones obtained using MeDIP (r = 0,56) and MethylCap (r = 0.49) in experiments with similar numbers of aligned reads.Citation19,Citation22,Citation38
Validation of promoter-proximal DMRs
Our comparison of cell lines with normal leukocytes is an indirect approach to biomarker screening; therefore, we validated the list of 1,769 differentially methylated loci as potential biomarkers. In particular, we sought to compare them with publicly available data on clinical samples. For this purpose, we considered data from two sources. In the first, a summary of analyzed Illumina Infinium HumanMethylation27 BeadChip data was available for the methylomes of 24 fresh frozen CRC compared with neighboring normal colon.Citation39 In this study, 627 DMCs spanning 513 genes were found. Of the 1,769 complexity-reduced SuBLiME genic PP-DMR biomarkers discovered, 1,026 have probes on the HumanMethylation27 BeadChip. Of the 513 genes differentially methylated in the Kibriya et al. study, 179 genes (35%) are common to the 1,026 PP-DMR genic biomarkers with probe coverage. Similar comparisons by Oster et al.Citation40 and Kim et al.Citation41 have also been made with shorter lists of genes differentially methylated in colorectal cancer and based on Infinium HumanMethylation27 BeadChip data.Citation40,Citation41 In all, 34 of 64 and 23 of 52 genes, respectively, identified in those studies were among those that we had also identified as differentially methylated in CRC.
The final comparison was with Illumina Infinium HumanMethylation450 BeadChip data publically available as part of The Cancer Genome Atlas (TCGA) project, from which the colorectal cancer component has recently been published.Citation42 We downloaded the raw BeadChip files from 254 colon adenocarcinomas and 38 matched normal colon tissues and compared them looking for differentially methylated CpG sites by using the moderated t-tests in the “limma” R-package.Citation43 In the model, we used cancer phenotype as the primary factor with gender as a covariate and a phenotype:gender interaction term. We observed particularly good concordance between the TCGA and SuBLiME data with a large intersection of genes considered as differentially methylated by the two approaches (Table S3 and Fig. S8). If we consider the 100 top ranked SuBLiME genic loci that also have BeadChip data, almost half of the CpG sites examined are significantly differentially methylated at an α level of 0.01 (1,172 of 2,527 CpG sites differentially methylated, or 46.4%). Over half (54) of the 100 top SuBLiME genic loci with array coverage have at least one CpG site with a log-fold change in the highest 1% of CpG sites in the TCGA data. This high concordance supports the validity in our strategy in comparing CRC cell lines to normal leukocytes. However, with such a scheme, some false positive gene loci will actually arise due to differences in the methylation pattern between colorectal adenocarcinoma and leukocyte cells. In the top 100 genic loci, the TCGA data suggests OTOP2, USH1G, ADAMTS15, TINAGL1 and GPC2 are possibly such markers (Table S3).
Combining the SuBLiME and TCGA data allows identification of exemplary potential blood-based biomarkers of CRC, i.e., those loci heavily methylated in clinical CRC tissue and CRC cell lines, but not in matched normal colon tissue and also having no methylation in normal leukocytes. By imposing a cut-off of at least 50% of CpG sites significantly methylated in clinical samples and at least one CpG site in the top 0.1% of all CpG sites ranked by log2-fold change, with no additional methylation observed in blood, we found IKZF1, ST8SIA1, FOXB1, EHD3, STSIA2, GRASP and DBX2 to be excellent biomarker candidates.
Comparison to biomarkers from the literature
A list of published colorectal cancer biomarker gene and miRNA loci was made by searching the literature with a particular emphasis on published methylation data on the HCT-116, HT-29 or SW-480 cell lines. The literature search identified data for 74 reported gene-proximal biomarkers hypermethylated in colorectal cancer that are covered by at least 5 CpG sites in the 500 bp upstream and downstream of the cTSS using Csp6I complexity-reduced SuBLiME method (Table S4). In addition, 4 miRNA loci that are hypermethylated in colorectal cancer are similarly covered in the 500 bp at either side of the pre-miRNA start coordinate. Per marker, 5–35 CpG sites are covered within 500 bp of the cTSS or pre-miRNA start coordinate with a total of 983 CpG sites covered in the complexity-reduced SuBLiME data. Given an α level of 0.01, the expectation is of about 10 false-positive results when making 983 tests. Comparisons were made between observed significant CpG sites and expected false positive significant CpG sites using Fisher exact tests (Table S5). Genes with promoters reported as methylated in CRC were readily detected by SuBLiME (p < 2.2e−16), while CpG sites known to be unmethylated were not significant across all three cell lines. A boxplot of normalized CpG counts within 500 bp to either side of the cTSS, grouped by cell line and previously reported methylation status, shows considerably more methylation in genes previously reported as methylated ().
Figure 4. Boxplot of normalized sum of methylation by cell line across the 500 bp proximal to the cTSS for published known methylated (M) and unmethylated (U) biomarkers.
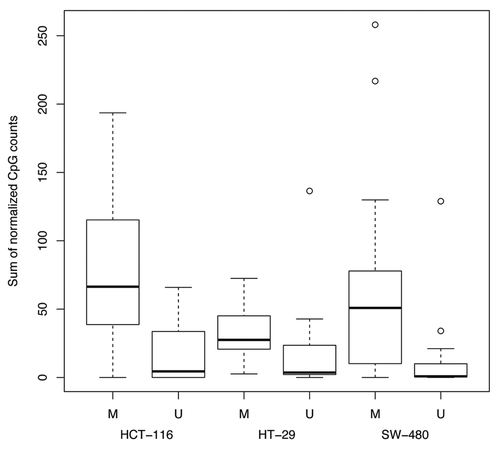
A total of 103, 105 and 144 CpG sites were significantly differentially methylated between normal leukocytes and HCT-116, HT-29 and SW-480 cell lines, respectively. SuBLiME was highly sensitive in the detection of known published biomarkers. When considering subsets of 43 and 24 loci with known methylation status in cell lines HCT-116 and SW-480, the number of CpGs showing significantly differential methylation was considerably higher than the number expected by chance (Table S5). However, the set of 9 known methylated loci in HT-29 was too small to call the frequencies of differentially methylated CpG sites as greater than by chance (p = 0.116). Generally, SuBLiME-normalized counts were in agreement with methylation states reported in the literature and, importantly, the exact test did not report more false positives than expected. Table S4 shows instances in which the normalized sum of CpGs around the TSS was high, suggestive of differential methylation; however, the rate of significance, using the statistical test, was low. The power to detect differentially methylated CpG sites within published hypermethylated biomarkers is a function of the depth of sequencing. By reducing the resolution and aggregating CpG sites together prior to the statistical test, more gene loci could be classified as differentially methylated.
Gene set analysis
Ingenuity pathway analysis (IPA) and the H-invitational DB enrichment analysis tool (HEAT)Citation44 were used to analyze the 820 genes with a weighted significance ratio ≥ 2. IPA analysis found 315 loci that were network eligible. The top five canonical pathways scored by IPA as overrepresented included G-protein coupled receptor signaling followed by cAMP-mediated signaling, human embryonic stem cell pluripotency, Wnt/β-catenin signaling and basal cell carcinoma signaling (Fig. S9). The top five biological functions of PP-DMR included organ development, tissue development, cellular development, nervous system development and function and organismal development (Fig. S10). A full list of results for canonical pathways and biological functions significantly overrepresented in this list of genes and gene networks with the highest significance scores, together with the genes present in these, are detailed in the Supplemental Material (Figs. S11–14 and Table S6).
The HGNC symbols of the 820 differentially methylated genes were also matched with the HEAT database tool, with matching possible for 708 symbols. The HEAT tool reported that, in most instances, the 708 silenced gene loci had promoters containing AP-2 α (TFAP2A) and Sp1 motifs, while approximately half had promoters with myeloid zinc finger-1 (MZF1), Krüppel-like factor 4 (Klf4), MafB or hypoxia-inducible factor 1 (HIF1A) motifs. About one third of the promoters contained aryl hydrocarbon receptor nuclear translocator (ARNT), Myc, paired box 5 (Pax5) or early growth response protein 1 (Egr1) motifs. With regards to cellular location, the methylated loci were highly enriched for gene products usually located in the nucleus, cell membrane, proteasome core complex or forming part of intermediate filaments.
Many gene loci that were methylated in the promoter-proximal region encoded for transcription factors and DNA binding proteins (e.g., homeobox and zinc finger gene). In addition, the genes encoding for frizzled, hormone receptor, winged helix-turn-helix, helix-loop-helix and forkhead domains also showed methylated promoters. Genes encoding for secreted Wnt pathway proteins were often methylated, including Wnt1, Wnt2b, Wnt3, Wnt pathway-interacting bone morphogenic proteins (BMP) 6 and 7 and Wnt antagonist secreted frizzled related proteins (SFRP) 2, 4 and 5. Notably, many other genes with secreted gene products were also methylated in their promoter-proximal region; these included promoters of the A disintegrin and metalloproteinase with thrombospondin motif (ADAMTS), matrix metallopeptidase (MMP) and fibroblast growth factor (FGF) families. Gene loci encoding membrane or transmembrane proteins were often silenced in the cancer cell lines [of note, G-protein coupled receptor class B, secretin receptor, solute carrier (SLC), sialyltransferase membrane protein, otopetrin, protocadherin (PCDH) and TMEM families].
As our comparisons were between cell lines and normal leukocytes, some differences in methylated genes could be due to differences in tissue type and not related to carcinogenesis. However, the high validation rate of PP-DMRs with other data suggests that, broadly, the gene set enrichment analysis findings are robust. Methylation of nine, ten or 11 genes in the top networks (networks 1, 2 and 4, respectively) has been directly observed in comparisons between CRC and normal colon tissue (Table S6), supporting our conclusions. Nevertheless, validation of network 3, with four genes shown to be differentially methylated in CRC and normal colon tissue is less well supported. With this caveat in mind, we also performed a HEAT analysis of published colorectal cancer methylated biomarkers (genes listed in Table S4). The results were similar to those of the SuBLiME PP-DMR biomarkers (data not shown). In summary, it seems that these colorectal cancer cell lines have severe hypermethylation of gene loci associated with regulation of anatomical development, differentiation, cell signaling, communication and environmental sensing.
Discussion
While whole-genome bisulfite sequencing offers exquisite resolution of the methylome, it is still prohibitively expensive, particularly if one wishes to sequence many samples. One frustrating aspect of the method is that many sequence reads do not actually traverse CpG sites and, thus, in most instances, they yield no information about the methylome. Methods that enrich for DNA fractions containing methylated cytosines prior to deep sequencing allow for the generation of more informative reads per library and thus reduce sequencing requirements and cost per sample. However, when using enrichment methods, concessions must be made (the data are typically sparser, less precise and more difficult to analyze). Existing enrichment methods such as MethylCap or MeDIP trade independence from bisulfite treatment for lack of resolution and inability to estimate absolute methylation. These methods also conflate cytosine methylation in any context (CpN) into one signal. MeDIP requires DNA to be single-stranded prior to enrichment, which creates biases against capturing high GC-content DNA and palindromic sequences due to DNA renaturation. Other restriction enzyme-based complexity-reduction approaches, such as RRBS, MMSDK and HELP, cover a rather arbitrary fraction of the methylome but offer advantages in alignment efficiency and sequencing cost per sample. When considering the features of SuBLiME with other common genome-wide methylation analysis methods, we find that the primary advantage of SuBLiME is the adaptability of the method and its lack of dependence on density and context of methylated cytosines (for an in-depth analysis see Table S7). SuBLiME was devised as a method that retains the nucleotide-level resolution of whole-genome bisulfite sequencing, is sensitive to cytosine methylation in a non-CpG context and can optionally be combined with complexity-reduction using restriction enzyme digestion or other means. In principle, bisulfite-treated nucleic acids of interest can be labeled directly using biotin-dCTP or the amplified bisulfite-treated DNA can be labeled with biotin-dGTP or biotin-dCTP. Furthermore, nucleotide triphosphates with attached ligands other than biotin can also be used. However, biotin labeling is a preferred option, as biotin-ligated nucleotide triphosphates are commercially available. Unlike other enrichment methods, SuBLiME is also adaptable for purposes other than the enrichment of DNA prior to deep sequencing, including the labeling and capture of bisulfite-treated RNA. SuBLiME can also be adapted to partition a pool of bisulfite-treated nucleic acid by labeling from random primers. The captured and un-captured material can then be assayed using methods such as conversion-specific PCR.
Due to the high efficiency of biotin capture, SuBLiME is suitable for the capture of DNA in which methylation is present in contexts other than CpG dinucleotides or when it is present at low levels. Antibody or methyl DNA binding protein methods are very sensitive to the density of methylation and not suited to such use. As with any bisulphite-based method, detection of methylated cytosines is limited by the background level of non-conversion of cytosine to uracil. However, SuBLiME still represents a valuable discovery tool in this context, as truly methylated sites should be seen above a background of scattered non-conversion, and can be subsequently validated.
In whole-genome bisulfite sequencing, a binomial estimate of methylation at a CpG site is made and proportion of methylation estimates are bounded between 0 and 1. SuBLiME is subject to the caveats of any enrichment method where only library material containing methylated cytosines is enriched. This leads to an unbounded metric and makes absolute methylation estimation difficult. Furthermore, with low coverage, it is difficult to determine unmethylated CpG sites: a lack of reads aligning across a CpG site may reflect either no, or little methylation, poor amplification of a region in library preparation or just chance alone. While aggregation of CpG site data can recover some power for differential methylation detection, we recommend the use of SuBLiME with higher read coverage and at least two replicates in each group, to allow for variance estimation. SuBLiME is ideally suited to pairwise differential methylation analysis. In studies seeking complexity-reduction but requiring absolute methylation estimates, a method such as RRBS is recommended. In this pilot study, we labeled bisulfite-treated DNA using biotin-dCTP and also incorporated a complexity-reduction step using Csp6I restriction enzyme digestion. This complexity-reduction step allowed more samples to be analyzed with the same amount of sequencing. However, it is relatively easy to adapt this scheme to whole-genome analysis. The standard SOLiD library construction protocol may be used, with a cytosine-free adaptor P2, such as was done in the present study, but generating a blunt ligatable end. We recommend the internal DNA fragment to be small in order to avoid enrichment of non-converted cytosines.
If Illumina 454 or Ion Torrent sequencing is desired, similar care is needed to ensure that the labeling of a sequencing adaptor does not occur. This can be accomplished by initially using short linkers in which one strand does not contain any cytosines. Following ligation, bisulphite treatment and primer extension to incorporate biotin dGTP or dCTP, the biotin-labeled fraction can be captured and libraries prepared using standard methods. With the availability of increasing read lengths, the loss of sequence information will not have major impact.
This study was focused on the discovery of novel colorectal cancer DNA methylation biomarkers with potential as plasma-based diagnostics. The DNA from three cancer cell lines was compared with normal leukocyte DNA to look for DNA hypermethylated exclusively in colorectal cancer. Our group has previously generated array-based methylome data on normal leukocytes and a number of colorectal tumors and matched normal clinical samples; therefore, we had other data to compare with the data generated by this approach. While the pilot study data was rather low coverage and, hence, rather noisy, comparison to HumanMethyation450 BeadChip data demonstrated that, on average, the read counts were proportional to the degree of CpG methylation at a given site. Compilation of methylation data around the cTSS compensated for the low read coverage. This study detected many differentially methylated genic PP-DMRs. Some differentially methylated genes were known, but many were novel.
We used several approaches to validate these PP-DMR, including comparison to differentially methylated gene lists based on 27K BeadChip data recently reported by others,Citation19,Citation22,Citation38 as well as with 450K BeadChip data from the TCGA consortiumCitation42. In each instance, we observed high concordance. We also applied these aggregated data to the analysis of biomarkers previously described in the literature. Again, SuBLiME proved to be highly sensitive in identifying differentially methylated markers. In summary, our validation approaches suggest that pairwise comparisons of colorectal cancer cell lines to normal leukocytes, instead of normal colon tissue, is a reasonable approach, as most DNA methylation changes are cancer-specific and not tissue-specific. This suggests that many of the PP-DMR findings will translate to clinical specimens. We are currently validating a select number of these biomarkers in clinical tissues.
Materials and Methods
Cell Culture and Genomic DNA isolation
HCT116 and HT-29 colon cancer cells were cultured in McCoy’s 5A media, while SW-480 colon cancer cells were cultured in DMEM/F12. All cells were supplemented with 10% fetal bovine serum. Genomic DNA was isolated using a Wizard® SV Genomic DNA isolation kit (Promega) as per manufacturer’s instructions. Blood leukocyte DNA was from a commercial source (Roche Applied Science). Purified genomic DNA was quantified with a Nanodrop ND-1000 (Thermo Scientific).
Library construction and bisulfite treatment
DNA was fragmented using a Bioruptor UCD-200 sonicator (Diagenode) in 300 µL of 10 mM Tris, 0.1 mM EDTA, pH 7.5 at a power setting of “high” for four 15 min intervals on ice, with alternating cycles of 30 sec “on” or “off.” The fragmented DNA was ethanol precipitated and resuspended before preparing two aliquots of DNA per biological sample, to create two technical replicates. Now each replicate was end repaired using the End-It™ DNA End-Repair Kit (Epicenter Biotechnologies), repurified with a MinElute reaction clean up kit (Qiagen), A-tailed using Klenow Exo- (NEB) in 200 µM dATP and repurified again with a MinElute reaction clean up kit. Ligation was then performed using a Quick Ligation™ kit (NEB) in the presence of 10-fold excess of adaptor P2-Btns P2-BtnA and P2-BtnB, as per the manufacturer’s instructions. The DNA was cleaned up with a QiaQUICK PCR purification kit (Qiagen), eluted in 50 µL of elution buffer (EB) and then digested overnight with 20 U of Csp6I (Fermentas) in buffer B. DNA was purified using a QiaQUICK Nucleotide Removal Kit (Qiagen) and ligated with Quick ligase in the presence of 10-fold excess of adaptor P1-BtnAM and P1-BtnB. The linked DNA was purified with an Agencourt AMPure XP kit (Beckman Coulter) before running the DNA on a 3% low-range agarose gel (Bio-Rad) and the region at ~125–200 bp was cut from the gel under blue light and re-purified using a Wizard® SV Gel extraction and PCR clean up kit (Promega). The DNA was then bisulfite-treated using a MethylEasy kit (Human Genetics Signatures) with 2 µg of DNA per tube.
Labeling and enrichment
Approximately 2 µg of eluted bisulfite-treated DNA library had the complementary strand synthesized by primer extension in Platinum® Taq buffer with 10 pmol of primer P2-BtnB in the presence of 50 µM dNTPs, 2.5 mM MgCl2 and 1 U Platinum® Taq (Invitrogen), with temperature cycling of 3 min at 94°C before 15 min at 65°C, then cooling to 4°C. Then 2.5 µL of 10x Antarctic phosphatase buffer was added and mixed before addition of 5 U Antarctic phosphatase (NEB) and further incubation for 30 min at 37°C to dephosphorylate unincorporated nucleotides, before heat denaturation at 65°C for 8 min and placing the tube on ice. To the 28.5 µL solution, 100 µM dTTP, dATP and dGTP (NEB) were added, along with biotin-14-dCTP (Invitrogen), 12.5 pmol of primer P1-BtnA, 2 U of USER enzyme mix (NEB), 1 U of Platinum® Taq and 10x Platinum® Taq buffer and double distilled water to make up the volume to 50 µL. The tube was first incubated at 37°C for 10 min to allow the USER enzyme mix degrade the uracil containing strand, before DNA denaturation at 94°C for 2 min, then extension at 65°C for 10 min. Finally, the tube lid was opened and 2.5 nmol unlabeled dCTP in 2.5 µL (1 mM) was added and mixed before closing the lid and allowing extension to continue for another 5 min before cooling the tube to 4°C. A QiaQUICK PCR purification kit was used to purify the DNA with elution in 200 µL of EB. Control oligonucleotides were added as described in the Supplemental Materials to half of the samples. 1.0 µg of labeled DNA was mixed with streptavidin-coupled M-270 Dynabeads® (Invitrogen) as per manufacturer’s instructions, excepting that an additional wash with a 100 µgmL−1 bovine serum albumin solution was used prior to addition of the labeled material. After final washes with double distilled water, a further 45 µL of water was added and the tube heated to 90°C for 2 min to elute captured DNA before placing the tube immediately on a magnet with aspiration of the supernatant as soon as the magnetic beads cleared from solution. The supernatant was stored at -20°C until use. Additionally, the first wash solution from samples containing the control duplexes was retained and DNA recovered in the presence of 25 µg GlycoBlue™ glycogen (Life Technologies) by ethanol precipitation and resuspension in 10 mM TRIS-HCl, 0.1 mM EDTA, pH 8.0 solution. Amplification and sequencing steps are described in the Supplementary Methods.
Bioinformatics and statistics
Bioinformatics and statistical analyses including alignment, repair, quality control, filtering, annotation, differential methylation tests and data aggregation is given in the Supplemental Materials.
Data access
The data discussed in this manuscript have been deposited in the Short Read Archive as submission SRA048724 and data given the accession numbers SRX112193 through SRX112196.
Abbreviations: | ||
5-mC | = | 5-methyl-cytosine |
CHARM | = | comprehensive high-throughput arrays for relative methylation |
DMC | = | Differentially methylated cytosine |
DMR | = | differentially methylated region |
HEAT | = | H-invitational DB enrichment analysis tool |
HELP | = | HpaII tiny fragment enrichment |
MeDIP | = | methyl-DNA immunoprecipitation |
MethylCap | = | Methyl-CpG binding domain affinity capture |
MIRA | = | methylated CpG island recovery assay |
MMSDK | = | modified methylation-specific digital karyotyping |
PP-DMR | = | promoter-proximal differentially methylated regions |
RRBS | = | reduced representation bisulfite sequencing |
SuBLiME | = | streptavidin bisulfite ligand methylation enrichment |
WGBS | = | whole-genome bisulfite shotgun sequencing |
Additional material
Download Zip (1.5 MB)Acknowledgments
J.P.R. and P.L.M. were supported by the CSIRO Transformational Biology Capability Platform and J.P.R., J.S. and P.L.M. by the CSIRO Preventative Health Flagship. Thanks to Julian Grusovin for technical assistance in the early stages of this project. Also thanks to Apru Khatri, David Midgley and Keith Rand for critically reviewing this manuscript, in particular to Apru for the suggestion and help in preparing .
Disclosure of Potential Conflicts of Interest
The authors declare the SuBLiME technology is subject to a patent application. The technology has been licensed to Active Motif. We would like to state that the manuscript has been seen and approved by all three authors.
References
- Jaenisch R, Bird A. Epigenetic regulation of gene expression: how the genome integrates intrinsic and environmental signals. Nat Genet 2003; 33:Suppl 245 - 54; http://dx.doi.org/10.1038/ng1089; PMID: 12610534
- Hermann A, Gowher H, Jeltsch A. Biochemistry and biology of mammalian DNA methyltransferases. Cell Mol Life Sci 2004; 61:2571 - 87; http://dx.doi.org/10.1007/s00018-004-4201-1; PMID: 15526163
- Kangaspeska S, Stride B, Métivier R, Polycarpou-Schwarz M, Ibberson D, Carmouche RP, et al. Transient cyclical methylation of promoter DNA. Nature 2008; 452:112 - 5; http://dx.doi.org/10.1038/nature06640; PMID: 18322535
- Feinberg AP, Ohlsson R, Henikoff S. The epigenetic progenitor origin of human cancer. Nat Rev Genet 2006; 7:21 - 33; http://dx.doi.org/10.1038/nrg1748; PMID: 16369569
- Ross JP, Rand KN, Molloy PL. Hypomethylation of repeated DNA sequences in cancer. Epigenomics 2010; 2:245 - 69; http://dx.doi.org/10.2217/epi.10.2; PMID: 22121873
- Ting AH, McGarvey KM, Baylin SB. The cancer epigenome--components and functional correlates. Genes Dev 2006; 20:3215 - 31; http://dx.doi.org/10.1101/gad.1464906; PMID: 17158741
- Hansen KD, Timp W, Bravo HC, Sabunciyan S, Langmead B, McDonald OG, et al. Increased methylation variation in epigenetic domains across cancer types. Nat Genet 2011; 43:768 - 75; http://dx.doi.org/10.1038/ng.865; PMID: 21706001
- Baylin SB. DNA methylation and gene silencing in cancer. Nat Clin Pract Oncol 2005; 2:Suppl 1 S4 - 11; http://dx.doi.org/10.1038/ncponc0354; PMID: 16341240
- Meissner A, Gnirke A, Bell GW, Ramsahoye B, Lander ES, Jaenisch R. Reduced representation bisulfite sequencing for comparative high-resolution DNA methylation analysis. Nucleic Acids Res 2005; 33:5868 - 77; http://dx.doi.org/10.1093/nar/gki901; PMID: 16224102
- Hu M, Yao J, Polyak K. Methylation-specific digital karyotyping. Nat Protoc 2006; 1:1621 - 36; http://dx.doi.org/10.1038/nprot.2006.278; PMID: 17406428
- Khulan B, Thompson RF, Ye K, Fazzari MJ, Suzuki M, Stasiek E, et al. Comparative isoschizomer profiling of cytosine methylation: the HELP assay. Genome Res 2006; 16:1046 - 55; http://dx.doi.org/10.1101/gr.5273806; PMID: 16809668
- Yamada Y, Watanabe H, Miura F, Soejima H, Uchiyama M, Iwasaka T, et al. A comprehensive analysis of allelic methylation status of CpG islands on human chromosome 21q. Genome Res 2004; 14:247 - 66; http://dx.doi.org/10.1101/gr.1351604; PMID: 14762061
- Down TA, Rakyan VK, Turner DJ, Flicek P, Li H, Kulesha E, et al. A Bayesian deconvolution strategy for immunoprecipitation-based DNA methylome analysis. Nat Biotechnol 2008; 26:779 - 85; http://dx.doi.org/10.1038/nbt1414; PMID: 18612301
- Weber M, Davies JJ, Wittig D, Oakeley EJ, Haase M, Lam WL, et al. Chromosome-wide and promoter-specific analyses identify sites of differential DNA methylation in normal and transformed human cells. Nat Genet 2005; 37:853 - 62; http://dx.doi.org/10.1038/ng1598; PMID: 16007088
- Brinkman AB, Simmer F, Ma K, Kaan A, Zhu J, Stunnenberg HG. Whole-genome DNA methylation profiling using MethylCap-seq. Methods 2010; 52:232 - 6; http://dx.doi.org/10.1016/j.ymeth.2010.06.012; PMID: 20542119
- Serre D, Lee BH, Ting AH. MBD-isolated Genome Sequencing provides a high-throughput and comprehensive survey of DNA methylation in the human genome. Nucleic Acids Res 2010; 38:391 - 9; http://dx.doi.org/10.1093/nar/gkp992; PMID: 19906696
- Rauch T, Pfeifer GP. Methylated-CpG island recovery assay: a new technique for the rapid detection of methylated-CpG islands in cancer. Lab Invest 2005; 85:1172 - 80; http://dx.doi.org/10.1038/labinvest.3700311; PMID: 16025148
- Brinkman AB, Simmer F, Ma K, Kaan A, Zhu J, Stunnenberg HG. Whole-genome DNA methylation profiling using MethylCap-seq. Methods 2010; 52:232 - 6; http://dx.doi.org/10.1016/j.ymeth.2010.06.012; PMID: 20542119
- Bock C, Tomazou EM, Brinkman AB, Müller F, Simmer F, Gu HC, et al. Quantitative comparison of genome-wide DNA methylation mapping technologies. Nat Biotechnol 2010; 28:1106 - 14; http://dx.doi.org/10.1038/nbt.1681; PMID: 20852634
- Nair SS, Coolen MW, Stirzaker C, Song JZ, Statham AL, Strbenac D, et al. Comparison of methyl-DNA immunoprecipitation (MeDIP) and methyl-CpG binding domain (MBD) protein capture for genome-wide DNA methylation analysis reveal CpG sequence coverage bias. Epigenetics 2011; 6:34 - 44; http://dx.doi.org/10.4161/epi.6.1.13313; PMID: 20818161
- Laird PW. Principles and challenges of genomewide DNA methylation analysis. Nat Rev Genet 2010; 11:191 - 203; http://dx.doi.org/10.1038/nrg2732; PMID: 20125086
- Harris RA, Wang T, Coarfa C, Nagarajan RP, Hong CB, Downey SL, et al. Comparison of sequencing-based methods to profile DNA methylation and identification of monoallelic epigenetic modifications. Nat Biotechnol 2010; 28:1097 - 105; http://dx.doi.org/10.1038/nbt.1682; PMID: 20852635
- Irizarry RA, Ladd-Acosta C, Carvalho B, Wu H, Brandenburg SA, Jeddeloh JA, et al. Comprehensive high-throughput arrays for relative methylation (CHARM). Genome Res 2008; 18:780 - 90; http://dx.doi.org/10.1101/gr.7301508; PMID: 18316654
- Jin S-G, Kadam S, Pfeifer GP. Examination of the specificity of DNA methylation profiling techniques towards 5-methylcytosine and 5-hydroxymethylcytosine. Nucleic Acids Res 2010; 38:e125; http://dx.doi.org/10.1093/nar/gkq223; PMID: 20371518
- Rumble SM, Lacroute P, Dalca AV, Fiume M, Sidow A, Brudno M. SHRiMP: accurate mapping of short color-space reads. PLoS Comput Biol 2009; 5:e1000386; http://dx.doi.org/10.1371/journal.pcbi.1000386; PMID: 19461883
- Robinson MD, McCarthy DJ, Smyth GK. edgeR: a Bioconductor package for differential expression analysis of digital gene expression data. Bioinformatics 2010; 26:139 - 40; http://dx.doi.org/10.1093/bioinformatics/btp616; PMID: 19910308
- Krzywinski M, Schein J, Birol I, Connors J, Gascoyne R, Horsman D, et al. Circos: an information aesthetic for comparative genomics. Genome Res 2009; 19:1639 - 45; http://dx.doi.org/10.1101/gr.092759.109; PMID: 19541911
- Clark SJ. Action at a distance: epigenetic silencing of large chromosomal regions in carcinogenesis. Hum Mol Genet 2007; 16:Spec No 1 R88 - 95; http://dx.doi.org/10.1093/hmg/ddm051; PMID: 17613553
- de Bustros A, Nelkin BD, Silverman A, Ehrlich G, Poiesz B, Baylin SB. The short arm of chromosome 11 is a “hot spot” for hypermethylation in human neoplasia. Proc Natl Acad Sci U S A 1988; 85:5693 - 7; http://dx.doi.org/10.1073/pnas.85.15.5693; PMID: 2840671
- Makos M, Nelkin BD, Lerman MI, Latif F, Zbar B, Baylin SB. Distinct hypermethylation patterns occur at altered chromosome loci in human lung and colon cancer. Proc Natl Acad Sci U S A 1992; 89:1929 - 33; http://dx.doi.org/10.1073/pnas.89.5.1929; PMID: 1347428
- Ribieras S, Song-Wang XG, Martin V, Lointier P, Frappart L, Dante R. Human breast and colon cancers exhibit alterations of DNA methylation patterns at several DNA segments on chromosomes 11p and 17p. J Cell Biochem 1994; 56:86 - 96; http://dx.doi.org/10.1002/jcb.240560113; PMID: 7806594
- Haerian MS, Baum L, Haerian BS. Association of 8q24.21 loci with the risk of colorectal cancer: a systematic review and meta-analysis. J Gastroenterol Hepatol 2011; 26:1475 - 84; http://dx.doi.org/10.1111/j.1440-1746.2011.06831.x; PMID: 21722176
- Migliore L, Migheli F, Spisni R, Coppedè F. Genetics, cytogenetics, and epigenetics of colorectal cancer. J Biomed Biotechnol 2011; 2011:792362; http://dx.doi.org/10.1155/2011/792362; PMID: 21490705
- Bell JT, Pai AA, Pickrell JK, Gaffney DJ, Pique-Regi R, Degner JF, et al. DNA methylation patterns associate with genetic and gene expression variation in HapMap cell lines. Genome Biol 2011; 12:R10; http://dx.doi.org/10.1186/gb-2011-12-1-r10; PMID: 21251332
- Javierre BM, Rodriguez-Ubreva J, Al-Shahrour F, Corominas M, Graña O, Ciudad L, et al. Long-range epigenetic silencing associates with deregulation of Ikaros targets in colorectal cancer cells. Mol Cancer Res 2011; 9:1139 - 51; http://dx.doi.org/10.1158/1541-7786.MCR-10-0515; PMID: 21737484
- Sandoval J, Heyn H, Moran S, Serra-Musach J, Pujana MA, Bibikova M, et al. Validation of a DNA methylation microarray for 450,000 CpG sites in the human genome. Epigenetics 2011; 6:692 - 702; http://dx.doi.org/10.4161/epi.6.6.16196; PMID: 21593595
- Du P, Zhang X, Huang CC, Jafari N, Kibbe WA, Hou L, et al. Comparison of Beta-value and M-value methods for quantifying methylation levels by microarray analysis. BMC Bioinformatics 2010; 11:587; http://dx.doi.org/10.1186/1471-2105-11-587; PMID: 21118553
- Gu H, Bock C, Mikkelsen TS, Jäger N, Smith ZD, Tomazou E, et al. Genome-scale DNA methylation mapping of clinical samples at single-nucleotide resolution. Nat Methods 2010; 7:133 - 6; http://dx.doi.org/10.1038/nmeth.1414; PMID: 20062050
- Kibriya MG, Raza M, Jasmine F, Roy S, Paul-Brutus R, Rahaman R, et al. A genome-wide DNA methylation study in colorectal carcinoma. BMC Med Genomics 2011; 4:50; http://dx.doi.org/10.1186/1755-8794-4-50; PMID: 21699707
- Oster B, Thorsen K, Lamy P, Wojdacz TK, Hansen LL, Birkenkamp-Demtröder K, et al. Identification and validation of highly frequent CpG island hypermethylation in colorectal adenomas and carcinomas. Int J Cancer 2011; 129:2855 - 66; http://dx.doi.org/10.1002/ijc.25951; PMID: 21400501
- Kim YH, Lee HC, Kim SY, Yeom YI, Ryu KJ, Min BH, et al. Epigenomic analysis of aberrantly methylated genes in colorectal cancer identifies genes commonly affected by epigenetic alterations. Ann Surg Oncol 2011; 18:2338 - 47; http://dx.doi.org/10.1245/s10434-011-1573-y; PMID: 21298349
- Cancer Genome Atlas Network. Comprehensive molecular characterization of human colon and rectal cancer. Nature 2012; 487:330 - 7; http://dx.doi.org/10.1038/nature11252; PMID: 22810696
- Smyth GK. Linear models and empirical bayes methods for assessing differential expression in microarray experiments. Stat Appl Genet Mol Biol 2004; 3:e3; http://dx.doi.org/10.2202/1544-6115.1027; PMID: 16646809
- Yamasaki C, Murakami K, Takeda J, Sato Y, Noda A, Sakate R, et al. H-InvDB in 2009: extended database and data mining resources for human genes and transcripts. Nucleic Acids Res 2010; 38:Database issue D626 - 32; http://dx.doi.org/10.1093/nar/gkp1020; PMID: 19933760